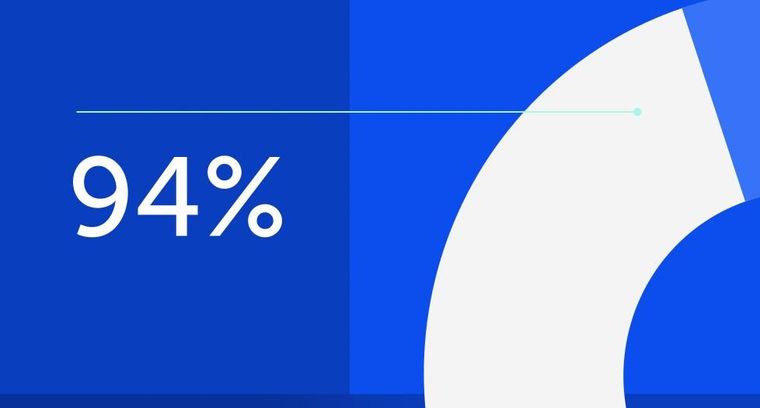
94% of researchers rate our articles as excellent or good
Learn more about the work of our research integrity team to safeguard the quality of each article we publish.
Find out more
ORIGINAL RESEARCH article
Front. Plant Sci.
Sec. Sustainable and Intelligent Phytoprotection
Volume 16 - 2025 | doi: 10.3389/fpls.2025.1594728
The final, formatted version of the article will be published soon.
Select one of your emails
You have multiple emails registered with Frontiers:
Notify me on publication
Please enter your email address:
If you already have an account, please login
You don't have a Frontiers account ? You can register here
Digital tools and non-destructive monitoring techniques are crucial for real-time evaluations of crop output and health in sustainable agriculture. Sustainable agriculture and environmental stewardship require precise AGB computation since it provides valuable insights into efficient crop management and carbon balance in ecosystems.This study uses cutting-edge computer vision and machine learning techniques to propose a novel, non-invasive approach for quantifying above-ground biomass (AGB) of pearl millet. We employed a transfer learning approach, leveraging pre-trained CNN models alongside shallow machine learning algorithms-such as Support Vector Regression (SVR), XGBoost, and Random Forest Regression (RFR). Smartphone-based RGB imaging was utilized for data collection. Convolutional neural networks (CNNs) were trained to predict the above-ground biomass of pearl millet using transfer learning, which incorporated the VegAnn model and its parameters into the convolutional layers of our model. Shapley additive explanations (SHAP) methodology was employed to evaluate predictor importance systematically. The SHAP analysis confirmed that the most influential features (such as Normalized Green-Red Difference Index, NGRDI, and plant height) substantially contributed to AGB estimation accuracy, whereas features with low SHAP values might be excluded from the final model without compromising predictive performance. A comparison of four machine learning models was performed using several feature sets, including all features, the five most significant, and the two most salient. The study found that XGBoost has a comprehensive feature set (R² = 0.98, RMSE = 0.26), while CNN-based models also showed high predictive ability. Notably, RFR performs best with the two most important features, whereas SVR is the least effective model throughout the analysis. These findings demonstrate the effectiveness of CNNs and shallow machine learning in estimating AGB non-invasively using cost-effective RGB imagery. The results show that our technique may be utilized to support automated biomass prediction and real-time plant growth monitoring. These tools could form the basis for small-scale carbon inventories to measure the carbon sequestered within vegetation biomass in smallholder agricultural systems. These inventories are crucial for understanding how agricultural practices contribute to carbon sequestration at the local level and for informing climateresilient strategies
Keywords: Digital agriculture, deep learning, Plant monitoring, carbon sequestration, CNN - convolutional neural network
Received: 20 Mar 2025; Accepted: 11 Apr 2025.
Copyright: © 2025 Dhawi, Ghafoor, Almousa, Ali and Alqanbar. This is an open-access article distributed under the terms of the Creative Commons Attribution License (CC BY). The use, distribution or reproduction in other forums is permitted, provided the original author(s) or licensor are credited and that the original publication in this journal is cited, in accordance with accepted academic practice. No use, distribution or reproduction is permitted which does not comply with these terms.
* Correspondence:
Faten Dhawi, Department of Agricultural Biotechnology, College of Agricultural and Food Sciences, King Faisal University, Al-Ahsa, 31982, Eastern Province, Saudi Arabia
Abdul Ghafoor, Center for water and Environmental Studies, king Faisal University, Al Ahsa, Saudi Arabia
Disclaimer: All claims expressed in this article are solely those of the authors and do not necessarily represent those of their affiliated organizations, or those of the publisher, the editors and the reviewers. Any product that may be evaluated in this article or claim that may be made by its manufacturer is not guaranteed or endorsed by the publisher.
Supplementary Material
Research integrity at Frontiers
Learn more about the work of our research integrity team to safeguard the quality of each article we publish.