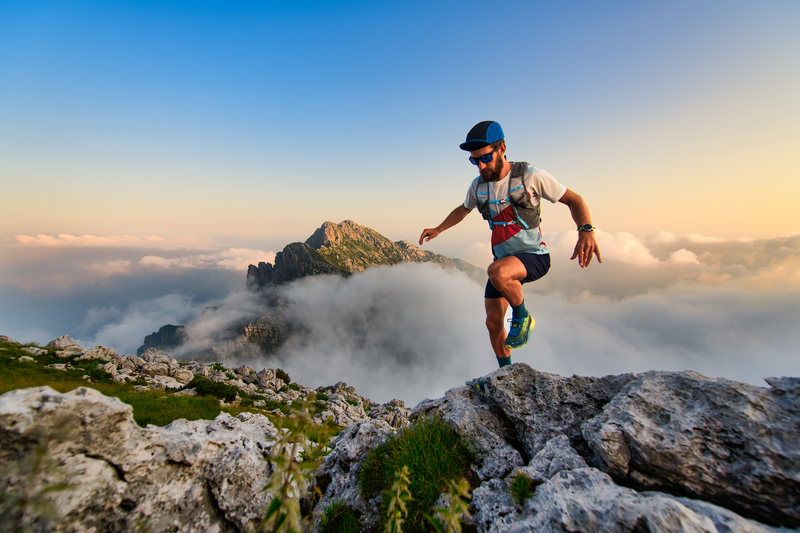
94% of researchers rate our articles as excellent or good
Learn more about the work of our research integrity team to safeguard the quality of each article we publish.
Find out more
ORIGINAL RESEARCH article
Front. Polit. Sci. , 18 May 2023
Sec. Dynamics of Migration and (Im)Mobility
Volume 5 - 2023 | https://doi.org/10.3389/fpos.2023.1091997
This article is part of the Research Topic Migration and Integration: Tackling Policy Challenges, Opportunities and Solutions View all 13 articles
Non-cognitive skills are increasingly essential in the labor market, especially given technological advances and evolving work environments. Unequal distribution of non-cognitive skills among various groups in the population may contribute to labor market inequalities. This article investigates the significance of non-cognitive skills for immigrant-native inequalities in the European labor market. Specifically, we examine the potential differences in non-cognitive skills between native and immigrant groups and how these differences may affect their income. Additionally, we explore whether equal levels of non-cognitive skills have comparable payoffs for native and immigrant groups in society. We use, comparative survey data from the Programme for the International Assessment of Adult Competencies and OLS regressions with country fixed effects. Our findings show that many immigrants exhibit lower levels of non-cognitive skills than native-born workers, despite differences between origin groups. This difference in non-cognitive skills explains part of the immigrant-native inequality in the labor market for most immigrant-origin groups. Moreover, our results indicate that immigrants, especially those from Central and Eastern European countries, benefit less from exercising comparable non-cognitive skills than native-born workers. Our study highlights the importance of non-cognitive skills in addressing the labor market disadvantage faced by immigrants, and emphasizes that policymakers and educators should recognize the significance of these skills when developing policies targeting immigrants.
This study examines the importance of non-cognitive skills for explaining immigrant-native inequalities in the European labor markets. The Nobel laureate Heckman argued that non-cognitive skills, such as perseverance, sociability, and openness to experience, are important determinants of a wide range of life outcomes, including educational achievement, labor market outcomes, health, and criminality (Heckman and Kautz, 2012). Various authors claim that due to technological developments, non-cognitive skills have become increasingly important in the labor market (Heckman and Jora Stixrud, 2006; Deming, 2017; OECD, 2017; Fernandez and Liu, 2019). Deming (2017) illustrated this empirically by showing that among graduates with high levels of non-cognitive skills, the probability of full-time work increased more than fourfold between 1979 and 1997 in the United States. During the same period, the wage returns for non-cognitive skills almost doubled. Given the increasing importance of non-cognitive skills for labor market outcomes, a critical question is whether and to what extent these skills influence immigrant-native inequalities in the labor market.
This article extends and contributes to previous research by examining whether and to what extent self-reported non-cognitive skills can explain immigrant-native inequalities in the labor market. We answer three questions related to immigrants' skills and their role in the labor market. First, do immigrants have and exercise a lower level of non-cognitive skills than native-born workers? Second, are the economic returns on non-cognitive skills the same for immigrants as for native-born workers? Third, can differences in levels and returns on non-cognitive skills explain immigrant-native inequalities in income? We use the European Commission's definition of non-cognitive skills as “non-job specific skills that are related to individual ability to operate effectively in the workplace (…) and that are cross-cutting across jobs and sectors and relate to personal competences (confidence, discipline, and self-management) and social competences (teamwork, communication, and emotional intelligence)” (European Commission, 2011, p. 10).
We rely on human capital, discrimination, and immigrants' selectivity theories to develop hypotheses about the role of non-cognitive skills in immigrant-native inequalities in the labor market. While human capital and immigrants' selectivity theory point to immigrant-native differences in levels and portability of educational credentials and (unobservable) skills, discrimination theory emphasizes the “unequal treatment of otherwise equal workers” (Becker, 1957) as a possible explanation for immigrant-native inequality in the labor market. To test our hypotheses, we use data from the international PIAAC survey (the Programme for the International Assessment of Adult Competencies; OECD, 2012, 2013, 2016, 2019; Rammstedt et al., 2016). The survey covers over 39 countries, with data collected between 2011 and 2012 for most of the countries.1 The key advantage of PIAAC data is that besides standard measures of educational and labor market outcomes, they provide information on different types of skills—literacy, numeracy, and problem-solving, as well as non-cognitive skills. In addition, the data include detailed immigrant-specific information on the place of education and age at migration—information not provided in most previous studies.
The focus on immigrants' non-cognitive skills is essential because, in addition to knowing the extent to which immigrant-native inequalities in the labor market can be explained by differences in formal education and training, it is also extremely important to know the roles of non-cognitive skills' levels and returns thereupon in generating income inequalities in Western labor markets (e.g., Deming, 2017; Fernandez and Liu, 2019). Such knowledge about levels and economic returns on non-cognitive skills among immigrants and natives is critical to designing suitable labor market policies targeting immigrants.
Considerable attention has been devoted to the study of immigrant economic outcomes. The main finding from previous research is that immigrants in European countries are at an economic disadvantage. After arrival in the host country, immigrants experience difficulties finding jobs, and when they are employed, they often have lower status and lower paid jobs than native-born workers with the same education and work experience (OECD, 2018, 2020).
Three theoretical approaches may explain the disadvantage of immigrants in the labor market. The first approach is the human capital theory, which suggests that human capital acquired in the country of origin does not pay off as much as that developed in the destination country (Chiswick, 1978; Chiswick and Miller, 2009; Kanas and Van Tubergen, 2009). There has been ample support in the literature that economic returns on foreign credentials and work experiences are lower than on credentials and experiences acquired in the host country (Friedberg, 2000; Bratsberg and Terrell, 2002; Zeng and Xie, 2004; Kanas and Van Tubergen, 2009). Because many immigrants to Europe come from economically less developed countries, their educational credentials and work experience are often of lower quality and more difficult to transfer than credentials and skills acquired in European countries (Bratsberg and Terrell, 2002; Zeng and Xie, 2004). Moreover, native employers may be reluctant to fully recognize foreign credentials and work experiences because they are often uncertain about their labor market value (Friedberg, 2000; Bratsberg and Ragan, 2002; Bratsberg and Terrell, 2002; Zeng and Xie, 2004; Kanas and Van Tubergen, 2009). In line with these arguments, Bratsberg and Terrell (2002) found that in the U.S. labor market, immigrants from countries with lower pupil-teacher ratios and expenditures per pupil received lower returns on their schooling. Likewise, Bratsberg and Ragan (2002) found that the returns on education acquired abroad are lower for immigrants from less developed countries and countries where English is not an official language. A similar pattern of findings has also been shown outside the U.S. context. Kanas and Van Tubergen (2009) have found that in the Netherlands, Caribbean immigrants from former Dutch colonies received higher schooling returns than immigrants from the Mediterranean region. Interestingly, these differential returns on education are less pronounced for employment, suggesting that the quality and transferability of educational credentials are less important for accessing the labor market than for the quality of employment. Friedberg (2000) has shown that education and work experiences acquired abroad were significantly less valued in the Israeli labor market than education and skills developed domestically. The returns on schooling obtained abroad were lowest among immigrants from Asia and Africa, while immigrants from Western countries received the highest returns relative to other immigrant groups. According to Friedberg (2000), these findings support the hypothesis that school quality in Asia and Africa is lower and less suited to the Israeli labor market. Still, they could also reflect higher levels of discrimination against Asians and Africans than Western immigrants.
In previous research, immigrants' human capital has been mainly measured by educational credentials, work experiences, and language skills. Only recently have authors extended this measurement to include cognitive skills (Kerckhoff et al., 2001; Ferrer et al., 2006; Fernandez and Smith, 2015; Hanushek et al., 2015; Lancee and Bol, 2017). The term cognitive skills, as used in the literature, refers to factors like IQ and other achievement tests in the main domains of the school curriculum (Heckman and Kautz, 2012). For example, Ferrer et al. (2006) examined the importance of literacy skills for immigrants' earnings in Canada. They found that literacy skill differences account for about two-thirds of the earnings disadvantage of immigrants compared to natives. However, they did not find evidence that returns on literacy skills were lower for immigrants than for native-born Canadians. Using PIAAC data from 11 countries, Lancee and Bol (2017) examined the role of foreign credentials and numeracy skills in explaining immigrant group differences in earnings. They found that while differences in numeracy skills explained one-third of the negative effect of non-western credentials on earnings, the limited transferability of non-Western diplomas also played a role.
Second, immigrant-native inequality in the labor market can also be affected by immigrants' self-selection into immigration and destination sorting (Chiswick, 1978; Feliciano, 2005; Schmidt and Cornelia Kristen, 2022). Those who decide to leave their country of origin may have specific characteristics that lead them to a migration decision. For instance, immigrants who decide to leave their country of origin may be more entrepreneurial and ambitious than the average population in their country of origin. If they are also relatively more entrepreneurial and ambitious in comparison with the population in the destination country, this may account for income differences that cannot be explained by formal training and education but may be related to non-cognitive skills. Or, conversely, if immigrants are less favorably selected on their non-cognitive skills, this may explain their economically vulnerable position in destination countries (Chiswick, 1978; Feliciano, 2005). However, detecting such patterns of self-selection is often challenging or even impossible without knowing the non-cognitive skills of natives and immigrants. Available PIAAC data can provide information on large samples of natives and immigrants about income determinants (i.e., cognitive and non-cognitive skills) that were considered until recently as “unobserved.” These data allow us to empirically increase the “explained” and mitigate the “unexplained” portions of native-immigrant income gaps. Even though this article does not aim to test the “selection” hypothesis, selectivity theory might explain different distributions of non-cognitive skills between immigrants and natives within countries. Following human capital theory, these differences in their turn may explain native-immigrant income inequalities.
A third possible explanation for native-immigrant inequalities is offered by discrimination theory. This theory posits two main types of discrimination in the labor market: taste-based discrimination and statistical discrimination. According to taste-based discrimination, immigrant-native disparities in the labor market are due to the personal prejudices of customers, co-workers, or employers against associating with immigrants (Becker, 1957). In European countries, immigrants with non-Western backgrounds, particularly those with visible physical traits (e.g., skin color) or marked cultural differences (e.g., wearing a scarf), are exposed to taste-based discrimination (Midtbøen, 2013; Di Stasio et al., 2021). Statistical discrimination, on the other hand, emphasizes the role of the employer's uncertainty about foreign human capital (Pager et al., 2009). Because employers are less familiar with foreign educational systems, they often assume that immigrants lack the necessary human capital (Midtbøen, 2013) or that their human capital is incompatible with the host country's labor market (Friedberg, 2000; Damelang and Abraham, 2016). Using a factorial survey, Damelang and Abraham (2016) showed that applicants with a foreign vocational degree were less likely to be invited for a job interview as an office worker than those holding a German vocational degree. The country of origin mattered as well. Holding the place of education constant, immigrants from Bulgaria, Turkey, and Portugal had a significantly lower probability of being invited for a job interview than Germans, suggesting that besides concerns with the portability of foreign credentials, employers' preferences to work with natives are important as well. Empirically, taste-based and statistical discrimination have the same consequence: employers will pay immigrant workers less than equally productive native-born workers (Pager et al., 2009).2
A few recent studies have examined the importance of self-reported non-cognitive skills for immigrants' labor market outcomes (Lancee and Bol, 2017; Kosyakova and Laible, 2022; Laible and Brenzel, 2022). In their study using PIAAC international data and OLS regression, Lancee and Bol (2017), found that immigrants' self-reported readiness to learn was positively related to their hourly earnings, net of immigrants' cognitive skills. However, they did not examine other dimensions of non-cognitive skills or the relationship between skill differences and immigrant-native wage inequality. Laible and Brenzel (2022) studied the role of the self-reported “big five” personality traits (i.e., extroversion, neuroticism, agreeableness, conscientiousness, and openness to experience). Based on the OLS regression, they found that these traits explained ~6% of immigrant-native wage inequality in Germany. Similarly, Kosyakova and Laible (2022), using the IAB-BAMF-SOEP Survey of Refugees in Germany and growth curve models, showed that certain self-reported personality traits (i.e., openness to new experiences, conscientiousness, risk appetite, locus of control, and resilience) were positively related to destination-language acquisition among refugee immigrants.
Drawing on existing theoretical frameworks and previous research, we have formulated two conflicting expectations about non-cognitive skills. Based on human capital and immigrants' selectivity theories, we expect immigrants and natives to differ in their levels of non-cognitive skills leading to immigrant-native inequality in income, net of educational credentials, work experience, and cognitive skills. In addition, we contend that non-cognitive skills are less susceptible to issues of limited observability ex-post (i.e., once an individual has been employed for a certain period) and at the intensive margin (i.e., in terms of income) when compared to foreign credentials. This may help alleviate concerns about the quality and portability of non-cognitive skills. Hence, we expect that economic returns on non-cognitive skills will be similar for immigrants and natives and that discrepancies in non-cognitive skills levels will explain immigrant-native income inequality. In contrast, according to discrimination theory, we expect that economic returns on non-cognitive skills will be lower for immigrants than natives, net of educational credentials, work experience, and cognitive skills. Furthermore, we expect that differences in returns on non-cognitive skills will explain income inequality between immigrants and natives.
This study uses international data from the Programme for the International Assessment of Adult Competencies (Rammstedt et al., 2016). The purpose of the PIAAC survey is to measure adults' proficiency in key information-processing skills (literacy, numeracy, and problem-solving) and to gather information about how adults use their skills at home, at work, and in the wider community. The data have been collected in 39 countries: 24 countries participated in Round 1, with data collection taking place between August 2011 and March 2012; nine countries took part in Round 2 of the assessment, with data collection taking place between April 2014 and March 2015; and six countries participated in Round 3, with data collection taking place between July and December 2017.
The target population for PIAAC consists of all non-institutionalized adults between ages 16 and 65 (inclusive) who resided in the country at the time of data collection, regardless of citizenship, nationality, or language. Throughout the PIAAC data collection process, efforts were made to address non-response resulting from language barriers, reading, or writing difficulties, and learning or mental disabilities. To ensure the quality of the data, countries were encouraged to use translations and interpreters for the main questionnaire and to capture and monitor reasons for non-response (Van De Kerckhove and Leyla Mohadjer, 2013). Each participating country was required to produce a probability-based sample representative of the target population of the country. Of the 17 countries analyzed, Estonia had the highest percentage of the target population not covered (2.8%), while Denmark had the lowest (>0.1%). The interviews were carried out at home, in the official national language of the country. The response rate ranged from 45% (Sweden) to 71% (Ireland) in the examined countries (OECD, 2019).
The sample for this study consists of immigrants and native men and women between the ages of 16 and 65 in 17 European countries (Austria, Belgium, Czech Republic, Denmark, Estonia, France, Germany, Greece, Hungary, Ireland, Italy, the Netherlands, Norway, Spain, Slovenia, Sweden, and the UK). Our selection of countries was motivated by the sample size of immigrant populations. Countries with < 100 immigrants were excluded from the final sample. Immigrants are defined as those born abroad and who are at least 17 years old at the age of migration. In addition, based on geographic birth region, we further distinguish between immigrants from North America and Western Europe (including richer Pacific and Asian countries), Central and Eastern Europe, and Other Regions. Based on these pre-selections, we excluded individuals missing information on the main dependent and independent variables: monthly income variable (7,556 observations deleted), geographic region (137 observations deleted), numeracy skills (five observations deleted), readiness to learn scale (24 observations deleted), planning scale (3 observations deleted), and task discretion scale (24 observations deleted). Regarding control variables, missing values are accounted for by including dummy variables that indicate whether data for any specific control variable were missing. The sample size ranges from 52,619 (natives) to 1,221 (North America and Western Europe), 2,088 (Central and Eastern Europe), and 1,460 (Other Regions) with a total of 57,388.
Our dependent variable is based on self-reported monthly earned income, including bonuses (wage and salary earners and self-employed), and converted into deciles by the data provider to make the measurement comparable across countries. We relied on the measures of income in deciles because this variable has been filled in in all countries and has the fewest missing observations. For example, alternative continuous measures of monthly income (or hourly earnings) were unavailable in the public-use files for several countries (in our sample for Austria, Germany, Hungary, and Sweden). The results are robust to an alternative specification of wages: hourly earnings (including bonuses) in deciles (see Supplementary Table 2 in the robustness analyses).
The main independent variable is a categorical variable differentiating between natives and immigrants from three geographic regions. Natives refer to those who were born in the destination country. Immigrants were born abroad and came at 17 or older from the following geographic regions: (1) North America and Western Europe, including rich countries in East Asia and the Pacific; (2) Central and Eastern Europe; (3) Other Regions.3
The other independent variables of interest are non-cognitive skills. Our measure of non-cognitive skills is based on self-reported measures of skills including those used at work.4 These measures correspond to the European Commission's definition of non-cognitive skills (European Commission, 2011). Specifically, we use PIAAC's Readiness to Learn scale corresponding to the European Commission's personal effectiveness skill. The respondents were asked to rate the extent to which they related to six statements when dealing with problems and tasks they encountered, using a scale from 1 “not at all” to 5 “to a very high extent”. The examples of statements are: “When I hear or read about new ideas, I try to relate them to real-life situations to which they might apply,” and “I like learning new things,” see Supplementary Table 1 for a complete list of items. Factor analysis revealed a one-factor structure, and the six items were subsequently averaged to form a reliable scale (alpha >0.78 across countries).
We utilize PIAAC's Influencing Skills scale to assess influencing skills, which aligns with the European Commission's impact and influence skills. Respondents were asked to indicate how frequently they use specific skills at work, with response options ranging from 1 “never” to 5 “every day.” The examples of skills assessed are: “Instructing and teaching people,” and “Giving presentations,” see Supplementary Table 1. Factor analysis revealed one factor, and the six items were therefore averaged to create a reliable scale (alpha >0.72 across countries).
Finally, we use PIAAC's Planning scale, and Task Discretion scale, which correspond to the European Commission's achievement skills (i.e., planning, organization, and work autonomy). The Planning scale is measured by three items, with answer categories ranging from 1 “never” to 5 “every day.” The examples of items assessed are: “Planning own activities,” and “Planning others' activities,” see Supplementary Table 1. Factor analysis showed a one-factor structure; the items were therefore averaged to form a reliable scale (alpha > 0.61 across countries). The Task Discretion scale is measured by four items, with answer categories varying between 1 “not at all” and 5 “to a very high extent.” The examples of items are: “Own planning of the task sequences,” and “Own planning of style of work,” see Supplementary Table 1. Factor analysis indicated a one-factor solution, so we took an average of items to form a reliable scale (alpha > 0.72 across countries). While these scales are internationally comparable and validated, they may not reflect the accurate non-cognitive skills of workers. For example, people may possess specific non-cognitive skills but do not exercise them because they are not required on the job.
The following individual-level variables are included as controls. Formal education is measured by the International Standard Classification of Education (ISCED-97). The six categories are (1) “Lower secondary or less (ISCED 1,2, 3C short or less),” (2) “Upper secondary (ISCED 3A-B, C long),” (3) “Post-secondary, non-tertiary (ISCED 4, A-B-C),” (4) “Tertiary—professional degree (ISCED 5B),” (5 “Tertiary—bachelor degree (ISCED 5A),” (6) “Tertiary—master/research degree (ISCED 5A/6).”
Cognitive skills are measured by PIAAC's numeracy and literacy skills. Numeracy skills are defined as “the ability to access, use, interpret and communicate mathematical information and ideas, to engage in and manage the mathematical demands of a range of situations in adult life.” In contrast, literacy skills refer to “understanding, evaluating, using and engaging with written texts to participate in society, to achieve one's goals, and to develop one's knowledge and potential” (OECD, 2013). To measure cognitive skills, respondents were asked to perform a battery of tasks encompassing basic assignments and complex problems. The tasks were framed as real-life problems such as interpreting the election results (literacy) or filling the driver's logbook (numeracy skills). Respondents who passed short, low-difficulty tests were randomly assigned a subset of full tests. Those who “failed” short tests bypassed the full skills assessments and were redirected to a separate “reading components” assessment that tested basic reading skills. Because each respondent participated only in a subset of cognitive tests, PIAAC used multiple imputations (plausible values—PVs) to increase the accuracy of the cognitive measures. Ten PVs are drawn for each respondent per domain (OECD, 2016, 2019). Literacy and numeracy skills are measured on a 500-point scale. The scores are standardized in the subsequent regression analyses to have a within-country mean of zero and a within-country standard deviation of one. Given the importance of language skills for immigrants' labor market outcomes, it is important to note that the cognitive tests were presented to respondents in the official language of the host country. Thus, the scores on cognitive tests and literacy in particular will also reflect immigrants' difficulties in speaking the host-country language. We also control for the total years of work experience and working hours. Finally, we include two immigrant-specific variables: a dummy indicating whether one obtained the highest education abroad and years since migration (YSM).5 Among the sociodemographic variables, we account for being female, living with a partner, and having children in the household. Finally, all models also control for country-fixed effects to absorb any systematic differences related to countries.
Because our research questions are mainly descriptive, we rely on descriptive and associative methods for data analyses. We run ordinary least square regressions (OLS) with country-fixed effects to adjust for the clustering of respondents at the country level. The regression analyses can be represented in four equations:
where the independent variables are Mig, geographic origin groups; Cog, literacy and numeracy skills, NonCog, non-cognitive skills, C, control variables, with i indexing individuals, c indexing countries, λ denoting country-fixed effects, and ε denoting the error term.
See Supplementary Table 1 for a list of all variables and their descriptions used in analyses. To account for weighted replicate samples in PIAAC international data and imputation error in plausible values, we used STATA module repest command in STATA developed by OECD (Keslair, 2020).
Table 1 displays summary statistics of the main variables by geographical region of origin. A possible explanation for immigrant disadvantage in the labor market are differences in levels and portability of origin-country-specific human capital. Table 1 shows that this explanation holds particularly for immigrants from Other Regions. Other Regions immigrants are overrepresented in lower secondary education (28%) compared to immigrants from North America and Western Europe (13%) and immigrants from Central and Eastern Europe (17%) and natives (17%). They also have substantially fewer years of work experience (15 years) than other immigrant groups and natives, who have on average about 19.4 years of work experience. In contrast, immigrants from North America and Western Europe are overrepresented in tertiary education (45%), while the proportion of Central and Eastern European immigrants with a tertiary degree is comparable to natives (24 vs. 25%), with immigrants from Other Regions in-between (33%). This apparent advantage of all groups of immigrants in tertiary education only sometimes translates into jobs with higher skill requirements or incomes. While immigrants from North America and Western Europe have on average the highest monthly income and occupational status, immigrants from Central and Eastern European countries and Other Regions earn less and are underrepresented in skilled occupations than natives (25 and 32% vs. 46%). All immigrants, particularly those from Other Regions, have on average lower literacy and numeracy skills compared to natives. While lower scores of immigrants on numeracy and literacy tests indicate lower cognitive skills, they are also likely to reflect immigrants' difficulties with reading host-country language. Because it is impossible to disentangle these two factors in our analyses, we will interpret differences in cognitive skills as driven by both the cognitive and language skills of immigrants.
Regarding non-cognitive skills, immigrants from North America and Western Europe score significantly higher, while Central and Eastern European and Other Regions immigrants score significantly lower on openness to learning (Central and Eastern Europeans only), influence, planning and task discretion skills than natives (see Figure 1). This finding confirms previous research showing that the degree of immigrants' selectivity in terms of non-cognitive skills varies substantially by country of origin (Feliciano, 2005; Schmidt and Cornelia Kristen, 2022). Because three out of four scales measuring non-cognitive skills refer to skills exercised on a job, these results can point either to differences in levels of non-cognitive skills between immigrants and natives or to immigrants sorting into jobs requiring different usage of non-cognitive skills. To account for immigrants sorting into occupations with lower skill demands, we included aggregated measures of occupational classification in the robustness analyses presented in the next section.
Figure 1. Average non-cognitive skills by geographical region of origin. Source: PIAAC (2012), own calculations.
Table 2 presents the regression results of monthly income. We first present the results without controlling for cognitive and non-cognitive skills (cf. Equation 1, Table 2, Model 1). This enables us to compare our results to standard specifications used in previous research and to closely examine the role of cognitive and non-cognitive skills in explaining immigrant-native inequality in income. In line with previous research, age, educational attainment, work experience, and working hours are associated with a higher monthly income (Chiswick and Miller, 1995; Hanushek et al., 2015). We also find that, all else being equal, time spent in the host country (YSM) reduces the income gap between immigrants and natives, while having education received abroad increases it (Friedberg, 2000; Zeng and Xie, 2004). Regarding socio-demographic variables, females have significantly lower monthly income than males. Having a partner is positively related to a higher monthly income, while there is no significant relationship between having children and monthly income. The latter finding is driven by the opposing association between having children and income for men and women, where having children is related to higher income for men and lower income for women (Adsera and Chiswick, 2007; Kanas and Steinmetz, 2021)—see also Supplementary Tables 3, 4. Even after controlling for basic human capital and sociodemographic variables, all geographic origin groups earn a significantly lower monthly income than natives.
Equation 2, Model 2 adds the cognitive skills variables. There is a small positive effect of numeracy and literacy skills on monthly income. A one standard deviation increase in numeracy skills is associated with a higher monthly income by a 0.01 income decile. The relationship between literacy skills and monthly income turns out to be even smaller (b = 0.002, p = 0.000). It should be mentioned that both skills are highly correlated (r = 0.85, p = 0.000) and in fact answering questions about numeracy skills also requires reading fluency from immigrants. Additional results show that excluding numeracy skills from Model 2, yields a larger and statistically significant association between literacy skills and income (b = 0.01, p = 0.000). Inclusion of literacy and numeracy skills explains 28% of the income gap between North America and Western Europe immigrants and natives. For Central and Eastern European immigrants, these skills explain 34% of the gap, and for Other Regions immigrants, the inclusion of literacy and numeracy skills accounts for a 42% of the income gap with natives.
Turning to the role of non-cognitive skills (cf. Equation 3, Model 3), we find that influence and planning are positively related to monthly income while the coefficients for readiness to learn and task discretion are not significant. More specifically, one standard deviation increase in influence and planning skills are associated with an increase in monthly income decile by 4 and 1%, respectively. Additional analyses, show that the coefficients of readiness to learn (b = 0.09, p < 0.001) and task discretion (b = 0.07, p < 0.001) turn out positive and statistically significant when entered separately in the model. These additional results suggest that being open to new experiences and the ability to work autonomously are related to higher income through their associations with influencing others and the ability to plan one's activities. Controlling for non-cognitive skills further explains the remaining income gap between immigrants and natives. Further analyses indicate that by prioritizing non-cognitive skills over cognitive skills, the income disparity between natives and immigrants from North America and Western Europe decreases by 11%. Moreover, the income gap between natives and immigrants from Central and Eastern Europe is reduced by 25%, while the income gap between natives and immigrants from Other Regions is reduced by 22%.
Equation 4, Models 4–7 include a series of interaction effects between non-cognitive skills and immigrant geographical origin variables. Our results support the expectation that the economic benefits from using non-cognitive skills are lower for immigrants than natives. More specifically, the associations between readiness to learn, planning, and task discretion skills on the one hand and monthly income on the other hand, are significantly lower for Central and Eastern European immigrants than for natives.6,7
We performed several sensitivity analyses to see whether our results are robust to different measures of income, gender differences, and additional controls. First, the monthly income in our baseline model refers to monthly earned income in deciles including bonuses of wage, salary, and self-employed earners. In the robustness analyses, we first checked whether our results remain the same when hourly rather than monthly wages are considered (including bonuses), as these are not affected by the number of hours worked in a week. In comparison to the baseline model, our analysis reveals a positive and statistically significant correlation between hourly earnings and task discretion. We also find that when hourly wages are considered, Central and Eastern European immigrants benefit less from exercising influence skills than natives. Other than that, our results remain robust to these alternative income specifications (see Supplementary Table 2).
Second, we also examined whether our findings are similar for men and women. Our analysis, as shown in Supplementary Tables 3, 4, reveals that replicating our baseline models separately for men and women does not significantly alter our conclusions regarding the role of non-cognitive skills. We find that influence and planning skills are positively associated with monthly income, while the coefficient of readiness to learn does not reach statistical significance. However, in the male sample, the coefficient of task discretion turns negative and significant, which is unexpected and implies that men with greater work autonomy receive lower monthly income, all else being equal. Similar to the baseline model, Central and Eastern Europeans benefit less from readiness to learn, planning (among females only), and task discretion skills compared to natives. Moreover, among females, non-cognitive skills appear to be less advantageous for immigrants from the Other Regions category, as the returns on readiness to learn and task discretion skills are significantly smaller for female immigrants from the Other Regions than for natives.
Third, to control for a potential confounding mechanism, we also replicate our results with nine one-digit Occupation (ISCO) categories and 21 one-digit industry (ISIC) categories included in the analyses (Supplementary Table 5). When we control for occupations and industries, our findings regarding the levels of non-cognitive skills remain consistent with the baseline model. Compared to the baseline model (cf. Table 2, Model 3), the coefficients of influence and planning skills remain relatively unchanged. At the same time, similar to the male sample, we find that task discretion skills are negatively related to monthly income. Notably, even after accounting for broad occupational and industry categories, most interactions between non-cognitive skills and Central and Eastern European immigrants remain significant.
Although human capital and immigrants' selection have been identified as a key factor determining immigrant-native inequalities in the labor market, previous research has focused on rather crude estimates of their importance. Most studies in the field of migration have measured human capital by language skills, education, and work experiences, assuming that these factors will capture other (unobserved) skills that influence the productivity of immigrant workers (Chiswick and Miller, 1995, 2002; Dustmann and Fabbri, 2003). More recently the authors have extended the measures of immigrants' human capital by focusing on direct measures of immigrants' cognitive skills (Kerckhoff et al., 2001; Farkas, 2003; Ferrer et al., 2006; Hanushek et al., 2015; Lancee and Bol, 2017) although, with a few exceptions, their findings have been limited to the U.S. context. This paper contributes to this line of research by examining the relationships between non-cognitive skills and immigrant-native inequalities in monthly income across 17 European countries. A growing body of research points to the increasing importance of non-cognitive skills in the contemporary labor markets but the evidence about their importance for foreign-born workers is missing. This paper addresses this gap by focusing on three questions about non-cognitive skills and immigrant-native inequalities in the labor market. First, do immigrants have a lower level of non-cognitive skills than native-born workers? Second, are the economic returns on skills the same for immigrants as for native-born workers? Third, can differences in levels and returns on skills explain immigrant-native inequalities in income?
Regarding the first question, we find that immigrants significantly differ in the level and usage of non-cognitive skills as compared to natives. Although immigrants from North America and Western Europe display significantly higher levels of non-cognitive skills than natives, those coming from Central and Eastern European origins exhibit significantly lower readiness to learn skills. Additionally, immigrants from Central and Eastern Europe as well as Other Regions tend to use influence, planning, and task discretion skills less frequently than natives. While our measure of readiness to learn indicates individual attitudes and ability to acquire new information and skills, the latter measures reflect skills exercised at work, namely, the impact on one's co-workers and supervisors, the ability to plan one's activities, and autonomy in one's work (European Commission, 2011; Fernandez and Liu, 2019). The relatively high score on readiness to learn skills among immigrants from North America and Western Europe and Other Regions is in line with previous research suggesting a more favorable selection among immigrants in European destinations particularly when they come from far away geographic regions (Feliciano, 2005). The fact that Central and Eastern European and Other Regions immigrants score significantly lower than natives on non-cognitive skills used at work could either reflect the lower level of such skills among immigrants compared to natives or immigrants sorting into jobs with lower opportunities for exercising such skills.
Our results show that even after we account for educational credentials, work experience, and cognitive skills, non-cognitive skills are positively related to higher monthly income, thus partially explaining immigrant-native income inequality. Further analyses have revealed that non-cognitive skills alone account for a range of 11% (North America and Western Europe immigrants) to 25% (Other Regions immigrants) of income disadvantage experienced by immigrants. The associations between non-cognitive skills and income are not only statistically significant but also economically meaningful. One standard deviation increase in influence and planning skills is associated with an increase in monthly income deciles by 4 and 1%, respectively. These associations are much larger than those between cognitive skills and income and comparable with 6 years of work experience or a one-unit increase in educational attainment. Interestingly, readiness to learn and task discretion are insignificant when entered together with other dimensions of non-cognitive skills. These findings suggest that openness toward learning and exercising work autonomy operate through other non-cognitive skills. For example, workers who are more open to learning are more likely to land jobs where they exercise other non-cognitive skills at work which in turn influences their income. Based on the U.S. PIAAC data and focusing on the same dimensions of non-cognitive skills (Fernandez and Liu, 2019) have found that only influence and planning were associated with U.S. workers' earnings and occupational status. These non-cognitive skills also interacted with a university degree in the way that they substituted for the lack of a degree among less-educated workers. In contrast, using the same data among immigrants in Western countries and focusing only on readiness to learn as a measure of non-cognitive skills, Lancee and Bol (2017) have shown that it is positively associated with the hourly earnings of immigrants.
Regarding the second question, our findings provide some support for discrimination theory as they show that immigrants from Central and Eastern European countries benefit less from using non-cognitive skills compared to native workers. Interestingly, with the exception of the female sample, we find little support that the returns on non-cognitive skills are significantly lower for the most discriminated groups at European labor market, namely Other Regions immigrants. The heterogeneity within the Other Regions immigrant group may be a possible explanation for this finding. Specifically, roughly one-third of immigrants in the Other Regions category have tertiary education and skilled occupations, while another third have less than lower secondary education, and one-fifth hold elementary occupations (as depicted in Table 1).
The answer to our third question is that it is mainly differences in levels of non-cognitive skills that explain immigrant-native inequality in income. In line with human capital and immigrants' selectivity theories, our results clearly show that immigrant-native inequality is substantially reduced by differences in cognitive and non-cognitive skills. Thus, immigrants' disadvantage in the labor market can be largely explained by differences in productivity due to lower levels of cognitive and non-cognitive skills. This is an important finding because previous research in the migration literature has paid little attention to cognitive and non-cognitive skills, mainly due to data limitations. However, we also find some evidence that observationally similar immigrants from Central and Eastern Europe benefit less from their usage of planning skills than do natives, contributing to immigrant-native income inequality. The lower returns on non-cognitive skills for these immigrants compared to natives align with the discrimination theory that predicts that equally productive workers are paid unequally (Becker, 1957). To the extent that our measures of cognitive skills control for immigrants' language proficiency, these findings are unlikely to be affected by immigrants' language difficulties with the host-country language. While a high score in cognitive skills reflects proficiency in reading skills, it could still be that those who scored high on cognitive tests experience difficulties in speaking and writing in the host-country language. Moreover, while our robustness analyses control for broad occupational and industry categories, we cannot exclude the possibility that our findings of differential returns on skills are (partly) influenced by immigrants sorting into jobs with lower demands and rewards for non-cognitive skills. More detailed measurement of industries and occupations (e.g., four-digit ISIC and ISCO classifications instead of one-digit classifications) could address this limitation.
This study shows that non-cognitive skills play a role in the emergence and persistence of native-immigrant labor market inequalities. This highlights the need for more attention to non-cognitive skills in research, policy design, and in the implementation of training programs. Three strategies specifically may be beneficial for this. The first one aims at raising the overall level of non-cognitive skills. Whereas, active labor market policies tend to focus on job-specific or general cognitive skills, our article clearly shows the need for—and potential benefit of—developing programs for non-cognitive skills. We think a second strategy should further analyze the gap in the use of non-cognitive skills in practice. From this paper, we might infer that some immigrants end up in jobs where they do not fully use their skills. But to be more conclusive here, we would need more insight into immigrant-native differences in the gap between existing skills and applied skills. Finally, our article shows that it is important to raise awareness about the unequal returns on non-cognitive skills for migrants and natives. There are many examples of programs promoting gender-equal rewards for cognitive skills and experience; these may provide a source of inspiration. Greater attention to non-cognitive skills may not only help reduce immigrant-native inequalities in the labor market, but better equip employees—immigrants as well as natives—for the demands of the twenty-first-century labor market.
The analyses of the present study are based on the German PIAAC dataset provided by the Research Data Center PIAAC at GESIS: Rammstedt, B., Martin, S., Zabal, A., Konradt, I., Maehler, D., Perry, A., Massing, N., Ackermann-Piek, D., & Helmschrott, S. (2016). Programme for the International Assessment of Adult Competencies (PIAAC), Germany – Reduced version. Data file version 2.2.0 [ZA5845]. Cologne: GESIS Data Archive. doi: 10.4232/1.12660.
AK and MF conceived the presented idea. AK developed the theoretical framework and conducted analyses. Both authors discussed the results and contributed to the final manuscript.
The authors declare that the research was conducted in the absence of any commercial or financial relationships that could be construed as a potential conflict of interest.
All claims expressed in this article are solely those of the authors and do not necessarily represent those of their affiliated organizations, or those of the publisher, the editors and the reviewers. Any product that may be evaluated in this article, or claim that may be made by its manufacturer, is not guaranteed or endorsed by the publisher.
The Supplementary Material for this article can be found online at: https://www.frontiersin.org/articles/10.3389/fpos.2023.1091997/full#supplementary-material
1. ^The countries where data collection took place between 2011 and 2012 are: Australia, Austria, Belgium (Flanders), Canada, Czech Republic, Denmark, Estonia, Finland, France, Germany, Ireland, Italy, Japan, Korea, Netherlands, Norway, Poland, Russian Federation, Slovak Republic, Spain, Sweden, United Kingdom (England and Northern Ireland), and United States. In nine countries (Chile, Greece, Indonesia, Israel, Lithuania, New Zealand, Singapore, Slovenia, and Turkey), data collection took place between 2014 and 2015. In six countries (Ecuador, Hungary, Kazakhstan, Mexico, Peru, and United States), data collection took place in 2017.
2. ^Since we focus on the income of employees with some job tenure and not on hiring decisions, we can argue that any evidence of differential treatment will be driven by employers' preference for discrimination rather than information asymmetry, which is often the case in hiring situations.
3. ^The countries in the Western Europe category include: EU member states and other Western European countries (the UK, Norway, Iceland, and Switzerland). Rich countries in East Asia and the Pacific include: Australia, Japan, New Zealand, Korea, and New Caledonia. The countries in Eastern and Central Europe include: Albania, Belarus, Bosnia and Herzegovina, Bulgaria, Croatia, Ukraine, Russian Federation, Serbia, the former Yugoslavian Republic of Macedonia, Turkey, Republic of Moldova, and Montenegro. The public-use PIAAC data files only provide information on whether the respondent was born in the destination country, age at migration, and the aggregated categories of the birth region.
4. ^It is common in previous research on noncognitive skills to rely on self-reported measures (see Heckman and Kautz, 2012; Deming, 2017 for an overview). An important critique of self-reported measures is that they are generally less reliable because of their subjectivity and social desirability bias. Furthermore, without a common metric, individual responses may not be interpersonally comparable (King et al., 2003; Van Soest et al., 2011). While objective measures of non-cognitive skills would be preferable, (internationally comparative) data on non-cognitive skills are scarce (Anghel and Balart, 2017).
5. ^Following Friedberg (2000), we set YSM = 0 for natives. In this specification, the coefficients on migrant geographical origin dummies represent the initial income disadvantage between newly arrived immigrant origin groups and natives, and the coefficient on YSM represents how this disadvantage changes over time since migration.
6. ^Additional analyses, not presented here, further show that the returns on noncognitive skills are not significantly different across geographical origin groups.
7. ^To get a better view of the total effect of non-cognitive skills on migrants' income, we rerun our results focusing on an immigrant sample only. The regression coefficients for noncognitive skills are positive in three cases (in one case, influence, it is statistically significant at a 5% level) and insignificant negative in one case (task discretion skills).
Adsera, A., and Chiswick, B. R. (2007). Are there gender and country of origin differences in immigrant labor market outcomes across European destinations? J. Popul. Econ. 20, 495–526. doi: 10.1007/s00148-006-0082-y
Anghel, B., and Balart, P. (2017). Non-cognitive skills and individual earnings: New evidence from PIAAC. SERIEs 8, 417–473. doi: 10.1007/s13209-017-0165-x
Becker, G. S. (1957). The Economics of Discrimination, 2d Edn. Chicago, IL: The University of Chicago Press.
Bratsberg, B., and Ragan, J. F. (2002). The impact of host-country schooling on earnings: A study of male immigrants in the United States. J. Hum. Resour. 37, 63–105. doi: 10.2307/3069604
Bratsberg, B., and Terrell, D. (2002). School quality and returns to education of U.S. Immigrants. Econ. Inq. 40, 177–198. doi: 10.1093/ei/40.2.177
Chiswick, B. R. (1978). The effect of Americanization on the earnings of foreign-born men. J. Polit. Econ. 86, 897–921. doi: 10.1086/260717
Chiswick, B. R., and Miller, P. W. (1995). The endogeneity between language and earnings: International analyses. J. Labor Econ. 13, 246–288. doi: 10.1086/298374
Chiswick, B. R., and Miller, P. W. (2002). Immigrant earnings: Language skills, linguistic concentrations and the business cycle. J. Popul. Econ. 15, 31–57. doi: 10.1007/PL00003838
Chiswick, B. R., and Miller, P. W. (2009). The international transferability of immigrants' human capital. Econ. Educ. Rev. 28, 162–169. doi: 10.1016/j.econedurev.2008.07.002
Damelang, A., and Abraham, M. (2016). You can take some of it with you! Zeitschrift Fur Soziologie 45, 91–106. doi: 10.1515/zfsoz-2015-1005
Di Stasio, V., Bram Lancee, S. V., and Yemane, R. (2021). Muslim by default or religious discrimination? Results from a cross-national field experiment on hiring discrimination. J. Ethnic Migr. Stud. 47, 1305–1326. doi: 10.1080/1369183X.2019.1622826
Dustmann, C., and Fabbri, F. (2003). Language proficiency and labour market performance of immigrants in the UK. Econ. J. 113, 695–717. doi: 10.1111/1468-0297.t01-1-00151
European Commission (2011). Transferability of Skills across Economic Sectors : Role and Importance for Employment at European Level. Luxembourg: Publications Office of the European Union.
Farkas, G. (2003). Cognitive skills and non-cognitive traits and behaviors in stratification processes. Ann. Rev. Sociol. 29, 541–562. doi: 10.1146/annurev.soc.29.010202.100023
Feliciano, C. (2005). Educational selectivity in US immigration: How do immigrants compare to those left behind? Demography 42, 131–152. doi: 10.1353/dem.2005.0001
Fernandez, F., and Liu, H. (2019). Examining relationships between soft skills and occupational outcomes among U.S. adults with—and without—University Degrees. J. Educ. Work 32, 650–664. doi: 10.1080/13639080.2019.1697802
Fernandez, F., and Smith, W. (2015). Education and Wage Gaps: A Comparative Study of Immigrant and Native Employees in the United States and Canada. American Institutes for Research. Available online at: http://piaacgateway.com/s/Smith_Fernandez_PIAAC.pdf
Ferrer, A., David, D. A., and Green, W. C. (2006). The effect of literacy on immigrant earnings. J. Hum. Resour. 41, 380–410. doi: 10.3368/jhr.XLI.2.380
Friedberg, R. M. (2000). You can't take it with you? Immigrant assimilation and the portability of human capital. J. Labor Econ. 18, 221–251. doi: 10.1086/209957
Hanushek, E. A., Guido Schwerdt, S. W., and Woessmann, L. (2015). Returns to skills around the world: Evidence from PIAAC. Eur. Econ. Rev. 73, 103–130. doi: 10.1016/j.euroecorev.2014.10.006
Heckman, J. J., and Kautz, T. D. (2012). NBER Working Paper Series Hard Evidence on Soft Skills Hard Evidence on Soft Skills.
Heckman, J. J., Stixrud, J., and Urzua, S. (2006). The effects of cognitive and noncognitive abilities on labor market outcomes and social behavior. J. Labor Econom. 24, 411–482.
Kanas, A., and Steinmetz, S. (2021). Mind the gap: The role of family policies and the gender-egalitarian climate in shaping gender and ethnic labour market inequalities in Europe. Migrat. Stud. 9, 1569–1589. doi: 10.1093/migration/mnab035
Kanas, A., and Van Tubergen, F. (2009). The impact of origin and host country schooling on the economic performance of immigrants. Soc. Forces 88, 269. doi: 10.1353/sof.0.0269
Kerckhoff, A. C., Raudenbush, S. W., and Glennie, E. (2001). Education, cognitive skill, and labor force outcomes. Sociol. Educ. 74, 1–24. doi: 10.2307/2673142
Keslair, F. (2020). “Analysing PIAAC data with stata,” in Large-Scale Cognitive Assessment. Methodology of Educational Measurement and Assessment, eds D. Maehler and B. Rammstedt (Cham: Springer). doi: 10.1007/978-3-030-47515-4_7
King, G., Christopher JL Murray, J. A. S., and Tandon, A. (2003). Enhancing the validity and cross-cultural comparability of measurement in survey research. Am. Polit. Sci. Rev. 97, 567–583. doi: 10.1017/S0003055403000881
Kosyakova, Y., and Laible, M.-C. (2022). Importance of personality for destination language acquisition: Evidence for refugees in Germany. Int. Migrat. Rev. 2022, 1979183221132538. doi: 10.1177/01979183221132538
Laible, M. C., and Brenzel, H. (2022). Does personality matter? Non-cognitive skills and the male migrant wage gap in Germany. Int. Migrat. Rev. 56, 376–409. doi: 10.1177/01979183211037315
Lancee, B., and Bol, T. (2017). The transferability of skills and degrees: Why the place of education affects immigrant earnings. Soc. Forces 96, 691–716. doi: 10.1093/sf/sox058
Midtbøen, A. H. (2013). The invisible second generation? Statistical discrimination and immigrant stereotypes in employment processes in Norway. J. Ethnic Migrat. Stud. 40, 1657–1675. doi: 10.1080/1369183X.2013.847784
Pager, D., Bonikowski, B., and Western, B. (2009). Discrimination in a low-wage labor market: A field experiment. Am. Sociol. Rev. 74, 777–799.
Rammstedt, B. S., Martin, A., Zabal, I., Konradt, D., Maehler, A., Perry, N., et al. (2016). Programme for the International Assessment of Adult Competencies (PIAAC), Germany – Reduced Version. Data File Version 2.2.0 [ZA5845]. Cologne: GESIS Data Archive. doi: 10.4232/1.12660
Schmidt, R., Kristen, C., and M?hlau, P. (2022). Educational selectivity and immigrants' labour market performance in Europe. Euro. Sociol. Rev. 38, 252–268.
Van de Kerckhove, W., Mohadjer, L., and Krenzke, T. (2013). “Treatment of outcome-related non-response in an international literacy survey,” in Joint Statistical Meetings, Survey Research Methods Section (Montreal, QC).
Van Soest, A., Liam Delaney, C. H., and Arie Kapteyn, J. P. S. (2011). Validating the use of anchoring vignettes for the correction of response scale differences in subjective questions. J. R. Statist. Soc. A 174, 575–595. doi: 10.1111/j.1467-985X.2011.00694.x
Keywords: immigrants, labor market inequalities, PIAAC, Europe, non-cognitive skills
Citation: Kanas A and Fenger M (2023) Non-cognitive skills and immigrant-native inequalities in the labor market in Europe. Front. Polit. Sci. 5:1091997. doi: 10.3389/fpos.2023.1091997
Received: 07 November 2022; Accepted: 24 April 2023;
Published: 18 May 2023.
Edited by:
Debora Pricila Birgier, University of Gothenburg, SwedenReviewed by:
Marion Mercier, UMR8007 Laboratoire d'Economie de Dauphine (LEDA), FranceCopyright © 2023 Kanas and Fenger. This is an open-access article distributed under the terms of the Creative Commons Attribution License (CC BY). The use, distribution or reproduction in other forums is permitted, provided the original author(s) and the copyright owner(s) are credited and that the original publication in this journal is cited, in accordance with accepted academic practice. No use, distribution or reproduction is permitted which does not comply with these terms.
*Correspondence: Agnieszka Kanas, a2FuYXNAZXNzYi5ldXIubmw=
Disclaimer: All claims expressed in this article are solely those of the authors and do not necessarily represent those of their affiliated organizations, or those of the publisher, the editors and the reviewers. Any product that may be evaluated in this article or claim that may be made by its manufacturer is not guaranteed or endorsed by the publisher.
Research integrity at Frontiers
Learn more about the work of our research integrity team to safeguard the quality of each article we publish.