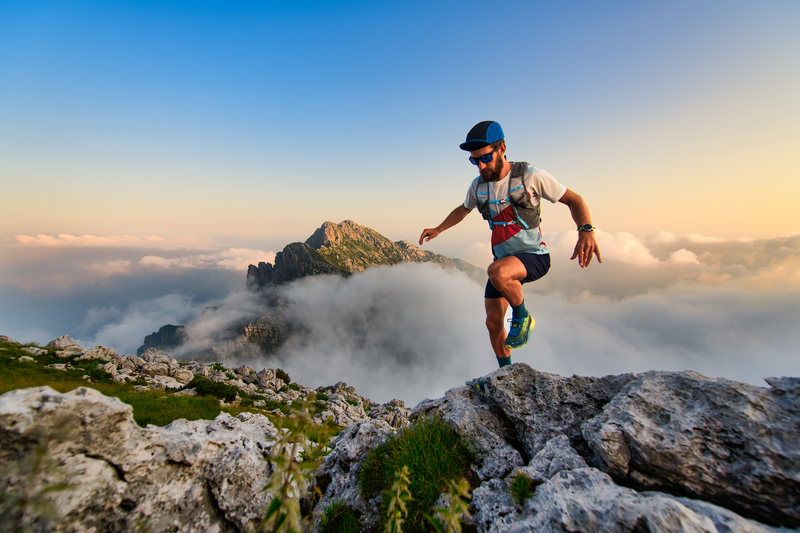
95% of researchers rate our articles as excellent or good
Learn more about the work of our research integrity team to safeguard the quality of each article we publish.
Find out more
ORIGINAL RESEARCH article
Front. Plant Sci.
Sec. Sustainable and Intelligent Phytoprotection
Volume 16 - 2025 | doi: 10.3389/fpls.2025.1576756
This article is part of the Research Topic Advanced Methods, Equipment and Platforms in Precision Field Crops Protection, Volume II View all 11 articles
The final, formatted version of the article will be published soon.
You have multiple emails registered with Frontiers:
Please enter your email address:
If you already have an account, please login
You don't have a Frontiers account ? You can register here
Precision agriculture relies on advanced technologies to optimize crop protection and resource utilization, ensuring sustainable and efficient farming practices. Anomaly detection plays a critical role in identifying and addressing irregularities, such as pest outbreaks, disease spread, or nutrient deficiencies, that can negatively impact yield. Traditional methods struggle with the complexity and variability of agricultural data collected from diverse sources. To address these challenges, we propose a novel framework that integrates the Integrated Multi-Modal Smart Farming Network (IMSFNet) with the Adaptive Resource Optimization Strategy (AROS). IMSFNet employs multimodal data fusion and spatiotemporal modeling to provide accurate predictions of crop health and yield anomalies by leveraging data from UAVs, satellites, ground sensors, and weather stations. AROS dynamically optimizes resource allocation based on real-time environmental feedback and multi-objective optimization, balancing yield maximization, cost efficiency, and environmental sustainability. Experimental evaluations demonstrate the effectiveness of our approach in detecting anomalies and improving decision-making in precision agriculture. This framework sets a new standard for sustainable and data-driven crop protection strategies.
Keywords: precision agriculture, anomaly detection, Multi-modal data fusion, Resource optimization, sustainable farming
Received: 14 Feb 2025; Accepted: 07 Apr 2025.
Copyright: © 2025 Shan and Zhen. This is an open-access article distributed under the terms of the Creative Commons Attribution License (CC BY). The use, distribution or reproduction in other forums is permitted, provided the original author(s) or licensor are credited and that the original publication in this journal is cited, in accordance with accepted academic practice. No use, distribution or reproduction is permitted which does not comply with these terms.
* Correspondence:
Yifeng Shan, Ningbo University of Finance and Economics, Ningbo, China
Disclaimer: All claims expressed in this article are solely those of the authors and do not necessarily represent those of their affiliated organizations, or those of the publisher, the editors and the reviewers. Any product that may be evaluated in this article or claim that may be made by its manufacturer is not guaranteed or endorsed by the publisher.
Research integrity at Frontiers
Learn more about the work of our research integrity team to safeguard the quality of each article we publish.