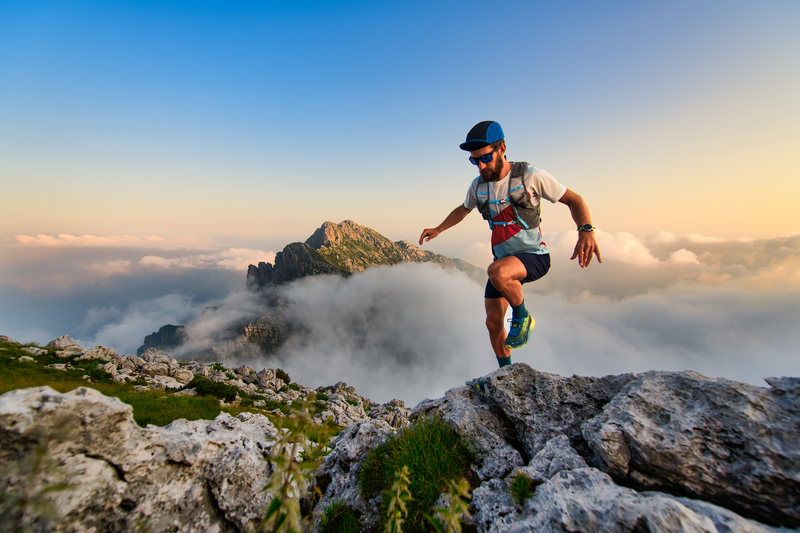
95% of researchers rate our articles as excellent or good
Learn more about the work of our research integrity team to safeguard the quality of each article we publish.
Find out more
ORIGINAL RESEARCH article
Front. Plant Sci.
Sec. Sustainable and Intelligent Phytoprotection
Volume 16 - 2025 | doi: 10.3389/fpls.2025.1574920
This article is part of the Research Topic Plant Pest and Disease Model Forecasting: Enhancing Precise and Data-Driven Agricultural Practices View all 10 articles
The final, formatted version of the article will be published soon.
You have multiple emails registered with Frontiers:
Please enter your email address:
If you already have an account, please login
You don't have a Frontiers account ? You can register here
Tea pest and disease detection is crucial in tea plantation management, however, challenges such as multi-target occlusion and complex background impact detection accuracy and efficiency. To address these issues, this paper proposes an improved lightweight model, WMC-RTDETR, based on the RT-DETR model. The model significantly enhances the ability to capture multi-scale features by introducing wavelet transform convolution, improving the feature extraction accuracy in complex backgrounds, and increasing detection efficiency while reducing the number of model parameters. Combined with multiscale multihead self-attention, global feature fusion across scales is realized, which effectively overcomes the shortcomings of traditional attention mechanisms in small target detection. Additionally, a context-guided spatial feature reconstruction feature pyramid network is designed to refine the target feature reconstruction through contextual information, thereby improving the robustness and accuracy of target detection in complex scenes. Experimental results show that the proposed model achieves 97.7% and 83.1% respectively in mAP50 and mAP50:95 indicators, which outperform the original model. In addition, the number of parameters and floating-point operations are reduced by 35.48% and 40.42% respectively, enabling highly efficient and accurate detection of pests and diseases in complex scenarios. Furthermore, this paper successfully deploys the lightweight model on the Raspberry Pi platform, which proves that it has good real-time performance in resourceconstrained embedded environments, providing a practical solution for low-cost disease monitoring in agricultural scenarios.
Keywords: Tea pest and disease detection, RT-DETR, Wavelet Transform, multiscale multihead self-attention, contextual feature reconstruction, embedded deployment
Received: 11 Feb 2025; Accepted: 31 Mar 2025.
Copyright: © 2025 Zhang, Song, Yu and Ji. This is an open-access article distributed under the terms of the Creative Commons Attribution License (CC BY). The use, distribution or reproduction in other forums is permitted, provided the original author(s) or licensor are credited and that the original publication in this journal is cited, in accordance with accepted academic practice. No use, distribution or reproduction is permitted which does not comply with these terms.
* Correspondence:
Jun Song, Nanjing Forestry University, Nanjing, China
Disclaimer: All claims expressed in this article are solely those of the authors and do not necessarily represent those of their affiliated organizations, or those of the publisher, the editors and the reviewers. Any product that may be evaluated in this article or claim that may be made by its manufacturer is not guaranteed or endorsed by the publisher.
Research integrity at Frontiers
Learn more about the work of our research integrity team to safeguard the quality of each article we publish.