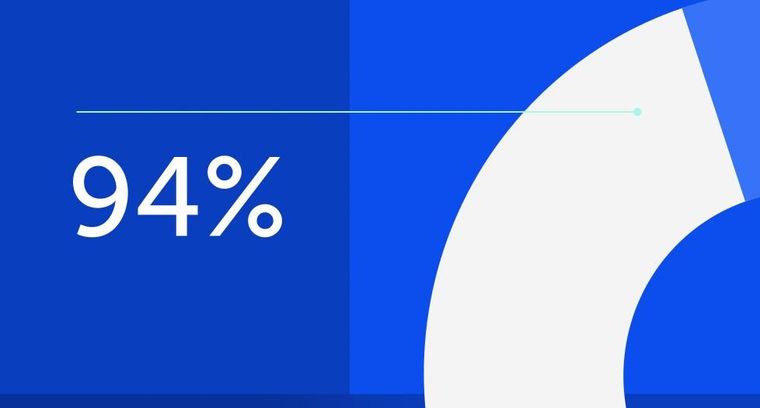
94% of researchers rate our articles as excellent or good
Learn more about the work of our research integrity team to safeguard the quality of each article we publish.
Find out more
ORIGINAL RESEARCH article
Front. Plant Sci.
Sec. Sustainable and Intelligent Phytoprotection
Volume 16 - 2025 | doi: 10.3389/fpls.2025.1565070
This article is part of the Research TopicAdvanced Methods, Equipment and Platforms in Precision Field Crops Protection, Volume IIView all 13 articles
The final, formatted version of the article will be published soon.
Select one of your emails
You have multiple emails registered with Frontiers:
Notify me on publication
Please enter your email address:
If you already have an account, please login
You don't have a Frontiers account ? You can register here
Precision crop protection plays a crucial role in sustainable agriculture by enabling timely and targeted interventions against pests, diseases, and abiotic stressors. However, the effectiveness of data-driven models in this domain is severely limited by the scarcity of annotated images, the imbalanced distribution of rare crop conditions, and the high variability of field environments across time and space. Traditional data augmentation techniques-such as geometric transformations and color jittering-fail to capture the biological diversity and contextual complexity required for robust model generalization. Furthermore, conventional generative approaches are often detached from the underlying spatiotemporal dynamics and domainspecific agronomic knowledge. To address these challenges, we propose a novel generative framework that combines a Spatial-Temporal Adversarial Generation Network (ST-AGN) with an Adaptive Domain-Aware Synthesis Strategy (ADASS). The ST-AGN generates high-resolution, biologically plausible images conditioned on crop status, environmental attributes, and field location. ADASS dynamically selects and integrates synthetic samples based on domain coverage, task uncertainty, and feedback from real-time monitoring. This combination enables targeted simulation of rare or underrepresented agricultural scenarios and improves the generalization ability of downstream detection and prediction models. Experimental results on four public agricultural datasets demonstrate that our framework outperforms six state-of-the-art generative models across multiple evaluation metrics, including FID, Inception Score, and task-level precision and recall. The method not only enhances synthetic image quality but also significantly boosts performance in crop disease detection, stress classification, and resource optimization tasks. This research highlights the potential of domain-aware generative modeling as a scalable and adaptive solution to data scarcity in precision agriculture, paving the way for more intelligent, efficient, and sustainable crop protection systems.
Keywords: Generative Adversarial Networks, precision agriculture, Synthetic image generation, Spatiotemporal modeling, crop protection
Received: 22 Jan 2025; Accepted: 18 Apr 2025.
Copyright: © 2025 Xiang. This is an open-access article distributed under the terms of the Creative Commons Attribution License (CC BY). The use, distribution or reproduction in other forums is permitted, provided the original author(s) or licensor are credited and that the original publication in this journal is cited, in accordance with accepted academic practice. No use, distribution or reproduction is permitted which does not comply with these terms.
* Correspondence: Guangfang Xiang, Pingdingshan University, Pingdingshan, China
Disclaimer: All claims expressed in this article are solely those of the authors and do not necessarily represent those of their affiliated organizations, or those of the publisher, the editors and the reviewers. Any product that may be evaluated in this article or claim that may be made by its manufacturer is not guaranteed or endorsed by the publisher.
Supplementary Material
Research integrity at Frontiers
Learn more about the work of our research integrity team to safeguard the quality of each article we publish.