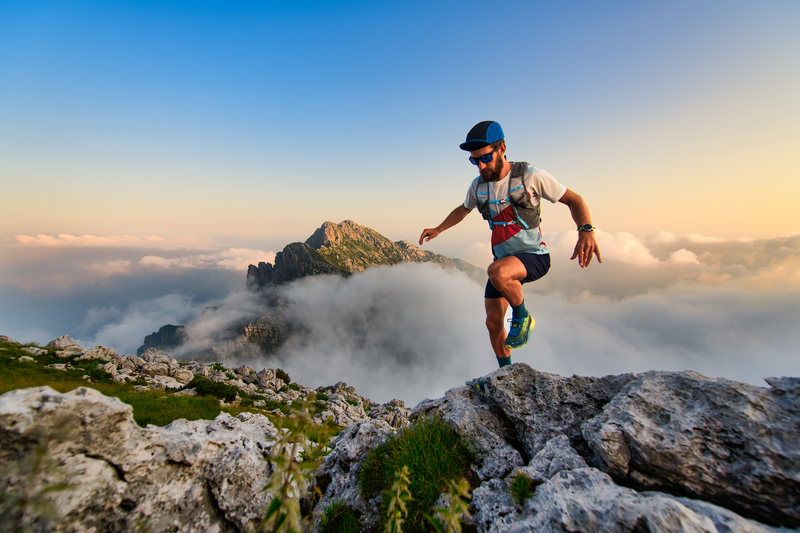
94% of researchers rate our articles as excellent or good
Learn more about the work of our research integrity team to safeguard the quality of each article we publish.
Find out more
ORIGINAL RESEARCH article
Front. Plant Sci.
Sec. Sustainable and Intelligent Phytoprotection
Volume 16 - 2025 | doi: 10.3389/fpls.2025.1564301
This article is part of the Research Topic Precision Information Identification and Integrated Control: Pest Identification, Crop Health Monitoring, and Field Management View all 12 articles
The final, formatted version of the article will be published soon.
You have multiple emails registered with Frontiers:
Please enter your email address:
If you already have an account, please login
You don't have a Frontiers account ? You can register here
Growing consumer demand for high-quality strawberries has highlighted the need for accurate, efficient, and non-destructive methods to assess key postharvest quality traits, such as weight, size uniformity, and quantity. This study proposes a multi-objective learning algorithm that leverages RGB-D multimodal information to estimate these quality metrics. The algorithm develops a fusion expert network architecture that maximizes the use of multimodal features while preserving the distinct details of each modality. Additionally, a novel Heritable Loss function is implemented to reduce redundancy and enhance model performance. Experimental results show that the coefficient of determination (R² ) values for weight, size uniformity and number are 0.94, 0.90 and 0.95 respectively. Ablation studies demonstrate the advantage of the architecture in multimodal, multi-task prediction accuracy. Compared to single-modality models, non-fusion branch networks, and attention-enhanced fusion models, our approach achieves enhanced performance across multi-task learning scenarios, providing more precise data for trait assessment and precision strawberry applications.
Keywords: Strawberry quality, fruit traits estimation, Computer Vision, deep learning, RGB-D modality fusion
Received: 21 Jan 2025; Accepted: 20 Feb 2025.
Copyright: © 2025 Cheng, Cheng, Miao, Fang and Gong. This is an open-access article distributed under the terms of the Creative Commons Attribution License (CC BY). The use, distribution or reproduction in other forums is permitted, provided the original author(s) or licensor are credited and that the original publication in this journal is cited, in accordance with accepted academic practice. No use, distribution or reproduction is permitted which does not comply with these terms.
* Correspondence:
Yifan Cheng, Huazhong University of Science and Technology, Wuhan, China
Disclaimer: All claims expressed in this article are solely those of the authors and do not necessarily represent those of their affiliated organizations, or those of the publisher, the editors and the reviewers. Any product that may be evaluated in this article or claim that may be made by its manufacturer is not guaranteed or endorsed by the publisher.
Research integrity at Frontiers
Learn more about the work of our research integrity team to safeguard the quality of each article we publish.