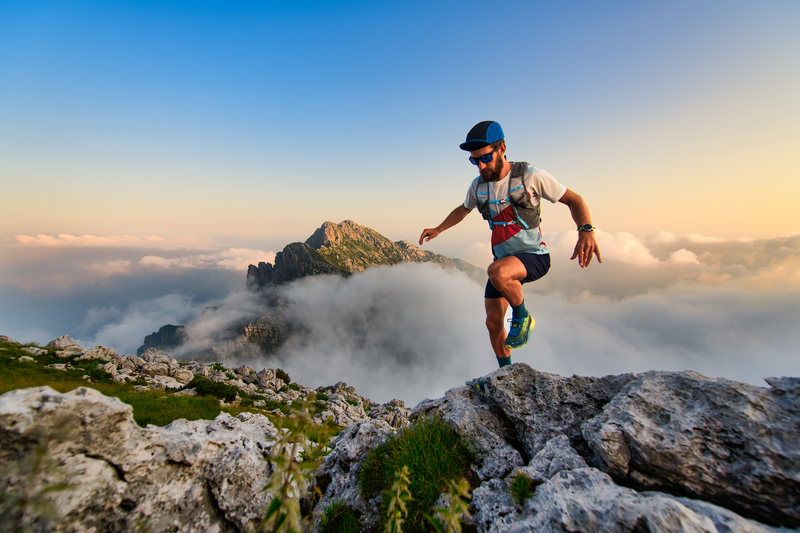
95% of researchers rate our articles as excellent or good
Learn more about the work of our research integrity team to safeguard the quality of each article we publish.
Find out more
ORIGINAL RESEARCH article
Front. Plant Sci.
Sec. Technical Advances in Plant Science
Volume 16 - 2025 | doi: 10.3389/fpls.2025.1563551
This article is part of the Research Topic Machine Vision and Machine Learning for Plant Phenotyping and Precision Agriculture, Volume II View all 20 articles
The final, formatted version of the article will be published soon.
You have multiple emails registered with Frontiers:
Please enter your email address:
If you already have an account, please login
You don't have a Frontiers account ? You can register here
Accurate assessment of the planting effect is crucial during the potato cultivation process. Currently, manual statistical methods are inefficient and challenging to evaluate in real-time. To address this issue, this study proposes a detection algorithm for the potato planting machine’s seed potato scooping scene, based on an improved lightweight YOLO v5n model. Initially, the C3-Faster module is introduced, which reduces the number of parameters and computational load while maintaining detection accuracy. Subsequently, re-parameterized convolution (RepConv) is incorporated into the feature extraction network architecture, enhancing the model’s inference speed by leveraging the correlation between features. Finally, to further improve the efficiency of the model for mobile applications, layer-adaptive magnitude-based pruning (LAMP) technology is employed to eliminate redundant channels with minimal impact on performance. The experimental results indicate that: 1) The improved YOLOv5n model exhibits a 56.8\% reduction in parameters, a 56.1\% decrease in giga floating point operations per second (GFLOPs), a 51.4\% reduction in model size, and a 37.0\% reduction in Embedded Device Inference Time compared to the YOLOv5n model. Additionally, the mean average precision (mAP) at mAP@0.5 achieves up to 98.0\%. 2) Compared with the YOLO series model, mAP@0.5 is close, and the parameters, GFLOPs, and model size are significantly decreased. 3) Combining the ByteTrack algorithm and counting method, the accuracy of counting reaches 96.6\%. Based on these improvements, we designed a potato precision planter metering system that supports real-time monitoring of omission, replanting, and qualified casting during the planting process. This system provides effective support for potato precision planting and offers a visual representation of the planting outcomes, demonstrating its practical value for the industry.
Keywords: Potato, object detection, Lightweight, multiple object tracking, YOLOv5n
Received: 20 Jan 2025; Accepted: 25 Mar 2025.
Copyright: © 2025 Xiao, Song, Li, Liao, Pu and Du. This is an open-access article distributed under the terms of the Creative Commons Attribution License (CC BY). The use, distribution or reproduction in other forums is permitted, provided the original author(s) or licensor are credited and that the original publication in this journal is cited, in accordance with accepted academic practice. No use, distribution or reproduction is permitted which does not comply with these terms.
* Correspondence:
Changlin Song, School of Mechanical Engineering, Xihua University, Sichuan, 610039, Sichuan Province, China
Disclaimer: All claims expressed in this article are solely those of the authors and do not necessarily represent those of their affiliated organizations, or those of the publisher, the editors and the reviewers. Any product that may be evaluated in this article or claim that may be made by its manufacturer is not guaranteed or endorsed by the publisher.
Research integrity at Frontiers
Learn more about the work of our research integrity team to safeguard the quality of each article we publish.