- School of Journalism and Communication, Peking University, Beijing, China
The COVID-19 pandemic was accompanied by an infodemic, which has now become a global concern. Despite the relatively timely and extensive guidelines regarding COVID-19 prevention and treatment, effective and standardized solutions for managing this infodemic are still lacking. In light of the ubiquity of social media in China, various algorithms have been applied to new media platforms to help combat COVID-19, particularly, misinformation and disinformation. Inspired by the model of ‘blocking the spread of the virus, treating the infected population, and improving immunity’ for the prevention and control of the COVID-19 pandemic, this study examines three dominant forms of algorithms—collaborative filtering recommendation, content-based recommendation, and knowledge-based recommendation—and proposes a theoretical model called Block, Push, and Intervene (BPI). This model calls for the timely blocking of misinformation and disinformation, precisely delivering authentic information to people affected by the infodemic and intervening in some potential issues in advance. Based on the BPI framework, we conducted semi-structured interviews with relevant staffs in charge of Bytedance, Tencent, Sina Weibo, Baidu, and The National Internet Information Office's Center for Reporting Illegal and Adverse Information, to summarize the patterns of algorithms used against the infodemic. Additionally, an online panel survey is used to analyze public perceptions of the severity of the infodemic on each platform. By evaluating the cross-validated results of the survey sample and semi-structured interviews on the role of algorithms against infodemic, this study contributes both to the understanding of the working details and practices surrounding information epidemics in the context of China, as well as to the systematic research on the unique use of information technology in the midst of public health crises.
Introduction
The COVID-19 pandemic broke out in Wuhan, China sometime between the end of 2019 and the beginning of 2020. The high transmissibility of this novel strain and the lack of specific medicines over such a short period both contributed to the acceleration of its spread. On January 21, the National Health Commission of China issued the first daily report of the pandemic, and it took merely 10 days (January 21-January 31, 2020) for the number of total confirmed cases to surge from 291 to 11,791. Meanwhile, the disease also began exhibiting a trend of multiple outbreaks, with countries such as Italy, the United Kingdom, and Japan witnessing a large-scale increase in the number of confirmed cases. On March 21, 2020, the World Health Organization (hereinafter referred to as WHO) identified this COVID-19 epidemic as a global pandemic. As one of the most globalized countries—the United States—experienced a large-scale outbreak of the disease, the number of confirmed cases began to rise worldwide.
Given its seriousness, the digital space and Internet platforms are flooded with information about the pandemic, some of which is science-based, whilst some is false information or are deliberately fabricated rumors. In the Internet age, the perceptions of the general public, who face the risk of infection and disruption of their normal lives, can be greatly impacted by such misinformation. Regarding the prevention and control of the COVID-19 pandemic, all walks of life have reached a consensus. However, the problems associated with it in terms of information have not received widespread attention. The impact of the infodemic on the physical and mental health of the public could also hinder the prevention and control of the disease, which would result in the failure to fully implement appropriate precautionary measures. Therefore, the management of the Infodemic has practical significance for tackling the COVID-19 pandemic and the improvement of the physical and mental health of citizens, which is the primary focus of this research.
Literature Review
Although scientists in China have studied the infodemic, the body of foreign research dates further back and is more comprehensive. Global research on the infodemic can be divided into five categories: the definition of an infodemic, its specific manifestations, its impact mechanism, its formation mechanism, and the potential responses to it. Chinese research primarily provides an overview of the infodemic and the case analysis of the infodemic.
When the SARS pandemic broke out in 2003, Rothkopf (2003) first proposed the concept of the “Infodemic,” which is a combination of the two terms, “information” and “epidemic.” The WHO (2020) defines an infodemic as an overload of information (some correct and some incorrect), which makes it difficult for people to find trustworthy sources of information and guidance to rely on, and may even harm people's health. The two major manifestations of an infodemic are misinformation and disinformation. The former emphasizes that errors in information are unintentional, while the latter is misinformation deliberately disseminated by users for various purposes.
The specific manifestations of the infodemic in the research community can be divided into the performance of the information itself and the performance of the public after it is affected.
For the former, researchers have typically adopted two research methods—social network analysis and content analysis. Gruzd and Mai (2020) analyzed a typical case of the infodemic by using the spread of rumors on Twitter—claiming that “the COVID-19 pandemic does not exist”— as an example. They concluded that in this infodemic case, the main promoters were supporters of former US President Donald Trump; however, its proliferation was international, as similar remarks had also appeared on a large scale in Brazil. Gruzd and Mai's research introduced social robots into the research horizon of the infodemic. Similarly, Chong (2020) analyzed the case of the infodemic in South Korea, and the study found that the network community aggregates play an important role in the spread of the infodemic. Further, Han et al. (2021) used a cross-sectional study that revealed that exposure to the COVID-19 infodemic had a direct effect on the reduction of COVID-19-related knowledge and personal preventive health intentions with the mediated effect of perceived self-efficacy, severity, and vulnerability. Although the interactive relations among the behavioral variables were not analyzed, it explained the effect of infodemic in a more comprehensive way.
The above-mentioned manifestations of the infodemic have had a public impact through connections with Internet users. In terms of public influence, Cheng et al. (2020) studied its impact on different groups in terms of anxiety and sleep using surveys. The study found that audiences consuming information in different ways have significant differences in anxiety and sleep disorders. This suggested that the type of audience should be taken into consideration in the management of an infodemic. Hence, it is necessary to adopt differing methods of refuting rumors and information dissemination for different types of audiences.
In a study on the formation mechanism of the Infodemic, Grimes (2020) endows false information with a viral nature. He argues that vague statements about the truth often go viral easily on the Internet, and each of us is a rumor carrier. This argument closely links the spreading mechanism of the Infodemic with that of the COVID-19 pandemic, and provides a familiar concept reference for understanding the Infodemic. Moreover, (Fang, 2020) suggests that the double loss of information order at the top-down and bottom-up levels is the root cause of the worsening of the “epidemic of trust.” This understanding helps researchers to understand the Infodemic from the perspective of disorder. Taking the realities into account, Zubek et al. (2021) discovered that constantly using the Internet and reading reports on COVID-19 cases and deaths could affect mood fluctuations. Their social experiment emphasizes the role of Internet use in daily routines of the modern public in the spread of the infodemic.
Several scholars have borrowed existing theoretical models for analysis of the impact mechanism of the infodemic. Greenspan and Loftus (2020) analyze why the infodemic has a profound negative impact on the public and the influence of audience memory. This research treats the infodemic more as a continuous process, with each stage foreshadowing the nest, leading to the exponential increase of the infodemic in the absence of governance. Agarwal and Alsaeedi (2020) use the information behavior framework to analyze the mechanism of the impact of the infodemic on the public. Their research incorporates the production, consumption, and audience perception of information into the research scope of the Infodemic, and provides a reference for research perspectives to determine appropriate response strategies.
In November 2020, the WHO issued the first edition of the infodemic management training handbook, which described how medical practitioners and pandemic prevention and control personnel should respond to the infodemic from the perspective of risk communication and rumor management. However, the handbook does not propose an overall action framework for reference. It is primarily a summary of existing research in the academic world, and is strongly scientific and educational in nature.
In the academic sector, the most representative study is Yang et al. (2020), that discusses the possible role of information and communication technology (ICT) in the management of infodemic in the form of a review. Harris (2020) approaches the issue of how to use laws to regulate the infodemic from the perspective of jurisprudence, and analyzes the trade-offs between managing the infodemic and maintaining freedom of speech.
Research on the potential approaches to infodemic can be divided into two categories—case analysis and strategy suggestions.
Myers (2021) suggests that greater integration of the public into the public health information system could be valuable in terms of diminishing the threat of mis/disinformation. Although there remains a lack of empirical research on the effects of such a method, this study demonstrates how such an approach could build a wall against the infodemic. As Italy was the first western nation to be affected by the COVID-19 pandemic, Di Mascio et al. (2021) focuses on the Italian case, where independent regulatory agencies were able to fight against the infodemic based on their expertise in the sector of public health. Zheng (2020) uses four social media platforms as examples, namely Toutiao, Weibo, Tencent News, and Dingxiang Doctor to illustrate the number of rumors released and read on these four platforms in the early stages of the pandemic. A study by Chen (2021) looks at five major Chinese anti-rumor platforms—China Internet Joint Rumor-Refusal Platform, Tencent Jiaozhen, Weibo Anti-rumor, Baidu Anti-Rumor, and Toutiao Anti-rumor—and analyzes the relationship between the volume and geographic reach of their anti-rumor information. The choice of platforms is useful in the context of this research. These five main bodies of research have essentially constructed the major components of the Chinese Internet infodemic management system.
In the context of strategy, scholars in the fields of journalism and communication and information management have contributed largely macro-level suggestions. Ding (2020) proposes that artificial intelligence technology can be applied to pandemic information management, and Fang et al. (2020) emphasizes the main responsibilities of the platform and the importance of cooperation of multiple subjects from the perspective of social governance. Scholars in the field of information science have provided a reference for algorithm innovation in response to the infodemic from the perspective of algorithms. Liu and Huang (2021) propose a pandemic rumor recognition algorithm based on text augmentation and generative adversarial networks (GAN) method.
The WHO's ‘COVID-19 Situation Report-100’ mentions the WHO's EPI-WIN framework, which collects global digital media usage data every week to predict potential risks. However, it has not incorporated more Infodemic management subjects into the framework, and has not yet been widely used in actual global Infodemic management.
At present, research on algorithms and information dissemination can be divided into two main categories—research on the operating mechanism of algorithms in the field of information dissemination, and research on the social influence of algorithms participating in information dissemination.
In case of the former, the study by Hailing et al. (2009) is the most representative and is of great relevance to this research. It classifies Internet recommendation systems in detail, and divides the existing recommendation algorithms into four categories—content-based recommendation, collaborative filtering recommendation, knowledge-based recommendation and combined recommendation. The first three can be applied to each link of information dissemination, while the combined recommendation algorithm is a combination of these three recommendation methods. In a sense, it is holistic thinking that runs through the entire process of information dissemination and even infodemic management.
In case of the latter, Shin and Valente (2020) analyze vaccine hesitation and study the relationship between algorithm recommendations on the Amazon platform and the frequency of vaccine hesitation-related books. The novelty of this research pertains to the connection between the awareness and diffusion of vaccine hesitation among the public and the recommendation ranking of algorithms. This study inspired scholars to pay attention to whether the Internet platform effectively interferes with the vaccine hesitation problem in the middle and late stages of a pandemic.
To study audience influence, Cui and Wu (2019) conducted a survey on the usage of Toutiao users and found that the algorithm-driven platform can effectively increase users' knowledge of public affairs and soft news. They also explored the role of gender as a mediation effect. The first finding of the research suggests that an algorithm-driven content distribution platform similar to Toutiao can participate in the management of an infodemic by disseminating accurate knowledge of public affairs and suppressing the generation of false perceptions.
By reviewing the literature in the two major areas of infodemic and algorithms, it was observed that the current research in the field of infodemic management is quite extensive. Scholars have a relatively deep understanding of the definition, source, and development history of infodemic. In terms of response strategies to the Infodemic 3, most studies are either lacking in depth or are driven by a single case, leading to inadequate systematic understanding of appropriate response strategies. Against the backdrop of this infodemic that is still spreading in many countries, it is of great practical significance to use Chinese experience as a model to summarize such response strategies, especially in the era of intelligent communication.
Methods and Materials
As a large-scale infodemic occurs in the Internet space, the government, the Internet platforms, and other entities have their own responsibilities for the identification and processing of information. As artificial intelligence algorithms have become an important technical tool for Internet platforms, the core research questions of this study are as follows.
Research Question 1: During the COVID-19 pandemic, how was the Chinese Infodemic prevention and control system developed?
Research Question 2: How do algorithms play a role in this prevention and control system?
Since the academic community has not yet formed a preliminary understanding of the Chinese response to the Infodemic, it is imperative to describe its basic framework and background. This study proposes a Block, Push and Intervene (BPI) model and compares the severity of infodemic in specific pandemic stages in China and other countries, so as to determine Chinese achievements in global infodemic governance. Furthermore, this article analyzes the components of the Chinese infodemic prevention and control system, as well as users' perceptions.
Because the proposed research questions are relatively complex and involve multiple subjects, and the academic community has not yet formed a more scientific research framework or model, this study attempts to propose theoretical models for reference based on the characteristics of the COVID-19 pandemic, the infodemic, and the algorithms. Using a mixed-method approach, this study integrates in-depth interviews and surveys to demonstrate theoretical models and conduct a comprehensive analysis of the basic modes of Chinese response to the infodemic.
In the in-depth interview, this study refers to the five research objects selected by Chen (2021). However, considering the similarity of Baidu and Toutiao's software functions and the lack of the Baidu platform's own secondary dissemination function, the Central Cyberspace Administration of Chinese Illegal and Bad Information Reporting Center (the organization of the China Internet Joint Rumor Refusal Platform), Tencent Jiaozhen, Sina Weibo and Toutiao are chosen as the main subjects. The respondents consist of those responsible for the anti-rumor works of the four main bodies.
Since there is no complete and clear framework for understanding the issue of infodemic governance, this study selects the semi-structured interviews approach. The aim is to determine the status of each subject's participation in Infodemic governance under the existing cognitive framework, and to obtain relevant information regarding their participation. Each interview consists of approximately 12 topics, and the average interview time is 45 min. The interview questions revolve around two major themes—the working mode of participating in infodemic governance during the COVID-19 pandemic, and the role and operating mechanism of algorithms in infodemic governance. From 2 March to 28 March 2021, interviews were conducted with the four selected respondents.
In order to gain a deeper understanding of the differences in user perceptions caused by the differences in the infodemic response measures of various platforms, a questionnaire survey regarding Tencent, Sina Weibo, and Toutiao is conducted to investigate the perceptions of users on the infodemic when using these platforms during the pandemic. This survey can better evaluate the various measures in response to the infodemic. For the convenience of sample collection, this study adopts a snowball sampling method, while taking into account characteristics such as province and age. The questionnaire consists of 25 items, including the basic demographic information of the respondent and the use of Internet platforms. Three Internet media platforms, WeChat, Weibo, and Toutiao, were involved in the interview, and this study utilizes items with 7-point Likert-scales to measure the respondents' usage and perception on the infodemic. The specific analysis will be shown in the fourth chapter of this article.
Model Setting: BPI Model
In light of insufficient systematic and theoretical research on infodemic governance, this study hopes to propose an innovative, feasible theoretical framework, the BPI model, based on the dual Chinese experience in dealing with the COVID-19 pandemic and the infodemic. Further, this study hopes to illustrate the Chinese infodemic prevention and control system and its effects with the help of this model.
According to the Chinese “COVID-19 Prevention and Control Plan (7th Edition),” the main prevention and control modes for blocking the spread of the COVID-19 pandemic can be summarized as follows—blocking the spread of the virus, treating infected people, and improving immunity of the public. Research has proved that this prevention and control model is extremely effective. This was affirmed by the representative of the WHO in China, Gauden Galea, who believes that this model is useful at a global scale. All these prevention and control measures are of reference significance.
Does this model have reference significance in the context of the infodemic as well? Since digital media and social media are the focus areas of the infodemic, the appropriate governance path should incorporate the operating characteristics of the internet platform itself. As producers and distributors of information, Chinese Internet platforms use algorithms extensively in the process of information dissemination, and allow algorithms to play the role of gatekeeper. As mentioned previously, Xu et al. (2009) summarize three types of recommendation algorithms, which correspond to the three major components of the COVID-19 pandemic prevention and control model.
Collaborative filtering recommendation can use information feedback that appears in a group of similar interests as the basis for whether the information is delivered to other users in the group. Specifically, in a particular content section, if a rumor is reported by the user, the algorithm judges it as low-quality content. This reduces the number of times that this content is recommended to other users who follow the section, thereby blocking the spread of rumors. Content-based recommendation involves recommending related content to users based on their browsing history. For example, it is possible to distribute the content of the rumors to users who have already seen their content, thereby reducing or eliminating their impact. Knowledge-based recommendation involves recommending specific knowledge produced by experts or professionals to those users who are interested in that field, so that scientific knowledge on COVID-19 prevention, vaccination, and other related issues can be recommended to those who are concerned about the pandemic. In this recommendation model, if the production of scientific knowledge is advanced before the generation of rumors, then users gain the ability to use this knowledge to resist the influence of the rumors.
Regarding the participants in the governance of infodemics, Rong and Yan (2012) describe the three components of the governance body involved in the multiple co-governance of Internet rumors—government as the dominance of the good governance of Internet rumors, the public as the foundation, and non-governmental organizations as the link in the structure. Online rumors are not equivalent to the Infodemic but an important component of it; however, the basic framework of online rumor management and infodemic management are homogeneous to a certain extent. This study argues that because Internet platforms are at the center of information dissemination, they play a unique role in the spread as well as governance of infodemics and are an important part of non-governmental organizations. Therefore, this study defines three types of participants in infodemic management—government departments, Internet platforms, and Internet users.
Consequently, this study posits that the Chinese COVID-19 pandemic prevention and control model and the three models of the algorithm recommendation system can be combined into a basic model or framework for infodemic management. We term it as the BPI (Block-Push-Intervene) model, which means blocking the spread of rumors and false information, pushing accurate information to users affected by the infodemic precisely, and distributing scientific information to users to enhance their immunity to infodemics. The schematic diagram of the model is shown in Figure 1.
In case of this model, two details warrant special mention. First, this is not a linear model, because neither the Infodemic nor the information dissemination itself is linear. The three links between the different compartments of the model form a relationship based on organic interactions and mutual promotion.
Specifically, in the “Push” section, the important basis for its content and audience selection is the rumors and false information selected in the “Block” link and their readers. Therefore, the “Block” stage contributes to the “Push” stage in terms of content and audience selection. In the process of “Push”, users can also give feedback on the content of refuted rumors. Because the understanding of scientific knowledge is always a gradual process, not all the content of refuting rumors is completely scientific and authentic. The feedback of the rumor-refuting content can be passed through collaborative filtering to determine whether it should be recommended and disseminated, so as to prevent the large-scale dissemination of unnecessary information, which is the “Block” in this model.
In the “Push” section, the algorithm not only distributes the content of rumors to users affected by the Infodemic, but also establishes a focus group that is vulnerable to the Infodemic based on a large amount of data. This group can be used as one of the primary target audiences in the “Intervene” section. The user feedback of the content in the “Intervene” process has also become the basis for re-screening the target audience for an accurate push, and this feedback has become the basis of algorithm optimization. This also shows that there is an interactive effect between the “Push” and “Intervene” compartments.
After the rumors and false information identified in the “Block” stage have accumulated to a certain level, the basic development process of the COVID-19-related infodemic can be determined. This process can be used as a basis for inferring the content required in the “Intervene” stage. On digital media and social media platforms, content producers are not only authoritative organizations, but also providers of user-generated content (UGC). Due to the varying credibility of content publishers, UGC is a major breeding ground for false information and rumors. Therefore, in the “Intervene” section, false information may interfere with user cognition and judgment in advance, and collaborative filtering can also play a role. In such a scenario, the infodemic can be blocked in the early intervention stage, i.e., the two links of “Intervene” and “Block” are also mutually promoting.
The second detail that warrants explanation is that, although the proposal of the BPI model is based on the three major measures for the prevention and control of the COVID-19 pandemic, this does not mean this model only has reference significance for the information related to this pandemic. In fact, the BPI model can also be applied to other types of infodemics. To control false information and rumors related to any issue, it is necessary to first block the spread of such information, disseminate correct information to the affected group, and take further measures to ensure timely intervention. This model also fits the existing research findings in the field of public relations.
In a nutshell, the BPI model is based on the juxtaposition and integration of the three types of recommendation algorithms and the three major measures for the prevention and control of the COVID-19 pandemic. The three main stakeholders, i.e., Internet platforms, government departments, and Internet users are involved in blocking the transmission of misinformation, pushing accurate information to users, and intervening in advance. The interrelated steps comprise the main framework for algorithm-based infodemic management.
The BPI model provides an integrative perspective on dealing with the infodemic. Compared to other models related to the governance of rumors on Internet platforms, this model can help better understand the intervening in role of technology and the agents involved in the process of combating infodemics.
According to the BPI model, this study also provides a preliminary answer to RQ1—the Chinese infodemic prevention and control system is led by the Office of the Cyberspace Administration of China and other government departments, in conjunction with Internet media platforms such as Tencent, Sina, and Toutiao. Internet user reports provide important clues for rumors or false information, and a multi-agent interactive prevention and control system is introduced for verification by experts in related fields.
The Composition and Strategic Choice of the Chinese Infodemic Prevention and Control System
This study utilizes the BPI model framework to analyze the composition and strategy selection of the Chinese infodemic prevention and control system. It aims to understand how each component prevents the spread of rumors or false information during the pandemic, pushes true information, and intervenes in advance, and focuses on the role of algorithms in this process.
Through in-depth interviews, this study summarizes the basic modes and overview of the main components of the Chinese Infodemic prevention and control system to deal with Infodemics, as shown in Figure 2.
Central Cyberspace Administration of Chinese Illegal and Bad Information Reporting Center: The Center of the Prevention and Control System
Under the guidance of 32 national ministries and commissions, the Reporting Center has established a very close cooperative relationship and information access mechanism with various government ministries and commissions. This facilitates the dissemination of official information and the verification of false information. The China Internet Joint Rumor Refusal Platform established by the Reporting Center establishes an intermediary bridge between the central and local governments. Internet platforms represented by Tencent, Toutiao, Baidu, and Sina Weibo are all cooperating units of the platform, while People's Daily Online, Xinhua Net, and 20 other provincial-level rumor-refuting platforms are all member units. The transmission of central information to different localities, the feedback of local information to the central government, and the verification of information involving both parties on the Internet platform can all be carried out on this platform.
The Reporting Center's rumor rejection mechanism mainly consists of four major components—discovery, verification, rumor refutation, and dissemination. The schematic diagram is shown in Figure 3.
At the discovery stage, the Reporting Center has three sources of information: Xinhua Net's big data service which assists the Reporting Center in obtaining information on rumors and public opinion trends, the Reporting Center's 12377 hotline and the United Rumor-defying platform website which both accepts individual reports from netizens, and the member units in the above organizational structure which report these rumors and false information to the Reporting Center.
At the verification stage, the Reporting Center plays the intermediary role of the joint anti-rumor platform, and verifies rumors and false information through two channels, i.e., upward and downward. The schematic diagram is shown in Figure 4.
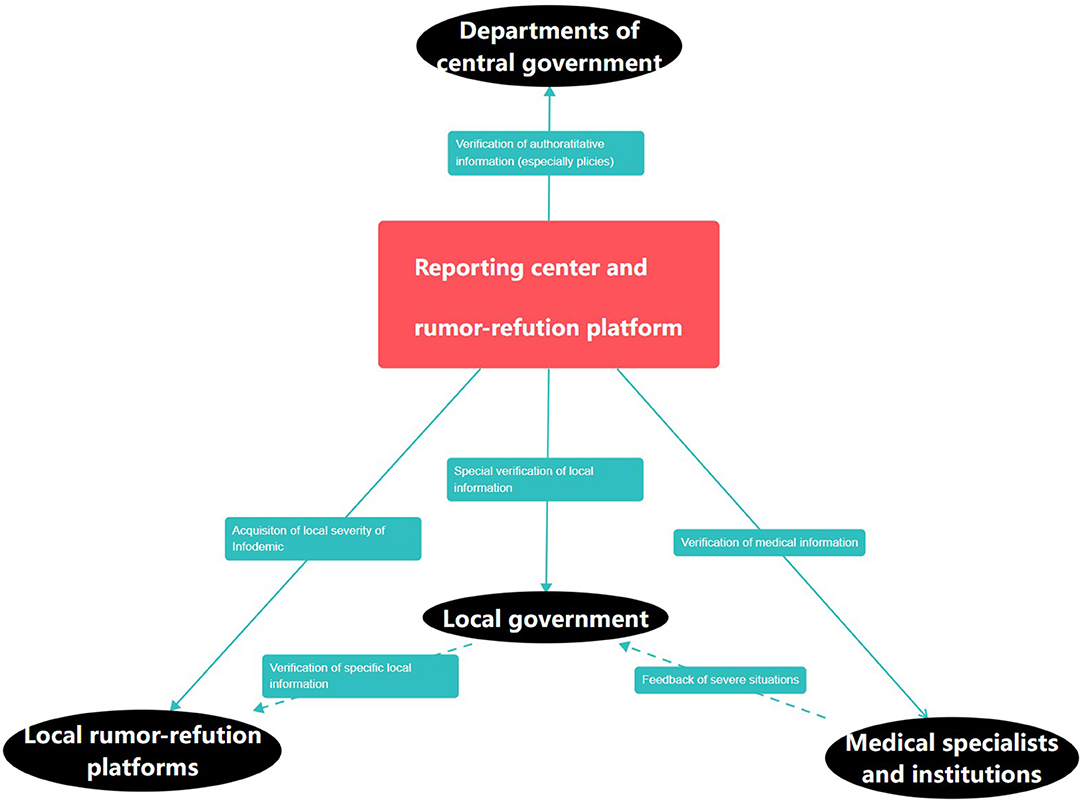
Figure 4. Schematic diagram of the upper and lower dual-channel verification of the Reporting Center (Solid lines represent the interactions between the Reporting Center and other subjectives, while dotted lines represent the interactions between the other subjectives mentioned).
The Reporting Center carries out the process of refuting false information or rumors verified on the joint rumor-refuting platform and releases true information. In the process of contact and verification, the Reporting Center itself produces a certain amount of anti-rumor content, and processes some authoritative releases as new anti-rumor content on the joint anti-rumor platform.
At the communication stage, the joint anti-rumor platform serves as a platform for the dissemination of rumor information. However, subject to the size of its audience, the Reporting Center also cooperates with its partner units to publish its anti-rumor content on Internet platforms such as WeChat, Weibo, and Toutiao to maximize the scope of dissemination, in order to more comprehensively reach Internet users who may be affected by rumors and false information. In particular, the Reporting Center leverages Internet platforms to recommend up-to-date certified content and rank this content higher in the information or recommendation flow.
Staffs of the Reporting Center revealed that to achieve international cooperation, a dedicated office had been established to participate in the management of rumors and false information in the international field. However, during the COVID-19 pandemic, the Reporting Center did not establish cooperation with other countries or related organizations in managing the infodemic.
In summary, the working model of the Reporting Center is a reflection of the basic framework of Chinese infodemic governance. In other words, government departments and Internet platforms have their respective advantages of authority and strong dissemination, and are jointly committed to the governance of infodemics.
Tencent Jiaozhen: A Representative Platform for Accurate Push
Established in November 2015, Tencent Jiaozhen not only reaches users, but also serves various content-based products within Tencent's entire product group to achieve greater influence. It has adopted a human-machine collaboration approach to discovering, verifying, and refuting rumors and false information.
To ensure the discovery of rumors, Tencent accepts user reports while also searching the entire network for clues regarding possible rumors or false information. Additionally, various content-based products under Tencent have feedback interfaces for rumors or false information that are connected to Tencent's Jiaozhen.
To verify unknown rumors, Tencent Jiaozhen uses its professional verification team and associated capabilities. Tencent Jiaozhen's algorithm system can automatically investigate and deal with known rumors and feed authentic information back to users.
For refuting rumors, Tencent Jiaozhen publishes verified content on the homepage of Tencent's rumor-refuting platform, and uses algorithms to accurately push this content to users exposed to the relevant rumors through the WeChat dispelling assistant.
It is worth noting that Tencent's report feedback system has introduced a large number of algorithm-powered technologies to provide users with a better experience. There are three main functions in the micro program of Jiaozhen.
The first is that the user can search through all the content that has been refuted by Jiaozhen. In other words, all the verified content is stored in their database. Secondly, users can participate by submitting evidence of rumors, which the Tencent staff will follow up on. Thirdly, users who are reluctant to use the search function can communicate directly with the “Jiaozhen robot” and submit keywords, for instance, and the robot will then search for the verified content in the archived data. The operating logic of this robot is a combination of content-based recommendation and knowledge-based recommendation.
The interviews clearly demonstrate that Tencent Jiaozhen has consciously applied the algorithm to the interception of rumors and the accurate push of rumor-defying content. The algorithm-driven “Jiaozhen robot” improves the efficiency of user reporting and efficiently collects information about the pandemic. It has partially realized the function of dispelling rumors, while the “Rumor Refuting Assistant” has completely realized the accurate dispelling of rumors to groups affected by the infodemic. This addresses the problem of information redundancy caused by large-scale dissemination, and has improved the accuracy of dispelling rumors.
Sina Weibo: Partial Ignorance of the Function of Algorithms
During the COVID-19 pandemic, Weibo first established a cooperative relationship with relevant departments and professional institutions in China. Weibo refutes verified rumors according to the differences between original posting and reposting, and large influence and small influence, and accordingly adopts different processing methods. While original content with greater social influence is rejected in the processing of labeling, that with lesser social impact is directly deleted from the platform. Under normal circumstances, the Weibo rumor-rejection algorithm does not delete or tag the reposted content. The Weibo rumor rejection staff calls it “grasp the big and let go of the small” work method to adapt to the heavy task pressure. After verifying any rumors or false information on the platform, they are summarized once a day, and then this content is pushed to all Weibo users through private messages.
As compared to WeChat's approach, Weibo's method of dispelling rumors is not as precise. When interviewed, Weibo's anti-rumors staff suggested that the team had discussed this issue before. However, they believed that if the rumor-dispelling information is pushed backwards to individuals precisely, it may arouse users' disgust because of the large and dynamic volume of rumors and false information.
The platform also has certain social robots that are machine accounts controlled by humans to automatically publish content based on specific keywords in batches on Weibo. Sina Weibo uses a filtering mechanism based on underlying algorithms to handle social robots during the COVID-19 infodemic.
Sina Weibo accounts can be divided into certified and non-certified accounts. Certified users enjoy a higher priority in the recommendation system, and consequently, the information that they publish has greater social influence. In order to prevent authenticated users from publishing rumors or false information, which may have a severe social impact, Sina Weibo has established a credit score mechanism; if an authenticated user publishes a rumor or false information, a certain credit score deduction would be applied upon verification, and the user's priority in the recommendation system would be lowered.
Sina Weibo has also adopted user education in response to the infodemic. The accounts of users who post rumors or false information, or make malicious comments, are blocked and they will not be able to post for 3 days. Following that, the ban can be lifted if the user answers three questions correctly, and they will be informed of the reason for the ban. The effect of education improves users' self-consciousness and prudence in communicating during the infodemic.
In September 2020, Sina Weibo has established a cooperative relationship with the China Association for Science and Technology, proposing to share scientific knowledge regarding a new wave of pandemic and vaccine-related issues that may arise in winter.
In summary, when Sina Weibo participated in infodemic management, it predominantly used manual discovery and verification of rumors, and adopted a large-scale dissemination method for the dispelling of rumors while failing to perform an accurate push the way Tencent does. Although the Weibo anti-rumor team argued that an accurate push to users exposed to rumors or false information may disturb them, this study posits that pushing all the anti-rumor content of the day beforehand could result in information redundancy from a user's perspective. This study further discusses the choice of making an accurate push in the summary section of the interview and in the questionnaire survey.
Toutiao: A Model for In-Depth Algorithm Participation
Toutiao's anti-rumor approach is divided into two main parts. The first is to participate in the management of rumors and false information, to reduce the risk of rumors and false information, and to limit the volume of such content. The second is to actively promote science and anti-rumor columns and conduct special anti-rumor campaigns for middle-aged and elderly people, and to encourage health authors to produce anti-rumor and health science content.
The working mechanism of Toutiao's infodemic management involves first discovering rumors and false information through back-end monitoring and receiving user reports. Then, professional authors on the site, Bytedance's own medical team or external medical health experts are contacted to verify this information. Confirmed rumors or false information is directly removed to prevent it from spreading further. Furthermore, Toutiao accurately pushes more content to users.
Similar to Tencent's precise push mode, Toutiao pushes related rumor-defending articles to users who have been exposed to the content of the rumors. These articles are shared with users in the form of application notifications, mobile phone pop-ups, or recommendation streams (information feeds). The form of the push is contingent upon the social influence of the specific piece of rumor or false information.
Toutiao applies the relevant algorithms to the discovery of rumors or false information, refutation of rumors, and early intervention. Toutiao's algorithm uses NLP (Natural Language Processing) technology to make a preliminary judgment on whether the content is rumor or false information based on the popularity of the content and user feedback, and add it to the manual screening library. If a rumor is confirmed to have reappeared, the algorithm automatically matches and removes it from the rumor database, completely independent of human involvement. In addition to using NLP technology to identify textual rumors or false information, Toutiao also uses optical character recognition (OCR) technology to search for rumors and false information contained in pictures, and uses speech-to-text (STT) technology to analyze video content. Through utilizing these three technologies, Toutiao can effectively identify rumors in various forms of information such as text, pictures, and videos.
In the process of dispelling rumors, users' historical reading data of rumors is important to determine an accurate push. Choosing push methods of different strengths according to the severity of the rumors also ensures the effectiveness of dispelling rumors and reduce the interruption for users. Toutiao's algorithm automatically includes rumor and popular science content in the recommendation stream on a daily basis based on the classification and quality of such content, and recommends it to those in need. The content that is particularly high-quality or that the anti-rumor work team believes needs to be disseminated is given a higher recommendation weight in the information flow.
In the development stage of the COVID-19 pandemic, Toutiao quickly recognized that the launch of the vaccine could become a possible infodemic outbreak point. Therefore, it collected vaccine-related science knowledge in advance and pushed it to users in the information flow using the above methods, paying special attention to the authenticity of vaccine-related information, and further conducted verification and refutations of rumors.
In a nutshell, Toutiao and Weibo both rely on algorithms to identify rumors and false information that involve social robots.
For users with higher ratings and stronger influence on the site, Toutiao uses the above-mentioned recommendation weight adjustment method. If the algorithm detects that rumors or false information have been posted, the account's recommendation weight will be flagged and reduced.
Table 1 displays the results of the interviews more intuitively and concisely from the six perspectives of rumor identification, rumor verification, dissemination blocking, rumor rejection methods, early intervention, and algorithm participation.
With Chinese Internet platforms actively participating in infodemic management, the relevant algorithms can effectively identify and manage the reappearance of existing rumors. Therefore, the ability of the governance body to adapt and adjust to the process of prevention and control of the pandemic can be improved in the long-term.
Neither the Reporting Center nor any Internet platforms have established an effective cooperation mechanism with foreign organizations or professional institutions regarding rumors or false information about foreign pandemics. There is no consensus on the basic model of Infodemic management in various countries. Therefore, in the management of Infodemics, there are structural deficiencies in information interconnection and cooperation mechanisms at a global scale.
In the “Push” stage of Infodemic management, Tencent and Toutiao are representative platforms that apply algorithms for accurate push, while Sina Weibo does not use this method. Based on this study, an accurate push is perhaps more in line with the effective management of Infodemics, which have a negative impact not only on Internet users, but also on their cognition and mental state. Rumor-refuting content is essentially a kind of information. If such content is pushed to users who don't see the rumor or false information, then this kind of information is not only ineffective, but will also cause confusion among them. As a result, they would also become affected by the infodemic. The precise push is more in line with the goal of controlling the infodemic, that is, to achieve a “Pareto Optimality” in a sense. The governance of the infodemic is an important part of the governance of the Internet society and one of the important components of national governance, and “Pareto improvement” is also one of the important goals of national governance and policy design.
The results of the interview regarding the “Intervene” link of Infodemic management broadened the existing scope of this study. Early intervention not only includes the popularization of pandemic-related knowledge, but also includes the advanced construction of a rumor verification contact network and user education.
To a certain extent, the user education practices of Toutiao and Sina Weibo are more fundamental early intervention practices for groups affected by the infodemic. Based on the analysis above, this study summarizes the basic mode of Chinese Internet platforms participating in infodemic governance in the “Intervene” link; in the development stage of the COVID-19 pandemic, these entities established contact with experts in relevant fields in advance to verify rumors, educate users and try to fundamentally improve their self-judgment and immunity against rumors or false information.
This study uses semi-structured interviews to summarize the Infodemic prevention and control system in China and also test the effectiveness of the BPI model. More importantly, semi-structured interviews have also enriched the structure of this model. The basic framework of Infodemic management should also include professional institutions and experts in medical and health fields. These are important participants in the verification of rumors and false information, and are also among the main producers of content.
Although semi-structured interviews have helped establish a more comprehensive understanding of Chinese experience in responding to the Infodemic, they do not reveal the effectiveness in terms of the specific choice of measures of each subject. Internet users are the group most affected by the infodemic. Therefore, considering the perception of these users while using different Internet platforms is necessary to understand the basic mode and reference experience of Chinese response to the Infodemic. The detailed results of the questionnaire are shown in the next section.
Audience Perception of Infodemic Prevention and Control
Upon developing a relatively comprehensive understanding of the actions of various platforms in the Chinese infodemic prevention and control system, this study further adopts the method of questionnaire-based surveys to evaluate the actions of various platforms from the perspective of the audience. The questionnaire is based primarily on the BPI model. It measures the perception of the audience from three perspectives—user behavior, infodemic perception on the platform, and self-emotional evaluation.
According to the BPI model, the Chinese infodemic prevention and control system also includes Internet users. The users' perceptions of platform behavior are also important factors in evaluating the effectiveness of this system. Therefore, this study investigates these behaviors and perceptions of users during the COVID-19 pandemic by means of questionnaire surveys.
The scale questions designed by this research can be divided into three categories, user behavior, perception of infodemic on the platform, and self-emotional assessment. Out of 555 samples, 7 invalid samples were eliminated by observing the box diagram of the time taken to fill the questionnaire, and 10 invalid samples were eliminated by screening on the basis of smartphone usage. As a result, 538 valid samples were obtained. In this study, the statistical analysis software Stata MP16.0 was used. The Cronbach alpha coefficient of the questionnaire was found to be 0.8075, which is suitable for further statistical analysis through the reliability test.
According to the results of the survey, WeChat users utilize the platform most frequently (mean = 4.360, sd = 0.961), followed by Weibo (mean = 3.958, sd = 1.23) and Toutiao (mean = 3.462, sd = 1.224) as can be seen in Figure 5.
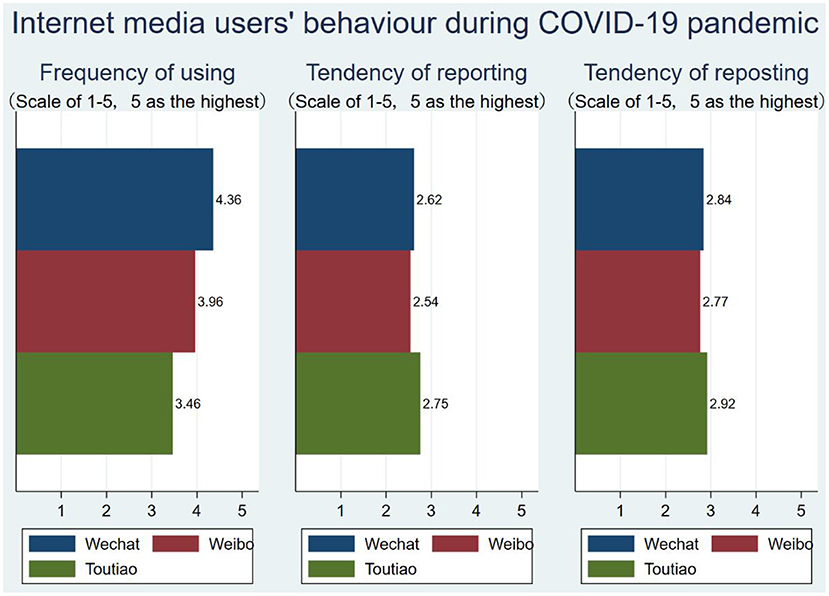
Figure 5. A bar graph comparing the behaviors of users of different Internet media platforms during the COVID-19 pandemic.
Toutiao users are more inclined to report observed rumors or false information (mean = 2.754, sd = 1.260), while the tendency of WeChat (mean = 2.615, sd = 1.336) and Weibo (mean = 2.538, sd = 1.280) users are quite similar. Additionally, users of Toutiao are also more inclined to repost information regarding the pandemic situation that they observe on the platform (mean = 2.918, sd = 1.185). This tendency reveals that users of Toutiao may have a higher degree of trust in the pandemic-related information on the platform, and this information would, therefore, be easier to spread on other social media platforms. Integrating the tendency to report and the tendency to repost, this study infers that the users of Toutiao have a higher level of involvement and participation in the content available on the platform.
As shown in Figure 6, users were found to believe that the number of rumors on WeChat is significantly higher than that on the other two platforms (mean = 4.360, sd = 0.961). In terms of the speed of dispelling rumors (mean = 3.626, sd = 0.976) and the abundance of accurate information (mean = 3.754, sd = 0.926), Toutiao also performs better according to user perceptions. This has lent credence to the above inferences that Toutiao experiences a lower level of infodemic and higher levels of infodemic governance. This has made its users more prone to repost information on the platform and actively participate in the reporting of rumors or false information, which also promotes the formation of a virtuous cycle.
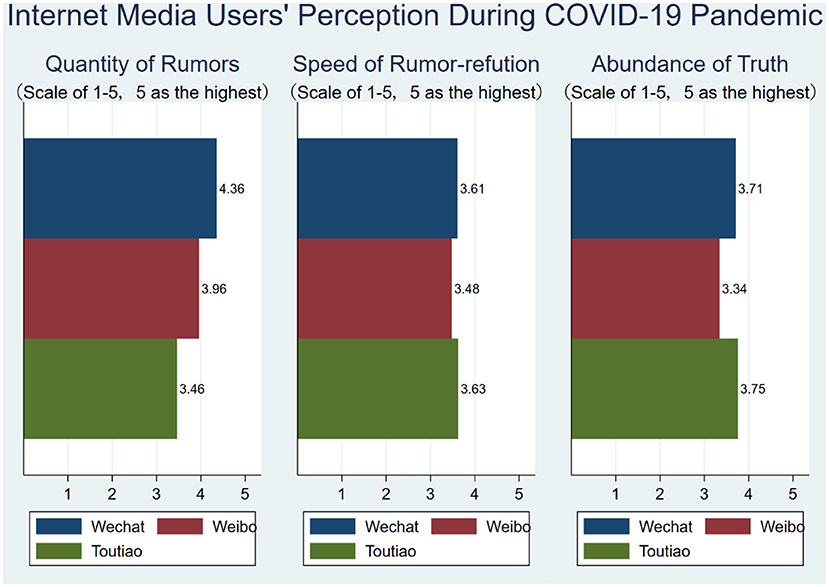
Figure 6. Bar graph of user perception comparison of Internet media platforms during the COVID-19 pandemic.
This study analyzes “negative emotions such as anxiety and loss of confidence perceived when using a specific media platform” to evaluate the impact of the infodemic on users. As can be seen in Figure 7, the negative sentiment index of Toutiao and WeChat users is low, while that of Weibo users is relatively high (mean = 2.912, sd = 1.257). This study analyzes the reason for this phenomenon using inferential statistics and regression analysis. The key outcome of the infodemic is its impact on peoples' physical and mental health. Therefore, it is necessary to conduct an in-depth analysis of this aspect.
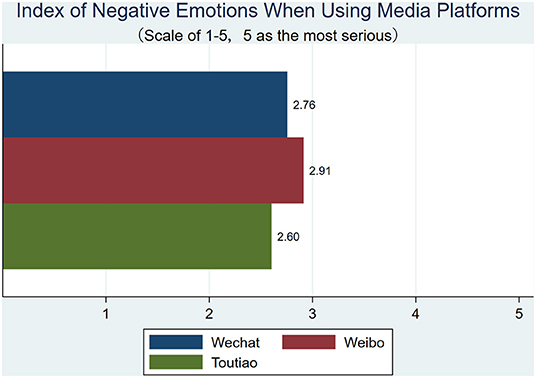
Figure 7. Bar graph of negative sentiment index when using media platforms during the COVID-19 pandemic.
Firstly, this study attempts to examine the statistical differences between specific categorical variables through the chi-square test. Gender is an important basis for accurate push targeting, and according to Cui and Wu (2019), it affects users' perceptions of public affairs. Using the chi-square test, it was found that there is a significant difference between males and females (χ2 = 7.5019, P < 0.01) in the use of Weibo. It shows that the proportion of female samples using Weibo is greater than that not using Weibo.
Using age as a column variable, it was observed that there are significant differences in the use of Weibo (χ2 = 202.0839, P < 0.01) and Toutiao (χ2 = 122.0432, P < 0.01) among samples of different ages. The usage rate of samples older than 30 is significantly lower than that of samples aged 30 years and younger. In case of Toutiao, the usage rate of samples older than 40 is significantly higher than that of samples aged 40 and below. These clear divisions are likely to affect users' platform usage behavior and infodemic perception. Therefore, in this study, ages 30 and 40 were used as the dividing lines for further analysis of the samples, and two new binary age variables “ageWeibo” and “ageToutiao” were generated.
Since the chi-square test results show that there is a relationship between Weibo usage and gender, this study also carried out a gender-based variance analysis on the seven questionnaires dealing with Weibo usage behavior and perception.
The results show that the frequency of use of Weibo is significantly higher among women than men, and is about 0.5 higher on the 5-level scale (F =7.56, P < 0.01). As compared to women, men are more inclined to report rumors or false information (F = 4.60, P < 0.05).
As compared to male users, female users believed that Weibo's rumors dispelling speed was high (F = 5.68, P < 0.05). This result and the previous analysis confirm that because female users are more inclined to believe that Weibo dispels rumors faster, they are less inclined to report rumors or false information.
Using the same method and grouping basis, a variance analysis was conducted on seven scale questions related to WeChat. The results show that on the WeChat platform, men are more inclined to report rumors or false information than women (F = 5.45, P < 0.05).
Based on these analyses, a preliminary conclusion was formulated. On social media platforms such as Weibo and WeChat, male users are more inclined to report rumors or false information than female users. This also reflects a phenomenon worth analyzing—why do men and women tend to report differently on social media platforms? This study speculates that the convenience of reporting and the difference in sensitivity of men and women to rumors or false information may be two potentially influencing factors.
Based on the chi-square test, this research generates two new binary age variables. Therefore, this study also uses these two variables as the basis for grouping, and carries out the variance analysis of scale questions of the respective media platforms.
Based on the seven questions on Weibo, there is a significant difference in the frequency of Weibo usage (F = 32.74, P < 0.01) and the perceived number of rumors (F = 42.04, P < 0.01) by different age groups. Those older than 30 years old showed lower frequency of Weibo usage and poorer perception of rumors and false information. These differences are >1 on the five-level scale, which is a significant difference. Therefore, to build a regression model, the new age variables are considered as interaction terms for the frequency of Weibo usage and the number of rumors perceived on the Weibo platform.
Analysis based on the seven scale questions on Toutiao revealed that there is a significant difference in the number of rumors perceived by users of different ages (F = 9.49, P < 0.01). As mentioned previously, this study will use the new age variables and the number of rumors perceived as interaction terms while conducting regression analysis for Toutiao.
A linear regression analysis was performed on the questionnaire results regarding WeChat. It was found that WeChat users of different genders have significantly different tendencies to report rumors or false information. Therefore, gender and reporting tendency are considered as interaction terms. Additionally, because WeChat is the largest Chinese social platform, users often use it to repost information that they see on other platforms. Especially during the COVID-19 pandemic, WeChat has become an important medium for sharing information about the pandemic with family members and friends. Therefore, the number of rumors perceived by users of Weibo and Toutiao were included as proxy variables in the regression model to replace an indicator that is difficult to measure, i.e., frequency of reposting of rumors or false information on other platforms via WeChat.
A regression analysis was run for WeChat using the negative emotions perceived by its users as the dependent variable. The independent variables were the other six scale questions on WeChat and the number of rumors perceived by users on Weibo and Toutiao. The interactive terms were reporting tendency on WeChat and gender.
The Breush-Pagan test result is realistic (F = 3.23, P < 0.01). The null hypothesis of homoscedasticity is rejected, and the model has the problem of heteroscedasticity. In order to correct this, traditional OLS regression approach was abandoned and instead, feasible generalized least squares regression was used for analysis. The results are shown in Table 2.
Based on the interaction items of reporting tendency and gender, it is evident that the reporting behavior of men significantly affects the perceived negative emotions (P < 0.01). More specifically, for male users, for an increase of one unit on the five-level scale in the tendency to report rumors or false information seen on WeChat, the level of negative emotions perceived rose by 0.182 levels. This implies that men's reporting tendency is positively correlated with the level of negative emotions they feel.
This finding appears to indicate a more indirect relationship; however, in light of the actual scenario, it is easy to understand. The tendency to report rumors or false information reflects the user's awareness and trust in the information. The higher the tendency to report, the greater the awareness and the lower the degree of trust. In the process of using WeChat, if there is a low level of trust in the information itself, or if the content on the platform makes users suspicious, then there is a natural increase in the level of negative emotions. According to Cui and Wu (2019), men pay more attention to social affairs than women, and WeChat is an important medium for such content. Therefore, during the COVID-19 pandemic, WeChat should consider reducing the push of pandemic-related information to male users—particularly the content produced by non-authoritative organizations—so as to reduce their suspicion and negative emotions, thus limiting their impact on the infodemic.
For Weibo and Toutiao, OLS regression analysis was performed to explore the impact of various factors on the level of negative emotions perceived by users.
It was found that the number of perceived rumors and frequency of usage on the Weibo platform are related to the age variable. Therefore, this study separates the age variable with the number of perceived rumors on the Weibo platform (Weibo2) and frequency of use (Weibo1). The results are shown in Table 3.
Therefore, for the regression analysis of Weibo, this research has the following analysis results:
It can be seen that on the five-level scale, when the tendency to actively repost the pandemic-related information on Weibo increases by one unit, the level of negative emotions perceived by users increased by 0.286 levels, which is statistically significant (P < 0.01). Further, when the user's perceived sufficiency of real information about the pandemic on Weibo increases by one unit, the level of negative emotions they perceive drops by 0.213 units; this negative correlation is also statistically significant (P < 0.05). In terms of the interaction effect, those aged 30 or lower and those above 30 both showed statistically significant differences in the number of rumors perceived (P < 0.01 in both cases). However, the coefficients for the two cases are different. Specifically, for users aged 30 or lower, when the frequency of perceived rumors or false information increases by one unit, the negative emotions they experience increases by 0.418 units. For users aged 30 or above, when the frequency of perceived rumors or false information increases by one unit, the level of negative emotions perceived increases by 0.367 units. Therefore, in terms of perceived rumors or false information, users aged 30 and below are more greatly affected by the infodemic.
From these results, it can be seen that the active reposting tendency and the volume of rumors or false information perceived are positively correlated with perceived negative emotions. Therefore, when managing the infodemic, Weibo should pay attention to methods of reducing the impact of users on the infodemic in terms of reposting behavior. For example, the keyword recognition function of the algorithm can be used to remind users to think carefully when reposting information related to COVID-19, and provide relevant content for users to refer to. This would help in mitigating the psychological frustration experienced by users when they repost rumors or false information. Due to the obvious age stratification of its users, Weibo should also consider more careful content screening for users aged 30 and below while managing the infodemic. The positive correlation between the number of rumors or false information and the perceived negative emotions demonstrates that controlling the number of rumors on the platform is critical to the management of the infodemic. At the same time, Weibo should also produce more content and reduce the impact of the infodemic on users by increasing the abundance of real information on the platform.
Similarly, based on the correlation between certain variables and age, regression analysis was performed for Toutiao as well. The results are shown in Table 4.
According to these results, it can be seen that the number of rumors or false information perceived by Toutiao users of different ages is positively correlated with the negative emotions they perceived. For those aged 40 or lower, when the level of rumors or false information they perceive increases by 1 unit, the negative emotion they experience increases by 0.493 units. For those above 40, when the degree of rumors or false information increases by 1 unit, the negative emotions they perceive increases by 0.502 levels.
Therefore, in response to the infodemic, Toutiao should also take targeted measures against users who use it more frequently, i.e., users over the age of 40, such as pushing more content from authoritative sources to provide more targeted scientific information. This is conducive to achieving a more precise reduction of the impact of the infodemic on its users.
The analysis of the survey results has provided answers to the initial research questions. In the process of infodemic management, the algorithm-driven accurate push is an effective means of dispelling rumors, which can better reduce the has impact of infodemic on users. Additionally, on platforms where the gender and age of users play a major role, the accurate push is even more realistic. It can be observed from the statistical analysis that, as compared to WeChat and Toutiao which use the algorithm-driven accurate push, Weibo users perceive a lower abundance of accurate information, and their levels of perceived negative emotions are also higher. It can also be seen that the number of rumors exposed by users in the age group with a higher frequency of Weibo usage during the pandemic has a greater impact on the level of negative emotions. These findings support the necessity of using an accurate push to dispel rumors and provide true information.
In addition, it was also found that the function settings for reporting on different platforms not only affect the reporting tendency of users, but also affect the negative emotions perceived by them. User reporting is an important method of discovering rumors or false information. Therefore, it is necessary for Internet platforms to set up a user-friendly reporting portal for the better management of Infodemics.
Conclusion
Based on the results obtained, this study attempts to address the specified research questions. The Chinese response to the infodemic prevention and control system is led by the Cyberspace Administration of China and other government departments, in conjunction with Internet media platforms such as Tencent, Sina, and Toutiao. They aim to use Internet user reports as important evidence of rumors or false information, and to encourage experts in related fields to build a multi-agent interactive prevention and control system for rapid verification. The China Internet Joint Rumor Refusal Platform established by the Cyberspace Administration of China integrates the resources of government departments, Internet media platforms, and local media to build a systematic and complete framework for the management of the infodemic, for which Internet media platforms are the front-line forces. They use algorithms and manual methods to effectively and quickly identify rumors, and accurately push the content of rumors through algorithms to minimize their impact. Experts and scholars in medical and health fields are the primary sources for verification of rumors or false information. Smooth communication channels and cooperation mechanisms among these government departments, Internet media platforms, and experts are important to guarantee the efficiency of the Chinese response to the Infodemic.
This prevention and control system plays an important role in the function of different entities. Each entity performs its own duties to ensure high efficiency in responding to the infodemic caused by the sudden COVID-19 pandemic. Each entity exerts its comparative advantages, especially by the use of algorithm technology on the Internet platform, to reduce the secondary disaster of the Infodemic caused by information redundancy. The advantage of government departments lies in their mobilization ability; hence, it is more effective to build a platform that allows multi-subject cooperation by government departments. The advantage of Chinese Internet media platforms lies in the accumulation of a large amount of data and the advancement of algorithm technology. Therefore, the discovery of rumors and the promotion of rumor-defying content by such platforms has technical advantages. Experts themselves do not have strong information dissemination capabilities, and it is more appropriate for them to be the producers of content to refute rumors. Based on the framework of multi-agent participation in governance, the initiative of Internet users has also been brought into full play. The information reported by users closes the loopholes in algorithms and manual identification. The Chinese experience in responding to infodemics is an important manifestation of digital thinking.
Algorithms are a very effective tool when dealing with infodemics, because they can help realize the rapid salvage of massive amounts of information and achieve precise access to those affected by the infodemic.
The BPI model proposed in this study has also essentially been proved through the process of analyzing the semi-structured interviews and questionnaire surveys. This model is an abstraction and generalization of Chinese Infodemic management, and can be used as a theoretical tool that can be extended to other countries. Based on the above research and analysis, the revised BPI model is shown in Figure 8 below.
As compared to the BPI model proposed for the first time above, after in-depth interviews and questionnaire surveys, the main body of ‘medical and health institutions and experts’ has been identified as an important subject for verification of rumors at the participation stage, linking education of users and timely interventions.
Although China has made remarkable advancements in infodemic response, the algorithm-driven multi-subject co-governance model has strong promotion significance. However, the management process still faces two issues. First, the importance of algorithms has not been universally recognized by Internet media platforms. Secondly, China has a low degree of international cooperation in the infodemic response process. The processing efficiency of domestic rumors or false information on an international scale is still low. Therefore, as the dominant force in the Chinese Infodemic management system, government departments should take the lead in promoting the role of algorithms, and jointly build and share data and algorithm tools with various Internet media platforms. There is also a need to establish a mature information exchange mechanism in the global field, especially during events such as the COVID-19 pandemic. The exchange of information on the pandemic among various countries would play an important role in reducing the level of Infodemics at a global scale.
Although this study is the first to systematically explore countermeasures in the academic field of Infodemics, particularly involving the study of the function of algorithms, it still has great limitations.
First, subject to the interview conditions, this study failed to include all mainstream Internet media platforms in China in the scope of the semi-structured interviews. Therefore, the summary of the ways in which these platforms participate in the management of infodemics is only representative and not comprehensive. Some subjects with relatively small user levels but unique methods of participating in Infodemic management were not included among the interviewees, such as Dr. Dingxiang, which is a professional medical science popularization institute.
Second, in the questionnaire survey, due to the sample size and the influence of cross-platform content dissemination, this study failed to use the instrumental variable method that is most commonly used for such research. Since this study was not carried out during the period when the COVID-19 pandemic in China was most serious, the respondents' perceptions may also deviate from the actual situation at the time, and might not be very accurately reflected.
Third, due to the limitations of the online snowball survey, this study did not use a more accurate composite scale when measuring the user's perception of negative emotions.
Fourth, due to the limitations of the researcher's disciplinary background, this study only discusses the functional use and characterization aspects of the operating mechanism of the algorithm, and does not discuss application scenarios from the perspective of this mechanism. Fifth, during the interview process, the interviewees were only asked in detail about the algorithm tools found in the literature review. Therefore, certain other algorithm tools used by the interviewees may potentially be omitted.
As a pioneering study, this research hopes to inspire more researchers to pay attention to the academic topic of infodemic management, especially when the global pandemic is spreading, to think about how to reduce the negative impact of this pandemic on the public from a perspective other than epidemiology. In the future, researchers can consider a large-scale user survey, especially in the context of the functional design of user reporting and information recommendation on the platform. This could help in exploring the possibility of a set of algorithm-driven feature designs that can be promoted in the process of managing infodemics.
In addition, this study only summarizes the Chinese experience, and does not analyze the reasons why other countries with more serious infodemics have failed in governance. It is hoped that other researchers will be able to explore the modes of infodemic management in these countries from different perspectives and compare them with the Chinese experience, so as to provide a better reference for the governance of global infodemics.
Data Availability Statement
The original contributions presented in the study are included in the article/supplementary material, further inquiries can be directed to the corresponding author.
Ethics Statement
Ethical review and approval was not required for the study on human participants in accordance with the local legislation and institutional requirements. The patients/participants provided their written informed consent to participate in this study.
Author Contributions
Both the authors confirm being the sole contributors of this work and have approved it for publication.
Conflict of Interest
The authors declare that the research was conducted in the absence of any commercial or financial relationships that could be construed as a potential conflict of interest.
Publisher's Note
All claims expressed in this article are solely those of the authors and do not necessarily represent those of their affiliated organizations, or those of the publisher, the editors and the reviewers. Any product that may be evaluated in this article, or claim that may be made by its manufacturer, is not guaranteed or endorsed by the publisher.
References
Agarwal, N. K., and Alsaeedi, F. (2020). Understanding and fighting disinformation and fake news: Towards an information behavior framework. Proc. Assoc. Inform. Sci. Technol. 57:e327. doi: 10.1002/pra2.327
Chen, H. M. (2021). Research on related issues of online rumor refutation in public health emergencies from the audience's perspective: Based on the analysis of major online rumor refutation platforms in the COVID-19 pandemic. Contemp. Commun. 2021, 109–112.
Cheng, C., Ebrahimi, O. V., and Lau, Y. C. (2020). Maladaptive coping with the infodemic and sleep disturbance in the COVID-19 pandemic. J. Sleep Res. 30:e13235. doi: 10.1111/jsr.13235
Chong, M. (2020). Network typology, information sources, and messages of the infodemic twitter network under COVID−19. Proc. Assoc. Inform. Sci. Technol. 57:e363. doi: 10.1002/pra2.363
Cui, D., and Wu, F. (2019). The knowledge effect of algorithm push news——take Toutiao as an example. Shanghai J. Rev. 2, 30–36. doi: 10.16057/j.cnki.31-1171/g2.2019.02.005
Di Mascio, F., Natalini, A., Barbieri, M., and Selva, D. (2021). The role of regulatory agencies in agenda-setting processes: insights from the Italian response to the COVID-19 infodemic. Swiss Polit. Sci. Rev. 27, 271–282. doi: 10.1111/spsr.12465
Ding, B. T. (2020). Epidemic information management in the era of artificial intelligence: challenges and changes. Library Inform. Knowl. 198, 111–118. doi: 10.13366/j.dik.2020.06.109
Fang, X. D. (2020). Infodemic roots, laws and governance countermeasures: out of control and reconstruction of international information dissemination order under the background of new technology. News Writing 2020, 35–44.
Fang, X. D., Gu, X., and Xu, Z. L. (2020). The roots, laws and governance countermeasures of infodemic - out of control and reconstruction of international information dissemination order under the background of new technology. Journalism Writ. 2020, 35–44.
Greenspan, R. L., and Loftus, E. F. (2020). Pandemics and infodemics: Research on the effects of misinformation on memory. Human Behav. Emerg. Technol. 3, 8–12. doi: 10.1002/hbe2.228
Grimes, D. R. (2020). Health disinformation & social media. EMBO Rep. 21:e51819. doi: 10.15252/embr.202051819
Gruzd, A., and Mai, P. (2020). Going viral: How a single tweet spawned a COVID-19 conspiracy theory on Twitter. Big Data Soc. 7, 1-9. doi: 10.1177/2053951720938405
Hailing, X., Xiao, W., Xiaodong, L., and Baoping, Y. (2009). Comparative research on internet recommendation systems. J. Softw. 20, 350–362. doi: 10.3724/SP.J.1001.2009.03388
Han, J. W., Park, J., and Lee, H. (2021). Effect of exposure to COVID-19 infodemic on infection-preventive intentions among Korean adults. Nursing Open 2021, 1–10. doi: 10.1002/nop2.965
Harris, Z. (2020). Malaysia's infodemic and policy response. Inst. Strateg. Int. Stud. 2, 1–4. Available online at: http://www.jstor.org/stable/resrep24756
Liu, K., and Huang, Z. Y. (2021). Rumor identification in major emergencies. J. South Chin. Univers. Technol. 49, 18–28.
Myers, N. (2021). Information sharing and community resilience: toward a whole community approach to surveillance and combatting the “infodemic”. World Med. Health Policy 13, 581–592. doi: 10.1002/wmh3.428
Rong, L., and Yan, L. (2012). Multiple subject structure of internet rumor governance in the context of good governance. Soc. Sci. Guangxi 2012, 103–107. doi: 10.3969/1004-6917.2012.09.023
Rothkopf, D. J. (2003). SARS Also Spurs an 'Information Epidemic': [ALL EDITIONS]. Combined editions Edn. Long Island, NY.
Shin, J., and Valente, T. (2020). Algorithms and health misinformation: A case study of vaccine books on amazon. J. Health Commun. 25, 394–401. doi: 10.1080/10810730.2020.1776423
WHO. (2020). Novel Coronavirus (2019-nCoV) situation report 13 2020. Available online at: https://www.who.int/emergencies/diseases/novel-coronavirus-2019/situation-reports
Xu, H. L., Wu, X., Li, X. D., and Yan, B. P. (2009). Comparative study of internet recommender systems. J. Softw. 2, 10.
Yang, S., Fichman, P., Zhu, X., Sanfilippo, M., Li, S., and Fleischmann, K. R. (2020). The use of ICT during COVID−19. Proc. Assoc. Inform. Sci. Technol. 57:e297. doi: 10.1002/pra2.297
Zheng, Y. L. (2020). Research on the dissemination characteristics and strategies of rumor-refuting information in the early stage of the pandemic. Journalism Commun. 10, 29–30.
Keywords: digital governance, COVID-19, infodemic, internet platforms, algorithm
Citation: Wang Z and Xu J (2022) An Empirical Research on How to Tackle Infodemic in China: Stakeholders and Algorithms. Front. Polit. Sci. 4:858093. doi: 10.3389/fpos.2022.858093
Received: 19 January 2022; Accepted: 24 March 2022;
Published: 28 April 2022.
Edited by:
Roxana Radu, University of Oxford, United KingdomReviewed by:
János Kertész, Central European University, HungaryMuhammad Rafi, Nanjing University, China
Copyright © 2022 Wang and Xu. This is an open-access article distributed under the terms of the Creative Commons Attribution License (CC BY). The use, distribution or reproduction in other forums is permitted, provided the original author(s) and the copyright owner(s) are credited and that the original publication in this journal is cited, in accordance with accepted academic practice. No use, distribution or reproduction is permitted which does not comply with these terms.
*Correspondence: Jing Xu, eHVqaW5nQHBrdS5lZHUuY24=