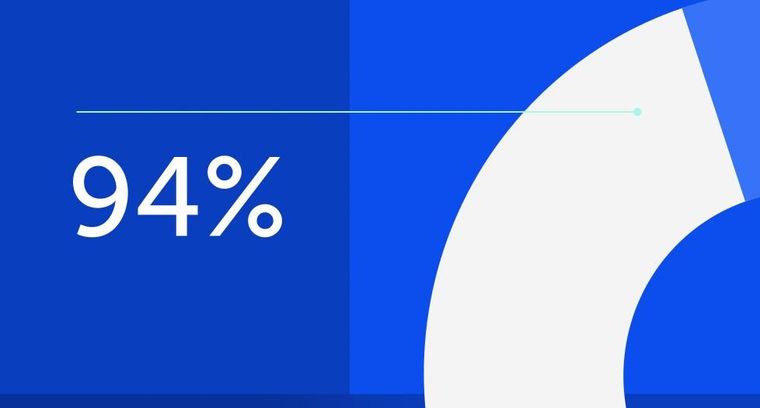
94% of researchers rate our articles as excellent or good
Learn more about the work of our research integrity team to safeguard the quality of each article we publish.
Find out more
METHODS article
Front. Polit. Sci., 06 January 2025
Sec. Political Science Methodologies
Volume 6 - 2024 | https://doi.org/10.3389/fpos.2024.1453640
This article is part of the Research TopicMethods in political science – Innovation & DevelopmentsView all 5 articles
We examine measurement concerns about computer-aided political event data in the state-of-the-art after 2015. The focus is on how to compare and quantify the mathematical and/or conceptual distance between what a machine codes/classifies from information describing an event and the actual circumstances of the event, or the ground truth. Three primary arguments are made: (1) It is important for users of event data to understand the measurement side of these data to avoid faulty inferences and make better decisions. (2) Avant-garde event data systems are still not free from some of the fundamental problems that plague legacy systems (investigated are theoretical and real-world examples of measurement issues, why they are problematic, how they are dealt with, and what is left to be desired even with newer systems). (3) One of the most crucial goals of event data science is to attain congruence between what is machine-coded/classified vs. the ground truth. To support these arguments, the literature is benchmarked against well-documented sources of measurement error. Guidance is provided on how to make performance comparisons within and across language models, identify opportunities to improve event data systems, and more articulately discuss and present findings in this area of research.
Political event data “record interactions among social and political actors” (Kim et al., 2019, p. 1) and are typically acquired from digital news reports as textual data.1 Event data users/researchers typically ask the question of “what entity (entities) did what to another (other) entity (entities)?” The traditional configuration for analyzing a single event is that there is a source, or “actor” (Halterman et al., 2023, p. 8), an action, and a target, or “recipient” (Halterman et al., 2023, p. 8).2 It is also important to ascertain where the event occurs (geolocation), how the main action(s) in the event is(are) carried out (modality), and the context of the event. Such key elements that comprise an event are known as attributes.
Event data and the systems utilized to process them have substantial, real-world implications. According to Parolin et al. (2022, p. 700):
Political and social scientists monitor, analyze and predict political unrest and violence, preventing (or mitigating) harm, and promoting the management of global conflict. They do so using event coder systems, which extract structured representations from news articles to design forecast models and event-driven continuous monitoring systems.
Beyond the community of political and social scientists, there are informational settings where text as data are objects of study and inference: digital journalism, information extraction/automated content extraction (ACE) in computer science, and semantic role labeling (SRL).3,4 Governments, policymakers, and practitioners are also interested in the application of text as data because it can assist with making data-driven decisions about foreign policy, human rights policies, civil war prevention, and the impacts of other factors (e.g., environmental or economic policies) on these issues.
The event data used in international relations and the causal and forecasting applications to civil conflict, protest, etc. are all based on extracting information from narrative (text) reports about these events. Such news, diplomatic, or human rights reports (among others) present multiple and information extraction and coding problems.5 Just as we have ontologies to classify the kinds of events in the news reports [e.g., (non-) violent protests, types of bombing attacks, and degrees of economic sanctions], the literature on the methodology of event data and text as data applied here does not include a taxonomy or well-discussed and vetted measurements for errors. Here, we review and present the current measurement and state-of-the-art around the information extraction, measurements, and coding of such news reports for event data. We address: How and to what degree of certainty can one assert that the attributes in an event coded/classified by a machine are properly assigned? What standards of measurement quality and validation are in use for capturing information about actors, events, locations, and other attributes of narratives of events? A way forward to better navigate measurement is also provided, which includes opportunities to improve on measurement, ideas for future research, and best practices.
Distance refers to how far off the information that a machine codes/classifies is from what is known as the ground truth, or what is actually detailed in the text describing an event. Distance can be measured in mathematical and conceptual terms. There are numerical performance metrics mentioned later of how well a machine extracts events and the attributes from text.6
Reducing distance to provide a faithful account of an event is the goal of machine coding of event data from news reports—starting first because humans can only do so much relative to the volume of text to be processed. Doing this consistently and at scale is what one wants from a machine, particularly when dealing with dynamic event attributes about actors, events, and targets. Two main aspects should be considered. First, coding actors and their roles—the sources and targets of events—may change. Second, details about the type or nature of an event action (verb) may change in reports or time. For each then there are different kinds of classification errors (time and identity-based for political actors who are sources and targets) and modality or types of events for the actions and statements they make.
For the first problem, major difficulties are that entities can be time- and politically-bound and associated with multiple aliases (Solaimani et al., 2017). Consider Barack Obama. What should he be coded as: “U.S. actor,” “U.S. Government,” “elite,” “community activist,” or a combination of these? Also, how far off is a machine on the distances between these labels? As another example, think about the last Shah of Iran, Mohammad Reza Pahlavi. Was he an Iranian elite? He was an official leader for one period and then not for another. Like Obama, he assumed different roles at different times. How should he be coded? These examples illustrate that labels matter because a goal of utilizing event data systems is to pinpoint exactly who a particular actor is at a particular moment in time. With the examples of Obama and Pahlavi, if a real-time event data system in use today were to code both as currently a “state leader,” the label would be misleading and inaccurate. These are just examples of established actors. To complicate matters, another goal for event data systems is that they should be able to identify new actors and do so in near- or real-time. Then, there are more questions: After the new actor becomes known, what else can be said about them? With which actors and groups are they associated and are they directly related to them or one or two hops away (i.e., what does their network profile look like)?
The second concern about the actions or event characteristics is one of modality, which is how an action is carried out. Actions differ by mode. Using an attack (a killing) as an example, to a computational linguist, a killing might just be a killing. To a social scientist, however, the nature of the killing matters tremendously (e.g., killing someone with a knife is a vastly different type of attack than killing someone with a rocket launcher). Modality factors into distance debates and scoring (discussed next) because how an action is defined impacts the determination of how far off the machine is from what it is intending to capture.
Scoring applies performance metrics to quantify distance. It assesses whether a machine is correctly coding or classifying actors and event entities (nouns or named entities recognition—NER) and actions (verbs and modalities of events). There are multiple numerical performance metrics of distance to be considered: Did one get an actor correct or identify them with the proper side or country in a conflict over time? Did the event modality properly reflect the nature of the event described, such as when is a “strike” a labor action vs. a military one?7 Of interest are measures of accuracy and precision in the NER and (multi-label) classification of events.
Since scores are computed for sentences, paragraphs, news reports, etc., the unit of analysis matters greatly. Event data systems often code events at the news report level, sentence level, or both (there are additional units discussed later). Whatever the scoring performance metrics, the unit is key for determining how well an event data system performs. For clarity, consistency, and comparison, here we use a taxonomy of units in which “news report” describes the superordinate category (this term is used to replace some authors' mentions of “news article,” “article,” “news document,” “document,” and “manifesto,” where it makes sense to do so). News report is followed by “news story” (a news report can consist of multiple news stories), “paragraph,” and “sentence” (the paragraph is the higher category except in the case of a single-sentence paragraph, which would make them the same). The “span” is also used, but it is not necessarily the lowest category because how authors specify its length could make it the same as the paragraph or sentence.
Scoring differences are also a concern when comparing a human domain expert with a machine. To a political scientist, “rebel separatists” are a distinct entity, but to an off-the-shelf large language model (LLM), “rebel” and “separatist” might be treated as distinct entities. This issue is general to natural language processing (NLP) methods and not just endemic to LLMs. It can happen with Term Frequency-Inverse Document Frequency (TF-IDF) and Bag of Words (BoW) approaches or from the improper stemming of words. Going back to accuracy and precision, the conjecture is that one can get more accuracy with NER and NLP tools, but domain-specific knowledge is needed for precision, which is a more important metric here because false positives have large consequences in event data research (e.g., incorrectly registering the assassination of a world leader that did not occur).
How should one quantify machine coding and classification of event data? Standard performance metrics (hereafter referred to as “metrics”), include accuracy, precision, recall, the F1 score, the variants of these metrics, and human-level decisions. Exact match accuracy and the F1 score are “the two standard performance metrics for question answering tasks” (Rajpurkar et al., 2018). As an example of these metrics for event data attributes, consider the following sentence that contains source, target, action, and geolocation:
Militants attacked U.S. soldiers in Syria
This could be coded with NLP, an event data coding program, or a language model (possibly as part of a larger paragraph or text). Here, the source (subject) is “Militants,” the target (object) is “U.S. soldiers,” the action (verb) is “attacked,” and the geolocation is “Syria.” Imagine that the researcher wants to determine how a model performs coding just the source.8 For the calculation, the following items are needed: a unit of analysis (the sentence), a ground truth (that “Militants” are the source of the event), and the coder or model's prediction (the coder or model predicts a source of “Militants”). The result is that the coder or model's prediction and the ground truth are congruent. To compute the metrics, a confusion matrix like that in Table 1, is the standard tool.
Starting with accuracy, the model correctly identifies the source in the sentence (and it makes one correct prediction out of one prediction made), and there are no true negatives, false positives, or false negatives, which means that the accuracy score for identifying the source is , which equals 1.0 or 100%. For precision, the model correctly predicts “Militants” as the source (there is one instance of a true positive), and it does not incorrectly predict another entity as the source (there is no instance of a false positive). The precision score for predicting the source is thus , which equals 1.0% or 100%. When the model predicts “Militants” as the source actor, it is correct every time. For recall, the model correctly identifies all instances of the source in the sentence (all occurrences of the true positive class), and because there are no false negatives, the resulting score is , which equals 1.0% or 100%. For F1, there is a true positive but no false positives or false negatives, so the computation is , which equals 1.0% or 100%.9 This exercise of applying a confusion matrix to the source can be repeated the same way for the target and geolocation.
The confusion matrix works well for a source, target, or geolocation (basically, did you get these attributes right or not?), but dealing with precision in particular for an action (verb) is more difficult because it is like a moving target (you either did or did not get an action, and then you need to apply a specific ontology and mode). The next sentence illustrates:
The prime minister attacked his opponent in the debate
Suppose a model correctly identifies the action (“attacked”), but mistakenly classifies the Political Language Ontology for Verifiable Event Records (PLOVER) quad-code (nature of the attack) as “material conflict” rather than “verbal conflict” (it misses the subtlety and context of the sentence). The result is a false negative which alters the related confusion matrix table and the associated metric computations.10
Distance debates and scoring errors can arise because of drop-off . This refers to any loss of fidelity and/or performance on certain tasks when going from human to machine (and vice versa). Humans are not perfect at coding event data, but neither are machines and there is still a risk of human-machine drop-off , which is any loss of coding/classifying ability when going from human expert annotations to machine annotations.11 In the most extreme cases, the annotation rules that a human devises are so complicated that a machine cannot currently implement them perfectly.
There are multiple sources and kinds of drop-off in performance and the one most commonly raised in the literature is related to languages. This matters a great deal in the political and the social sciences. In multilingual settings, translation drop-off is a major issue. This refers to a loss of translation fidelity when going from coding non-English corpora by native-speaking domain experts to relying on a machine translation infrastructure. Translation drop-off is therefore a type of human-machine drop-off. Currently, a machine coder utilizing a translation infrastructure to address non-English corpora will overall not be as accurate and precise as a human expert coding the same corpora natively, and one could expect greater training biases from relying solely on machines. Ho and Chan (2023) find that the drop-off for lower-resource languages is particularly large. Translation drop-off does not go away even with recent advancements such as Halterman et al. (2023)'s PLOVER and accompanying POLitical Event Classification, Attributes, and Types (POLECAT) dataset. The PLOVER/POLECAT system relies on translation technology from Google. While this approach may work well in the Political Instability Task Force (PITF) sphere, what if it is applied to the Centro de Investigación y Educación Popular (CINEP) event reports for Colombia written in Spanish? What about applying it to event reports from Francophone Africa? In these scenarios, there is not only a change in languages, but context and event classes also change. The abandonment of human coders is not a perfect pathway to solving the event extraction/encoding problem. Should a machine be penalized for certain characteristics inherent in a particular language? These considerations should be carefully contemplated when working with event data and LLMs in general.
Moving on from dictionaries, news archives, and just a few languages, some event data coding methodologies since Beieler et al. (2016) use transformer models, including the various Bidirectional Encoder Representations from Transformers (BERT), question-answering, and prompt-based models.12 Noteworthy is that the literature is rapidly evolving in multiple areas. There are many disparate things going on at once, such as researchers using different techniques, having varied purposes, and targeting different audiences.13 Assessing the literature chronologically is misleading for gauging progress, and the works are presented by which attribute(s) or problem/topic area they mainly address. Thus, we look at measurement from a methodological perspective, breaking down the contributions, findings, and open areas of inquiry.
With human-coded event data, actors are recorded in a dictionary that aligns them to the side or positions in political roles. This idea can then be extended to machine-coded event data using an electronic lookup of the actors (e.g., Norris et al., 2017). Extending this to new actors, actions, and languages, however, is labor- and intellectually-intensive (Osorio et al., 2019). Miller et al. (2022) argue that it is important to account for changing conflict dynamics when evaluating event data: Actors and environments are continually changing, which makes adaptability a much-needed characteristic of event data systems. Failing to recognize and address the varying conflict landscape (e.g., relying on older definitions and parameters to such a degree that it leads to the exclusion of new actors/actions) could result in conceptual bias, especially in situations where the objective of collecting event data is to account for broad trends in violence.
Alternatives to human coding of actors can broadly be placed into two groups. The first are those that mine past data to suggest new categories or groups of actors. The second are machine learning or transformer-related methods using BERT-like models.
In the first group, rather than rely on human coders for actor and political domain information, Solaimani et al. (2017) introduce the Recommend Political Actors In Real-time From News Websites (RePAIR) framework to identify new political actors and their roles (government vs. rebel) in real-time using suggestions from a “frequency-based actor ranking algorithm with alias actor grouping from news articles that also integrates an external knowledge-base (Wikipedia) to capture the timeline of an existing actor's role change and to suggest possible new roles” (p. 1333–1334).14 A major problem with manually adding actors and their roles to a dictionary is that an automated coder cannot identify new actors that are not in the dictionary, which results in coding error and drop-offs.15 RePAIR analyzes a sentence's semantic structure with an ACE method, then an algorithm is applied that relies on actor ranking by frequency. The most frequent new political actors are recommended over various time windows.
To identify actors in a corpus, Osorio et al. (2020) employ sparse parsing using the dictionary approach with the Hadath system, which is a supervised machine learning method that codes event data from Modern Standard Arabic news stories dealing with the Afghanistan conflict from 2008 to 2018. The actors list in the dictionary pertains to the conflict and is “based on knowledge of the case, available list of relevant actors made by country experts, and the discovery of additional actors” (p. 52) (the latter are identified with NER). The dictionary is comprised of over 300 named entities in Arabic that are “related to organizations or individuals including the main insurgent groups, coalition forces, international, and local actors relevant to the Afghan conflict” (p. 52). The heavy involvement of expert knowledge in the research can help with actor errors, but the work is still limited by sparse parsing technology.
Using machine learning approaches is more recent. Dai et al. (2022) produce structured, sentence-level political event records by applying a transformer model to unstructured text acquired by parsing the existing Conflict and Mediation Event Observations (CAMEO) and Python Engine for Text Resolution And Related Coding Hierarchy (PETRARCH) dictionaries (i.e., synthetic news stories are generated from coded events). The sentences are constructed by taking random actors, agents, and synonyms, and substituting them into the placeholders in the CAMEO dictionaries. The news stories are then used for training data (the test data are also synthetic), so dictionaries and hand-labeling are not required. This eliminates human dictionary updating and can lead to updated events about new actions and actors. They then perform source and target coding. It is not stated how actor errors are reduced, but utilizing the CAMEO and PETRARCH dictionaries for training data and including negative samples might attenuate these errors.
Subsequent approaches adopt more fully these transformer, machine learning methods. Parolin et al. (2022)'s Multilingual Multi-Task Learning BERT for Coding Political Event Data (Multi-CoPED) is capable of source and target detection. Preparing the system to identify these attributes is part of sequence labeling: Each word in the sentence of interest receives a tag denoting what type of word it is, and tags are assigned to sources and targets. Hu et al. (2022)'s work with ConfliBERT involves applying the CAMEO ontology for sources and targets labeling using a dataset made with corpora from the politics domain. The domain-specific training of the model should help with minimizing actor errors. Halterman et al. (2023) use automated Wikipedia (offline version) lookups for entity resolution, which it is argued helps with identifying present and past actors quickly. Wikipedia is used in tandem with a version of CAMEO's agents file that is expanded to allow for references to actors that are more general in nature [e.g., “soldiers” (p. 16)]. To identify/code entities, their POLECAT relies on a flexible, supervised machine learning process. To minimize errors, human experts provide “hundreds of positive and negative labels using an active learning-directed semi-random sample of politically relevant news articles” (p. 12) for each PLOVER category. These labels are used as inputs for POLECAT's machine learning (ML) classifiers. A criticism is that while relying on Wikipedia may be a step forward from what was done before the state-of-the-art (SOTA) literature reviewed here, it is still an imperfect approach because not every entity has a Wikipedia page and there are lag times for pages to be established.16
Another factor that can lead to errors with actors has to do with the transliteration of names. For example, if dealing with a human entity, how do we know for certain that we have the right person instead of someone else with the same or similar name?17 There are opportunities to improve in this area, and it is just one of the many difficulties that event data systems face with actor recognition.
The category under which a specific action in an event gets classified is an action class, and there are generally many within a particular ontology.18 Action classes answer the question of what? in the who did what to whom? structure. While CAMEO is a common framework for data coding, it is open to additions and revision to new areas of inquiry or topics (e.g., drug and gang violence) and by new events or their attributes.
Osorio et al. (2020) employ a sparse parsing approach using dictionaries to identify events in a corpus with Hadath. To construct the dictionary, the first step is to implement part-of-speech tagging on the text data to create an initial inventory of verbs. Subsequently, human annotators sift through this inventory, assessing the verbs' pertinence to the Afghan conflict. The annotators then introduce different variants and synonyms of each verb to account for all verb conjugations. To filter out irrelevant news reports, six human coders classify each news report manually as relevant or not based on an explicit mention of a conflict-related event that took place in Afghanistan. Accepted news reports present factual incidents and describe an event about “acts of violence, provision of governance in the context of war, or traditional conflict mitigation” (p. 51). Excluded are opinion pieces, news stories filled with general commentary, and summary reports. Upon evaluation of intercoder reliability, half of the coders' classifications are unreliable. To ameliorate the problem, a machine learning text classifier trained using the labels provided by the coders who exhibit the highest level of agreement is deployed.
Addressing new ontology or action extensions has been done via (1) upsampling, (2) natural language inference (NLI), and (3) zero-shot prompts to automate and lower the cost of action and mode classification and extension. Halterman and Radford (2021) introduce a dataset and task to automate the process of “upsampling” (p. 1), which is going from coarse labels to fine-grained labels or spans of information. The work only attempts to detect the size of a protest (number of attendees), but the approach is probably extensible to other attributes. Using language inference, Lefebvre and Stoehr (2022) propose PR-ENT, a flexible and unsupervised event-coding model that relies on prompting and textual entailment. The approach is described as few-shot, and the event-coding system is comprised of PR-ENT and an interactive codebook. PR-ENT relies on two major steps to code events: The first is masking then prompting a pre-trained cloze language model to predict the missing verb(s). The second is for a human to select from these answer candidates, and then map the selected answer to its corresponding event type.19 It is found that the accuracy of PR-ENT remains competitive despite being efficient and flexible, and that precision is much greater than just employing prompting by itself. Involving a human expert in the event-coding process is one way to mitigate event classification errors. The model relies on the Armed Conflict Location and Event Data (ACLED) dataset, and the sample is comprised of 3,000 training events and 1,000 testing events for Africa. The distribution of event types in the sample mirrors that of the entire dataset.
Hu et al. (2022)'s ConfliBERT model is used for multi-class event classification on a few datasets.20 For this task and depending on the dataset, the model is either used to categorize several types of terrorist attacks, police activities, or the CAMEO ontology is applied to perform pentacode classification. ConfliBERT is also used to perform multi-label classification on a couple of datasets.21 The model is either used to predict types of attacks initiated by terrorist organizations or to predict multiple crime categories stemming from organized criminal activity. It is found that for these experiments, ConfliBERT's overall performance exceeds that of BERT models trained on generic corpora (and when taking in limited training data). It is argued that the performance gap between these models is a consequence of training ConfliBERT on information that allows it to absorb the unique characteristics inherent in the target domain (which has its own nomenclature, distinct semantics, and stylistic elements of language) vs. training on information indiscriminately. The model is trained on news reports from “United Nations' websites and databases, international humanitarian nongovernmental organizations, think tanks, and government sources such as the Foreign Relations of the United States” (p. 5472), and news reports from Gigaword, the Phoenix Real-Time data, Wikipedia, and 35 global news agencies. For the initial step of classifying and filtering news reports in the sample, binary classification tasks are performed at the news report and sentence levels.
Parolin et al. (2022)'s Multi-CoPED is used to code the CAMEO pentacode that corresponds with the primary conflict action in a sentence. Multi-CoPED uses the Multi-Task Learning BERT model (MTL-BERT) for action detection instead of action repositories from CAMEO and “instead of resorting to the lexico-syntactic patterns from CAMEO (like PETRARCH coders do)” (p. 704). MTL-BERT is comprised of a contextualized word embeddings extractor that uses BERT, a source and target detector, and an action detector. The research uses newswire data crawled from a selection of different global news agencies. Domain-specific news is retained, and out-of-domain news is pre-processed and filtered using the metadata information. What remains is the data used for training and validation exercises (3,728 total sentences, with 2,207 of them in English and 1,521 in Spanish). To handle the multilingual aspect of the event coding, MTL-BERT is initialized using the weights from a BERT multilingual pre-trained model.
Dai et al. (2022) code/classify events at the root-code level for CAMEO's 20 event types and complete set of finer-grained 295 action codes. The research analyzes sentences that are no more than 30 tokens in length. It is noted that this length introduces a bias into the model in favor of coding sentences that are shorter and simpler, and that future works should allow longer sequence lengths. It is not entirely clear how event errors are avoided, but the reliance on the CAMEO and PETRARCH dictionaries for training data might help because they are highly domain-specific. Also, the inclusion of negative samples in the training data at the very least helps with reducing false positives. For the model, relevant samples are selected in the form of news stories represented at the sentence-level: four million samples for training, 40,000 for validation, and 40,000 for testing (the ratio of positive to negative samples is 39 to one, respectively, with one sample equal to roughly one sentence). To aid in selecting relevant samples, randomly-drawn negative samples from The New York Times are incorporated into the model so that it learns to not provide coded events when there are no reported events. It is found that the model “only fails to code events for 15 input samples that contain events and erroneously codes events for 53 samples that should not contain events” (p. 3), but it is argued that this result might be more attributable to differences in the synthetic samples vs. those taken from The New York Times, rather than the strength of the model, as synthetic samples “often fail to sufficiently mimic their real world targets” (p. 3).
Halterman et al. (2023)'s PLOVER/POLECAT relies on DistilBERT (Sanh et al., 2019), a variant of the BERT model, to extract event types from news reports. As mentioned, the system relies on humans to label event types within individual news stories as part of its pipeline, and the coders do this for every event type. The complete process of initial machine filtering for PLOVER is not explicitly detailed, but it involves using search strings set forth by the PITF to filter Factiva for politically-relevant news reports in Arabic, Chinese, English, French, Portuguese, Russian, and Spanish. Some of the news reports are sourced from the ICEWS project's news corpus and cover the past two decades. To have sufficient samples of news reports for each event type-mode combination and the entity labeling work performed, a combination of these real-world news reports and synthetically-generated ones is used. For the human aspect of the PLOVER pipeline, roughly 10 expert coders are employed for labeling. News reports are presented to coders in a semi-random manner employing active learning “to continuously update selected stories for coding based upon an underlying machine learning model” (p. 14). Their approach of harvesting reports in multiple languages and converting them to English for processing in DistilBERT (trained in English) for POLECAT is in contrast to Hu et al. (2022)'s ConfliBERT approach. ConfliBERT is trained separately with data in English, Spanish, and Arabic (but has not been implemented cross-language yet). For ConfliBERT, data are harvested in native languages and training and coding are performed in native languages in ConfliBERT flavors/languages. Future work could thoroughly compare the two approaches and determine if any step(s) generated errors on the event data.
Hu et al. (2024)'s Zero-Shot fine-grained relation classification model for PLOVER ontology (ZSP) approach works by leveraging a tree-query framework to deconstruct the task of political event ontology relation classification into three dimensions: context, modality, and class disambiguation. It is argued that accounting for these dimensions is especially useful for classifying events characterized by modality (e.g., past or future) or hypothetical aspects, which event data systems that do not incorporate these dimensions into their pipeline have difficulty coding. Working alongside the tree-query framework is an NLI model pre-trained on the PLOVER codebook, which is expert-written and contains annotation rules and instructions. The task performed in the research is relation classification, and events are classified in a source-target (or actor-recipient) pair using the PLOVER ontology and the knowledge accessed via the NLI-based ZSP model, with each pair receiving a PLOVER code. A benefit of using a zero-shot approach is that external labeled data, which can be costly in terms of time and money, are not required.
Past event data coding applications typically took the location as with the source, target, or some combination of the two to determine the location of the event. Yet this is not appropriate in the case of sub-national and cross-border political interactions. More recent methods have been adopted to address the errors around determining the location of an event.22 Halterman (2017) employs a langauge agnostic word2vec framework (via a SpaCy framework) to learn locations in texts. In parallel, Imani et al. (2017) (in English) and Imani et al. (2019) (in Arabic and Spanish) employ automatic geolocation extraction to news reports. The major hurdle is to determine the precise location of an event in a news report when there are other location candidates mentioned in the same news report. Imani et al. (2017) refer to the collection of locations directly linked to an event as “focus locations” (p. 1956). A single event can only take place at a single location, which is referred to as the “primary focus location” (p. 1956). The process of identifying the primary focus location involves applying an NER tool (Stanford CoreNLP) to extract potential location named entities from the first few sentences of the training news report. Then, semantic characteristics are extracted from the location-containing sentences using the word2vec model and sentence embedding techniques.23 Lastly, the classifier is trained on labeled training examples (binary; sentences include a focus location or they do not) and is used to predict the precise location of an event within unlabeled test sentences. To find the primary location of interest among candidate locations from the grouping of focus sentences for each news report, the location name that appears the most is selected. An issue with Imani et al. (2017) and Imani et al. (2019) is that they only retain the news reports for which locations are correctly parsed by various NERs (including Stanford and MITIE). The New York Times dataset is also used, but only news reports that have certain keywords in their title are selected because not all of the annotated locations in this dataset are candidate locations. Note that the data are already pre-selected to filter to political events, and that a major problem with this approach is that it leads to sample selection bias. The difficult-to-handle cases should also be included in the analysis.
Osorio et al. (2020)'s Hadath system employs sparse parsing using dictionaries to identify locations in a corpus. To mitigate the risk of location errors (specifically, false positives), Hadath uses a locations filter to ensure that whatever location is identified is actually a physical location. The dictionary upon which Hadath relies is comprised of nuances that enable it to differentiate the city of Kabul from Kabul Street, for example. Hadath's output database provides daily data that are georeferenced and district-level; however, the system searches exclusively for a toponym, either a province or district in Afghanistan, within any line of text that contains an actor or action. It is argued that the potential issue with this approach is that there are many instances in which locations are not mentioned in the paragraph from which an event is extracted because the beginning of the news report is where the location of an event is frequently specified.
Halterman et al. (2023)'s POLECAT does not rely on bylines to determine geolocation; this information is acquired from the news story. Even when events do not have a clearly identifiable, associated location, they do not get coded as having taken place in the city mentioned in the byline. POLECAT does, however, have the capacity to record such events and many events without an accompanying location are retained. This approach helps it get around some of the issues with geolocation that legacy systems face when they code from bylines, such as when event locations are incorrectly assigned to country capitals because of where the news story is filed or when they are incorrectly assigned to the location of the news source's headquarters (Halterman et al., 2023). One potential consequence of this approach is the undercoding of locations.
Before some of the more recent literature, the standard way to determine the date that an event took place was to parse the byline of a news report to obtain its publication date (Halterman et al., 2023), which is the method used by Osorio et al. (2020). The issue with this approach is that it is prone to errors. Miller et al. (2022) argue that researchers should be wary of date resolution errors, such as when an historical event shows up in the news on its anniversary date (e.g., if a news report about the anniversary of the 1941 Japanese attack on Pearl Harbor is published with today's date, it could appear to a machine that the attack recently happened). To better discern publication date from event-reported date, Halterman et al. (2023) use DistilBERT for the extraction of event types from a news story, and then apply RoBERTa-QA (Liu et al., 2019) to recover the date for each event type.
Other sources of event data error come from reports being translated and from the complex interactions of case and news report selection and simultaneous needs to code events (with or without machines). More recent approaches try to reduce the accumulated linguistic, sample selection, and aforementioned discrete coding problems (actors, actions, locations, and dates). Machine learning technology is frequently applied to address these issues primarily because of its efficiency.
With Osorio et al. (2020)'s Hadath system, after curating relevant news stories with a classifier, NLP [sparse parsing technology (Schrodt, 2001)] codes the text along five feature categories: source actor, target actor, action, date, and daily, district-level location.
Other approaches move to the newer BERT approach. Parolin et al. (2022)'s Multi-CoPED codes in English, Portuguese, and Spanish into a structured format like the CAMEO event data. This approach eschews human coding and employs contextual knowledge from BERT models and multi-task learning. Using a small training set, their model produces high quality results coding event sources, actions, and targets. This lowers the cost for multilingual parsing with good performance, helping to overcome some of the major limitations of legacy systems. Multi-CoPED's MTL-BERT model outperforms all baseline models on English, Spanish, and Portuguese data in terms of exact match F1, partial match F1, and macro F1 for source and target detection, and standard F1 and macro F1 for action detection (pentacodes).24 The Multi-CoPED system outperforms all baseline models on precision, recall, and F1 (standard) for the end-to-end coding (source, target, action, and overall) of CAMEO data. For action detection, MTL-BERT trained on English and Spanish data achieves an absolute macro F1 performance increase of 25.9% over PETRARCH for testing in English and 31.9% over UPETRARCH for testing in Spanish. For end-to-end coding, Multi-CoPED trained on English and Spanish data achieves an absolute overall F1 performance increase of 23.3% over PETRARCH2 for testing in English and 30.7% over UPETRARCH for testing in Spanish.
Hu et al. (2022)'s pre-training of ConfliBERT on a large domain-specific corpora (33.7 GB) allows it to excel in terms of standard F1, example F1, and macro F1 over a standard BERT on all tasks25 involving nine different datasets covering various topic areas.26 In particular, one of the ConfliBERT configurations gains an absolute macro F1 performance increase of 5.03% on binary classification in the violence domain and 4.38% on TS multi-class classification in the protest domain, while another configuration gains 2.96% on NER in the defense domain.
To the traditional features of an event in who did what to whom?, Halterman et al. (2023)'s PLOVER/POLECAT accounts for mode and context. The POLECAT dataset is fully automated and consists of machine-coded event data produced from millions of news reports written in various languages, and the data are intended to cover 2010 to the present. Instead of using actor and event dictionaries, the data are generated via an automated coder that relies on a synergy of NLP tools, neural networks using transformers, and actor information acquired via Wikipedia. The accuracy figures of PLOVER/POLECAT's Next Generation Event Coder's (NGEC) fine-tuned RoBERTa QA for coding actor, recipient, date, and location are 89.27, 68.64, 71.19, and 69.49, respectively. This surpasses the BERT baseline of 78.81, 61.86, 68.36, and 69.21, respectively.
Most of the work on event data machine coding has involved texts and reports in English. There are notable exceptions in Spanish such as Osorio and Reyes (2017) and Osorio et al. (2019). Working with English text alone is already difficult and expanding to a multilingual setting comes with its own set of challenges.27 To handle multilingual event data with machines, one approach is to extract features with a sentence embedding algorithm that codes word meanings and their semantic relationships into a vector, which is what Imani et al. (2019) do with Arabic and Spanish. A newer approach is to pre-train BERT-based models on non-English text. Nguyen et al. (2023) do this with Vietnamese social media text, and Doan et al. (2023) and Doan et al. (2024) do this with Norwegian, Swedish, Danish, and Icelandic text coded from parliamentary speeches. Before pre-training, some authors apply machine translation technology to convert non-English text into English, which is how Halterman et al. (2023) handle Arabic, Chinese, French, Portuguese, Russian, and Spanish. The tradeoff with this approach is fidelity for efficiency, as the major problem is translation drop-off. To mitigate this problem, Parolin et al. (2022) use translations made by native speakers of the target languages (Portuguese and Spanish) before pre-training to help ensure that there is correctness in syntax and semantics on the testing samples. The tradeoff of doing so, however, is efficiency for fidelity. It should be noted that one can approach multilingual data using within-language, cross-language, or zero-shot techniques (e.g., one can create a new coder in language X, use language X to train language Y, or use a zero-shot for language Y).
Relatedly, but not pertaining to multilingual data, Machlovi (2023) augments a BERT model with a deep learning pipeline to better understand the language of violence and peaceful events data through improved contextual knowledge of the words that comprise such data.
Ho and Chan (2023) argue that assessing validity with regard to multilingual text analysis should be conducted from the perspective of transferability, which is conceived of as “the extent to which the performance of a multilingual text analytic method can be maintained when switching from one language context to another” (p. 1). This is in light of what is described as a black box problem plaguing those who seek to interpret the transferability of arguably uninterpretable multilingual language models. For the modeling process, an mBERT is fine-tuned on “annotated manifestos and media texts” (p. 1) from the Comparative Agendas Project in the following Indo-European languages: English, German, French, Italian, and Spanish, which are treated as “seen” (p. 3) languages for the model. The research examines how the model performs when trained on text from seen languages (and picks up context from these languages) and applied to text from “unseen” (p. 3) languages (Basque and Chinese), which have their own unique contexts. Transferability is then evaluated by utilizing parliamentary data28 from Basque, Hong Kong, Taiwan, and the UK.
Licht (2023)'s work on language drop-offs in comparative manifestos presents another approach to multilingual text analysis. The work follows on from the finding that incorrect translations can result from solely translating dictionary keywords or the words preserved post-tokenization of documents in their native languages (Proksch et al., 2019; Reber, 2019, as cited in Licht, 2023). As an alternative to translation, the strategy is to enlist multilingual sentence embeddings (MSE), which he describes as a technique for encoding sentence-like texts into fixed-length, numerical vectors. He argues that this arrangement ensures that texts conveying similar meanings are positioned near each other in a shared vector space regardless of their language, which means that documents written in differing languages can be represented in the same feature space. The main task of the research is to use MSE-based classifiers to classify the topics and positions of sentences that originate from election manifestos found in the Comparative Manifestos Project dataset (Volkens et al., 2009), and compare the performance of this approach with that of classifiers trained with BoW representations of machine-translated texts.
For Ho and Chan (2023) and Licht (2023), it is worth noting that their works are cross-lingual, but not about event data. Their approaches entail highly-curated and specific data applications to very structured data, and will not generalize to news reports well. The election manifestos they analyze are quite formal and follow specific requirements for structure, unlike news reports, which can be a lot more informal and unstructured (Croicu, 2024).
The ideal scenario is to enumerate all possible sources of error and demonstrate how some event data system is able to exhaust them all, but this is not realistic at present. Researchers can, however, recognize errors and try to reduce them so that validity can be improved. Althaus et al. (2022)'s “total event data error (TEDE) framework for identifying and assessing” (p. 604) various types of errors that threaten validity is useful for understanding additional sources of measurement error not discussed thus far. TEDE is essentially a funneling of the broad sets of information from a large set of news sources into documents, paragraphs, named entities, linguistic objects (NLP tags), etc. down to the attributes of a complete event. Moving through the stages, errors can be made in different ways. It should be noted that the importance of a certain error source depends on the goals of the researcher and the circumstances. Identical copies of events describes a scenario in which there are duplicate entries of the same event in the data. Osorio et al. (2020) tackle this issue by removing duplicated events as part of the post-coding process. Event enumeration errors occur “when the number of distinct event records produced from a news report differs from the true number of events it describes” (Althaus et al., 2022, p. 609). Parolin et al. (2022)'s Multi-CoPED handles reciprocal events. Setting up the system to account for them is part of the task of sequence labeling. A tag identifying a reciprocal relation is applied to a word representing an entity that assumes the role of both source and target (at least two entities are required for a reciprocal event to be possible). The system generates multiple coded events for events characterized by reciprocal relations. Halterman et al. (2023)'s POLECAT dataset is enabled to list multiple actor and/or recipient countries in a single event entry, and multiple entities can be actors or recipients. It is noted that this approach differs from ICEWS, which tends to represent multi-actor and/or multi-recipient events as separate dyadic events. For instance, in the case of a four-state multilateral meeting, ICEWS would represent this as having as many as 12 directed-dyad events, whereas in POLECAT, it is preserved as one event comprised of four participants (Halterman et al., 2023).
Other issues that are not explicitly addressed by the reviewed literature, but are identified by Althaus et al. (2022), are discussed next. Attribute linkage errors are the result of incorrectly joining properly identified attributes, such as when the locations of two separate events are unintentionally merged. Event segmentation errors transpire when attributes belonging to a single event are fragmented into distinct event records or attributes from multiple events are amalgamated into one record, such as when separate accounts of a peaceful protest and a violent riot are erroneously merged into one record for a riot event. Different versions of events happens when multiple records provide distinct accounts or descriptions of the same event, and the event data system recognizes multiple events instead of one. Similar records, different events occurs when records for separate events share common characteristics and the event data system mixes up the records or mistakenly assumes that they refer to the same event.
This section identifies areas where errors can be better handled, explains how measurement can be conducted when interacting with political network data made possible by one of the SOTA models, and offers suggestions for researchers to improve how the results of their models are presented and compared with other models.
To summarize the sources of error that could benefit from more attention, these include entity errors (e.g., resolving issues associated with the transliteration of names and the identification of new actors not listed in any dictionary or Wikipedia), attribute linkage errors, event segmentation errors, different versions of events, and similar records, different events. Also, while Halterman et al. (2023) make progress by determining geolocation from the text of a news report, there is still room for improvement.
Another opportunity is to build political network data. Utilizing ConfliBERT data, political networks can be constructed based on the corpus of text and results from the model. The data would consist of the relations among/between individuals, groups, events, and locations. This is possible because ConfliBERT data allow one to measure the similarity between various political actors in a Euclidean vector space, which means that insights can be gleaned on how the aforementioned items are similar and dissimilar. Using the emergence of a new political actor as one example, the relationship of this actor to their group can be measured against a known political actor and their group (e.g., comparing the vector space for a new leader and rebel organization with Osama bin Laden and Al-Qaeda). Additionally, how these political actors are discussed can assist with the identification of a latent network similarity between various political actors.
There are recommendations for authors to make the measurement part of their work clearer and more precise. This guidance is intended to be helpful. First, authors should make it very obvious what their model is and what the comparison models are, what the training and test data are, what the unit of analysis is (that all models in a comparison are using), what ontology they are applying, what metrics they are using and why they are using them, and what the limitations are for all of these items. While foundational, they are not always apparent in every work.
Consistent terminology in and across works is also important. Some authors use multiple terms to describe the same unit of analysis when it may be better to use a single term to avoid confusion. Also, when one term is used to describe a particular unit, it should be clear what the unit entails. For example, saying that the “span of text” is the unit of analysis is not always sufficiently informative. The number of words comprising the span should be specified if the span is intended to be bounded.29 Where it makes sense and is possible, using consistent terminology and being specific about the unit of analysis is beneficial for comparison and interpretation.
Another pitfall is estimating the performance of a model and how it compares with other models by solely focusing on accuracy, precision, recall, and F1, while ignoring other metrics that taken together would provide a more complete account of performance. Computational time and storage should also be considered in one's estimation of overall performance (Alsarra, 2023). Model effectiveness is one thing; model efficiency is another.30
The landscape of recent event data research is contoured by a greater reliance on machines (particularly, transformer-based models) that are showing much promise in delivering results more efficiently. This paper focuses on the measurement aspect of the research. Measurement is a determination of the distance between what a machine codes/classifies from information describing an event and what really is happening in the event. Measurement is foundational and transcends technology: Legacy and state-of-the-art systems are subject to the same measurement concerns. Understanding measurement and how to apply it matters because it helps ensure that appropriate assessments about the performance of a model on specific tasks are made, which enables the fair demonstration of how it compares with other models. To responsibly handle these data, it is imperative to respect the measurement component to avoid faulty inferences and misinformed decision making. Discussed are important definitions and concepts, what researchers are doing to advance the SOTA and how they are dealing with a host of measurement errors, areas to improve on mitigating certain sources of error, and a way forward to maneuver in this field of research.
Lastly, there are final thoughts looking ahead to the near future. In terms of modeling, it appears that LLMs will continue to dominate the coding/classifying of event data. In terms of model training, expect to see more domain-trained LLMs, as the sizeable marginal returns in performance achieved by these models are a result of fine-tuning with domain-specific training data.31 In terms of model application, LLMs will probably be leveraged more than they are now for the tasks of constructing political networks and identifying new actors and actions more quickly and effectively. These tasks are highly important and challenging, and represent a major opportunity for researchers operating at the cutting edge to fully exploit the SOTA tools available, as well as those yet to be developed.
The original contributions presented in the study are included in the article/Supplementary material, further inquiries can be directed to the corresponding author.
PB: Writing – original draft, Writing – review & editing. MS: Conceptualization, Investigation, Methodology, Writing – original draft, Writing – review & editing.
The author(s) declare financial support was received for the research, authorship, and/or publication of this article. This material is based upon work supported by the National Science Foundation under Grant Nos. OAC-CSSI-1931541 and 2311142.
The authors declare that the research was conducted in the absence of any commercial or financial relationships that could be construed as a potential conflict of interest.
All claims expressed in this article are solely those of the authors and do not necessarily represent those of their affiliated organizations, or those of the publisher, the editors and the reviewers. Any product that may be evaluated in this article, or claim that may be made by its manufacturer, is not guaranteed or endorsed by the publisher.
Any opinions, findings, and conclusions or recommendations expressed in this material are those of the author(s) and do not necessarily reflect the views of the National Science Foundation.
The Supplementary Material for this article can be found online at: https://www.frontiersin.org/articles/10.3389/fpos.2024.1453640/full#supplementary-material
1. ^While our focus is on text, data that appear in other formats such as images, video, and speech are also researched. See Alanyali et al. (2016), Steinert-Threlkeld (2019), Zhang and Pan (2019), Sobolev et al. (2020), Wen et al. (2021), Mitts et al. (2022), Steinert-Threlkeld et al. (2022), and Abedin et al. (2023).
2. ^This is mentioned as a reference for how event attributes have historically been arranged into a framework. It could be enlarged to accommodate other attributes as desired. As technology has progressed from dictionary-based methods to BERT models, the machine-extraction of additional attributes is more feasible. Also, in the last 20 years, there has been development in the ontologies applied to event data (e.g., the more recent PLOVER that accounts for modality and context vs. the older PETRARCH that primarily focuses on source, action, and target).
3. ^For information on how this is bridged to different domains (see Olsen et al., 2024).
4. ^This paper does not cover sentiment analysis. It also does not address decisions about source bias, which can be very domain, country, and application specific. While source-related issues issues are important, they are well-known research design problems whose implications are explored elsewhere (see Davenport and Ball, 2002, Shellman et al., 2007, Shellman, 2008, and Shaver et al., 2022). We presuppose that researchers have already done their due diligence in this regard.
5. ^Our focus is on curated, mainstream media-generated data like that historically used in event data projects like those we describe here. Others might be more interested in researching user-generated data (e.g., data generated from individuals submitting records to the Crowd Counting Consortium, social media posts, etc.) All of those face the same and additional concerns to the many raised here.
6. ^There are also subjective, human-level judgements that can be administered.
7. ^A good analogy here is “health” as a form of scoring, and blood pressure, heart rate, blood oxygen, or others as performance metrics.
8. ^We focus here on the subject, but one can see the extension across the verb and target object combinations. This can then be extended to more categories, but the basic scoring problem of defining and computing a metric is similar.
9. ^Alternatively, if the scores for precision and recall are used for this computation, it would be .
10. ^Only binary classification is demonstrated here. The confusion matrix can also be used for the multi-label case, but it is not the same as the confusion matrix for the binary case because the former picks up another dimension. If using quad categories or pentacodes, the multi-label would be an exact verb phrase (e.g., “attack verbally” vs. “attack materially”), and this would map back to the ontology. This type of situation where the action is correctly identified, but the nature of the action is not, can occur when a model does not account for contextual information.
11. ^Machine-human drop-off is possible as well, and this designation would be reserved for situations where a machine performs better than a human.
12. ^Generative language models that produce synthetic text are also used, but rarely (see Dai et al., 2022).
13. ^Some authors are focused on national security and create practical event data to solve real-world problems. Others are hyper-focused on advancing the detection of a specific event attribute. Some are mostly concerned with preserving the linguistic verisimilitude of coding/classifying event data in one language vs. another, whereas others are content with just applying an LLM in the same domain across numerous languages. And, there are some who seek perfection in one language and for a very specific application.
14. ^A web scraper acquires the news reports every two hours from about 400 RSS feeds.
15. ^This approach is also costly to maintain and slow to update.
16. ^This also applies to other attributes such as new types of events.
17. ^An ABC News report claims that, due to difficulties in translating Arabic to English, there are 112 different English spellings of the name of a particular Libyan leader who was assassinated in 2011. Here are some of the representations of his name: Muammar Qaddafi, Muammar Al-Gathafi, Muammar al-Qadhafi, Mu'ammar Al Qathafi, Muammar Al Qathafi, Moamar El Gaddafi, Moammar El Kadhafi, Moamer El Kazzafi, and Mu'Ammar El Qathafi (Bass, 2009). The report states that The Associated Press, The New York Times, and Xinhua have used 40 different spellings of his name, and that 72 different spellings are listed by the Library of Congress. Adding to the confusion, one of his sons spelled his last name “Qadhafi” during an interview with Newsweek's Christopher Dickey (Daily Beast, 2011), while The Atlantic claims that the purported diplomatic passport of another one of his sons, which was allegedly discovered by a rebel inside the Bab al-Aziziya military compound, shows his last name spelled as “Gathafi” (Fisher, 2011). There are thus many representations of this former leader's name in English (and in French).
18. ^The PLOVER ontology utilizes “16 overarching event types for the classification of events into distinct (verbal or material) cooperative or hostile event types” (Halterman et al., 2023, p. 7).
19. ^Lefebvre and Stoehr (2022) argue that this pipeline allows for greater resource efficiency and flexibility compared with the typical approach of having an event type ontology developed by domain experts, then having a large dataset labeled by annotators, and then employing experts to establish a supervised system of coding.
20. ^Global Terrorism Database (GTD) [START (National Consortium for the Study of Terrorism and Responses to Terrorism), 2022], India Police Events data (Halterman et al., 2021), and a new dataset created with corpora from the politics domain (Hu et al., 2022).
21. ^South Asia Terrorism Portal (SATP) data and InSight Crime data (Parolin et al., 2021).
22. ^Althaus et al. (2022) provide a couple of examples regarding where an event data system could go wrong: Paris, Illinois, could be mistaken for Paris, France. Also, locations are susceptible to imprecision, and if the most detailed geographic description available appears to be at the level of a country (e.g., the system records the location of the event as Thailand), but the event actually took place in a border city (e.g., Aranyaprathet, Thailand), the location of the event could be erroneously placed at the centroid of the country's (e.g., Thailand's) national boundaries instead of at the border city (e.g., Aranyaprathet).
23. ^Imani et al. (2019) follow the same general process, but with non-English data. They extract features with a sentence embedding algorithm that codes word meanings and their semantic relationships into a vector with the fastText_multilingual model.
24. ^For source and target detection in English, Spanish, and Portuguese: PETRARCH, PETRARCH2, UPETRARCH, SRL-based models, and LSTM-based models. For action detection in English, Spanish, and Portuguese: PETRARCH, PETRARCH2, UPETRARCH, and LSTM-based models. For end-to-end coding in English: PETRARCH, PETRARCH2, UPETRARCH, and LSTM-based models. For end-to-end coding in Spanish: UPETRARCH and LSTM-based models.
25. ^Binary classification, sentence binary classification, document binary classification, multi-class classification, relevant multi-label classification, all multi-label classification, multi-label classification, sentence multi-label classification, document multi-label classification, TS multi-class classification, PC multi-class classification, ST NER, and NER.
26. ^General, violence, protest, terrorism, crime, politics, and terrorism defense.
27. ^E.g., with Osorio et al. (2020)'s Hadath system, NLP is used to code events from Arabic text. This requires a machine to read from right to left, meaning that the system must be careful about the order of actors and actions. Also, because the Arabic verbs in their actions dictionary do not include pronunciation diacritics, duplicate verb conjugations that yield the same plain Arabic script after diacritics are removed are deduplicated.
28. ^Natural and synthetic data are used.
29. ^See Olsen et al. (2024) for more information on how spans are approached in NLP work.
30. ^A cost function analysis is a helpful tool in this regard.
31. ^Also, the results from Hu et al. (2024) indicate that the zero-shot approach does not perform as well as fine-tuning.
Abedin, A., Bais, A., Buntain, C., Courchesne, L., McQuinn, B., Taylor, M. E., et al. (2023). A call to arms: AI should be critical for social media analysis of conflict zones. arXiv preprint arXiv:2311.00810.
Alanyali, M., Preis, T., and Moat, H. S. (2016). Tracking protests using geotagged FLICKR photographs. PLoS ONE 11:e0150466. doi: 10.1371/journal.pone.0150466
Alsarra, S. (2023). Development techniques for large language models for low resource languages. Phd thesis, ProQuest Dissertations &Theses.
Althaus, S., Peyton, B., and Shalmon, D. (2022). A total error approach for validating event data. Am. Behav. Sci 66, 603–624. doi: 10.1177/00027642211021635
Beieler, J., Brandt, P. T., Halterman, A., Simpson, E., and Schrodt, P. A. (2016). “Generating political event data in near real time: opportunities and challenges,” in Computational Social Science, ed. R. M. Alvarez (Cambridge: Cambridge University Press). doi: 10.1017/CBO9781316257340.005
Croicu, M. (2024). Deep active learning for data mining from conflict text corpora. arXiv preprint arXiv:2402.01577.
Dai, Y., Radford, B., and Halterman, A. (2022). “Political event coding as text-to-text sequence generation,” in Proceedings of the 5th Workshop on Challenges and Applications of Automated Extraction of Socio-political Events from Text (CASE), 117–123. doi: 10.18653/v1/2022.case-1.16
Daily Beast. (2011). Saif Gaddafi on How to Spell His Last Name. Available at: https://www.thedailybeast.com/saif-gaddafi-on-how-to-spell-his-last-name (accessed June 12, 2024).
Davenport, C., and Ball, P. (2002). Views to a kill: exploring the implications of source selection in the case of Guatemalan state terror, 1977-1995. J. Conflict Resol. 46, 427–450. doi: 10.1177/0022002702046003005
Doan, T. M., Baumgartner, D., Kille, B., and Gulla, J. A. (2024). Automatically detecting political viewpoints in Norwegian text,” in International Symposium on Intelligent Data Analysis (Springer), 242–253. doi: 10.1007/978-3-031-58547-0_20
Doan, T. M., Kille, B., and Gulla, J. A. (2023). “SP-BERT: a language model for political text in scandinavian languages,” in International Conference on Applications of Natural Language to Information Systems (Springer), 467-477. doi: 10.1007/978-3-031-35320-8_34
Fisher, M. (2011). Rebel Discovers Qaddafi Passport, Real Spelling of Leader's Name. Washington, DC: The Atlantic.
Halterman, A. (2017). Mordecai: full text geoparsing and event geocoding. J. Open Source Softw. 2:91. doi: 10.21105/joss.00091
Halterman, A., Bagozzi, B. E., Beger, A., Schrodt, P., and Scarborough, G. (2023). “PLOVER and POLECAT: a new political event ontology and dataset,” in International Studies Association Conference Paper. doi: 10.31235/osf.io/rm5dw
Halterman, A., Keith, K. A., Sarwar, S. M., and O'Connor, B. (2021). Corpus-level evaluation for event QA: the IndiaPoliceEvents corpus covering the 2002 Gujarat violence. arXiv preprint arXiv:2105.12936.
Halterman, A., and Radford, B. J. (2021). Few-shot upsampling for protest size detection. arXiv preprint arXiv:2105.11260.
Ho, J. C.-T., and Chan, C. (2023). Evaluating transferability in multilingual text analyses. Comput. Commun. Res. 5, 1–20. doi: 10.5117/CCR2023.2.3.HO
Hu, Y., Hosseini, M., Parolin, E. S., Osorio, J., Khan, L., Brandt, P., et al. (2022). “ConfliBERT: a pre-trained language model for political conflict and violence,” in Proceedings of the 2022 Conference of the North American Chapter of the Association for Computational Linguistics: Human Language Technologies, 5469–5482. doi: 10.18653/v1/2022.naacl-main.400
Hu, Y., Skorupa Parolin, E., Khan, L., Brandt, P. T., D'Orazio, V. J., and Osorio, J. (2024). “Leveraging codebook knowledge with NLI and ChatGPT for political zero-shot relation classification,” in Proceedings of the 62nd Annual Meeting of the Association for Computational Linguistics (ACL 2024), Bangkok, Thailand (Association for Computational Linguistics). doi: 10.18653/v1/2024.acl-long.35
Imani, M. B., Chandra, S., Ma, S., Khan, L., and Thuraisingham, B. (2017). “Focus location extraction from political news reports with bias correction,” in 2017 IEEE International Conference on Big Data (Big Data) (IEEE), 1956–1964. doi: 10.1109/BigData.2017.8258141
Imani, M. B., Khan, L., and Thuraisingham, B. (2019). “Where did the political news event happen? Primary focus location extraction in different languages,” in 2019 IEEE 5th International Conference on Collaboration and Internet Computing (CIC) (IEEE), 61–70. doi: 10.1109/CIC48465.2019.00017
Kim, H., D'Orazio, V., Brandt, P. T., Looper, J., Salam, S., Khan, L., et al. (2019). UTDEventData: an R package to access political event data. J. Open Source Softw. 4:1322. doi: 10.21105/joss.01322
Lefebvre, C., and Stoehr, N. (2022). Rethinking the event coding pipeline with prompt entailment. arXiv preprint arXiv:2210.05257.
Licht, H. (2023). Cross-lingual classification of political texts using multilingual sentence embeddings. Polit. Anal. 31, 1–14. doi: 10.1017/pan.2022.29
Liu, Y., Ott, M., Goyal, N., Du, J., Joshi, M., Chen, D., et al. (2019). RoBERTa: a robustly optimized BERT pretraining approach. arXiv preprint arXiv:1907.11692.
Machlovi, N. (2023). SPOCK: subjective projection for optimizing deep neural classification models based on contextual representation from large knowledgebase. PhD thesis, Fordham University.
Miller, E., Kishi, R., Raleigh, C., and Dowd, C. (2022). An agenda for addressing bias in conflict data. Sci. Data 9:593. doi: 10.1038/s41597-022-01705-8
Mitts, T., Phillips, G., and Walter, B. F. (2022). Studying the impact of ISIS propaganda campaigns. J. Polit. 84, 1220–1225. doi: 10.1086/716281
Nguyen, Q.-N., Phan, T. C., Nguyen, D.-V., and Van Nguyen, K. (2023). ViSoBERT: a pre-trained language model for Vietnamese social media text processing. arXiv preprint arXiv:2310.11166.
Norris, C., Schrodt, P. A., and Beieler, J. (2017). PETRARCH2: another event coding program. J. Open Source Softw. 2:133. doi: 10.21105/joss.00133
Olsen, H., Simon, É., Velldal, E., and Øvrelid, L. (2024). “Socio-political events of conflict and unrest: a survey of available datasets,” in Proceedings of the 7th Workshop on Challenges and Applications of Automated Extraction of Socio-political Events from Text (CASE 2024), 40–53.
Osorio, J., Pavon, V., Salam, S., Holmes, J., Brandt, P. T., and Khan, L. (2019). Translating CAMEO verbs for automated coding of event data. Int. Inter. 45, 1049–1064. doi: 10.1080/03050629.2019.1632304
Osorio, J., and Reyes, A. (2017). Supervised event coding from text written in Spanish: introducing eventus ID. Soc. Sci. Comput. Rev. 35, 406–416. doi: 10.1177/0894439315625475
Osorio, J., Reyes, A., Beltrán, A., and Ahmadzai, A. (2020). “Supervised event coding from text written in Arabic: introducing Hadath,” in Proceedings of the Workshop on Automated Extraction of Socio-political Events from News 2020, 49–56.
Parolin, E. S., Hosseini, M., Hu, Y., Khan, L., Brandt, P. T., Osorio, J., et al. (2022). “Multi-CoPED: a multilingual multi-task approach for coding political event data on conflict and mediation domain,” in Proceedings of the 2022 AAAI/ACM Conference on AI, Ethics, and Society, 700–711. doi: 10.1145/3514094.3534178
Parolin, E. S., Khan, L., Osorio, J., Brandt, P. T., D'Orazio, V., and Holmes, J. (2021). “3M-transformers for event coding on organized crime domain,” in 2021 IEEE 8th International Conference on Data Science and Advanced Analytics (DSAA) (IEEE), 1–10. doi: 10.1109/DSAA53316.2021.9564232
Proksch, S.-O., Lowe, W., Wäckerle, J., and Soroka, S. (2019). Multilingual sentiment analysis: a new approach to measuring conflict in legislative speeches. Legis. Stud. Q. 44, 97–131. doi: 10.1111/lsq.12218
Rajpurkar, P., Jia, R., and Liang, P. (2018). Know what you don't know: unanswerable questions for SQuAD. arXiv preprint arXiv:1806.03822. doi: 10.18653/v1/P18-2124
Reber, U. (2019). Overcoming language barriers: assessing the potential of machine translation and topic modeling for the comparative analysis of multilingual text corpora. Commun. Methods Meas. 13, 102–125. doi: 10.1080/19312458.2018.1555798
Sanh, V., Debut, L., Chaumond, J., and Wolf, T. (2019). DistilBERT, a distilled version of BERT: smaller, faster, cheaper and lighter. arXiv preprint arXiv:1910.01108.
Schrodt, P. A. (2001). “Automated coding of international event data using sparse parsing techniques,” in Annual Meeting of the International Studies Association, Chicago (Citeseer).
Shaver, A. (2022). News media reporting patterns and our biased understanding of global unrest. Technical report, Empirical Studies of Conflict Project.
Shellman, S. M. (2008). Coding disaggregated intrastate conflict: machine processing the behavior of substate actors over time and space. Polit. Anal. 16, 464–477. doi: 10.1093/pan/mpn008
Shellman, S. M., Reeves, A., and Stewart, B. (2007). Fair &Balanced or Fit to Print. The Effects of Media Sources on Statistical Inferences. Athens: University of Georgia.
Sobolev, A., Chen, M. K., Joo, J., and Steinert-Threlkeld, Z. C. (2020). News and geolocated social media accurately measure protest size variation. Am. Polit. Sci. Rev. 114, 1343–1351. doi: 10.1017/S0003055420000295
Solaimani, M., Salam, S., Khan, L., Brandt, P. T., and D'Orazio, V. (2017). “RePAIR: recommend political actors in real-time from news websites,” in 2017 IEEE International Conference on Big Data (Big Data), 1333–1340. doi: 10.1109/BigData.2017.8258064
START (National Consortium for the Study of Terrorism and Responses to Terrorism). (2022). Global Terrorism Database 1970–2020 [data file]. Available at: https://www.start.umd.edu/gtd (accessed December 4, 2024).
Steinert-Threlkeld, Z. C. (2019). The future of event data is images. Sociol. Methodol. 49, 68–75. doi: 10.1177/0081175019860238
Steinert-Threlkeld, Z. C., Chan, A. M., and Joo, J. (2022). How state and protester violence affect protest dynamics. J. Polit. 84, 798–813. doi: 10.1086/715600
Volkens, A., Bara, J., and Budge, I. (2009). “Data quality in content analysis. the case of the comparative manifestos project,” in Historical Social Research/Historische Sozialforschung, 234–251.
Wen, H., Lin, Y., Lai, T., Pan, X., Li, S., Lin, X., et al. (2021). “RESIN: a dockerized schema-guided cross-document cross-lingual cross-media information extraction and event tracking system,” in Proceedings of the 2021 Conference of the North American Chapter of the Association for Computational Linguistics: Human Language Technologies: Demonstrations, 133–143. doi: 10.18653/v1/2021.naacl-demos.16
Keywords: event data, political methodology, natural language, political conflict, international relations
Citation: Brandt PT and Sianan M (2025) Measurement of event data from text. Front. Polit. Sci. 6:1453640. doi: 10.3389/fpos.2024.1453640
Received: 23 June 2024; Accepted: 25 November 2024;
Published: 06 January 2025.
Edited by:
Jan-Erik Refle, University of Geneva, SwitzerlandReviewed by:
Zachary Steinert-Threlkeld, University of California, Los Angeles, United StatesCopyright © 2025 Brandt and Sianan. This is an open-access article distributed under the terms of the Creative Commons Attribution License (CC BY). The use, distribution or reproduction in other forums is permitted, provided the original author(s) and the copyright owner(s) are credited and that the original publication in this journal is cited, in accordance with accepted academic practice. No use, distribution or reproduction is permitted which does not comply with these terms.
*Correspondence: Patrick T. Brandt, cGJyYW5kdEB1dGRhbGxhcy5lZHU=
Disclaimer: All claims expressed in this article are solely those of the authors and do not necessarily represent those of their affiliated organizations, or those of the publisher, the editors and the reviewers. Any product that may be evaluated in this article or claim that may be made by its manufacturer is not guaranteed or endorsed by the publisher.
Research integrity at Frontiers
Learn more about the work of our research integrity team to safeguard the quality of each article we publish.