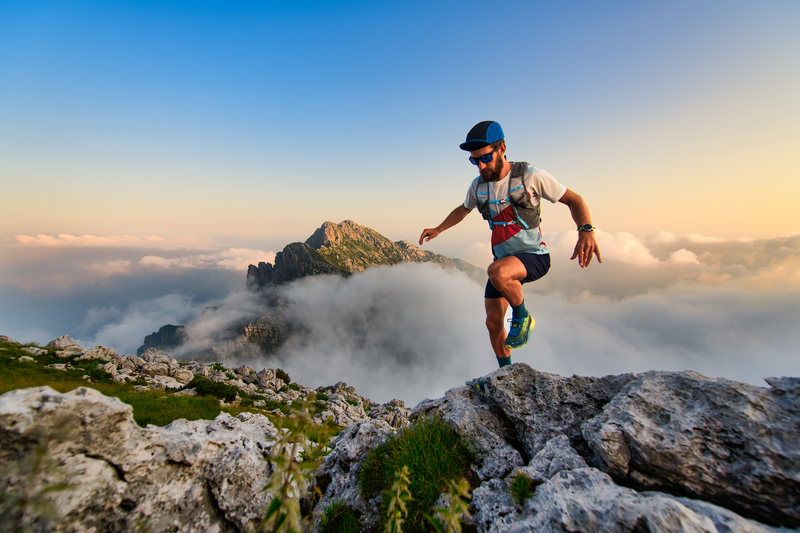
95% of researchers rate our articles as excellent or good
Learn more about the work of our research integrity team to safeguard the quality of each article we publish.
Find out more
ORIGINAL RESEARCH article
Front. Plant Sci.
Sec. Plant Breeding
Volume 16 - 2025 | doi: 10.3389/fpls.2025.1553514
The final, formatted version of the article will be published soon.
You have multiple emails registered with Frontiers:
Please enter your email address:
If you already have an account, please login
You don't have a Frontiers account ? You can register here
Upland cotton (Gossypium hirsutum) faces the challenge of limited genetic diversity in the elite or improved gene pool. To address this issue, we explored alleles contributed by five 'converted' exotic lines sampling most of the undomesticated botanical races of G. hirsutum, in BC1F2 and F3 populations. Joint analysis of all populations along with population-specific analyses identified 38 unique QTL for six different fiber quality traits. At 15 of these loci, DES56 or the elite allele improved upon all the exotics. For another 15, only a single of the five exotics improved upon the elite allele, suggesting the rare alleles that may not have been sampled in the cotton domestication or improvement. At the remaining 8 QTL, multiple exotic lines contributed the superior allele, suggesting that DES56 (and by extension the elite gene pool) has chronically poor alleles at these loci. Converted strains T1046, T326, and T063 showed the highest potential for contributions to cotton fiber quality breeding programs. Upper Half Mean Length and Fiber Strength showed multiple QTL regions affecting both traits simultaneously, while the Uniformity Index showed the smallest heritability values. The estimation of pairwise genetic distances for six parental lines indicates that DES56 has a higher genetic similarity with each exotic line than the exotic lines have with each other. Most of the detected QTL were 'minor' (explaining less than 10% of variance) supporting the implementation of genomic selection techniques to utilize the cumulative effects of most of these QTL distributed genome-wide. Finally, some regions were consistently unfavorable for exotic introgression such as on chromosomes A13 and D09, indicating the possible genomewide haplotypes that may combine the benefits of a history of scientific breeding of the elite gene pool.
Keywords: Gossypium hirsutum, Exotic lines, GBS, QTL mapping, Joint linkage association mapping, Fiber quality, Upland Cotton Abbreviations-SNP-Single Nucleotide Polymorphism, DNA-deoxyribonucleic acid
Received: 30 Dec 2024; Accepted: 24 Mar 2025.
Copyright: © 2025 Mangla, Liu, Vitrakoti, Somala, Shehzad, Chandnani, Das, Wallace, Snider, Jones, Chee and Paterson. This is an open-access article distributed under the terms of the Creative Commons Attribution License (CC BY). The use, distribution or reproduction in other forums is permitted, provided the original author(s) or licensor are credited and that the original publication in this journal is cited, in accordance with accepted academic practice. No use, distribution or reproduction is permitted which does not comply with these terms.
* Correspondence:
Andrew H Paterson, University of Georgia, Athens, United States
Disclaimer: All claims expressed in this article are solely those of the authors and do not necessarily represent those of their affiliated organizations, or those of the publisher, the editors and the reviewers. Any product that may be evaluated in this article or claim that may be made by its manufacturer is not guaranteed or endorsed by the publisher.
Research integrity at Frontiers
Learn more about the work of our research integrity team to safeguard the quality of each article we publish.