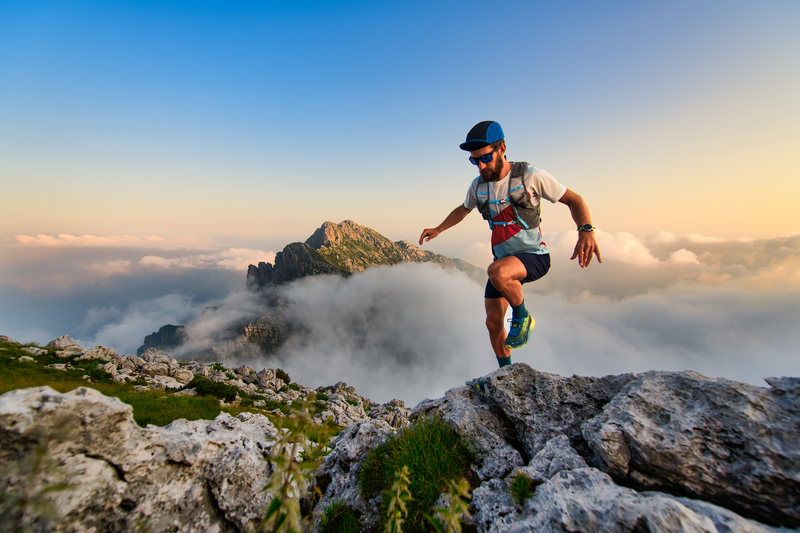
95% of researchers rate our articles as excellent or good
Learn more about the work of our research integrity team to safeguard the quality of each article we publish.
Find out more
ORIGINAL RESEARCH article
Front. Plant Sci.
Sec. Sustainable and Intelligent Phytoprotection
Volume 16 - 2025 | doi: 10.3389/fpls.2025.1565409
This article is part of the Research Topic Machine Vision and Machine Learning for Plant Phenotyping and Precision Agriculture, Volume II View all 20 articles
The final, formatted version of the article will be published soon.
You have multiple emails registered with Frontiers:
Please enter your email address:
If you already have an account, please login
You don't have a Frontiers account ? You can register here
Medicinal plants have been an integral part of traditional medicine, offering diverse therapeutic properties and serves as a foundation for modern drug discovery. However, the traditional ways of identification and classification of these plants rely heavily on expert knowledge. This process is often time-consuming and prone to errors. The proposed study explores the potential of Artificial Intelligence for automatic classification and recognition of medicinal plants using leaf images to address the shortcomings of the traditional approach. 7 state-of-the-art Deep Convolutional Neural Network (DCNN) architectures i.e., MobileNetV2, ResNet50V2, DenseNet169, Hybrid InceptionResNetV2, VGG16, DenseNet201, and Xception have been employed in the study for identification of 10 medicinal plants from leaf images. Indian Medicinal Leaves Image (IMLI) dataset has been used for evaluation of these deep learning architectures on standard evaluation metrics like precision, recall, F1-score, accuracy etc. Additionally, confidence level for each of the architecture is evaluated to know the performance of these models in real-time scenario. The results demonstrate the efficacy of CNN models in achieving high classification accuracy, with ResNet50V2 architecture emerging as the most reliable architectures with average accuracy of 96.93% followed by DenseNet169 with accuracy of 95.38% for identification of Indian Medicinal Plant. The presented research highlights the transformative potential of artificial intelligence in automating medicinal plant classification tasks, paving way for scalable, efficient, and accurate solutions in herbal medicine applications and botanical studies.
Keywords: medicinal plants, Plant identification, deep learning, Hybrid InceptionResNetV2, Tulsi, Botanical studies
Received: 23 Jan 2025; Accepted: 24 Mar 2025.
Copyright: © 2025 Sambasivam, Prabu Kanna, Chauhan, Gandotra and Kumar. This is an open-access article distributed under the terms of the Creative Commons Attribution License (CC BY). The use, distribution or reproduction in other forums is permitted, provided the original author(s) or licensor are credited and that the original publication in this journal is cited, in accordance with accepted academic practice. No use, distribution or reproduction is permitted which does not comply with these terms.
* Correspondence:
G Sambasivam, Xiamen University, Malaysia, Sepang, 43900, Selangor, Malaysia
Yogesh Kumar, Pandit Deendayal Energy University, Gandhinagar, India
Disclaimer: All claims expressed in this article are solely those of the authors and do not necessarily represent those of their affiliated organizations, or those of the publisher, the editors and the reviewers. Any product that may be evaluated in this article or claim that may be made by its manufacturer is not guaranteed or endorsed by the publisher.
Research integrity at Frontiers
Learn more about the work of our research integrity team to safeguard the quality of each article we publish.