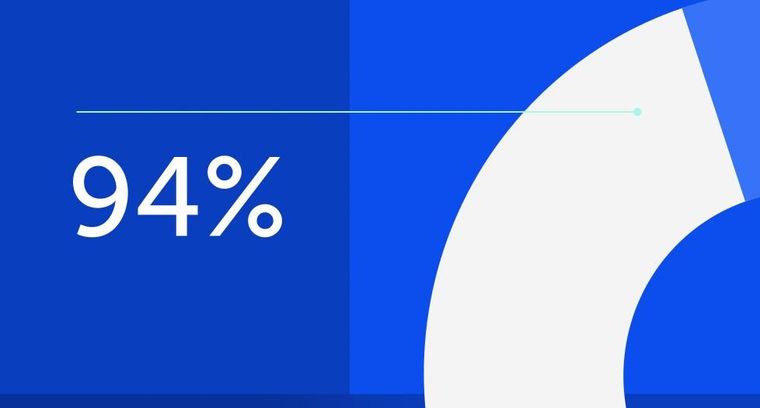
94% of researchers rate our articles as excellent or good
Learn more about the work of our research integrity team to safeguard the quality of each article we publish.
Find out more
ORIGINAL RESEARCH article
Front. Polit. Sci., 07 September 2022
Sec. Political Participation
Volume 4 - 2022 | https://doi.org/10.3389/fpos.2022.921188
This article is part of the Research TopicAffective Polarisation in Comparative PerspectiveView all 9 articles
Despite its potentially pernicious consequences for social relations and democracy, the study of affective polarization has only recently proliferated. Thus, the reasons driving this development—or its consequences—are not yet adequately understood. This article addresses the role of one specific factor frequently discussed in both academic and popular debate—namely, the role of crosscutting communication among people of different political leanings. It is a longstanding notion that crosscutting communication is crucial to overcoming the prejudice, polarization, and attitudinal biases brought on by streamlined information diets. However, there is empirical evidence to suggest that crosscutting experiences sometimes elevate polarization—especially when individuals also have access to like-minded views and when disagreement is perceived as intense. The study sheds light on the connection by testing hypotheses about the association between crosscutting communication and affective polarization in both offline and online modalities of political communication. The empirical analyses were based on panel data from the E-DEM project covering a random sample of Spanish citizens interviewed up to three times between November 2018 and May 2019—that is, the time running up to the Spanish national election in 2019. The results suggest that individuals who reported engagement in face-to-face discussions with supporters of various parties (crosscutting discussions) during this time reported significantly lower levels of affective polarization compared to engagement in discussions with co-partisans exclusively. Online crosscutting and consensual discussion experiences, however, were linked to comparable levels of anti-out-group sentiment, suggesting that concerns about the impact of online communication being different from offline communication in general—and perhaps more harmful—may be overstated. Descriptive evidence furthermore indicates that most respondents who engaged in political discussions had experiences of discussions with both co-partisans and supporters of opposing parties rather than co-partisans exclusively. Again, this was true for offline and online communication alike. Insofar as the results translate to other contexts as well, they indicate that future efforts to explain any surges in affective polarization should primarily be focused on other areas of inquiry.
Recent years have seen an upsurge in affective polarization in many countries, whereby supporters of different political parties increasingly express distrust and resentment toward each other on a personal level (Iyengar et al., 2012; Druckman and Levendusky, 2019; Reiljan, 2020). In pluralist communities, some degree of affective polarization is unavoidable, but researchers have highlighted various potentially negative consequences for the political system and beyond (McCoy et al., 2018). Although empirical evidence is limited to date, it has been suggested that high and sustained levels of affective polarization risk poisoning social relations (Druckman and Levendusky, 2019; Iyengar et al., 2019), eroding trust in democratic institutions (Torcal and Carty, 2022), and leading to the legitimization of autocratic tendencies to keep mistrusted groups away from political influence (Garrett et al., 2014; Mason, 2016; McCoy et al., 2018). Despite the worry of pernicious consequences for social relations and democracy, the study of this phenomenon has only recently proliferated (Reiljan, 2020). Thus, the reasons driving this development—and its consequences—are not yet adequately understood, especially in contexts beyond the United States (Gidron et al., 2020).
In this paper, I focus on one potential source of affective polarization frequently featured in both academic and popular debate—namely the communication that flows horizontally between citizens in their informal social networks and the extent to which they comprise dissenting perspectives and opinions (Iyengar et al., 2019; Levendusky and Stecula, 2021; Santoro and Broockman, 2022). I refer to the communication that occurs between citizens with various political voting preferences as crosscutting communication, in keeping with earlier studies (e.g., Mutz, 2002, 2006; Lee et al., 2015; Matthes et al., 2019). The relationship between crosscutting communication and attitudes, feelings, and behaviors is still a matter of debate, both theoretically and empirically. On the one hand, a longstanding notion in social and political theory is that the exchange of dissimilar views has an important social and democratic function (Mutz, 2002). Exposure to a diversity of perspectives has been considered important to counter polarization (Sunstein, 2002) and prejudice (Allport, 1954), and to promote intergroup tolerance (Mutz, 2002), and well-reflected political views (Arendt, 1968; Manin, 1987; Habermas, 1989). However, social psychologists have pointed out that the processing of counter-attitudinal information is cognitively and emotionally challenging, and that it may activate biased information processing that reinforces prejudice and polarization (Festinger, 1954; Druckman and McGrath, 2019; Little, 2019). While previous empirical research suggests that pro-attitudinal information tends to reinforce attitudes and elevate polarization (Padró-Solanet and Balcells, 2022), empirical evidence on the role of counter-attitudinal information has generated inconsistent findings (Kubin and von Sikorski, 2021). More specifically, empirical research has revealed links between crosscutting communication and both attitude moderation (e.g., Mutz, 2002, 2006; Parsons, 2010; Lee et al., 2015), and attitude reinforcement (Taber and Lodge, 2006; Karlsen et al., 2017; Bail et al., 2018).
The study contributes to the extant body of research by addressing several as-of-yet unresolved issues. First, I develop and subsequently test hypotheses about the association between crosscutting communication and affective polarization. In line with previous research (e.g., Wojcieszak and Price, 2010; Kim, 2019), I lean on ideas about deliberative and biased information processing, to anchor the hypotheses in theory. Second, I describe and test the relationship in both the offline and online modes of political communication. Although concerns abound in both scholarly and lay sectors that social media, in particular, and online communication, in general, may exacerbate polarization in society (Pariser, 2011; Sunstein, 2018)—empirical studies have reached different conclusions (Kubin and von Sikorski, 2021; Nordbrandt, 2021b; Lorenzo-Rodríguez and Torcal, 2022). Moreover, a limited number of studies hitherto (Wojcieszak and Price, 2010; Baek et al., 2012; Strandberg et al., 2019; Fletcher et al., 2020) have contrasted the attitudinal implications of offline and online communication within the same study, let alone with identical measures, hampering the possibilities for fruitful comparisons between the offline and online modes of political talk. With its aim to throw further light on the relationship between affective polarization and crosscutting communication in both offline and online modes of political communication, this study contributes toward filling this gap. Third, the study contributes to the growing comparative research agenda on affective polarization polarization (Gidron et al., 2020; Reiljan, 2020; Boxell et al., 2022), by zooming in on Spain at an interesting point in time—namely, the 6 months preceding the 2018–2019 election year. With the discussion over Catalonian independence and the nationalist far-right party Vox's symbolic parliamentary entry, this was a period of increased and growing affective polarization in the Spanish population (Torcal and Comellas, 2022). Up until now, studies focusing on the situation in the United States have contributed significantly to the body of knowledge about affective polarization (e.g., Iyengar et al., 2012, 2019; Garrett et al., 2014; Iyengar and Westwood, 2015; Mason, 2015, 2016; Rogowski and Sutherland, 2016; Druckman and Levendusky, 2019; Druckman et al., 2021). Because the United States is far from being representative of all countries, it is crucial to extend knowledge about the sources and consequences of affective polarization in other country contexts—despite the challenges in measuring polarization in multiparty systems (Wagner, 2021).
The empirical analyses were based on panel survey data from the E-DEM project (Torcal et al., 2020). These data provide exciting and relatively rare possibilities to isolate over time changes within the unit of analysis (individuals in this case) instead of compare mean differences between individuals, which is the confinement of cross-sectional data. E-DEM covers a sample of Spanish citizens interviewed up to four times between November 2018 and May 2019. According to the findings, face-to-face participation in crosscutting debates was linked to a statistically significant lower level of anti-out-party sentiments than consensual conversations with fellow partisans. No discernible difference was found between crosscutting and consensual experiences in the online mode of political communication. Descriptive evidence furthermore indicates that crosscutting discussions were the most frequent form of political communication in the time preceding the Spanish 2018–2019 election year. Again, this was true for offline and online communication alike. This finding is noteworthy because it contradicts a widespread assumption in the popular and scholarly discourse that online networks tend to be characterized overwhelmingly by opinion homophily—not least on social media (Pariser, 2011; Sunstein, 2018)—and it agrees with studies that have challenged this notion (Dubois and Blank, 2018; Eveland et al., 2018). Importantly, it speaks against the idea that “echo chambers” are a major driver of affective polarization in general. Although the study found no indication of de-polarization in crosscutting communication, the results are nonetheless comforting in that they show that crosscutting exchanges do not always result in disturbingly high levels of intergroup hostility. The study further yielded no compelling support in favor of the notion that online discussions, in general, play a different (and more harmful) role in polarization processes than face-to-face discussions. Insofar as the results translate to other contexts as well, they indicate that future efforts to explain any surges in affective polarization should primarily be focused on other areas of inquiry.
The rest of the paper is organized as follows: I start by outlining previous research on affective polarization and its origins followed by a theoretical discussion on the mechanisms linking crosscutting communication to either de-polarization or elevated polarization. I conclude by formalizing hypotheses regarding the association in both offline and online political discourse. Then, before reporting the results, I describe the data and my methods. I conclude by discussing the implications of the results, and I point to avenues for future study.
Challenging the dominant understanding of mass polarization of the time, Iyengar, Sood, and Lelkes argued in 2012 that the most consequential and pernicious form of mass polarization is affective, not ideological. In their seminal article, Iyengar et al. (2012) defined affective polarization as peoples' proclivity to view opposing partisans negatively and co-partisans positively. Since then, scholars have widely embraced this notion and the research field is growing rapidly.
Affective polarization is commonly viewed as a byproduct of partisan identity, and it is intertwined with the processes of social identity formation (Huddy, 2001; Mason, 2015, 2016; Iyengar et al., 2019). Decades of research into the formation of social identity have revealed that people have an innate desire to form social bonds and feel a sense of belonging (Tajfel and Turner, 1979). Integral parts of these processes include the identification of different social groups with which a person feels kinship—that is, “in-groups.” Conversely, people who fall outside the in-group are characterized as “out-group members” (Tajfel and Turner, 1979; Huddy, 2001). Social bonding and identification thus necessarily involve categorization into in-group and out-groups because there cannot be a “we” if there is no analogous “they” (Hogg, 2018). From a social identity perspective, affective polarization may intensify as party identities become increasingly salient whereby more people divide the world into a liked and trusted political in-group and a disliked and distrusted political out-group (Mason, 2015, 2016).
Many factors likely interact to intensify the “we vs. they” categorization (Iyengar et al., 2019). Previous studies have proposed reasons including heightened ideological sorting (Mason, 2016; Rogowski and Sutherland, 2016), a political and high-choice media environment (Berry and Sobieraj, 2013; Del Vicario et al., 2016; Lelkes et al., 2017; Tucker et al., 2018; Iyengar et al., 2019), as well as negative campaign advertising and stereotypes (Sood and Iyengar, 2016). While there is empirical evidence to back up these notions, there have also been findings that call for cautiousness in prematurely interpreting all these factors as major culprits behind surges in affective polarization (Gentzkow and Shapiro, 2010; Prior, 2013; Nordbrandt, 2021b; Lorenzo-Rodríguez and Torcal, 2022; Torcal and Comellas, 2022).
This article specifically focuses on a possible relationship between affective polarization and crosscutting communication in informal social networks (Iyengar and Westwood, 2015; Iyengar et al., 2019). Because affective polarization is a relatively new area of research, evidence on this precise link is limited. Nevertheless, some general observations can be made of the state of the art on the link between crosscutting communication and polarization broadly defined. The first point is that empirical studies have used different designs and operationalization. The concept of communication can refer to the act of sending a message from one actor to a receiver (which results in exposure), or an interactive exchange of information between at least two individuals (discussion). Most empirical evidence so far has been based on observations of information exposure from traditional media like TV, radio, and newspapers (e.g., Kim, 2015, 2019; Padró-Solanet and Balcells, 2022), as well as online sources like social media, news websites, and blogs (e.g., Garrett et al., 2014; Barberá et al., 2015; Beam et al., 2018). Fewer studies have studied the discussion in real-world offline settings (e.g., Parsons, 2010; Druckman and Levendusky, 2019; Levendusky and Stecula, 2021) and the virtual world (e.g., Wojcieszak and Price, 2010; Santoro and Broockman, 2022). Some evidence is derived from self-reported perceptions of exposure or discussion (e.g., Parsons, 2010; Garrett et al., 2014; Kim, 2015, 2019), other studies track actual exposure (e.g., Barberá, 2015; Levendusky and Stecula, 2021; Padró-Solanet and Balcells, 2022), and still, other pieces of evidence are based on experimental manipulation of crosscutting exposure or discussion (e.g., Taber and Lodge, 2006; Wojcieszak and Price, 2010; Karlsen et al., 2017; Bail et al., 2018; Druckman and Levendusky, 2019; Guess and Coppock, 2020; Wojcieszak et al., 2020; Santoro and Broockman, 2022). Previous studies further leaned on different measures of polarization, ranging from issue (e.g., Taber and Lodge, 2006; Wojcieszak and Price, 2010; Karlsen et al., 2017; Druckman and Levendusky, 2019), and ideological polarization (e.g., Barberá, 2015; Bail et al., 2018), to elite and mass affective polarization (e.g., Garrett et al., 2014; Kim, 2015, 2019; Beam et al., 2018; Guess and Coppock, 2020; Wojcieszak et al., 2020; Levendusky and Stecula, 2021; Padró-Solanet and Balcells, 2022; Santoro and Broockman, 2022). Lastly, evidence furthermore mainly comes from the United States. The bulk of the above-referenced studies draws inferences from the United States with unclear implications for transferability to other contexts.
The results of earlier investigations do not consistently point to many systemic trends. There is evidence of both growing (e.g., Taber and Lodge, 2006; Wojcieszak and Price, 2010; Garrett et al., 2014; Karlsen et al., 2017; Bail et al., 2018; Kim, 2019) and attenuated (e.g., Parsons, 2010; Barberá, 2015; Beam et al., 2018; Druckman and Levendusky, 2019; Guess and Coppock, 2020; Levendusky and Stecula, 2021; Padró-Solanet and Balcells, 2022) levels of polarization linked to crosscutting communication in studies with varied designs and measurements across different modalities of communication (offline/online). Some have suggested that the implications of crosscutting communication likely have scope conditions such as the presence of deliberative norms (Wojcieszak et al., 2020; Levendusky and Stecula, 2021), neutral dissemination of information (Karlsen et al., 2017), the saliency of national identity (Padró-Solanet and Balcells, 2022), and the topic of discussion (Santoro and Broockman, 2022).
In sum, previous research lends itself to at least two hypotheses about the relationship between crosscutting communication and affective polarization: it could be associated with (H1) attenuated, or (H2) elevated levels of polarization. To build more solid links between theory and these expectations, I will anchor the hypotheses in two primary strands of theory—namely, theories about deliberative and biased information processing, respectively.
For centuries, scholars from various schools of thought have highlighted political talk in the informal public sphere, and especially communication between citizens whose political views differ, as one of the core elements of democratic life (Manin, 1987; Habermas, 1989; Mutz, 2002). To explain why it may be useful to demonstrate what it is that crosscutting communication is presumed to ease.
Although social grouping is instinctive according to social identity theory (Tajfel and Turner, 1979; Huddy, 2001; Hogg, 2018), social grouping, and homophily can be troublesome for societal and political relations. Allport (1954) famously proposed that social categorizing and interaction in socially homogeneous networks might invoke group thinking and prejudice: “an antipathy based upon a faulty and inflexible generalization or stereotyping [...]. It may be directed toward a group, or an individual because he is a member of that group” (Allport, 1954; p. 9). Scholars like Sunstein (2002) and others (e.g., Isenberg, 1986; Farrar et al., 2009), argue that information sharing in politically homogeneous groups of like-minded people can cause attitudes to shift toward the extreme because of the social comparison and confirmation bias that such a form of communication can provoke (e.g., Binder et al., 2009; Grönlund et al., 2015; Lee et al., 2015). In this view, social comparison is elicited in all types of group situations. When like-minded people come together in a group, they tend to alter their behavior in such a way as to prompt other members of the group to see them in a more favorable light. To signal their belonging to the group, they tend to adjust the attitudes they express to align with what they perceive to be the dominant position of the group, and/or to express these attitudes in a manner more antagonistic to the members of other groups (Isenberg, 1986; Sunstein, 2002; Farrar et al., 2009). This may lead the group members to converge toward opinions that are more extreme than they were initially and sharply at odds with those held by out-groups, or to engage in “othering” of out-group members (Spivak, 1985). Othering goes beyond the natural reflex to sort people into the in-group or an out-group as it involves an often-unconscious normative judgment where the out-group is constructed as inferior, morally or psychologically, compared to the in-group. As a result, othering and the idea of affective polarization are related. According to empirical evidence, communication among homogeneous groups tends to reinforce pre-held beliefs, resulting in attitude polarization (Padró-Solanet and Balcells, 2022).
A long line of scholars has instead assigned crosscutting communication great value as a potential antidote to the problematic sides of social homophily (e.g., Mill, 1859; Arendt, 1968; Manin, 1987; Habermas, 1989; Mutz, 2002). Scholars of social and political theory have believed that communication involving contrasting perspectives and views can stimulate a deeper reflection on one's views and the reasons underpinning others' views. This type of more effortful processing of information where individuals take both new and old as well as pro and con arguments into consideration, is here referred to as deliberative information processing (Mercier and Landemore, 2012). Mill (1859) and Arendt (1968) both made classic claims that communication across differences is a crucial component of political life. Mill (1859) emphasized its ability to fill in knowledge gaps and correct false perceptions, opening the door for more reasoned political judgments. Instead, Arendt (1968) emphasized its ability to familiarize people with viewpoints different from their own and to foster a deeper understanding of the views and circumstances of others who are differently situated—something that is frequently referred to as “enlarged mentality” or “representative thinking” (Mutz, 2002; Wojcieszak and Price, 2010). On the face of it, the latter thoughts have clear parallels to Allport's (1954) famous ideas about intergroup contact. Allport contended that regular contact with members of out-groups—especially in informal, interpersonal, and friendly environments—should alleviate anxiety and negative stereotyping and increase empathy toward members of the out-group. Political intergroup contacts may be most powerful if members of different political groups get to know each other on a personal plane before they learn about their attitudes and group identity. If people get a chance to uncover non-political shared values and interests, they may become less prone to develop hostile feelings once disagreeable political leanings come to the surface (Mutz, 2002; Santoro and Broockman, 2022). In sum, crosscutting communication may help participants overcome the stereotyping encouraged by groupthink by furthering their understanding of the perspectives and circumstances of out-group members. Simply put, it should be more difficult to harbor great antipathy for an individual who has opinions that you find objectionable if you are aware of their motivations for thinking that way or if you can form a bond with them that is unrelated to politics.
Previous empirical studies on intergroup contact in general and crosscutting communication specifically have garnered evidence in line with expectations of deliberative information processing. Several meta-analyses have concluded that both direct and indirect contacts between out-group members tend to be associated with less prejudiced attitudes (Tropp and Pettigrew, 2006; Pettigrew et al., 2011; Lemmer and Wagner, 2015; Dovidio et al., 2017). Some more recent studies have however, indicated that the positive effects of intergroup contact is less generic than previously assumed and that they may depend on some scope conditions (Dinesen et al., 2019; Paluck et al., 2019). For instance, prejudice can vary in type, with some types being “more malleable than others” (Paluck et al., 2019, p. 153). Additionally, it is still debatable whether interactions between members of different groups on a deeper, more personal level—what Allport referred to as “high-quality contact”—have a greater anti-prejudice impact than less formal interactions (Paluck et al., 2019). Studies of political communication between opposing partisans have furthermore disclosed links to various democratic goods that may be indicative of deliberative information processing. These include depolarized emotions (Parsons, 2010; Levendusky and Stecula, 2021); reflection from different standpoints (Lee et al., 2015); an increased propensity to adjust opinions when faced with persuasive arguments (Levitan and Visser, 2008; Himmelroos and Christensen, 2020); awareness of multiple rationales (Cappella et al., 2002; Price et al., 2002; Nir, 2005); more public-minded attitudes (Nordbrandt, 2021a); and greater tolerance toward out-group members (Mutz, 2002, 2006). Most of these studies, however, lean on cross-sectional data, which restricts the analyses to between-unit variation.
Based on a model of deliberative information processing in attenuating prejudice, stereotyping, and othering, the following hypothesis can be derived:
H1: Crosscutting communication is associated with attenuated levels of affective polarization.
Echoing (Mutz, 2002, p. 112), it would however be “quite naive to suggest that only good can flow from cross-cutting interactions; conversations among those of differing views also have the capacity to result in bitter arguments, violence, and/or a hostile and uneasy silence.” Social psychological theory has suggested that a host of psychological and affective mechanisms conspire to elicit biased information processing when people are presented with dissonant messages that risk offsetting the prejudice-reducing and depolarizing potential of crosscutting communication (Festinger, 1954; Druckman and McGrath, 2019; Little, 2019).
The tendency known as motivated reasoning, for instance, is a dissonance-reduction strategy whereby people inadvertently use various self-serving strategies to conclude what they wish to arrive at, even when faced with balanced arguments for and against (Kunda, 1990). Scholars have asserted that people tend to find it hard to “escape the pull of their prior attitudes and beliefs” (Taber and Lodge, 2006, p. 767) and stay open-minded despite disagreeable information, and “often employ mental gymnastics to avoid the implications of facts that challenge their political views” (Kuklinski, 2007, p. 1).
To establish connections between polarization of the affective sort and disagreement that are more precise, earlier studies have shown that emotions may be crucial toward comprehending the mechanisms underlying motivated reasoning. Consonant information is typically perceived as reassuring and “safe,” dissonant information instead tends to provoke uneasy feelings such as frustration, anger, or insecurity (Festinger, 1954; Marcus et al., 2000; Wojcieszak and Price, 2010). Affective responses associated with crosscutting exchanges may thus prompt an inadvertent need to rationalize the dismissal of the sources of the information by depicting them as unsympathetic, hypocritical, or unreliable. Ascribing out-group members to such traits is indeed a part of the concept of affective polarization (Druckman and Levendusky, 2019).
With regard to empirical research, evidence of a “backfire effect” (Bail et al., 2018) associated with counter-attitudinal information has been mixed. In an experimental study, Taber and Lodge (2006) notably discovered that participants tended to find evidence that supported their preconceived views more convincing than evidence that did not. Faced with disconfirming information, people spent a substantial amount of time arguing against it, while taking affirmative messages at face value. Both types of information elicited a biased information response, with affirmative messages leading to confirmation bias and incongruent information provoking disconfirmation bias with intensified attitude polarization as a result. Similarly, other more recent studies have furnished empirical evidence of backlash, whereby exposure to disconfirming messages reinforced preconceived notions and exacerbated polarization (e.g., Beam et al., 2018; Guess and Coppock, 2020; Levendusky and Stecula, 2021). However, Guess and Coppock (2020) recently demonstrated that evidence of backfire effects has been refuted by several studies, and they discovered that in three experimental trials, attitudinal reinforcement in response to counter-attitudinal information was the exception rather than the rule. Results from a study by Barabas (2004) suggest that the risk of backlash is higher in colloquial, non-moderated, communicative settings compared to discussion settings where deliberative norms such as civility and respectfulness are encouraged, such as deliberative forums.
Based on theories of motivated reasoning and (dis) confirmation bias, I propose the following competing hypothesis on the relationship between crosscutting communication and affective polarization:
H2a: Crosscutting communication is associated with elevated levels of affective polarization.
Another outcome consistent with biased information processing is, however, also possible. Not every empirical investigation that found crosscutting communication to be associated with relatively low levels of polarization provides evidence of de-polarization—that is, evidence of attitude moderation. Some studies indeed lend themselves to the suggestion that de-polarization has occurred (e.g., Beam et al., 2018; Guess and Coppock, 2020; Levendusky and Stecula, 2021), but other findings could rather indicate that polarization was simply lower in crosscutting settings than it was in homogeneous settings. Druckman and Levendusky (2019) found that people in heterogeneous discussion groups in fact did polarize relative to people who were not exposed to political information at all, but less than people in consensual discussion groups.
This possibility translates to the following hypothesis:
H2b: Crosscutting communication is associated with lower levels of affective polarization than consensual communication, but higher levels than the absence of political communication.
Scholars have further argued that people may revert more readily to motivated reasoning when faced with conflicting views if they also have access to information that reinforces their preconceived notions (Karlsen et al., 2017). As a result, a setting that encourages self-affirmation along with crosscutting exposure may encourage people to employ motivated reasoning to neutralize contradictory information. (Karlsen et al., 2017, p. 260–261) call this dynamic “trench warfare”: “people will interact and engage in debate with others who hold opposing political views, but this will only serve to strengthen their initial beliefs.” Note that Karlsen et al. (2017) offer their conceptualization of trench warfare about Internet communication and the dynamics of social media. On that note, I will now discuss how the change from a mainly analog to a world growing increasingly digital has affected public reasoning and possibly polarization processes.
The conditions for communication have undergone significant shifts in recent decades. Taking off in the 1990s, with the proliferation of the Internet, the number of available information channels exploded, and an ever-increasing number of people relied on online media channels for their information. However, there is little overlap in the study on the effects of offline and online political communication on citizens' daily lives. While some scholars are interested in both forms (e.g., Beam et al., 2018; Guess and Coppock, 2020; Levendusky and Stecula, 2021), few studies have appraised the effects of both in concert, even though previous studies have indicated that offline and online communications differ from each other in important regards (Baek et al., 2012).
One difference between online and offline social networks is the volume of crosscutting information that is shared in online spaces like social media. Sunstein's thesis, with its “echo chamber” allegory, offers one of the most pessimistic, thoroughly studied, and widely accepted interpretations of social media (Sunstein, 2002, 2018; Pariser, 2011; Beam et al., 2018). The echo chamber thesis rests on the notion that, when given the choice, people often opt not to subject themselves to situations and information that challenge their preconceptions to avoid cognitive dissonance and its ensuing stress. The accessibility of various online platforms and the option to remove unwanted information by selecting surroundings that echo one's preconceptions can be expected, in this view, to reduce the incentive to engage in deliberative information processing. Contrary to popular belief, a sizable body of empirical research has repeatedly discovered that people unintentionally come across more crosscutting information on online platforms like social media than they do in their face-to-face networks (Wojcieszak and Mutz, 2009; Barberá et al., 2015; O'Hara and Stevens, 2015; Barnidge, 2017; Dubois and Blank, 2018; Dahlgren, 2021). This means, that the most pertinent question we should be asking ourselves is not whether online sites are problematic from a democratic perspective because they are “echo chambers.” The arguably more interesting question, considering these findings, is of whether online platforms may promote polarization as a pluralist space where users regularly encounter both pro-attitudinal and counter-attitudinal information.
The second difference is the amount of uncivil discourse in online communication, as compared with that seen in the unmediated offline environment (Rowe, 2015; Bail et al., 2018; Theocharis et al., 2020, p. 2). According to empirical data, consumers frequently regard online environments as places where traditional standards of decency are more easily violated than they do in networks that enable face-to-face interaction, and online communication frequently elicits more unpleasant feelings than in-real-life (IRL) interaction environment (Rowe, 2015; Bail et al., 2018; Theocharis et al., 2020, p. 2). Simultaneously, scholars often assume that norms such as respectfulness and reciprocity are important for realizing deliberative as opposed to biased information processing (Baek et al., 2012; Chen and Lu, 2017; Pew Research Center, 2020). A tendency on the part of the human psyche to experience discomfort, anxiety, or even fear of social isolation when confronted with disagreement (Grönlund et al., 2015; Strandberg et al., 2019; Wojcieszak et al., 2020; Levendusky and Stecula, 2021) indicates the possible importance of norms and institutions that encourage respectful interchange to counteract selective judgment and motivated reasoning. This is also congruent Allport's assumption that intergroup contact should “be of a sort that leads to the perception of common interests and common humanity between members of the two groups” (Allport, 1954, p. 281) to engender intergroup sympathy (Levendusky and Stecula, 2021). Empirical research has connected exposure to online rudeness to decreased political trust, and the polarization of thought (Anderson et al., 2014; Borah, 2014; Lyons and Veenstra, 2016). However, empirical data also support the advantages of promoting objectivity in crosscutting discussions to lessen information processing bias (Wojcieszak et al., 2020).
If the operation of at least some social or deliberative norms is important for constructive intergroup contacts, we can expect online communication to be worse equipped than its offline counterpart to counteract polarization driven by negative affect. It may simply be easier to violate norms of civility when communication is mediated by a screen than when an actual person is sitting in front of you.
To throw further light on the empirical patterns, I pose and test the following hypothesis:
H3: Online communication is associated with a higher level of affective polarization than offline communication.
The study leaned on large-N panel survey data from the E-DEM project (Torcal et al., 2020), which was collected in the months before the Spanish general parliamentary election and the EU election in 2019. The panel covered a random sample of 2,501 Spanish citizens surveyed up to four times from November 2018 to May 2019. The sample, which was made up of 8,109 distinct responses with an average completion rate of roughly 76%, was created by nesting each survey occasion (or wave) within the one before it. The sample was stratified by gender, age, and region of residency, to enhance the representativeness of the sample in relation to the population. No substantial deviations between the sample and the socio-demographic composition of the population were reported. The panel design was strongly balanced, meaning that no additional participants were recruited along the way. It did contain gaps, meaning that not every respondent completed the interviews in each wave.1 However, I kept the final analyzed sample constant in the main models, to rule out the possibility that any changes between models would depend on the composition of the analyzed sample. There were no significant socio-demographic, socioeconomic, or political interest differences from the sample, according to an analysis of the final sample's representativeness. All in all, the study leaned on observations of 1,611 unique individuals observed 4,180 times over three-panel waves to gauge the relationship between crosscutting communication and affective polarization.
One of the major benefits of panel data vis-à-vis cross-sectional data is the possibility of retrieving within-unit estimates (Angrist and Pischke, 2008; Bell et al., 2018). Here, the within-unit estimates denote the predicted level of out-group dislike when an individual engages in crosscutting discussion in comparison with the predicted level of out-group dislike for that same person when she engaged in consensual discussions2. All observed and unobserved potential confounding factors that do not change over time are effectively maintained constant by fixing the analysis to the estimation of projected over time changes for one individual instead of comparing differences between individuals. Such factors include sex, ethnicity, parents, and stable personality traits, as well as other experiences in a person's background. All models further included fixed effects based on the panel wave (the month of completion of the survey), to abate the risk that events over the course of the election campaign that affected all respondents equally confounded the relationship3.
Moreover, leaning on panel data provides an opportunity to model the lagged value of the dependent variable among the covariates. Such a model effectively removes all potential bias from the residual that reflects the correlation between the predictors and the past values of the dependent variable (Beck and Katz, 2011). Such a correlation would result from reciprocity between the predictors and the outcome variable. In this study, reciprocity would mean that a network configuration (crosscutting/consensual) does not unidirectionally cause people to become more polarized. Instead, the level of affective polarization would also affect a person's propensity to engage in offline and online discussions, which is theoretically viable considering recent findings (Nordbrandt, 2021b). It is widely held, however, that including the lag of the dependent variable in models that apply panel fixed or random effects is inappropriate, due to the risk of introducing significant Nickell bias (Achen, 2000; Allison, 2015). I also provide the outcomes of models that incorporate the lagged value of the dependent variable as well as models with alternative estimation approaches and model specifications in Appendix C to test the robustness of the main non-lagged fixed effects models.
To measure the respondents' level of affective polarization, I used two different items tapping the extent to which the respondents dislike and distrust political out-groups. The first question used the well-known “feeling thermometer,” (Druckman and Levendusky, 2019) which ranges from 0 to 100, to ask respondents to rank their attitudes toward various voter groupings. Ratings close to 100 indicated that the respondent had exceptionally favorable views about that group, while ratings close to 0 indicated the opposite. The second item asked respondents to rate the extent to which they trusted different voter groups on a scale between 0 and 10. The voting groups the respondents were asked to rate in both items were voters of the following five national parties: PSOE (Spanish Socialist Workers Party/Partido Socialista Obrero Español), PP (Popular Party/Partido Popular), CS (Citizens/Ciudadanos), UP (United We Can/Unidas Podemos), and the national conservative party Vox. Thus, the type of affective polarization addressed in this study is affective polarization involving supporters of out-group parties rather than elites. Factor analysis revealed that the “feelings” and “trust” items loaded onto the same factor, and the inter reliability between the items (Cronbach's alpha) was 0.86, indicating a strong correlation. Thus, the items were first normalized to range between 0 and 1 and then combined into an additive index.
Like other recent studies (Harteveld, 2021; Torcal and Comellas, 2022), the measure of affective polarization employed in this study is based on Wagner's (2021) individual-level “Affective polarization index” (API). A respondent's level of affective polarization is indicated by the “spread of dislike and distrust” across all voter groups. An individual with a very low level of polarized attitudes has similar feelings and levels of trust toward all groups. However, having wildly disparate emotions and degrees of trust for various organizations or collections of groups is a sign of strong polarization. The indicator shows each respondent's average variation from her individual mean each wave in her feelings and levels of trust, weighted by the percentage of votes each party received. Since it can be discussed whether affective polarization is always more severe when it involves larger parties, I also report a corresponding unweighted measure in Appendix C. The final measure was normalized, such that it took on the value of 0 if there was no difference in individuals' feelings for different voter groups compared to the previous wave; and it took on the value of 1 if there was a maximum deviation from the mean (m = 0.349, sd = 0.193). The formal equation (adapted from Wagner, 2021) is as follows:
where feelingsiv is the feeling expressed toward any given voter group by each respondent in each wave, and feelingsi is the mean sympathy score across all voter groups for each respondent in each wave. Vp is the vote share of each party in the most recent election, measured as a proportion ranging from 0 to 1. The mean effect is also weighted by party size:
One decisive advantage of this measure is that it does not presuppose a clear and strong party preference on the part of the respondents. Instead, it captures any feeling of discrepancy of a respondent between certain voter groups, or clusters of voter groups, whether he or she strongly identifies as a partisan, which arguably captures the empirical reality better than assuming the existence of positive party identification for all voters (Wagner, 2021). It further considers the frequent clustering of parties into blocks in multiparty systems whereby individuals' feelings may follow a corresponding block structure (Wagner, 2021; Torcal and Comellas, 2022). Appendix C contains the results using the alternative unweighted like–dislike measure proposed by Wagner (2021), as well as Wagner's (2021) unweighted and weighted measures based on the affective distance of other parties from a respondent's most liked party4.
The independent variable is a survey item asking the respondents the following: Do you think the people you talk to about politics: (3) support the same party as you? or (2) divide their support among different parties? or (1) support a different party than yours? Since the measure is categorical, I modeled it as a dummy variable, treating categories one and two as indicators of crosscutting discussions where all or some discussants have different party preferences than the respondent and treating category three as indicating consensual discussions (all discussants share the respondent's party sympathy). Grouping category two and three into a joint category is consistent with my ambition to gauge the difference between being embedded in a network of mainly consensual opinions vs. having crosscutting influences in the communication network. I however report extended analyses in Appendix C, where categories two and three were separated, but the results of these supplementary analyses did not change the conclusions. Those who reported having had no political discussions were also included for comparison as a baseline category.
A similar question asked about respondents' participation in the equivalent kinds of online discussions on social networking sites. To compare the connections between network configuration and affective polarization depending on the mode of political communication (offline/online), I leaned on this item to build an identically coded measure.
As I have already noted, the concept of communication encompasses both exposure and discussion. The measure I use should primarily capture the engagement in discussion5. This is worth noting because the discussion entails an interactive component that exposure lacks. It is more likely that the participants in such communication will pay attention (listen) to the arguments provided by differently-minded others to be able to respond properly. Therefore, I expect that any mechanisms that consensual and crosscutting communication elicited should be more pronounced when there is discussion as opposed to exposure, which is important to keep in mind when comparing studies that variably measure exposure and discussion.
Many individual-level, time-varying control variables were included in all models, to abate the risk of omitted variable bias6. First, the study isolated the relationship for overall frequency of political talk offline and online (0 = never to 6 = daily), to remove the risk that the amount of political talk correlated with whom one talked to, thereby confounding the effect of communication network configuration. The main models were otherwise restricted to the inclusion of time-varying control variables that can be regarded as at least weakly exogenous, thereby avoiding the risk that post-treatment bias would absorb, thus obscuring the main effect.
All the models isolated the relationship between education and income—variables that tend to correlate strongly with many other attitudinal and behavioral variables (Jeynes, 2002). To reduce the chance that political disposition, interest, and information consumption would determine social network configuration and levels of polarization, thereby confounding the relationship, I also included controls for ideological self-positioning, political interest, and overall information consumption from different news sources (TV, radio, newspapers, magazines, and social networks), and the strength of partisan identification in the main models. Because the time sequence between ideology, political interest, frequency of political discussion, and overall information consumption and the primary independent variables (crosscutting communication) is not self-evident, I also report models without these variables, in Appendix C. All measures in the reported models were standardized to range from 0 to 17.
Before reporting the main results, I will start by reporting some descriptive evidence, which, to my knowledge, is the first of its kind.
Table 1 shows the shares of consensual and crosscutting political discussions (and lack thereof) that the respondents in the E-DEM panel engaged in during the months preceding the national election 2019 and their overlap between the two communication modalities (offline/online). First, many respondents who did engage in some form of political discussion were embedded in a crosscutting communication network. This was the case for both the offline and online modes. Furthermore, the percentage of respondents who said they mostly engaged in consensual conversations was significantly outnumbered by the percentage of respondents who indicated they engaged in some level of crosscutting debate. Again, this was true for offline (≈67% crosscutting) vs. (≈8% consensual) and online communication (≈28% crosscutting vs. ≈3% consensual) alike.
Furthermore, markedly fewer people engaged in political discussions of any kind online than offline. Almost 70% reported having had no online discussions at all, as compared with about 23% offline. This might be explained by the difference between being passively exposed to political discourse and being actively engaged in political discussion. The survey items would appear mainly to capture engagement. When political topics come up online, it is easier to be a passive observer (Lieberman and Schroeder, 2020). When they come up in face-to-face interactions, it is more difficult to continue to be so. The table also reveals that physical and online communication networks share many similarities. There were no systematic differences in the network configuration depending on personal characteristics such as education, income, ideology, gender, age, or political interest (see Appendix B).
Turning to the main results: Table 2 shows a set of fixed effects panel models testing the relationship between crosscutting and consensual discussions offline and online and the level of affective polarization. Figure 1 displays the predictive margins on affective polarization across the different types of political communication (crosscutting/consensual/no discussion) for Model 1 (offline), Model 2 (online), and Model 3 (offline vs. online), with all control variables held at their means.
Table 2. Affective polarization regressed on communication types (cross-cutting/consensual/no discussion) and offline vs. online communication.
Figure 1. (A–C) The predictive margins for Model 1 (offline) and Model 2 (online) across the different types of political communication (crosscutting/consensual/no discussion), and for Model 3 (offline versus online) across the different communication modalities (No discussion/offline only/online only/offline and online). All control variables held at their means.
Starting with offline communication, the coefficients in Model 1 and the predicative margins in Figure 1 indicate that persons who engaged in crosscutting discussions were predicted to have 2.2% (p < 0.01) less polarized attitudes compared to a scenario when the same person only had discussions with like-minded co-partisans8. However, engagement in crosscutting discussions was associated with 1.7% (p < 0.1) more affective polarization compared to having no political discussions at all, but the difference was not statistically significant at the conventional level of p above 0.05. Since crosscutting communication was only clearly associated with attenuated levels of out-group dislike compared to engagement in consensual discussions, the results support Hypothesis 2b sooner than Hypothesis 1 or Hypothesis 2a. The coefficients, however, were admittedly quite small.
When we turn our attention to online communication, Model 2 shows that there were no appreciable changes in the levels of polarization across any communication type (crosscutting, consensual, and no discussion). This was because the coefficients were nearly null throughout. Importantly, this lack of difference between the coefficients was not driven by crosscutting communication being associated with more affective polarization online than offline, but rather that consensual discussions were associated with slightly less polarized sentiments in the online compared to the offline modality. This, however, only indicates that there was no discernable difference between crosscutting and consensual communication in the online mode (H1 and H2a/H2b were unsupported). Model 2 says rather little about whether online communication as such was associated with higher levels of affective polarization than offline communication as stipulated by Hypothesis 3. To get a better idea of the difference in affective polarization between the offline and online modes of political talk, offline and online discussions were modeled in concert in Model 3. The predicted value for “Online only” in Model 3 indicates that engagement in online communication of any type (consensual/crosscutting) was associated with marginally more polarized emotions (about 1%) than offline communication, but the difference is not statistically significant. Individuals who engaged in both offline and online communication had marginally less affectively polarized feelings (about 1%) compared to exclusive engagement in offline communication, but this difference is not statistically significant either. An additional model (unreported here) included a binary variable to separate respondents who participated in any online discussions from those who did not also produced no statistically significant results. All in all, contrary to the fears often expressed in popular debate and a strand of previous research (Sunstein, 2002; Pariser, 2011), the results yielded no statistical support for the notion that online discussions were associated with higher levels of affective polarization than were offline discussions. Thus, Hypothesis 3 gained no compelling support. Considering that only a fraction (1.7%) of the total sample engaged in political communication online exclusively, this indicates that online communication as such was an unlikely source of escalating affective polarization in the time leading up to the 2019 Spanish national election.
To check the robustness of these results, I conducted a host of extended analyses with various estimation techniques where various restrictions were imposed or loosened on the models (Appendix C).
The robustness checks revealed that the patterns were strikingly like the main models regardless of how the relationship was modeled or what estimation technique was being used. Additional tests included reproducing the findings using a GLS random-effects model and an ordinary least squares (OLS) model on the first observation with the lagged dependent variable to account for the panel structure of the data; alternative measurements of affective polarization; dropping the sample restrictions; re-categorizing the crosscutting network variable; and including fewer controls.
The models that leaned on Wagner's (2021) alternative measures based on the “mean distance from most liked to party” were the only fixed effects panel models that did not produce a significant difference between consensual and crosscutting discussions. Substantially, however, the level of affective polarization was still lower for these individuals than for partisans who only had consensual discussions. Conversely, the unweighted “spread of like and dislike” measure yielded somewhat stronger coefficients and larger differences between engagement in consensual and crosscutting communication, respectively. This shows that consensual discussions were more negatively correlated with supporters of smaller parties than they were with supporters of larger parties.
The models that only consider within-unit variation (fixed effects models) and the models that consider only between-unit variation (pooled OLS models), or both (GLS random-effects models) yielded similar results. The pooled OLS models produced somewhat stronger coefficients than the reported main models in Table 2, indicating that the difference between the respondents was greater than the difference over time for the same individual. Given the similarities across estimators and models, it can be concluded that crosscutting discussions were associated with lower levels of affective polarization than consensual discussions, but mainly in real-world discussion settings.
Finally, various interaction analyses were performed (unreported here) to determine whether other factors, such as perceived agreement or disagreement in the discussions, the combination of offline and online discussions, and the strength of partisan identity, could influence the relationship between crosscutting discussions and affective polarization. These models turned up no significant interactions.
All in all, none of the models yielded a conflicting result compared to the main models in Table 2, strengthening the internal validity of the results.
The study examined, both theoretically and empirically, the association between affective polarization and crosscutting communication in the offline and online modes of political talk. The study's most important conclusion was that, compared to face-to-face engagement in consensual discussions with co-partisans only, face-to-face crosscutting discussions in the months before the Spanish national election in 2019 were associated with a somewhat lower level of affective polarization. Crosscutting experiences offline were however associated with somewhat more political out-group dislike compared to having had no political discussions at all. There were no discernable differences between crosscutting and consensual experiences in the online mode of political talk. The results thus lend partial support to study Hypothesis 2b—crosscutting communication was associated with lower levels of out-group dislike than consensual communication and higher levels than the absence of political discussion, but only in IRL settings. Furthermore, the study found no evidence of clear changes in affective polarization depending on the mode of communication, that is, whether political discourse occurred offline or online. Thus, Hypothesis 3, which posited that polarization would be more pronounced in online compared to offline communication modes, found no compelling support. The descriptive part of the study further showed no signs of widespread political homophily in social networks—neither offline nor online. This is an important finding because it implies that (online) communication networks characterized by social homophily were probably not a major source of the surges in affective polarization during the 2018–2019 election year in Spain (Torcal and Comellas, 2022).
Regarding the limitations of the study and avenues for future research, first, it bears noting that the study was conducted in Spain at a particular point in time. From a comparative standpoint, the degree of mass polarization in Spain remains uncertain, but previous research suggests that the average level is likely medium to high (Gidron et al., 2020; Torcal and Comellas, 2022). What is clear, however, is that the time point at which the data were collected was one of the rising tensions and polarization in Spain. Partly because politics and political identities tend to be especially salient to voters during election years, and partly because the most prominent issue during the time—Spanish nationalism—tends to provoke polarization to a greater degree than many other political issues (Torcal and Comellas, 2022). In nations and settings where public debates are conducted in a high tone, it is plausible that network configuration and exposure to consonant and discordant communications, respectively, have a distinct effect. In other words, it is conceivable that the correlation between crosscutting communication and affective polarization has a contextual component. The results of the study should therefore be viewed considering this context. However, it can be argued that the timing of the study is favorable for the generalizability of the study results. The connection between political communication and affective polarization will likely manifest itself most clearly during times of heightened tensions. Since I found that crosscutting communication did not alarmingly foment affective polarization, it is unlikely that it would do so during less tense times. In the same way, it can be argued that any polarizing role of online communication should be the strongest exactly during polarized election years. Because no clear connection between online communication and affective polarization was found in this study, it is conceivable that it is limited also during less polarized times and contexts. The results should, however, be primarily generalizable to election years and contexts with rather high average levels of affective polarization to be on the safe side. However, future research should examine findings in light of various cultural and political situations and determine whether any trends have a time component. That is, whether the relationship between communication structures and polarization follows the ebb and flow of political cycles.
The panel as such provided exciting and relatively rare opportunities to test expectations about the relationship between communication patterns and affective polarization. The time frame of the panel, however, was rather short: respondents were interviewed just a couple of months apart. It is only logical that the variation for any given individual will be fairly limited over such a brief period. To gauge with more confidence whether different communication patterns result in (de) polarization in causal terms, future studies should aim at using exogenous variation and producing data spanning a longer period.
A further avenue for future research would be to link communication patterns to qualitative experiences of different types of discussion. Discussions that are being nasty or unpleasant may elicit a different reaction than discussions that are perceived as being polite and respectful. While interaction models (unreported here) that took the perceived level of agreement/disagreement of the discussions into account did not reveal a conditional effect, a closer look at the perceived deliberative norms of a given discussion—and the power dynamics they entail—is still important for understanding the mechanisms governing the results. Cognitive pre-dispositions such as personality and normative values are furthermore likely central to whether a person tends to feel angry, interested, frustrated, upset, or stimulated by contestation in debates. Different heterogeneous effects should thus be studied further, both quantitatively and qualitatively.
By analyzing and contrasting previous research's explicit and implicit assumptions regarding the potential impacts of crosscutting communication on affective polarization, the study as a whole provided a theoretical contribution. It has further made a novel empirical contribution by describing patterns of communication both offline and online and by demonstrating that engagement in crosscutting discussions was associated with relatively modest levels of affective polarization—contrary to the fears expressed in a strand of previous research (e.g., Taber and Lodge, 2006). Furthermore, the study discovered that online communication had no stronger correlation with affective polarization than offline communication. It also found no support for the idea of widespread political homophily in social networks, both offline and online. In the best-case scenario, these findings will transfer to other political and cultural contexts beyond Spain. If it does, perhaps we need not be so worried about pernicious polarization being the result of a pluralist world where opinions frequently clash in the physical and virtual worlds.
The original contributions presented in the study are included in the article/Supplementary material, further inquiries can be directed to the corresponding authors.
Ethical review and approval was not required for the study on human participants in accordance with the local legislation and institutional requirements. The patients/participants provided their written informed consent to participate in this study.
All authors listed have made a substantial, direct, and intellectual contribution to the work and approved it for publication.
Partial funding for publication was provided by the Uppsala University library.
The author wishes to thank the Editor of the article and two reviewers for constructive comments during the review process.
The author declares that the research was conducted in the absence of any commercial or financial relationships that could be construed as a potential conflict of interest.
All claims expressed in this article are solely those of the authors and do not necessarily represent those of their affiliated organizations, or those of the publisher, the editors and the reviewers. Any product that may be evaluated in this article, or claim that may be made by its manufacturer, is not guaranteed or endorsed by the publisher.
The Supplementary Material for this article can be found online at: https://www.frontiersin.org/articles/10.3389/fpos.2022.921188/full#supplementary-material
1. ^Completed interviews in wave 1: 2,501, wave 2: 1,890, wave 3: 1,659, and wave 4: 2,059 (tot. 8,109). Completion rate wave 1: 59.9%, wave 2: 78.1%, wave 3: 94.0%, wave 4: 88.6% (tot. 75.9%).
2. ^The within-unit variation for offline communication (see operationalization in 3.3) is 0.453 on a scale from 0–2 (m = 1.41), which is roughly half of the total variation of the variable. The corresponding variation for online communication is 0.49 (m = 0.58), which is just over half of the overall variation.
3. ^To further inform the choice of a fixed effects panel model, I ran a Breusch–Pagan LM test. This confirmed that a panel model was to be preferred over a pooled model, due to the interdependence between the observations arising from the panel structure of the data. A Hausman test further suggested that a fixed-effects model was to be preferred over a random-effects model due to the correlation between the covariates and the errors.
4. ^Wagner's measure of the affective distance from one's most liked party bears resemblance to the measure developed by Reiljan (2020) but is specifically developed to measure individual-level affective polarization rather than aggregate levels of affective polarization. It is furthermore unrestricted to the inclusion of respondents who expressed positive partisanship, which is a way to avoid skewing the sample and to use more within-unit variation.
5. ^One thing about the measure of crosscutting and consensual communication bears noting First, the items admittedly do not allow me to measure discussion per se, but rather respondents' memory or perception of communication, reported in responses to several survey items. Observational studies that reproduce past events or abstract phenomena are never flawless reflections of reality. Every observation is filtered via the perspectives of the people who are making it. However, I could still make general conclusions regarding typical correlations in the Spanish community of everyday residents thanks to the survey data, which also gave me a sense of the communication patterns people use in their daily lives. Indeed, Klofstad et al. (2013) have invited us to consider the idea that perceptions about political talk are more consequential to the development of political behavior than the objective reality is.
6. ^Time invariable variables like gender and ethnicity are automatically accounted for by the individual fixed effects.
7. ^For scales, see Appendix A: Summary statistics.
8. ^The coefficients can be interpreted in percentages because all variables were normalized to range between 0 and 1.
Achen, C. H. (2000). Why Lagged Dependent Variables Can Suppress the Explanatory Power of Other Independent Variables. Paper presented at the Annual Meeting of the American Political Science Association.
Allison, P. D. (2015). Don't Put Lagged Dependent Variables in Mixed Models | Statistical Horizons. Available online at: https://statisticalhorizons.com/lagged-dependent-variables (accessed June 17, 2022).
Anderson, A. A., Brossard, D., Scheufele, D. A., Xenos, M. A., and Ladwig, P. (2014). The “Nasty Effect:” online incivility and risk perceptions of emerging technologies. J. Comput. Mediated Commun. 19, 373–387. doi: 10.1111/jcc4.12009
Angrist, J. D., and Pischke, J.-S. (2008). Mostly Harmless Econometrics: An Empiricist's Companion. Princeton University Press.
Baek, Y. M., Wojcieszak, M., and Delli Carpini, M. X. (2012). Online versus face-to-face deliberation: Who? Why? What? With what effects? New Media Soc. 14, 363–383. doi: 10.1177/1461444811413191
Bail, C. A., Argyle, L. P., Brown, T. W., Bumpus, J. P., Chen, H., Hunzaker, M. B. F., et al. (2018). Exposure to opposing views on social media can increase political polarization. Proc. Nat. Acad. Sci. U.S.A 115, 9216–9221. doi: 10.1073/pnas.1804840115
Barabas, J. (2004). How deliberation affects policy opinions. Am. Polit. Sci. Rev. 98, 687–701. doi: 10.1017/S0003055404041425
Barberá, P. (2015). How Social Media Reduces Mass Political Polarization. Evidence from Germany, Spain, and the U.S. Working Paper. Available online at: http://pablobarbera.com/static/barbera_polarization_APSA.pdf (accessed June 17, 2022).
Barberá, P., Jost, J. T., Jonathan, N, Tucker, J. A., and Richard, B. (2015). Tweeting from left to right: is online political communication more than an echo chamber? Psychol. Sci. 26, 1531–1542. doi: 10.1177/0956797615594620
Barnidge, M. (2017). Exposure to political disagreement in social media versus face-to-face and anonymous online settings. Polit. Commun. 34, 302–321. doi: 10.1080/10584609.2016.1235639
Beam, M., Hutchens, M., and Hmielowski, J. (2018). Facebook news and (de)polarization: reinforcing spirals in the 2016 US election. Inf. Commun. Soc. 21, 940–958. doi: 10.1080/1369118X.2018.1444783
Beck, N., and Katz, J. N. (2011). Modeling dynamics in time-series–cross-section political economy data. Ann. Rev. Polit. Sci. 14, 331–352. doi: 10.1146/annurev-polisci-071510-103222
Bell, A., Fairbrother, M., and Jones, K. (2018). Fixed and random effects models: making an informed choice. Qual. Quant. 53, 1051–1074. doi: 10.1007/s11135-018-0802-x
Berry, J. M., and Sobieraj, S. (2013). The Outrage Industry: Political Opinion Media and the New Incivility. Oxford: Oxford University Press.
Binder, A. R., Dalrymple, K. E., Brossard, D., and Scheufele, D. A. (2009). The soul of a polarized democracy: testing theoretical linkages between talk and attitude extremity during the 2004 presidential election. Commun. Res., 36, 315–340. doi: 10.1177/0093650209333023
Borah, P. (2014). Does it matter where you read the news story? Interaction of incivility and news frames in the political blogosphere. Commun. Res. 41, 809–827. doi: 10.1177/0093650212449353
Boxell, L., Gentzkow, M., and Shapiro, J. M. (2022). Cross-country trends in affective polarization. Rev. Econ. Statist. doi: 10.1162/rest_a_01160
Cappella, J. N., Price, V., and Nir, L. (2002). Argument repertoire as a reliable and valid measure of opinion quality: electronic dialogue during campaign 2000. Polit. Commun. 19, 73–93. doi: 10.1080/105846002317246498
Chen, G. M., and Lu, S. (2017). Online political discourse: exploring differences in effects of civil and uncivil disagreement in news website comments. J. Broadcast. Electronic Media 61, 108–125. doi: 10.1080/08838151.2016.1273922
Dahlgren, P. (2021). A critical review of filter bubbles and a comparison with selective exposure. Nordicom Rev. 42, 15–33. doi: 10.2478/nor-2021-0002
Del Vicario, M., Vivaldo, G., Bessi, A., Zollo, F., Scala, A., Caldarelli, G., et al. (2016). Echo chambers: emotional contagion and group polarization on facebook. Sci. Rep. 6:37825. doi: 10.1038/srep37825
Dinesen, P., Schaeffer, M., and Sønderskov, K. (2019). Ethnic diversity and social trust: a narrative and meta-analytical review. Ann. Rev. Polit. Sci. 23, 441–465. doi: 10.1146/annurev-polisci-052918-020708
Dovidio, J., Love, A., Schellhaas, F., and Hewstone, M. (2017). Reducing intergroup bias through intergroup contact: twenty years of progress and future directions. Group Process. Intergr. Relat. 20:136843021771205. doi: 10.1177/1368430217712052
Druckman, J. N., Klar, S., Krupnikov, Y., Levendusky, M., and Ryan, J. B. (2021). Affective polarization, local contexts and public opinion in America. Nat. Hum. Behav. 5, 28–38. doi: 10.1038/s41562-020-01012-5
Druckman, J. N., and Levendusky, M. S. (2019). What do we measure when we measure affective polarization? Public Opin. Q. 83, 114–122. doi: 10.1093/poq/nfz003
Druckman, J. N., and McGrath, M. C. (2019). The evidence for motivated reasoning in climate change preference formation. Nat. Clim. Chang. 9, 111–119. doi: 10.1038/s41558-018-0360-1
Dubois, E., and Blank, G. (2018). The echo chamber is overstated: the moderating effect of political interest and diverse media. Inf. Commun. Soc. 21, 729–745. doi: 10.1080/1369118X.2018.1428656
Eveland, W. P., Appiah, O., and Beck, P. A. (2018). Americans are more exposed to difference than we think: capturing hidden exposure to political and racial difference. Soc. Netw. 52, 192–200. doi: 10.1016/j.socnet.2017.08.002
Farrar, C., Green, D. P., Green, J. E., Nickerson, D. W., and Shewfelt, S. (2009). Does discussion group composition affect policy preferences? Results form three randomized experiments. Polit. Psychol. 615–647. doi: 10.1111/j.1467-9221.2009.00717.x
Festinger, L. (1954). A theory of social comparison processes. Hum. Relat. 7, 117–140. doi: 10.1177/001872675400700202
Fletcher, R., Cornia, A., and Nielsen, R. K. (2020). How polarized are online and offline news audiences? A comparative analysis of twelve countries. Int. J. Press/Politics 25, 169–195. doi: 10.1177/1940161219892768
Garrett, R. K., Gvirsman, S. D., Johnson, B. K., Tsfati, Y., Neo, R., and Dal, A. (2014). Implications of pro- and counterattitudinal information exposure for affective polarization: Partisan media exposure and affective polarization. Hum. Commun. Res. 40, 309–332. doi: 10.1111/hcre.12028
Gentzkow, M., and Shapiro, J. M. (2010). What drives media slant? Evidence from U.S. daily newspapers. Econometrica 78, 35–71. doi: 10.3982/ECTA7195
Gidron, N., Adams, J., and Horne, W. (2020). American Affective Polarization in Comparative Perspective. Cambridge: Cambridge University Press. doi: 10.1017/9781108914123
Grönlund, K., Herne, K., and Setälä, M. (2015). Does enclave deliberation polarize opinions? Polit. Behav. 37, 995–1020. doi: 10.1007/s11109-015-9304-x
Guess, A., and Coppock, A. (2020). Does counter-attitudinal information cause backlash? Results from three large survey experiments. Br. J. Polit Sci. 50, 1497–1515. doi: 10.1017/S0007123418000327
Habermas, J. (1989). The Structural Transformation of the Public Sphere: An Inquiry into a Category of Bourgeois Society. Cambridge: Polity Press.
Harteveld, E. (2021). Ticking all the boxes? A comparative study of social sorting and affective polarization. Electoral Stud. 72:102337. doi: 10.1016/j.electstud.2021.102337
Himmelroos, S., and Christensen, H. S. (2020). The potential of deliberative reasoning: patterns of attitude change and consistency in cross-cutting and like-minded deliberation. Acta Polit. 55, 135–155. doi: 10.1057/s41269-018-0103-3
Hogg, M. A. (2018). “Chapter 5 social identity theory,” in Contemporary Social Psychological Theories: Second Edition, ed Burke PJ (Redwood City, CA: Stanford University Press). pp. 112–138. doi: 10.1515/9781503605626-007
Huddy, L. (2001). From social to political identity: a critical examination of social identity theory. Polit. Psychol. 22, 127–156. doi: 10.1111/0162-895X.00230
Isenberg, D. J. (1986). Group polarization: a critical review and meta-analysis. J. Pers. Soc. Psychol. 50, 1141–1151. doi: 10.1037/0022-3514.50.6.1141
Iyengar, S., Lelkes, Y., Levendusky, M., Malhotra, N., and Westwood, S. J. (2019). The origins and consequences of affective polarization in the United States. Ann. Rev. Polit. Sci. 22, 129–146. doi: 10.1146/annurev-polisci-051117-073034
Iyengar, S., Sood, G., and Lelkes, Y. (2012). Affect, not ideology. Public Opin. Q. 76, 405–431. doi: 10.1093/poq/nfs038
Iyengar, S., and Westwood, S. J. (2015). Fear and loathing across party lines: new evidence on group polarization: fear and loathing across party lines. Am. J. Pol. Sci. 59, 690–707. doi: 10.1111/ajps.12152
Jeynes, W. H. (2002). The challenge of controlling for SES in social science and education research. Educ. Psychol. Rev. 14, 205–221. doi: 10.1023/A:1014678822410
Karlsen, R., Steen-Johnsen, K., Wollebæk, D., and Enjolras, B. (2017). Echo chamber and trench warfare dynamics in online debates. Eur. J. Commun. 32, 257–273. doi: 10.1177/0267323117695734
Kim, Y. (2015). Does disagreement mitigate polarization? How selective exposure and disagreement affect political polarization. J. Mass Commun. Q. 92, 915–937. doi: 10.1177/1077699015596328
Kim, Y. (2019). How cross-cutting news exposure relates to candidate issue stance knowledge, political polarization, and participation: the moderating role of political sophistication. Int. J. Public Opin. Res. 31, 626–648. doi: 10.1093/ijpor/edy032
Klofstad, C. A., Sokhey, A. E., and McClurg, S. D. (2013). Disagreeing about disagreement: how conflict in social networks affects political behavior. Am. J. Pol. Sci. 57, 120–134. doi: 10.1111/j.1540-5907.2012.00620.x
Kubin, E., and von Sikorski, C. (2021). The role of (social) media in political polarization: a systematic review. Ann. Int. Commun. Assoc. 45, 188–206. doi: 10.1080/23808985.2021.1976070
Kunda, Z. (1990). The case for motivated reasoning. Psychol. Bull. 108, 480–498. doi: 10.1037/0033-2909.108.3.480
Lee, H., Kwak, N., and Campbell, S. W. (2015). Hearing the other side revisited: the joint workings of cross-cutting discussion and strong tie homogeneity in facilitating deliberative and participatory democracy. Commun. Res. 42, 569–596. doi: 10.1177/0093650213483824
Lelkes, Y., Sood, G., and Iyengar, S. (2017). The hostile audience: the effect of access to broadband internet on partisan affect. Am. J. Pol. Sci. 61, 5–20. doi: 10.1111/ajps.12237
Lemmer, G., and Wagner, U. (2015). Can we really reduce ethnic prejudice outside the lab? A meta-analysis of direct and indirect contact interventions: meta-analysis of contact interventions. Eur. J. Soc. Psychol. 45, 152–168. doi: 10.1002/ejsp.2079
Levendusky, M, and Stecula, D. (2021). “We need to talk: how cross-party dialogue reduces affective polarization,” in Elements in Experimental Political Science, ed Druckman J (Cambridge: Cambridge University Press), doi: 10.1017/9781009042192
Levitan, L. C., and Visser, P. S. (2008). The impact of the social context on resistance to persuasion: effortful versus effortless responses to counter-attitudinal information. J. Exp. Soc. Psychol. 44, 640–649. doi: 10.1016/j.jesp.2007.03.004
Lieberman, A., and Schroeder, J. (2020). Two social lives: how differences between online and offline interaction influence social outcomes. Curr. Opin. Psychol. 31, 16–21. doi: 10.1016/j.copsyc.2019.06.022
Little, A. T. (2019). The distortion of related beliefs. Am. J. Pol. Sci. 63, 675–689. doi: 10.1111/ajps.12435
Lorenzo-Rodríguez, J., and Torcal, M. (2022). Twitter and affective polarisation: following political leaders in Spain. South Eur. Soc. Polit. doi: 10.1080/13608746.2022.2047554
Lyons, B. A., and Veenstra, A. S. (2016). How (Not) to talk on twitter: effects of politicians' tweets on perceptions of the twitter environment. Cyberpsychol. Behav. Soc. Netw. 19, 8–15. doi: 10.1089/cyber.2015.0319
Manin, B. (1987). On legitimacy and political deliberation. Polit. Theory 15, 338–368. doi: 10.1177/0090591787015003005
Marcus, G. E., Neuman, W. R., and MacKuen, M. (2000). Affective Intelligence and Political Judgment. Chicago, IL: University of Chicago Press.
Mason, L. (2015). “I Disrespectfully Agree”: the differential effects of partisan sorting on social and issue polarization. Am. J. Pol. Sci. 59, 128–145. doi: 10.1111/ajps.12089
Mason, L. (2016). A cross-cutting calm: how social sorting drives affective polarization. Public Opin. Q. 80, 351–377. doi: 10.1093/poq/nfw001
Matthes, J., Knoll, J., Valenzuela, S., Hopmann, D. N., and Sikorski, C. V. (2019). A Meta-Analysis of the effects of cross-cutting exposure on political participation. Polit. Commun. 36, 523–542. doi: 10.1080/10584609.2019.1619638
McCoy, J., Rahman, T., and Somer, M. (2018). Polarization and the global crisis of democracy: common patterns, dynamics, and pernicious consequences for democratic polities. Am. Behav. Sci. 62, 16–42. doi: 10.1177/0002764218759576
Mercier, H., and Landemore, H. (2012). Reasoning is for arguing: understanding the successes and failures of deliberation. Polit. Psychol. 33, 243–258. doi: 10.1111/j.1467-9221.2012.00873.x
Mutz, D. C. (2002). Cross-cutting social networks: testing democratic theory in practice. Am. Polit. Sci. Rev. 96, 111–126. doi: 10.1017/S0003055402004264
Mutz, D. C. (2006). Hearing the Other Side: Deliberative Versus Participatory Democracy. Cambridge: Cambridge University Press.
Nir, L. (2005). Ambivalent social networks and their consequences for participation. Int. J. Public Opin. Res. 17, 422–442. doi: 10.1093/ijpor/edh069
Nordbrandt, M. (2021a). Do cross-cutting discussions enhance pro-environmental attitudes? Testing green deliberative theory in practice. Environ. Polit. 30, 326–356. doi: 10.1080/09644016.2020.1787063
Nordbrandt, M. (2021b). Affective polarization in the digital age: testing the direction of the relationship between social media and users' feelings for out-group parties. New Media Soc. doi: 10.1177/14614448211044393
O'Hara, K., and Stevens, D. (2015). Echo chambers and online radicalism: assessing the internet's complicity in violent extremism. Policy Internet 7, 401–422. doi: 10.1002/poi3.88
Padró-Solanet, A., and Balcells, J. (2022). Media diet and polarisation: evidence from Spain. South Eur. Soc. Polit. doi: 10.1080/13608746.2022.2046400
Paluck, E. L., Green, S. A., and Green, D. P. (2019). The contact hypothesis re-evaluated. Behav. Public Policy 3, 129–158. doi: 10.1017/bpp.2018.25
Pariser, E. (2011). The Filter Bubble: What the Internet is Hiding From You. Penguin UK. doi: 10.3139/9783446431164
Parsons, B. M. (2010). Social networks and the affective impact of political disagreement. Polit. Behav. 32, 181–204. doi: 10.1007/s11109-009-9100-6
Pettigrew, T., Tropp, L., Wagner, U., and Christ, O. (2011). Recent advances in intergroup contact theory. Int. J. Intercult. Relat. 35, 271–280. doi: 10.1016/j.ijintrel.2011.03.001
Pew Research Center (2020). 64% of Americans Say Social Media Have a Mostly Negative Effect on the Way Things Are Going in the U.S. Today. Available online at: https://pewrsr.ch/3dsV7uR (accessed June 17, 2022).
Price, V., Cappella, J. N., and Nir, L. (2002). Does disagreement contribute to more deliberative opinion? Polit. Commun. 19, 95–112. doi: 10.1080/105846002317246506
Prior, M. (2013). Media and political polarization. Ann. Rev. Polit. Sci. 16, 101–127. doi: 10.1146/annurev-polisci-100711-135242
Reiljan, A. (2020). ‘Fear and loathing across party lines' (also) in Europe: affective polarisation in European party systems. Eur. J. Polit. Res. 59, 376–396. doi: 10.1111/1475-6765.12351
Rogowski, J. C., and Sutherland, J. L. (2016). How ideology fuels affective polarization. Polit. Behav. 38, 485–508. doi: 10.1007/s11109-015-9323-7
Rowe, I. (2015). Civility 2.0: a comparative analysis of incivility in online political discussion. Inf. Commun. Soc. 18, 121–138. doi: 10.1080/1369118X.2014.940365
Santoro, E., and Broockman, D. (2022). The promise and pitfalls of cross-partisan conversations for reducing affective polarization: evidence from randomized experiments. Sci. Adv. 8:eabn5515. doi: 10.1126/sciadv.abn5515
Sood, G., and Iyengar, S. (2016). Coming to dislike your opponents: the polarizing impact of political campaigns. SSRN Electronic J. doi: 10.2139/ssrn.2840225
Spivak, G. C. (1985). The rani of Sirmur: an essay in reading the archives. Hist. Theory 24, 247–272. doi: 10.2307/2505169
Strandberg, K., Himmelroos, S., and Grönlund, K. (2019). Do discussions in like-minded groups necessarily lead to more extreme opinions? Deliberative democracy and group polarization. Int. Polit. Sci. Rev. 40, 41–57. doi: 10.1177/0192512117692136
Sunstein, C. R. (2002). The law of group polarization. J. Polit. Philos. 10, 175–195. doi: 10.1111/1467-9760.00148
Sunstein, C. R. (2018). Republic: Divided Democracy in the Age of Social Media. Princeton, NJ: Princeton University Press.
Taber, C. S., and Lodge, M. (2006). Motivated skepticism in the evaluation of political beliefs. Am. J. Pol. Sci. 50, 755–769. doi: 10.1111/j.1540-5907.2006.00214.x
Tajfel, H., and Turner, J. C. (1979). “An integrative theory of intergroup conflict,” in The Social Psychology of Intergroup Relations, eds W. G. Austin, and S. Worchel (Monterey, CA: Brooks/Cole), 33–37.
Theocharis, Y., Barberá, P., Fazekas, Z., and Popa, S. A. (2020). The dynamics of political incivility on twitter. SAGE Open 10:2158244020919447. doi: 10.1177/2158244020919447
Torcal, M., and Carty, E. (2022). Partisan sentiments and political trust: a longitudinal study of Spain. South Eur. Soc. Polit. doi: 10.1080/13608746.2022.2047555
Torcal, M., and Comellas, J. M. (2022). Affective polarisation in times of political instability and conflict. Spain from a comparative perspective. South Eur. Soc. Polit. doi: 10.1080/13608746.2022.2044236
Torcal, M., Santana, A., Lorenzo, J., Carty, E., Revilla, M., Riera, P., et al. (2020). Political and affective polarisation in a democracy in crisis: the e-dem panel survey dataset (Spain, 2018-2019). Data Brief 32:106059. doi: 10.1016/j.dib.2020.106059
Tropp, L., and Pettigrew, T. (2006). Relationships between intergroup contact and prejudice among minority and majority status groups. Psychol. Sci. 16, 951–957. doi: 10.1111/j.1467-9280.2005.01643.x
Tucker, J., Guess, A., Barbera, P., Vaccari, C., Siegel, A., Sanovich, S., et al. (2018). Social media, political polarization, and political disinformation: a review of the scientific literature. SSRN Electronic J. doi: 10.2139/ssrn.3144139
Wagner, M. (2021). Affective polarization in multiparty systems. Elect. Stud. 69:102199. doi: 10.31219/osf.io/j7d4t
Wojcieszak, M., and Price, V. (2010). Bridging the divide or intensifying the conflict? How disagreement affects strong predilections about sexual minorities. Polit. Psychol. 31, 315–339. doi: 10.1111/j.1467-9221.2009.00753.x
Wojcieszak, M., Winter, S., and Yu, X. (2020). Social norms and selectivity: effects of norms of open-mindedness on content selection and affective polarization. Mass Commun. Soc. 23, 455–483. doi: 10.1080/15205436.2020.1714663
Keywords: affective polarization, offline, online, social media, social identity, panel data, crosscutting communication
Citation: Nordbrandt M (2022) Affective polarization in crosscutting communication networks: Offline and online evidence from Spain. Front. Polit. Sci. 4:921188. doi: 10.3389/fpos.2022.921188
Received: 15 April 2022; Accepted: 05 August 2022;
Published: 07 September 2022.
Edited by:
Mariano Torcal, Pompeu Fabra University, SpainReviewed by:
Eric Guntermann, University of California, Berkeley, United StatesCopyright © 2022 Nordbrandt. This is an open-access article distributed under the terms of the Creative Commons Attribution License (CC BY). The use, distribution or reproduction in other forums is permitted, provided the original author(s) and the copyright owner(s) are credited and that the original publication in this journal is cited, in accordance with accepted academic practice. No use, distribution or reproduction is permitted which does not comply with these terms.
*Correspondence: Maria Nordbrandt, bWFyaWEubm9yZGJyYW5kdEBzdGF0c3ZldC51dS5zZQ==
Disclaimer: All claims expressed in this article are solely those of the authors and do not necessarily represent those of their affiliated organizations, or those of the publisher, the editors and the reviewers. Any product that may be evaluated in this article or claim that may be made by its manufacturer is not guaranteed or endorsed by the publisher.
Research integrity at Frontiers
Learn more about the work of our research integrity team to safeguard the quality of each article we publish.