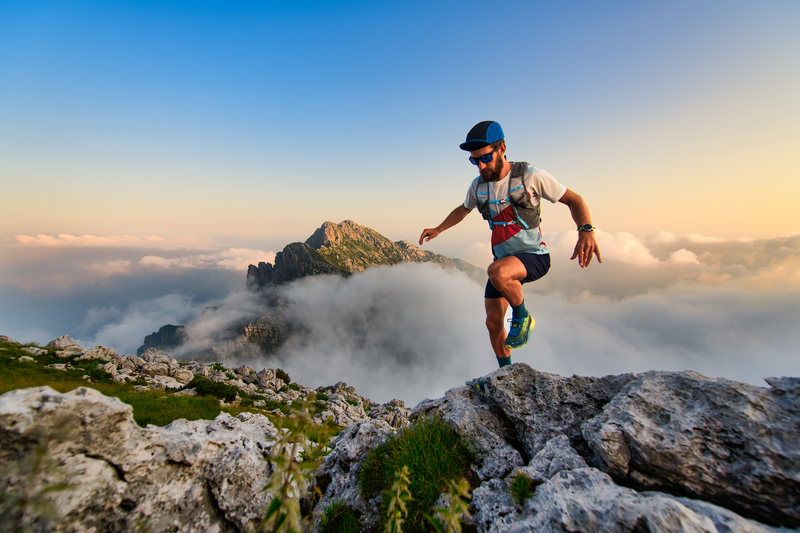
94% of researchers rate our articles as excellent or good
Learn more about the work of our research integrity team to safeguard the quality of each article we publish.
Find out more
ORIGINAL RESEARCH article
Front. Plant Sci.
Sec. Plant Breeding
Volume 16 - 2025 | doi: 10.3389/fpls.2025.1551940
This article is part of the Research Topic Advances in Genomics of Plant Pathogens and Host-Pathogen Interaction View all articles
The final, formatted version of the article will be published soon.
You have multiple emails registered with Frontiers:
Please enter your email address:
If you already have an account, please login
You don't have a Frontiers account ? You can register here
Maize is one of the versatile and commercially produced crop used for food, feed, fodder, ethanol, oil, and industrial raw materials. Eventually, maize is affected by various diseases but among those, maydis leaf blight (MLB), is one of the serious diseases caused by Cochliobolus heterostrophus and responsible for yield losses up to 40%. While developing cultivars for a specific ecology, days to flowering and maturity are also important breeding traits to consider. Thus, understanding the genetic basis of MLB resistance specifically "O" race of the pathogen and maturity related traits is crucial to develop climate-resilient maize hybrids. This study aimed to determine the gene actions and their interactions for the MLB resistance and maturity related traits using six parameter model generation mean analysis (P1, P2, F1, BC1P1, BC1P2, and F2). Five experimental crosses were attended using resistant (R) (CML269-1, and P72c1 and Xbrasil1177-2) and susceptible (S) (HKIPC4B and ESM113) lines in R×S (1), S×R (2), R×R (1), and S×S (1) combinations. The susceptible lines were belonging to early (HKIPC4B) to medium (ESM113) maturity while resistant were of, medium (CML269-1) to late (P72c1XXbrasil1177-2) maturity group. These six genetic populations were screened under artificially created epiphytotic conditions at hot-spots site. In the analysis, MLB resistance showed a dominance genetic effect with a significant (P<0.01) additive × additive interactions. Maturity-related traits showed significant dominance genetic effects (P < 0.01), with dominance × dominance interactions, suggesting suitability of hybrid breeding for these traits. The estimated genes responsible for MLB resistance ranged from 0.002 to 5.78 per cross. In MLB resistance, broad and narrow sense heritability were found to be 91.9 and 84.3 percent, respectively, which indicated the possibility Formatted: Font: Not Italic, Complex Script Font: Not Italic of genetic improvement through selection. Disease response and maturity related traits were negatively correlated, suggesting that long duration genotypes are more resistant to disease than short duration. The detailed understating of gene actions can aid in designing breeding strategies to develop resistant cultivars with required duration for various stress prone ecologies.
Keywords: gGeneration mean analysis, hHeritability, rResistance, sSusceptible and Maydis leaf blightcorrelation 1. INTRODUCTION Font: Italic, Complex Script Font: Italic Font: Italic, Complex Script Font: Italic Formatted: Normal (Web) Font: Italic, Complex Script Font: Italic
Received: 26 Dec 2024; Accepted: 28 Feb 2025.
Copyright: © 2025 Kumar, -rakhi7272@gmail.com, Kumar, Kumar, Singh, Yankanchi, Sarkar, Nath, Jat, Bagaria, Aggarwal, Piyal, Singh, Rakshit and Jat. This is an open-access article distributed under the terms of the Creative Commons Attribution License (CC BY). The use, distribution or reproduction in other forums is permitted, provided the original author(s) or licensor are credited and that the original publication in this journal is cited, in accordance with accepted academic practice. No use, distribution or reproduction is permitted which does not comply with these terms.
* Correspondence:
Pardeep - Kumar, ICAR-Indian Institute of Maize Research, Ludhiana, India
Disclaimer: All claims expressed in this article are solely those of the authors and do not necessarily represent those of their affiliated organizations, or those of the publisher, the editors and the reviewers. Any product that may be evaluated in this article or claim that may be made by its manufacturer is not guaranteed or endorsed by the publisher.
Research integrity at Frontiers
Learn more about the work of our research integrity team to safeguard the quality of each article we publish.