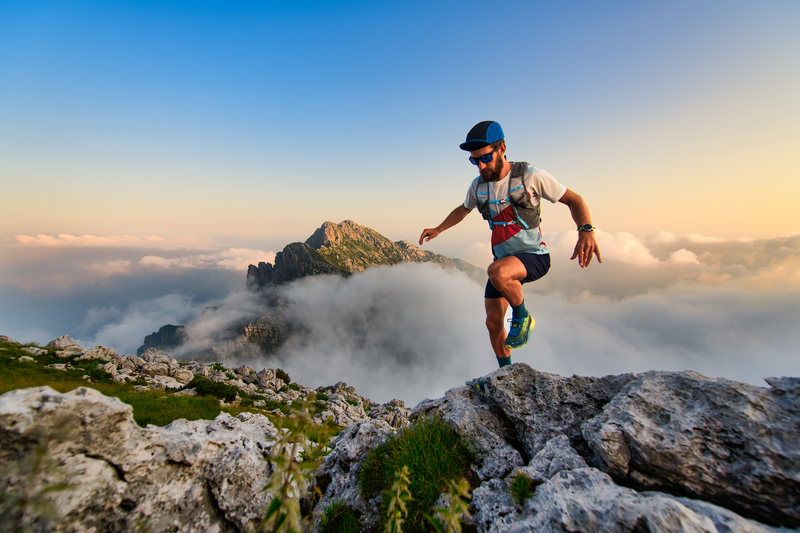
94% of researchers rate our articles as excellent or good
Learn more about the work of our research integrity team to safeguard the quality of each article we publish.
Find out more
REVIEW article
Front. Polit. Sci. , 30 January 2024
Sec. Political Science Methodologies
Volume 6 - 2024 | https://doi.org/10.3389/fpos.2024.1286893
The voter information tools collectively known as “Voting Advice Applications” (VAAs) have emerged as particularly popular tools in the realm of E-participation. Today, VAAs are integral parts of election campaigns in many countries around the world as they routinely engage millions of citizens, in addition to political actors and the media. This contribution assesses the integration of Artificial Intelligence (AI) in the design and dissemination of VAAs, considering normative, ethical, and methodological challenges. The study provides a comprehensive overview of AI applications in VAA development, from formulating questions to disseminating information, and concludes by highlighting areas where AI can serve as a valuable tool for enhancing the positive impact of VAAs on democratic processes.
The potential of information and communication technologies (ICTs) to re-engage people with the democratic process has been debated in the political science literature since the early 1970s (Dutton, 1992). With the emergence of more advanced technologies, such as the Internet, the use of ICTs in increasing the inclusiveness and accessibility of decision-making has become a particular priority for governments, as well as actors such as the European Union which has been funding numerous projects in the domain of E-participation. Amidst this transformative landscape, Artificial Intelligence (AI) has undoubtedly affected the use of ICTs. However, a judicious examination of the potential pitfalls associated with AI methods is imperative to ensure the integrity and efficacy of these innovative tools. While many have deplored the fact that AI can be used as a tool to produce and spread disinformation, AI has the potential to enhance the use of ICTs that aim to reduce information asymmetries and re-engage the most apathetic citizens in political processes.
E-participation can take different forms such as remote electronic voting (e.g. voting over the Internet), signing petitions online, or involvement in decision-making through digital fora. However, when asked about different proposals for tackling low electoral participation, one of the top choices among young people was the online interactive voter information tools (Cammaerts et al., 2016). These voter information tools, which are known in the scientific community as Voting Advice Applications (VAAs) are digital platforms that offer personalized recommendations based on how one's preferences on various policy issues match those political parties or candidates. By comparing users' responses to specific policy-related questions with the positions of parties or candidates (referred to here as “statements”), VAAs aim to facilitate informed decision-making during elections and address the issue of citizen competence by reducing the information asymmetries among different groups. More broadly, VAAs engage citizens in thinking about political issues and provide them with information about the positions of political parties or candidates on these issues. The underlying idea is that this public and freely accessible information can empower citizens by making them more competent in holding politicians accountable when casting their votes.
One could easily argue that the emergence of VAAs has provided the most visible impact of political science in the political lives of citizens. From their humble beginnings as pen-and-paper tests for civic education in schools in the Netherlands, VAAs have progressed to digital interventions that are integral parts of elections campaigns, involve state media, and are used by millions of people, with measurable impacts on political behavior and participation. While the development of VAAs has generally followed an independent path in the broader context of the digitalization of politics, VAAs have caught up with the developments in AI. Even though most VAAs today are nearly identical to those that were released two decades ago, AI has begun to challenge the traditional ways in which VAAs are designed and delivered to the public.
This contribution aims to take stock of the different ways in which VAAs have employed elements of AI in their design and dissemination, or could potentially employ in the future. To understand the context in which AI can be incorporated in the design and delivery of VAAs, however, one has to consider the various normative, ethical, and methodological limitations that already face the design of VAAs. Consequently, the article begins with a brief overview of VAAs and their purported effects and outlines the various normative, ethical, and methodological challenges that VAA designers face. The article continues with a state of the art in the design of VAAs with a special emphasis on those instances that involve the use of AI. In particular, the article focuses on using AI in developing the questions used in VAAs, in providing additional information about the policy issues that are featured in VAAs, in estimating the positions of political actors, in matching the position of users to those of political actors, in disseminating VAAs to the public, as well as in pre-processing user-generated VAA data for scientific research that can inform the design of VAAs at large. In each area, the article highlights the contentious issues, and, in the concluding section, offers thoughts on the areas where AI could provide valuable service to VAA designers in delivering VAAs with the potential to make a positive impact on democracy.
This article defines VAAs as “online interactive voter information tools that focus on policy issues during elections”. This definition distinguishes VAAs from other similar, but conceptually distinct, voter information tools (see Table 1). VAAs focus on policy issues, unlike other voter information tools, such as, for instance, SpacEU1 which offers information on voting rules and regulations for mobile citizens across the EU but does not have a focus on policy issues. Moreover, VAAs are interactive, in the sense that they offer an output that features the match between the user and political actors. On the contrary, the BBC Manifesto Guide.2 allows citizens to compare the positions of political parties on many different policy issues but does not offer an interactive element in terms of a matching algorithm that could allow them to compare these positions with their own. Furthermore, VAAs are disseminating during elections, unlike voter information tools such as the one developed by the “Puzzled By Policy?” project (Sánchez-Nielsen et al., 2014) where citizens could compare their views on immigration issues relate to existing policies within the EU without being specific to any particular electoral contest. Finally, VAAs are online tools, drawing therefore a conceptual distinction from interactive voter information tools that focus on policy issues around elections, but operate offline, such as many voting guides offered by regional newspapers and issue-specific organizations.
VAAs emerged in the late 1980s in the Netherlands as an educational tool for high schools in the form of a booklet with a computer disk (De Graaf, 2010), but soon moved beyond the classrooms into the World Wide Web, where they were hailed as technological solutions aiming to empower citizens by making them more competent when casting their votes. In most countries, VAAs have enjoyed particular longevity and have consequently become integral parts of election campaigns. For instance, Vaalikone has been offered by the Finnish Public Broadcasting Company (YLE) for every election in the country since 1996, while in the Baltic countries, it is “almost unthinkable” that an election campaign can be run today without such online tools (Eibl, 2019, p. 85). Furthermore, VAAs are often able to engage citizens, political actors, and the mainstream media, in unprecedented numbers compared to other forms of E-participation. For instance, the Swiss Smartvote has achieved over 85% response rate to their questionnaire among thousands of candidates in federal and cantonal elections, while Wahl-O-Mat was used about 21.3 million times in the 2021 federal election in Germany.
Notwithstanding their popularity, however, it is not at all clear whether VAAs have achieved their goals. Fifteen years of research on the effects of VAAs on political knowledge, behavior, and participation (Garzia and Marschall, 2016, p. 380–382) has yielded largely positive but sometimes inconclusive results, as a recent meta-analysis has concluded (Munzert and Ramirez-Ruiz, 2021). The early literature on VAA effects presented an overly optimistic picture based on self-reported effects among VAA users and simple cross-tabulations of survey data (see Ruusuvirta and Rosema, 2009; Fivaz and Nadig, 2010; Garzia, 2010), and these optimistic assessments have been widely cited and quoted as measurable effects on political knowledge and participation. Soon enough, those early studies were criticized on methodological grounds, for drawing on samples that were not representative of the voting population and for reporting effects without accounting for self-selection (Pianzola, 2014a). As VAA users are more likely to be highly educated and politically interested, these confounding factors could explain the higher levels of knowledge and participation among VAA users in the first place.
Subsequent research into the effects of VAAs accounted for these critiques in two different ways. A strand in the literature has focused on nationally representative surveys where self-selection was addressed by using more sophisticated statistical techniques such as a matching (e.g. Pianzola, 2014b; Gemenis and Rosema, 2014; Gemenis, 2018; Germann and Gemenis, 2019; Heinsohn et al., 2019), selection models (e.g. Garzia et al., 2014, 2017b; Wall et al., 2014), difference-in-differences designs (Benesch et al., 2023), and panel data analysis (Israel et al., 2017; Manavopoulos et al., 2018; Kleinnijenhuis et al., 2019). The results of these studies were rather mixed, showing a positive effect of VAA usage on electoral turnout in some countries such as Switzerland, the Netherlands, and Finland, but not in others like Greece, while in Germany VAA usage was associated with information-seeking activities but not more active engagement with electoral campaigns such as attending party rallies. Another strand, looked at randomized experiments in countries and regions like Estonia (Vassil, 2011), Finland (Christensen et al., 2021), Germany (Munzert et al., 2020), Hungary (Enyedi, 2016), Italy (Garzia et al., 2017b), Japan (Tsutsumi et al., 2018), Northern Ireland (Garry et al., 2019), Quebec (Mahéo, 2016, 2017), Switzerland (Pianzola et al., 2019; Stadelmann-Steffen et al., 2023), Taiwan (Liao et al., 2020), Turkey (Andı et al., 2023), Greece, Bulgaria, Romania, Spain, and Great Britain (Germann et al., 2023). Again, while most studies find evidence of VAA effects on voting behavior and political participation (Garry et al., 2019; Pianzola et al., 2019; Liao et al., 2020; Christensen et al., 2021; Germann et al., 2023; Stadelmann-Steffen et al., 2023), other studies find no meaningful effects (Enyedi, 2016; Tsutsumi et al., 2018; Munzert et al., 2020), or effects that were limited to undecided (or centrist) voters (Vassil, 2011; Mahéo, 2016; Andı et al., 2023).
Considering the methodological shortcomings in some of the experimental designs (Germann and Gemenis, 2019, p. 154–155), the lack of comparative replication studies, especially among the randomized experiments, and the “file drawer problem” where negative results are less likely to be published, we have valid reasons to be less enthusiastic about the electoral effects of VAAs compared to what has been suggested by the earlier literature. More importantly, however, we still do not know whether the electoral effects of VAAs, wherever these occur unambiguously, are associated with the design of VAAs. Given that the VAAs that have been the subject of these numerous studies feature designs that differ considerably, and that users like some VAA features better than others (Alvarez et al., 2014), the question of whether better-designed VAAs could become more effective in mobilizing citizens, especially those within groups that suffer from participatory inequalities, remains open.
Given that many scientific areas have benefited from the advent of AI, it is reasonable to expect that VAA designers, could, at least partially, reconsider the established methods behind the design of VAAs to enhance their performance in terms of their set goals. Before outlining, however, the innovative approaches that have brought AI into VAAs, we need to consider the contentious issues that are associated with the typical design of a VAA.
The proliferation of VAAs under many different actors has created a landscape characterized by both cooperation and rivalry among the different VAA developers, as evidenced in the scholarly debate. These debates, however, are taking place within a larger context where social and political actors as well as citizens (as the end users) have raised numerous normative, ethical, and methodological issues about the design of the VAAs. As these issues underpin any attempt to incorporate elements of AI in the design and delivery of VAAs, I offer a summary of the relevant challenges in this section.
In the normative realm, there are concerns that the VAAs which are based on social choice theory ignore the deliberative and contestatory conceptions of democracy (Fossen and Anderson, 2014). As such, they assume that the competence gap lies in political information about policy positions, and not in the lack of preferences on issues or the constricted perceptions of the political landscape (Anderson and Fossen, 2014, p. 218–221). It is not surprising, therefore, that citizens have criticized VAAs for “reproducing the mainstream political agenda by forcing their users to take a position in a limited spectrum of political topics and by excluding political parties or views that may be peripheral” (Triga, 2014, p. 140). In turn, this is reflected in methodological choices such as the choice of presenting the results in spatial maps that feature pre-defined or even clichéd dimensions (Fossen and van den Brink, 2015), an issue that has been brought up in the methodological debates as well.
Furthermore, it is widely known, that VAAs by and large attract, predominantly, young, male, educated, with high levels of political interest (Marschall, 2014). It is possible, therefore, that, despite the promise to the contrary, the use of VAAs may magnify the traditional participatory inequalities in the digital society (Marschall and Schultze, 2015), something which has also been pointed out by citizens when asked to evaluate VAAs in the context of political processes (Triga, 2014, p. 141).
Turning to ethical concerns, citizens have long questioned the objectivity and political neutrality of the researchers who design VAAs (Triga, 2014, p. 140). The example of VAAs in Lithuania has also illustrated how VAAs can be hijacked by populists by adopting ideologically unconstrained policy positions, adjusting their policy positions to the “average” voter to manipulate the results of the tool to their advantage (Ramonaitė, 2010). Moreover, political parties often accuse VAAs of providing false dichotomies, of misinterpreting their policy positions, failing to include smaller parties, or generally being “unfair”. (Trechsel and Mair, 2011, p. 14) for instance, mention, among others, the case of the German party CDU, which deplored the fact that political parties were not consulted in the process of drafting the VAA questions in the making of EU Profiler VAA, as has been the standard practice of Wahl-O-Mat, the most popular VAA in Germany. Furthermore, cyber-attacks on VAA websites have raised concerns about data security, given the more recent attempts at electoral manipulation through doxing, trolling, and disinformation (Hansen and Lim, 2019). Finally, informed consent should be acquired from respondents when VAAs feature experiments that are likely to involve changes in the selection and/or wording of the questions and, consequently, the content and presentation of the results (e.g., Holleman et al., 2016; Bruinsma, 2023).
Such ethical issues are not to be taken lightly, especially when they have legal ramifications. In the Netherlands, the Data Protection Authority (AP) requires that all websites associated with VAAs are required to have SSL encryption which ensures the secure transmission of all data that citizens provide in the online questionnaires.3 Before this requirement, the Netherlands Authority for Consumers and Markets (ACM) had taken action against four VAAs that used tracking cookies without the consent of the respondents. More recently, a regional court in Cologne forced Wahl-O-Mat offline during the campaign for the 2019 elections to the European Parliament on the issue.4 This emerged after a minor political party complained that the VAA website only allowed users to compare eight political parties at any particular time, which could be interpreted as a potential disadvantage for smaller and less known parties.
Methodologically, it has been shown that the selection of questions in VAAs has a considerable impact on the information that is provided to the users. Some configurations of questions favor certain parties, while other configurations of questions benefit other parties (Walgrave et al., 2009). Furthermore, the a priori aggregation of questions in “political maps” provided by many VAAs is empirically problematic. The scales used to construct the political dimensions often lack unidimensionality and reliability (Germann and Mendez, 2016; Bruinsma, 2020a). The reliability of estimating the positions of political actors in VAAs has been called into question (Gemenis and van Ham, 2014), while different algorithms that are used to match VAA users to parties or candidates are very likely to produce different results (Mendez, 2012; Louwerse and Rosema, 2014). When it comes to visualizing the matching results, research has shown that users do not assess the different options equally favorably (Alvarez et al., 2014; Bruinsma, 2020b). Such findings raise questions about citizen knowledge and competence when interpreting the results of VAAs (Triga, 2014, p. 137), especially since different users are likely to interpret the same results in very different ways (Bruinsma, 2020b).
While all these methodological problems have produced a lively debate and a distinct strand in the VAA literature (Garzia and Marschall, 2016, p. 382–383), the debate has not resulted in an evident cross-fertilization in terms of VAA design. VAA developers most often stick to their tried and tested recipe, while the questions and criticisms often remain open and unresolved.
The advent of AI methods has led to some further experimentation in the design of VAAs, with researchers proposing various approaches from the fields of text mining and machine learning. While these are often viewed as welcome methodological innovations on the classic VAA design, they raised important questions of their own. The following section presents these proposals within the context of the established methods, and explores the relevant contentious normative, ethical, or methodological issues.
As one of the key technologies of the 21st century, AI has affected how VAAs operate. This contribution reports on the use of AI in the core design elements of typical VAAs as well as the use of AI in developing additional innovative features in VAAs. In addition, the section looks at the use of AI in pre-processing VAA user response data and concludes with the use of AI in disseminating VAAs to the public.
VAAs aim to help citizens make more informed voting decisions predicated on a rational model in which citizens decide how to vote by comparing their stances on certain political issues to those of parties and/or candidates (Mendez, 2012). In many respects, the design of VAAs privileges an “issue voting” understanding of politics formalized in spatial theories of voting at the expense of other psychological/sociological factors and strategic considerations (Mendez, 2012, p. 265–266), and a representative view of democracy compared to other conceptions of democracy that allow for more meaningful participatory and deliberative exchanges (Fossen and Anderson, 2014). According to this view, VAAs attempt to match citizens to parties and/or candidates based on predefined statements outlining politically salient policy issues, ranging from as little as seven statements used in the Irish PickYourParty fielded in 2007 (Wall et al., 2009), to as many as 75 statements in the “deluxe” version of Smartvote in Switzerland, with most VAAs employing around 30 such statements (see Figure 1).
While there is no commonly agreed method on how to select and formulate the questions used by VAAs to match their users to political actors, the selection usually begins with a set of statements that reflect the assumptions of those who are involved in the design of the VAA about the issues that are deemed politically salient in the particular election, that is often supplemented by more generic statements used in electoral research surveys (e.g., Andreadis, 2013; Wall et al., 2009). A few VAA designers often invite journalists (see Krouwel et al., 2012), representatives of political parties (as in the cases of Stemwijzer and Wahl-O-Mat), and/or other stakeholders (see Marzuca et al., 2011) in the process. Yet others have suggested the possibility of more systematic selection methods based on topics derived from automated text analyses of newspaper corpora (Krouwel et al., 2012), issues that were featured in bills debated by the legislature (Skop, 2010), or issues extracted through a qualitative analysis of party manifestos (Krouwel et al., 2012).
As the process of selecting issues to formulate the questions in VAAs appears to be more of an art than a science, researchers have long expressed concerns that the selection of certain statements may favor certain parties in terms of matching them more often to VAA users (Walgrave et al., 2009). In practice, the issue statements used by different VAA designers tend to be similarly distributed across topics (König and Jäckle, 2018), and these distributions are similar to those found among the most important issue questions found in election studies and the content analysis of party manifestos (van Camp et al., 2014). While such findings might be reassuring in terms of VAAs inability to influence the election results, they also imply that the choice of issues/statements that are featured in the VAA questionnaire may be prone to researcher bias. It is, after all, a small academic community of political scientists that determines the content of both election study and VAA questionnaires, while citizens have little, if any, input in the process.
AI has been long used to automating the search for salient issues in political and electoral contexts, and VAA designers have ample empirical findings to consider. For instance, Lin et al. (2015) proposed an automated text mining mechanism to classify legislative documents into predefined categories to allow the public to monitor legislators and track their legislative activities. Ahonen and Koljonen (2018) used topic modeling on a longitudinal dataset of party manifestos to differentiate transitory from resilient issues and to study whether the meaning of resilient issues has changed over time. Scarborough (2018) used supervised learning methods on Twitter to estimate attitudes toward feminism, concluding that Twitter could be a useful measure of public opinion about gender, although sentiment, as captured on Twitter, is not fully representative of the general population. Bilbao-Jayo and Almeida (2018) proposed a multi-scale convolutional neural networks model on classifying party manifestos that could be potentially used to automate the analysis of the political discourse to allow public administrations to better react to their needs and claims. Da Silva et al. (2021) used deep learning based embedding in topic modeling to online comments regarding two legislative proposals in Brazil's Chamber of Deputies and demonstrated the applicability of the topic modeling tools for discovering latent topics that could be potentially used to increase citizen engagement regarding governmental policies.
Taking such insights to the field of VAAs, researchers have proposed to introduce AI in the process of statement selection, by automating the search for salient issues that can be used for formulating questions in VAAs. Buryakov et al. (2022a) proposed to assist the process of selecting statements by looking at the supply side of electoral competition. Taking Japan as a case example, they proposed to use the Bidirectional Encoder Representations from Transformer (BERT) natural language processing model which takes into account the context of each word, in order to tokenize the sentences in party manifestos and apply topic modeling afterward. To determine which of the topics were most relevant, Buryakov et al. (2022a) calculated the semantic similarity between the computed topics and statements from VAAs that were available in Japan during the previous two elections, and concluded that the results were particularly useful to the VAA designers to identify new issues that were relevant in the election under examination and formulate new statements that were used in a new VAA.
Taking the same method to the demand side of political competition, Buryakov et al. (2022b) applied the BERT model to e-petitions for policy proposals data from Taiwan, a government initiative that aims to reinforce communication between the citizens and the government. Just like in the case of the supply side data, the authors found that the generated statements could be of use to VAA designers, even though the study results were not used in an actual VAA.
One could easily imagine that VAA designers could expand this method of AI-assisted statement selection and formulation to other textual sources from both the supply side, such as parliamentary debates, and the demand side such as social media or blog posts. For instance, Andreadis (2017) explored the possibility of mining Twitter data across the electorate as an additional measure to determine issue salience as a guide for statement selection in VAAs. In addition, VAA designers can also explore issue salience as mediated by the traditional media by using media text corpora as data. Of course, each data source comes with its caveats in terms of how representative it is. This is principally relevant for gauging the preferences of the general public of which social media is particularly not representative. Moreover, determining the relevance of topics based on previous VAA statements can be restrictive. This means that researchers and VAA designers should be more creative with the data that will be used as inputs in the Buryakov et al. (2022a) method. For instance, one could use seed words from content analysis dictionaries generated from media sources instead of previous VAA statements, and responses to open-ended questions in a survey on a nationally representative sample instead of social media posts as the two inputs in the method.
Given the lack of benchmarks in terms of what the public considers to be important in different electoral contexts, which is also probably different from issue salience across political parties, the media, and the minds of VAA designers, the use of AI might offer very promising tools in selecting and formulating statements in VAAs, that could lead to “a re-emphasis of the core of the voting advice application as a civic tech tool, the policy statements themselves” (Buryakov et al., 2022b, p. 31).
A particularly innovative use of AI in the design of VAAs regards the use of conversational agents in helping users to better understand the statements used in VAAs. The task of assisting VAA users in their understanding of political issues lies at the heart of the function of VAAs as providers of political information on pertinent issues to reduce information asymmetries.
Until very recently the evaluation of whether VAAs help citizens acquire political information beyond the “political match” output was viewed as a byproduct of VAA usage rather than the main goal. For instance, Schultze (2014) found that using Wahl-O-Mat in Germany enhanced political knowledge of party positions on political issues, whereas in another intervention where Wahl-O-Mat was embedded in civic education classes Waldvogel et al. (2023) found a significant increase in young people's political efficacy and specific interest in the election campaign. Furthermore, Brenneis and Mauve (2021) explored the use of statements that presented specific arguments alongside the typical policy positions in a VAA. The results indicated that while users reported a better understanding of political issues and enjoyed the interaction with argument-based statements, but the matching algorithm that incorporated the argument-based statements did not improve the voting recommendations.
Against this backdrop, Kamoen and Liebrecht (2022) pioneered the use of conversational agents in VAAs, where chatbots were employed with the specific goal of assisting VAA users in better understanding the meaning of the statements employed by VAAs. More specifically, the chatbots were used as an auxiliary feature that allowed users to ask questions and obtain additional information on the statements used in the VAA (see Figure 2). The findings suggest that chatbot-assisted VAAs lead to higher user satisfaction, as did the provision of additional information through buttons in a structured user interface (Kamoen and Liebrecht, 2022). Furthermore, in additional randomized experiments, the authors explored the optimal chatbot design in terms of its structure (buttons, text field, or both), modality (text, voice, or both), and tone (formal or informal) (Kamoen et al., 2022; Liebrecht et al., 2022), and concluded that the use of these Conversational Agents VAAs (CAVAAs) improved the user experience, increased perceived and factual political knowledge, but did not have an impact on intentions to vote in the upcoming election (Kamoen and Liebrecht, 2022; van Zanten and Boumans, 2023).
Figure 2. Examples of a non-structured (A) and structured (B) conversational agent VAA (Figure from Kamoen and Liebrecht, 2022).
The use of conversational AI in VAAs provides a very promising approach to helping users understand the statements in VAAs, especially inasmuch as the effects on political knowledge are not mediated by political sophistication (i.e. affecting primarily those who are politically sophisticated). Interestingly, however, Kamoen and Liebrecht (2022) did not discuss the potential limitations of chatbots when it comes to concerns about political bias, an issue that has been pervasive in discussions about AI and politics. The lack of discussion can be justified in the context of the aforementioned research as the chatbot used by Kamoen and Liebrecht (2022) was trained to recognize questions and provide the user with a preformulated response.
What would happen, however, if one wished to extend the use of chatbots in VAAs by allowing users to interact with the chatbot freely? One could easily imagine users asking the chatbot to suggest a reasonable position on statements they have difficulty deciding upon or comprehending. In this respect, the findings are telling. Hartmann et al. (2023) prompted ChatGPT with 630 political statements from two VAAs and another voter information tool in three pre-registered studies in Germany, and found that ChatGPT has a pro-environmental, left-libertarian ideology. If prompted on what position to take, ChatGPT would be, for instance, in favor of imposing taxes on flights, restricting rent increases, and legalizing abortion. These results were confirmed in similar studies conducted in Canada (Sullivan-Paul, 2023) that revealed that ChatGPT has become even more left-leaning in its newer version, especially when prompted with questions regarding economic issues. More generally, and beyond ChatGPT, research has shown that the open-domain chatbots, that are most likely to be used in voter information tools, have a long way to go before they are considered to be unbiased and “politically safe” (Bang et al., 2021).
Overall, AI shows considerable promise as a supplementary information provider where conversational agents can be integrated into VAAs to provide information on demand to assist users who have difficulty comprehending VAA statements. As such, chatbots could contribute to reducing information asymmetries, although there exist barriers inasmuch trustworthiness is affected by fears that chatbots could provide biased or limited information about societally important issues (Väänänen et al., 2020).
One of the biggest methodological challenges in the design of VAAs is to find an unbiased and reliable method of estimating the positions of political political actors on the statements that are used for matching the users to the political actors in VAAs. For several decades, the primary reference source for party policy preferences in political science has been the data produced by the Manifesto Project using a laborious hand-coding that was developed in the late 1970s (see Volkens et al., 2013). The hand-coding of the Manifesto Project, however, is unreliable according to conventional content analysis standards, and it uses pre-defined policy categories that indicate the relative emphasis parties place on different issues instead of their expressed position on issues which is of interest to VAAs (Gemenis, 2013b).
For these reasons, VAA designers have developed ad hoc approaches to estimate the positions of political actors. In VAAs that are particularly popular and used by a considerable proportion of the electorate, such as Stemwijzer in the Netherlands and Wahl-O-Mat in Germany, the designers can enlist political parties to state and justify their official position on each of the statements used in the VAAs. Moreover, candidates have particular incentives to respond to such requests in those VAAs that compare the positions of users to those of individual candidates instead of parties, as they could receive exposure and potential electoral gains (Dumont et al., 2014). Again, this is particularly the case of popular candidate-centered VAAs such as Smartvote in Switzerland, and Vaalikone in Finland.
Given that political parties will often refuse or ignore such requests for positioning, VAA designers often resort to using the mean position obtained in expert surveys (e.g. Wall et al., 2009), or candidate surveys (see Andreadis and Giebler, 2018), a combination of party self-placement and placement by country experts (Trechsel and Mair, 2011; Krouwel et al., 2012; Garzia et al., 2017a), or the estimation of party positions by coders over multiple rounds in a Delphi survey (Gemenis, 2015; Gemenis et al., 2019). While one can argue about the advantages of either method over the others (Gemenis and van Ham, 2014), their common feature is that they rely heavily on human input, something that is considered by VAA designers as a prerequisite for establishing the validity of the estimates.
AI has brought the use of different supervised and unsupervised learning algorithms such as Wordscores (Laver et al., 2003), Wordfish (Slapin and Proksch, 2008), and SemScale (Nanni et al., 2022), methods that alternate between machine learning and human coding (Wiedemann, 2019), multi-scale convolutional neural networks that are enhanced with context information (Bilbao-Jayo and Almeida, 2018), and semi-supervised machine learning models that employ word-embedding techniques (Watanabe, 2021) in the analysis of party manifestos. Initially the methods showed promise to automate the process of estimating the policy positions of political parties on dimensions of interest, even though the classification of the manifesto content by machine learning algorithms corresponds to that of human coding only moderately. Moreover, machine learning estimates of party policy positions have been shown to lack validity, especially in estimating positions on specific policy statements employed in VAAs (Bruinsma and Gemenis, 2019; Ruedin and Morales, 2019). As Orellana and Bisgin (2023) argue, AI even in the context of the more advanced Natural Language Processing (NLP) methods is well suited for tasks such as estimating the similarity between documents, identifying the topics discussed in documents, and sentiment analysis, tasks entirely different to the estimation of precise positions on very specific topics that are typically featured in VAAs.
In this direction, Terán and Mancera (2019) introduced the idea of “dynamic candidate profiles” as an additional element to the static generation of candidate positions in typical VAAs. In “dynamic profiles” the positions of candidates are estimated dynamically throughout the election campaign using Twitter data. Terán and Mancera (2019) applied the method of “dynamic profiles” in the Participa Inteligente project, a social networking platform designed for the 2017 parliamentary elections in Ecuador. After assigning the words of the Twitter data corpus to relevant policy issues manually, they performed sentiment analysis on the Tweets mined from candidate profiles and the Tweets where the candidates were tagged, to determine whether candidates had a positive or negative stance on the issues associated with the keywords. In the end, each candidate's position in the VAA was calculated as the average between this dynamic estimation and a static estimation obtained by expert coding.
Nigon et al. (2023) extended this work by adding YouTube as a source for candidate profiling. Moreover, they proposed estimating the positions of candidates in a VAA using a data-driven NLP model with pre-trained question-answering (QA) models. Using VAA statements as questions and translated Twitter and YouTube data from politicians as context to predict the answer the politicians could have given. The results indicated that in most cases QA models are unable to provide conclusive answers to the VAA questions.
Terán and Mancera (2019) recognize the limitations of the “dynamic profiles” in terms that Twitter users can influence the position of political actors by coordinating the posting of compromising messages where candidates are tagged, often by using Internet bots. Moreover, in the case of QA models, the lack of quality data in politicians' social media profiles can adversely impact the accuracy of the profiles constructed by the data-driven model. More generally, the use of AI to automate or enhance the estimation of policy positions of political actors has not produced results of sufficient validity for use in VAAs (see Bruinsma and Gemenis, 2019). More importantly, the AI text analysis algorithms are not directly accessible or easily understandable by users, which makes the use of AI methods problematic in terms of the typical standards set by VAA designers and the call for more transparency in VAA design as in the “Lausanne Declaration on VAAs” (see Garzia and Marschall, 2014).
Without a doubt, the most controversial and debated aspect of VAA design has been the search for the optimal way to match each user to each party or candidate in a VAA. Broadly speaking, the debates about the “matching algorithms”, understood here simply as a set of instructions for calculating the match metric between the user and each of the parties or candidates in a VAA, mostly focus on four aspects: dimensionality, the distance metric, the use of weights, and the handling of missing values. More recently, this debate has been further complicated with the introduction of methods from the statistical/machine learning perspective where the algorithms are trained or calibrated based on contextual or past VAA user response data, and the outright use of AI with recommender systems.
The debate about dimensionality focuses on whether a low-dimensional solution, in which the responses/positions on VAA statements are aggregated into a small number of dimensions, before the distance metric is calculated, is preferable to a high-dimensional solution, where every statement is entered separately in the matching calculations (see Figure 3). In essence this difference is reflected in the visual output of the VAA (see Bruinsma, 2020b) with low-dimensional algorithms presented in the “spider” graphs of Smartvote in Switzerland and the two-dimensional maps of Kieskompas in the Netherlands and its various offshoots in many other countries, and high-dimensional algorithms presented through the ranking of parties as in Stemwijzer in the Netherlands, Wahl-O-Mat in Germany, and several VAAs in other countries.
Figure 3. Examples of recommendations based on a high-dimensional algorithm (Parteienavi 2013, top) and two-dimensional map (Kieskompas 2010, bottom).
From a mathematical (and ethical) standpoint, high-dimensional algorithms should be preferable to algorithms based on summated scales in a low-dimensional space as used in the Kieskompas family of VAAs, since the latter tend to produce misleading results near the center of the dimensions as the summation of opposing responses falsely assumes all items of the scale to be interchangeable (for an example, see Gemenis, 2012). To improve the visualization of matching in a candidate-based VAA in Switzerland, Terán et al. (2012) used fuzzy c-means (FCM) clustering to compute similarities based on distances in a high-dimensional space, but also to derive a two-dimensional space that included the percentage of similarity of the n-closest candidates. From a psychometric perspective, the matching using a low-dimensional solution can be improved through a careful examination of how different statements map onto different dimensions (if at all). Rather than hoping that the intuition of VAA designers is correct (and usually it is not), Germann and Mendez (2016) propose to evaluate and calibrate the selection of statements that make up the dimensions by performing a Mokken scale analysis on early VAA user response data. While this suggestion does not employ AI per se, it does employ the logic of statistical/machine learning by using past data as a benchmark to evaluate intuitively defined spatial maps.
Contrary to the low-dimensional matching algorithms that explicitly or implicitly use the Euclidean distance as a metric to calculate the matches, high-dimensional algorithms have a host of metric distances to consider. For instance, Stemwijzer uses a very simple distance matrix that awards one point if the position of the party and user are identical, while no points are awarded if both have different positions or if a user is neutral and a party agrees/disagrees with a statement (Louwerse and Rosema, 2014, p. 289). Wahl-O-Mat uses the city-block distance metric as presumed by the proximity model of issue voting, while Choose4Greece and other VAAs designed by the PreferenceMatcher consortium employ a matching algorithm that uses the average between the city block and the scalar product metric of the directional theory of issue voting (see Mendez, 2012). The EU Profiler and EUandI for the 2009 and 2014/2019 elections to the European Parliament respectively, used matching algorithms based on empirically defined distance matrices (Garzia et al., 2015). In addition, Dyczkowski and Stachowiak (2012) proposed a matrix based on the Hausdorff distance which is defined as the maximum distance of a set to the nearest point in the other set. Louwerse and Rosema (2014) consider a few other algorithms that are not currently used by VAAs, while one could imagine that VAAs could also use algorithms with distance matrices based on correlation or agreement coefficients. In particular, Katakis et al. (2013) argue that the Mahalanobis distance metric can provide more accurate recommendations as it takes into account the correlation between statements, although it requires prior VAA user response data to form the covariance matrix which establishes the correlations between the statements. Finally, Mendez (2017) explored the possibility of supplementing the matching algorithm with user demographic data typically collected by VAAs, although the proposal was not fielded in an actual VAA.
The debate regarding the use of weights is also an interesting one as it has gravitated from simple solutions to the use of machine learning methods. Stemwijzer, Wahl-O-Mat and other VAAs use a simple system where users can choose the statements that are more important for them, and the relevant scores are doubled in the distance matrix. Pajala et al. (2018) proposed a more complex algorithm in which the weights are calculated through regression coefficients in models that use as variables the candidates' social media activity, newspaper mentions, and political power operationalized by incumbency status, and applied the approach in a VAA for the 2015 parliamentary election in Finland designed in collaboration with Helsingin Sanomat, the largest daily newspaper in the country.
Directly relevant to AI is the concept of the “learning VAA” introduced by Romero Moreno et al. (2022) which uses machine learning to estimate both the distance matrix and the weights in the matching algorithm. To do so, (Romero Moreno et al., 2022) employ user response data from two supplementary questions that feature prominently in all VAAs designed by PreferenceMatcher: voting intention and the reasoning behind the voting intention. They, therefore, use self-identified issue voters' voting intention to train their model by estimating the distance and weight parameters for the algorithm so the parameters predict issue-voters' voting intentions with the highest accuracy. The advantage of the “learning VAA” approach is that it allows the spatial voting logic to be derived directly from the data Romero Moreno et al. (2022) and to reveal how VAA statements are perceived by users, which could be useful for VAA designers and researchers in the field of spatial voting. The downside of the “learning VAA” approach is that it does not guarantee the creation of meaningful distance matrices when the input VAA user response data are of low quality, which is often the case when VAA statements are poorly formulated (see Gemenis, 2013a; van Camp et al., 2014; Bruinsma, 2021).
Even more relevant from an AI perspective, is the use of recommender systems in VAAs. In this direction, Katakis et al. (2013) pioneered the so-called “social VAAs” that make voting recommendations without using the policy positions of political actors as input. Following Katakis et al. (2013, p. 1044–1046), I distinguish among three approaches that use, respectively, clustering, classifiers, and collaborative filtering.
The first use of machine learning in “social VAAs” involved clustering. This typically employed a k-means clustering technique that groups users into like-minded groups based on their responses to the VAA statements and provided voting recommendations based on the distribution of voting intention of the most similar users (Katakis et al., 2013, p. 1044–1045). This method was used in the 2012 Choose4Greece VAA and EUvox at the 2014 elections to the European Parliament.
Alternatively, by conceptualizing the matching algorithm as a machine-learning task of predicting voting intention, VAA designers can train different classifiers on a sample of early VAA users and provide voting recommendations based on voting intention predicted probabilities for each party included in the VAA (Katakis et al., 2013, p. 1045–1046). In this direction, Tsapatsoulis et al. (2015) used the Mahalanobis distance classifier to explore the optimal sample sizes for the training and test sets across different countries with varying numbers of parties and distributions of voters across parties. Moreover, since the policy statements in VAAs are usually correlated and grouped into categories (e.g., economy, foreign affairs, etc) one can apply Hidden Markov Model (HMM) classifiers, as typically used in correlated time-series data, to estimate the party-user similarity to improve the effectiveness of “social recommendations” (Agathokleous and Tsapatsoulis, 2016). Despite these proposals, no VAA to date has conceptualized voting recommendations purely as a machine-learning task.
A more intuitive method to provide a machine learning “community recommendation” is to use collaborative filtering. This can be achieved, for instance, by computing a correlation coefficient between the response of the user and those of the average supporter across all statements, for each party included in the VAA (Katakis et al., 2013, p. 1044). This method that has been used in the Choose4Greece and Choose4Cyprus VAAs since 2019 in parallel to the typical output based on VAA user-party matching algorithm.
With regard to how algorithms handle the missing values, here understood as the instances when users provide a “no opinion” answer to a statement or when it is not possible to find a statement position for a political actor, Agathokleous et al. (2013) observed that the voting recommendation accuracy drops significantly when matching is based on machine learning classifiers, although the matching algorithms that use party-actor distance matrices perform considerably better. More generally, missing values on the user side are always considered legitimate, indicating an explicit non-preference from the point of the user, whereas missing values from the side of the political actors are often indicative of uncertainty in the estimation process. Since VAAs feature a limited number of parties, a large number of missing values in parties' responses to statements can have an adverse impact on the quality of the voting recommendation. VAA designers therefore suggest either replacing missing values on the parties' side with the middle “neither agree, nor disagree” response, as in the EU Profiler and EUandI VAAs (Garzia et al., 2015), or divide the sum of matching weights with the party by the number of statements to which the user has responded (Mendez, 2017, p. 50–51) as in the PreferenceMatcher VAAs. The latter approach effectively penalizes parties for their missing responses.
The pitfalls of incorporating AI methods in the design of the VAA matching algorithm are rather apparent. In Pajala et al. (2018), the inclusion of incumbency in the weighting function of the matching algorithm proved to be disadvantageous for the challenger candidates, providing a bias for the political status quo and powerful political elites. In Katakis et al. (2013), the classifier-based matching algorithms did not perform particularly well, which is telling of the perils of treating voting intentions as a simple machine-learning task that assumes every person to be an issue voter, effectively disregarding all other theories of vote choice.
In general, the use of AI methods in the matching algorithm suffers from opaqueness. Their opaqueness prevents researchers from detecting spurious classifications due to overfitted models, and hinders the accountability of their application to the users, who may eventually lose their trust in VAAs. Even in the more simple “learning VAA” of Romero Moreno et al. (2022), users can only interpret the algorithms if they are given the distance matrices and salience weights and perform the calculations themselves, which is rather a complex task. One should note that AI has only exacerbated already existing deficiencies in communicating the more complex aspects of the matching algorithms used in VAAs, such as how spatial maps are constructed, the handling of missing values (that could lead to erroneous inferences or penalize parties that do not have positions on several of the VAA statements), and many other issues that have been presented above.
AI methods have been also used in (pre-)processing the data generated by VAA users, an area which is important inasmuch VAA user response data is used in VAA design and the evaluation of the impact of VAAs. Indeed, user response data has been used extensively to assess ex-post the effectiveness of the design (e.g., Mendez, 2012; Alvarez et al., 2014; Louwerse and Rosema, 2014) and the electoral effects (e.g., Kamoen et al., 2015; Gemenis, 2018; Germann et al., 2023) of VAAs, to train machine learning models in “social VAAs” (Katakis et al., 2013; Tsapatsoulis et al., 2015), and to dynamically calibrate VAA matching algorithms (Germann and Mendez, 2016; Romero Moreno et al., 2022).
With regard to data processing, AI has been used to pre-preprocess the VAA user response data by removing suspect entries (see Andreadis, 2014; Mendez et al., 2014). For instance, Djouvas et al. (2016), used unsupervised data mining techniques to identify “rogue” entries that were injected into the data at random with considerable success, although data mining techniques were unable to identify suspect entries that are neither random nor clustered. Despite the promising findings, the analysis of user-generated data is still hindered by the fact that some of the most prominent VAA designers are still unwilling to openly share VAA data, leading to replication and transparency concerns.
One of the overlooked aspects of VAAs concerns how VAAs reach the public. Even though the archetypical VAA is designed and fielded by a public civic education agency (e.g. Stemwijzer, Wahl-O-Mat), a public broadcaster (e.g., Vaalikone), or an entity that features the necessary prestige and visibility for the VAA to reach a considerable number of users at all walks of life, many VAAs are designed by researchers in academic institutions lack the means to engage their successful dissemination. VAA designers therefore engage in a media campaign or, as in the case of Kieskompas and its offshoots in many other countries, they seek a media partner, often with exclusive hosting rights for the VAA (see Krouwel et al., 2014). As this strategy often comes at the cost of uneven delivery to different strata of the population, given the media's ideological bias, and a heavy skew in the user response data, researchers have turned to disseminating their VAAs through paid advertising on social media.
Advertising on social media has emerged as a cost-effective method to enlist respondents in social science surveys. Facebook ads, in particular, offer a quick and affordable way to obtain large samples of social media users (Boas et al., 2020; Zhang et al., 2020; Gemenis, 2023; Neundorf and Öztürk, 2023). In this case, the use of AI concerns the use of ad delivery algorithms by advertising platforms. Like other social media platforms, Facebook employs algorithms to optimize ad delivery by favoring users who are most likely to click on them and, as a result, the characteristics of the end samples are likely to differ from the underlying population. Facebook ads tend to target audiences that are disproportionately dominated by older, male, college graduates (Neundorf and Öztürk, 2023), which is the typical VAA audience in the first place (Marschall, 2014).
While there exists some anecdotal evidence that social media advertising and its cascade effects can result in samples with a less skewed distribution of political interest compared to typical VAA dissemination campaigns, it is unlikely that social media campaigns alone can overcome the uneven coverage in VAAs. The uneven targeting by social media advertising algorithms and the well-known homophily effect among sharers and followers in social media, both of whom tend to be similar in terms of political interest, ensure there is only a limited trickle-down effect to those harder-to-penetrate groups of politically disinterested. The potential of social media advertising can only be realized with stratified advertising campaigns and randomized experiments to identify the most effective promotional campaign to engage the politically disinterested.
VAAs represent a significant innovation of ICTs to re-engage people with the democratic process, offering a technologically-driven approach to voter information and decision-making. While AI can potentially enhance the user experience with VAAs, it is essential to address the potential pitfalls of AI methods.
With regard to the formulation and selection of statements, the use of text-mining offers exciting possibilities to harness big data for social change. Rather than reinforcing the same researcher biases by recycling the same old questions originally developed for election studies and social surveys in the 1970s, VAA designers have a real opportunity to use text mining to uncover the issues that are salient for the public that might otherwise be underexposed due to political and/or media biases.
The use of AI as a supplementary information provider appears to be a promising way to decrease comprehension problems when it comes to statements used in VAAs. Conversational agents can help mitigate information disparities in line with the VAAs' broader goal of empowering citizens in political processes. Chatbots, however, are not politically neutral and researchers need to exercise considerable care in their implementation of conversational agents in VAAs.
The estimation of the policy positions of political actors is the area of VAA design that still needs human input as it cannot be automated effectively with AI methods. Even the most sophisticated text mining and NLP methods lack the level of validity required in VAAs as tools that are used (and often taken seriously) by millions of people. VAA users have trouble trusting, let alone understanding, methods that are considered opaque. The task of placing a handful of political parties on a handful of very specific policy statements is something that humans can perform fairly fast with sufficient validity. In the case of candidate-based VAAs where estimation is a time-consuming task, candidates should have serious incentives to participate and provide their positions on each of the statements themselves.
A lot can be said about the use of AI in the matching algorithms employed in VAAs. The evaluation of the use of chatbots as an enhancement of the typical VAA has been so far positive, and one can also argue that the user experience can be further improved by including enhancements based on the most intuitive use of machine learning in “social voting recommendations” (e.g., the comparison to the average party voter). Beyond these and the light use of AI-inspired methods to calibrate the typical VAA features, machine learning lacks the methodological transparency that is required in voter information tools.
The inability of the lesser-known VAAs to penetrate the low political interest groups through the traditional means of dissemination cannot be effectively resolved through the use of social media advertising. The AI algorithms employed by platforms such as Facebook Ads are subject to market effects and other biases and the use of social media advertising runs the risk of enhancing rather than alleviating the existing participatory inequalities. It seems therefore that the use of AI alone is unable to remedy the biased coverage of traditional media campaigns in the dissemination of VAAs, unless careful stratification and experimentation are involved. Moreover, there is a real need for generous public funding for VAAs, as agents of civic education with measurable impacts, beyond the meager budgets of individual researchers who typically cannot afford to engage in extensive experimentation beyond the black box algorithm.
Finally, any evaluation of AI methods should be subject to experimental evaluations where the dependent variable of interest is some measurable aspect of user experience or societal impact. Evaluating machine learning algorithms on whether they can predict vote intentions is trivial. What we need to know is whether the proposed change in the design of a VAA has an impact on the user experience, political knowledge, or participation, with a specific focus on alleviating the inequalities therein.
The use of AI in the design, dissemination, and evaluation of VAAs both offers promises and poses risks and the assessment depends on the specific area under scrutiny. The way forward is in the continuous evaluation of AI methods through a proper bench-marking with ethical and properly randomized experiments. As technology continues to shape political processes, the careful implementation of AI will help ensure that VAAs will continue to contribute positively to the democratic processes.
KG: Writing – original draft, Writing – review & editing.
The author(s) declare that no financial support was received for the research, authorship, and/or publication of this article.
The author declares that the research was conducted in the absence of any commercial or financial relationships that could be construed as a potential conflict of interest.
All claims expressed in this article are solely those of the authors and do not necessarily represent those of their affiliated organizations, or those of the publisher, the editors and the reviewers. Any product that may be evaluated in this article, or claim that may be made by its manufacturer, is not guaranteed or endorsed by the publisher.
2. ^The 2019 general election version can be found at: https://www.bbc.com/news/election-2019-50291676 Previous versions are typically archived on the BBC website.
3. ^Autoriteit Persoonsgegevens, “Stemhulpen verhogen beveiliging na optreden AP,” 17 February 2017.
4. ^“German court bans popular voting advice app Wahl-O-Mat,” Deutsche Welle, 20 May 2019.
Agathokleous, M., and Tsapatsoulis, N. (2016). Applying hidden Markov models to voting advice applications. EPJ Data Sci. 5, 1–19. doi: 10.1140/epjds/s13688-016-0095-z
Agathokleous, M., Tsapatsoulis, N., and Katakis, I. (2013). “On the quantification of missing value impact on voting advice applications,” in Engineering Applications of Neural Networks: 14th International Conference (EANN 2013), Part I 14 (Cham: Springer), 496–505.
Ahonen, P., and Koljonen, J. (2018). “Transitory and resilient salient issues in party manifestos, Finland, 1880s to 2010s: Content analysis by means of topic modeling,” in Social Informatics: 10th International Conference (SocInfo 2018), Part I 10 (Cham: Springer), 23–37.
Alvarez, R. M., Levin, I., Trechsel, A. H., and Vassil, K. (2014). Voting advice applications: how useful and for whom? J. Inform. Technol. Polit. 11:82–101. doi: 10.1080/19331681.2013.873361
Anderson, J., and Fossen, T. (2014). “Voting advice applications and political theory: Citizenship, participation and representation,” in Matching Voters to Parties and Candidates, eds. D. Garzia, and S. Marschall (Colchester: ECPR Press), 217–226.
Andı, S., Çarkoğlu, A., and Banducci, S. (2023). Closing the information gap in competitive authoritarian regimes? The effect of voting advice applications. Elect. Stud. 86, 102678. doi: 10.1016/j.electstud.2023.102678
Andreadis, I. (2013). “Voting advice applications: A successful nexus between informatics and political science,” in Proceedings of the 6th Balkan Conference in Informatics, 251–258.
Andreadis, I. (2014). “Data quality and data cleaning,” in Matching Voters to Parties and Candidates, eds. D. Garzia, and S. Marschall (Colchester: ECPR Press), 79–91.
Andreadis, I. (2017). “Using Twitter to improve the design of voting advice applications,” in Paper Presented at the ECPR General Conference (Oslo: University of Oslo), 6–9.
Andreadis, I., and Giebler, H. (2018). Validating and improving voting advice applications: Estimating party positions using candidate surveys. Statist. Polit. Policy 9, 135–160. doi: 10.1515/spp-2018-0012
Bang, Y., Lee, N., Ishii, E., Madotto, A., and Fung, P. (2021). Assessing Political Prudence of Open-Domain Chatbots. Available online at: https://arxiv.org/pdf/2106.06157.pdf
Benesch, C., Heim, R., Schelker, M., and Schmid, L. (2023). Do voting advice applications change political behavior? J. Polit. 85, 684–700. doi: 10.1086/723020
Bilbao-Jayo, A., and Almeida, A. (2018). Automatic political discourse analysis with multi-scale convolutional neural networks and contextual data. Int J Distrib Sensor Netw. 14, 11. doi: 10.1177/1550147718811827
Boas, T. C., Christenson, D. P., and Glick, D. M. (2020). Recruiting large online samples in the United States and India: Facebook, Mechanical Turk, and Qualtrics. Polit. Sci. Res. Meth. 8, 232–250. doi: 10.1017/psrm.2018.28
Brenneis, M., and Mauve, M. (2021). “Argvote: Which party argues like me? Exploring an argument-based voting advice application,” in Intelligent Decision Technologies: Proceedings of the 13th KES-IDT 2021 Conference (Cham: Springer), 3–13.
Bruinsma, B. (2020a). A comparison of measures to validate scales in voting advice applications. Qual. Quant. 54, 1299–1316. doi: 10.1007/s11135-020-00986-8
Bruinsma, B. (2020b). Evaluating visualisations in voting advice applications. Stat. Polit. Policy 11, 1–21. doi: 10.1515/spp-2019-0009
Bruinsma, B. (2021). Challenges in comparing cross-country responses in voting advice applications. J. Elect. Public Opin. Parties 34, 41–58. doi: 10.1080/17457289.2021.2001473
Bruinsma, B. (2023). Measuring congruence between voters and parties in online surveys: Does question wording matter? Meth, Data, Analy. 17, 21. doi: 10.12758/mda.2022.12
Bruinsma, B., and Gemenis, K. (2019). Validating Wordscores: The promises and pitfalls of computational text scaling. Commun. Methods Meas. 13, 212–227. doi: 10.1080/19312458.2019.1594741
Buryakov, D., Hino, A., Kovacs, M., and Serdült, U. (2022a). “Text mining from party manifestos to support the design of online voting advice applications,” in 2022 9th International Conference on Behavioural and Social Computing (BESC) (Matsuyama: IEEE), 1-7.
Buryakov, D., Kovacs, M., Kryssanov, V., and Serdült, U. (2022b). “Using open government data to facilitate the design of voting advice applications,” in International Conference on Electronic Participation (Cham: Springer), 19–34.
Cammaerts, B., Bruter, M., Banaji, S., Harrison, S., Anstead, N., et al. (2016). Youth Participation in Democratic Life: Stories of Hope and Disillusion. London: Palgrave Macmillan.
Christensen, H. S., Järvi, T., Mattila, M., and von Schoultz, Å. (2021). How voters choose one out of many: a conjoint analysis of the effects of endorsements on candidate choice. Polit. Res. Exch. 3, 1. doi: 10.1080/2474736X.2021.1892456
Da Silva, N. F., Silva, M. C. R., Pereira, F. S., Tarrega, J. P. M., Beinotti, J. V. P., Fonseca, M., et al. (2021). “Evaluating topic models in Portuguese political comments about bills from Brazil's Chamber of Deputies,” in Intelligent Systems: 10th Brazilian Conference (BRACIS 2021), Part II 10 (Cham: Springer), 104–120.
De Graaf, J. (2010). “The irresistible rise of the StemWijzer,” in Voting Advice Applications in Europe: The State of the Art, eds. L. Cedroni, and D. Garzia 35–46.
Djouvas, C., Mendez, F., and Tsapatsoulis, N. (2016). Mining online political opinion surveys for suspect entries: An interdisciplinary comparison. J. Innovat. Digit. Ecosyst. 3, 172–182. doi: 10.1016/j.jides.2016.11.003
Dumont, P., Kies, R., and Fivaz, J. (2014). “Being a VAA candidate: Why do candidates use voting advice applications and what can we learn from it,” in Matching Voters to Parties and Candidates, eds. D. Garzia, and S. Marschall (Colchester: ECPR Press), 145–159.
Dutton, W. H. (1992). Political science research on teledemocracy. Soc. Sci. Comput. Rev. 10, 505–522. doi: 10.1177/089443939201000405
Dyczkowski, K., and Stachowiak, A. (2012). “A recommender system with uncertainty on the example of political elections,” in International Conference on Information Processing and Management of Uncertainty in Knowledge-Based Systems (Cham: Springer), 441–449.
Eibl, O. (2019). “Summary for the Baltic states,” in Thirty Years of Political Campaigning in Central and Eastern Europe, eds. O. Eibl, and M. Gregor (London: Palgrave Macmillan) 83–85.
Enyedi, Z. (2016). The influence of voting advice applications on preferences, loyalties and turnout: an experimental study. Polit. Stud. 64, 1000–1015. doi: 10.1111/1467-9248.12213
Fivaz, J., and Nadig, G. (2010). Impact of voting advice applications (VAAs) on voter turnout and their potential use for civic education. Policy Inter. 2, 167–200. doi: 10.2202/1944-2866.1025
Fossen, T., and Anderson, J. (2014). What's the point of voting advice applications? Competing perspectives on democracy and citizenship. Elect. Stud. 36, 244–251. doi: 10.1016/j.electstud.2014.04.001
Fossen, T., and van den Brink, B. (2015). Electoral dioramas: on the problem of representation in voting advice applications. Representation 51, 341–358. doi: 10.1080/00344893.2015.1090473
Garry, J., Tilley, J., Matthews, N., Mendez, F., and Wheatley, J. (2019). Does receiving advice from voter advice applications (VAAs) affect public opinion in deeply divided societies? Evidence from a field experiment in Northern Ireland. Party Polit. 25, 854–861. doi: 10.1177/1354068818818789
Garzia, D. (2010). “The effects of VAAs on users' voting behaviour: An overview,” in Voting Advice Applications in Europe: The State of the Art, eds. L. Cedroni, and D. Garzia, 13–47.
Garzia, D., de Angelis, A., and Pianzola, J. (2014). “The impact of voting advice applications on electoral participation,” in Matching Voters to Parties and Candidates, eds. D. Garzia, and S. Marschall (Colchester: ECPR Press), 105–114.
Garzia, D., and Marschall, S. (2014). Matching Voters to Parties and Candidates: Voting Advice Applications in a Comparative Perspective (Colchester: ECPR Press).
Garzia, D., and Marschall, S. (2016). Research on voting advice applications: State of the art and future directions. Policy Inter. 8, 376–390. doi: 10.1002/poi3.140
Garzia, D., Trechsel, A., and De Sio, L. (2017a). Party placement in supranational elections: An introduction to the euandi 2014 dataset. Party Polit. 23, 333–341. doi: 10.1177/1354068815593456
Garzia, D., Trechsel, A. H., and De Angelis, A. (2017b). Voting advice applications and electoral participation: a multi-method study. Polit. Commun. 34, 424–443. doi: 10.1080/10584609.2016.1267053
Garzia, D., Trechsel, A. H., De Sio, L., and De Angelis, A. (2015). “euandi: Project description and datasets documentation,” in Robert Schuman Centre for Advanced Studies Research Paper No. 1.
Gemenis, K. (2012). “A new approach for estimating parties' positions in voting advice applications,” in Paper presented at the ‘Interdisciplinary Perspectives on Voting Advice Applications' Workshop (Limassol: Cyprus University of Technology), 23–24.
Gemenis, K. (2013a). Estimating parties' positions through voting advice applications: Some methodological considerations. Acta Politica 48, 268–295. doi: 10.1057/ap.2012.36
Gemenis, K. (2013b). What to do (and not to do) with the Comparative Manifestos Project data. Polit. Stud. 61, 3–23. doi: 10.1111/1467-9248.12015
Gemenis, K. (2015). An iterative expert survey approach for estimating parties' policy positions. Qual. Quant. 49, 2291–2306. doi: 10.1007/s11135-014-0109-5
Gemenis, K. (2018). The impact of voting advice applications on electoral turnout: evidence from Greece. Stat. Polit. Policy 9, 161–179. doi: 10.1515/spp-2018-0011
Gemenis, K. (2023). “Using Facebook targeted advertisements to recruit survey respondents,” in 18th International Workshop on Semantic and Social Media Adaptation and Personalization (SMAP 2023), 1–6. doi: 10.1109/SMAP59435.2023.10255213
Gemenis, K., Mendez, F., and Wheatley, J. (2019). Helping citizens to locate political parties in the policy space: a dataset for the 2014 elections to the European Parliament, in Research Data Journal for the Humanities and Social Sciences. doi: 10.1163/24523666-00401002
Gemenis, K., and Rosema, M. (2014). Voting advice applications and electoral turnout. Elect. Stud. 36, 281–289. doi: 10.1016/j.electstud.2014.06.010
Gemenis, K., and van Ham, C. (2014). “Comparing methods for estimating parties' positions in voting advice applications,” in Matching Voters to Parties and Candidates, eds. D. Garzia, and S. Marschall (Colchester: ECPR Press), 33–47.
Germann, M., and Gemenis, K. (2019). Getting out the vote with voting advice applications. Polit. Communic. 36, 149–170. doi: 10.1080/10584609.2018.1526237
Germann, M., and Mendez, F. (2016). Dynamic scale validation reloaded: assessing the psychometric properties of latent measures of ideology in VAA spatial maps. Qual. Quant. 50, 981–1007. doi: 10.1007/s11135-015-0186-0
Germann, M., Mendez, F., and Gemenis, K. (2023). Do voting advice applications affect party preferences? Evidence from field experiments in five European countries. Polit. Communic. 40, 596–614. doi: 10.1080/10584609.2023.2181896
Hansen, I., and Lim, D. J. (2019). Doxing democracy: influencing elections via cyber voter interference. Contemp. Polit. 25, 150–171. doi: 10.1080/13569775.2018.1493629
Hartmann, J., Schwenzow, J., and Witte, M. (2023). The Political Ideology of Conversational AI: Converging Evidence on ChatGPT's Pro-Environmental, Left-Libertarian Orientation. Available online at: https://arxiv.org/abs/2301.01768
Heinsohn, T., Fatke, M., Israel, J., Marschall, S., and Schultze, M. (2019). Effects of voting advice applications during election campaigns: Evidence from a panel study at the 2014 European elections. J. Inform. Technol. Polit. 16, 250–264. doi: 10.1080/19331681.2019.1644265
Holleman, B., Kamoen, N., Krouwel, A., Pol, J., Vreese, C., et al. (2016). Positive vs. negative: the impact of question polarity in voting advice applications. PloS ONE 11, e0164184. doi: 10.1371/journal.pone.0164184
Israel, J., Marschall, S., and Schultze, M. (2017). Cognitive dissonance and the effects of voting advice applications on voting behaviour: Evidence from the European elections 2014. J. Elect. Public Opin. Parties 27, 56–74. doi: 10.1080/17457289.2016.1268142
Kamoen, N., Holleman, B., and De Vreese, C. (2015). The effect of voting advice applications on political knowledge and vote choice. Irish Political Studies 30, 595–618. doi: 10.1080/07907184.2015.1099096
Kamoen, N., and Liebrecht, C. (2022). I need a CAVAA: How conversational agent voting advice applications (CAVAAs) affect users' political knowledge and tool experience. Front. Artif. Intellig. 5, 835505. doi: 10.3389/frai.2022.835505
Kamoen, N., McCartan, T., and Liebrecht, C. (2022). “Conversational agent voting advice applications: a comparison between a structured, semi-structured, and non-structured chatbot design for communicating with voters about political issues,” in International Workshop on Chatbot Research and Design (Cham: Springer), 160–175.
Katakis, I., Tsapatsoulis, N., Mendez, F., Triga, V., and Djouvas, C. (2013). Social voting advice applications–Definitions, challenges, datasets and evaluation. IEEE Trans. Cybern. 44, 1039–1052. doi: 10.1109/TCYB.2013.2279019
Kleinnijenhuis, J., van de Pol, J., van Hoof, A. M., and Krouwel, A. P. (2019). Genuine effects of vote advice applications on party choice: Filtering out factors that affect both the advice obtained and the vote. Party Politics 25, 291–302. doi: 10.1177/1354068817713121
König, P. D., and Jäckle, S. (2018). Voting advice applications and the estimation of party positions–a reliable tool? Swiss Polit. Sci. Rev. 24, 187–203. doi: 10.1111/spsr.12301
Krouwel, A., Vitiello, T., and Wall, M. (2012). The practicalities of issuing vote advice: a new methodology for profiling and matching. Int. J. Electr. Govern. 5, 223–243. doi: 10.1504/IJEG.2012.051308
Krouwel, A., Vitiello, T., and Wall, M. (2014). “Voting advice applications as campaign actors: Mapping VAAs' interactions with parties, media and voters,” in Matching Voters with Parties and Candidates, eds. D. Garzia, and S. Marschall (Colchester: ECPR Press), 67–78.
Laver, M., Benoit, K., and Garry, J. (2003). Extracting policy positions from political texts using words as data. Am. Polit. Sci. Rev. 97, 311–331. doi: 10.1017/S0003055403000698
Liao, D.-C., Chiou, W. B., Jang, J., and Cheng, S. H. (2020). “Strengthening voter competence through voting advice applications: An experimental study of the iVoter in Taiwan,” in Asian Education and Development Studies.
Liebrecht, C., Kamoen, N., and Aerts, C. (2022). “Voice your opinion! Young voters' usage and perceptions of a text-based, voice-based and text-voice combined conversational agent voting advice application (CAVAA),” in International Workshop on Chatbot Research and Design (Cham: Springer), 34–49.
Lin, F.-R., Chou, S.-Y., Liao, D., and Hao, D. (2015). “Automatic content analysis of legislative documents by text mining techniques,” in 2015 48th Hawaii International Conference on System Sciences (Kauai, HI: IEEE), 2199–2208.
Louwerse, T., and Rosema, M. (2014). The design effects of voting advice applications: Comparing methods of calculating results. Acta Politica 49, 286–312. doi: 10.1057/ap.2013.30
Mahéo, V.-A. (2016). The impact of voting advice applications on electoral preferences: a field experiment in the 2014 Quebec election. Policy Inter. 8, 391–411. doi: 10.1002/poi3.138
Mahéo, V.-A. (2017). Information campaigns and (under) privileged citizens: An experiment on the differential effects of a voting advice application. Polit. Communic. 34, 511–529. doi: 10.1080/10584609.2017.1282560
Manavopoulos, V., Triga, V., Marschall, S., and Wurthmann, L. C. (2018). The impact of VAAs on (non-voting) aspects of political participation: Insights from panel data collected during the 2017 German federal elections campaign. Stat. Polit. Policy 9, 105–134. doi: 10.1515/spp-2018-0008
Marschall, S. (2014). “Profiling users,” in Matching Voters to Parties and Candidates, eds. D. Garzia, and S. Marschall (Colchester: ECPR Press), 93–104.
Marschall, S., and Schultze, M. (2015). German E-campaigning and the emergence of a ‘digital voter'? An analysis of the users of the Wahl-O-Mat. German Polit. 24, 525–541. doi: 10.1080/09644008.2014.949681
Marzuca, A., Serdült, U., and Welp, Y. (2011). “Questão Pública: First voting advice application in Latin America,” in Electronic Participation: Third IFIP WG 8.5 International Conference (ePart 2011) (Cham: Springer), 216–227.
Mendez, F. (2012). Matching voters with political parties and candidates: an empirical test of four algorithms. Int. J. Electr. Govern. 5, 264–278. doi: 10.1504/IJEG.2012.051316
Mendez, F. (2017). Modeling proximity and directional decisional logic: What can we learn from applying statistical learning techniques to VAA-generated data? J. Elect. Public Opin. Parties 27, 31–55. doi: 10.1080/17457289.2016.1269113
Mendez, F., Gemenis, K., and Djouvas, C. (2014). “Methodological challenges in the analysis of voting advice application generated data,” in 9th International Workshop on Semantic and Social Media Adaptation and Personalization (SMAP 2014), 142–148. doi: 10.1109/SMAP.2014.32
Munzert, S., Guess, A., Barberá, P., and Yang, J. (2020). Do online voter guides empower citizens? Evidence from a field experiment with digital trace data. Public Opin. Quart. 84, 675–698. doi: 10.1093/poq/nfaa037
Munzert, S., and Ramirez-Ruiz, S. (2021). Meta-analysis of the effects of voting advice applications. Polit. Communic. 38, 691–706. doi: 10.1080/10584609.2020.1843572
Nanni, F., Glavaš, G., Rehbein, I., Ponzetto, S. P., and Stuckenschmidt, H. (2022). Political text scaling meets computational semantics. ACM/IMS Trans. Data Sci. (TDS) 2, 1–27. doi: 10.1145/3485666
Neundorf, A., and Öztürk, A. (2023). How to improve representativeness and cost-effectiveness in samples recruited through meta: a comparison of advertisement tools. PLoS ONE 18:e0281243. doi: 10.1371/journal.pone.0281243
Nigon, C., Mancera, J., and Terán, L. (2023). “Enhancing dynamic profiles on voting advice applications using social media analysis,” in 2023 Ninth International Conference on eDemocracy & eGovernment (ICEDEG) (Quito: IEEE), 1-7.
Orellana, S., and Bisgin, H. (2023). Using natural language processing to analyze political party manifestos from New Zealand. Information 14, 152. doi: 10.3390/info14030152
Pajala, T., Korhonen, P., Malo, P., Sinha, A., Wallenius, J., and Dehnokhalaji, A. (2018). Accounting for political opinions, power, and influence: a voting advice application. Eur. J. Oper. Res. 266:702–715. doi: 10.1016/j.ejor.2017.09.031
Pianzola, J. (2014a). Selection biases in voting advice application research. Elect. Stud. 36, 272–280. doi: 10.1016/j.electstud.2014.04.012
Pianzola, J. (2014b). Swing voting due to Smartvote use? Evidence from the 2011 Swiss federal elections. Swiss Polit. Sci. Rev. 20, 651–677. doi: 10.1111/spsr.12120
Pianzola, J., Trechsel, A. H., Vassil, K., Schwerdt, G., and Alvarez, R. M. (2019). The impact of personalized information on vote intention: evidence from a randomized field experiment. J. Polit. 81, 833–847. doi: 10.1086/702946
Ramonaitė, A. (2010). Voting advice applications in Lithuania: Promoting programmatic competition or breeding populism? Policy Intern. 2, 117–147. doi: 10.2202/1944-2866.1027
Romero Moreno, G., Padilla, J., and Chueca, E. (2022). Learning VAA: a new method for matching users to parties in voting advice applications. J. Elect. Public Opin. Parties 32:339–357. doi: 10.1080/17457289.2020.1760282
Ruedin, D., and Morales, L. (2019). Estimating party positions on immigration: assessing the reliability and validity of different methods. Party Polit. 25, 303–314. doi: 10.1177/1354068817713122
Ruusuvirta, O., and Rosema, M. (2009). “Do online vote selectors influence electoral participation and the direction of the vote?,” in Paper presented at the ECPR General Conference (Potsdam: University of Potsdam), 10–12.
Sánchez-Nielsen, E., Lee, D., Panopoulou, E., Delakorda, S., and Takács, G. (2014). “Engaging citizens in policy issues: Multidimensional approach, evidence and lessons learned,” in International Conference on Electronic Participation (Cham: Springer), 102–113.
Scarborough, W. J. (2018). Feminist Twitter and gender attitudes: opportunities and limitations to using Twitter in the study of public opinion. Socius 2018, 4. doi: 10.1177/2378023118780760
Schultze, M. (2014). Effects of voting advice applications (VAAs) on political knowledge about party positions. Policy Inter. 6, 46–68. doi: 10.1002/1944-2866.POI352
Skop, M. (2010). “Are the voting advice applications (VAAs) telling the truth? Measuring VAAs' quality,” in Voting Advice Applications in Europe: The State of the Art, eds. L. Cedroni, and D. Garzia, 199–230.
Slapin, J. B., and Proksch, S.-O. (2008). A scaling model for estimating time-series party positions from texts. Am. J. Pol. Sci. 52, 705–722. doi: 10.1111/j.1540-5907.2008.00338.x
Stadelmann-Steffen, I., Rajski, H., and Ruprecht, S. (2023). The role of vote advice application in direct-democratic opinion formation: An experiment from Switzerland. Acta Politica 58, 792–818. doi: 10.1057/s41269-022-00264-5
Sullivan-Paul, M. (2023). How Would ChatGPT Vote in a Federal Election? A Study Exploring Algorithmic Political Bias in Artificial Intelligence (Master's thesis). Tokyo: University of Tokyo.
Terán, L., Ladner, A., Fivaz, J., and Gerber, S. (2012). “Using a fuzzy-based cluster algorithm for recommending candidates in e-elections,” in Fuzzy Methods for Customer Relationship Management and Marketing: Applications and Classifications, eds. A. Meier, and L. Donzé (Hershey: IGI Global), 115–138.
Terán, L., and Mancera, J. (2019). Dynamic profiles using sentiment analysis and Twitter data for voting advice applications. Gov. Inf. Q. 36, 520–535. doi: 10.1016/j.giq.2019.03.003
Trechsel, A. H., and Mair, P. (2011). When parties (also) position themselves: an introduction to the EU Profiler. J. Inform. Technol. Polit. 8, 1–20. doi: 10.1080/19331681.2011.533533
Triga, V. (2014). “Social representations of VAAs: A comparative analysis,” in Matching Voters to Parties and Candidates, D. Garzia, and S. Marschall (Colchester: ECPR Press), 129–144.
Tsapatsoulis, N., Agathokleous, M., Djouvas, C., and Mendez, F. (2015). On the design of social voting recommendation applications. Int. J. Artif. Intellig. Tools 24, 1550009. doi: 10.1142/s0218213015500098
Tsutsumi, H., Uekami, T., Inamasu, K., Levy, H. I., Song, J., and Shinada, Y. (2018). “The impact of voting advice applications on voters' behavior and political interest,” in CeDEM Asia 2018: Proceedings of the International Conference for E-Democracy and Open Government (Lower Austria: Edition Donau-Universität Krems), 123.
Väänänen, K., Hiltunen, A., Varsaluoma, J., and Pietilä, I. (2020). “CivicBots–Chatbots for supporting youth in societal participation,” in Chatbot Research and Design: Third International Workshop (Cham: Springer), 143–157.
van Camp, K., Lefevere, J., and Walgrave, S. (2014). “The content and formulation of statements in voting advice applications: A comparative analysis of 26 VAAs,” in Matching Voters to Parties and Candidates, D. Garzia, and S. Marschall (Colchester: ECPR Press).
van Zanten, N., and Boumans, R. (2023). Voting assistant chatbot for increasing voter turnout at local elections: An exploratory study, in Social Sciences & Humanities Open. doi: 10.2139/ssrn.4340412
Vassil, K. (2011). Voting Smarter? The Impact of Voting Advice Applications on Political Behavior (PhD thesis). Fiesole: European University Institute.
Volkens, A., Bara, J., Budge, I., McDonald, M. D., and Klingemann, H.-D. (2013). Mapping Policy Preferences from Texts: Statistical Solutions for Manifesto Analysts. Oxford University Press.
Waldvogel, T., Oberle, M., and Leunig, J. (2023). What do pupils learn from voting advice applications in civic education classes? Effects of a digital intervention using voting advice applications on students' political dispositions. Social Sci. 12, 621. doi: 10.3390/socsci12110621
Walgrave, S., Nuytemans, M., and Pepermans, K. (2009). Voting advice applications and the effect of statement selection. West Eur. Polit. 32, 1161–1180. doi: 10.1080/01402380903230637
Wall, M., Krouwel, A., and Vitiello, T. (2014). Do voters follow the recommendations of voter advice application websites? A study of the effects of kieskompas.nl on its users' vote choices in the 2010 Dutch legislative elections. Party Polit. 20, 416-428. doi: 10.1177/1354068811436054
Wall, M., Sudulich, M. L., Costello, R., and Leon, E. (2009). Picking your party online: an investigation of Ireland's first online voting advice application. Inform. Polity 14, 203–218. doi: 10.3233/IP-2009-0179
Watanabe, K. (2021). Latent semantic scaling: A semisupervised text analysis technique for new domains and languages. Commun. Methods Meas. 15, 81–102. doi: 10.1080/19312458.2020.1832976
Wiedemann, G. (2019). Proportional classification revisited: automatic content analysis of political manifestos using active learning. Soc. Sci. Comput. Rev. 37, 135–159. doi: 10.1177/0894439318758389
Keywords: voting advice applications, artificial intelligence, text mining, recommender systems, chatbots
Citation: Gemenis K (2024) Artificial intelligence and voting advice applications. Front. Polit. Sci. 6:1286893. doi: 10.3389/fpos.2024.1286893
Received: 31 August 2023; Accepted: 16 January 2024;
Published: 30 January 2024.
Edited by:
JoseM Pavia, University of Valencia, SpainReviewed by:
Laura Uyttendaele, Université Catholique de Louvain, BelgiumCopyright © 2024 Gemenis. This is an open-access article distributed under the terms of the Creative Commons Attribution License (CC BY). The use, distribution or reproduction in other forums is permitted, provided the original author(s) and the copyright owner(s) are credited and that the original publication in this journal is cited, in accordance with accepted academic practice. No use, distribution or reproduction is permitted which does not comply with these terms.
*Correspondence: Kostas Gemenis, ay5nZW1lbmlzQGN1dC5hYy5jeQ==
†ORCID: Kostas Gemenis orcid.org/0000-0002-3973-5675
Disclaimer: All claims expressed in this article are solely those of the authors and do not necessarily represent those of their affiliated organizations, or those of the publisher, the editors and the reviewers. Any product that may be evaluated in this article or claim that may be made by its manufacturer is not guaranteed or endorsed by the publisher.
Research integrity at Frontiers
Learn more about the work of our research integrity team to safeguard the quality of each article we publish.