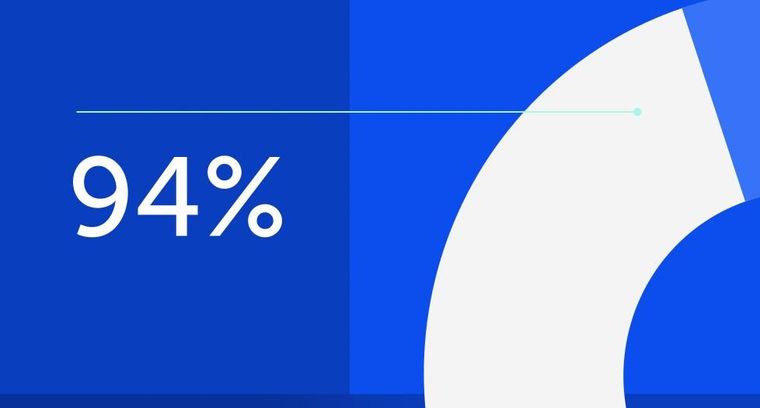
94% of researchers rate our articles as excellent or good
Learn more about the work of our research integrity team to safeguard the quality of each article we publish.
Find out more
ORIGINAL RESEARCH article
Front. Plant Sci.
Sec. Plant Biotechnology
Volume 16 - 2025 | doi: 10.3389/fpls.2025.1550302
This article is part of the Research TopicPlant-Based Solutions for Sustainable Agriculture and Environmental RemediationView all 3 articles
The final, formatted version of the article will be published soon.
Select one of your emails
You have multiple emails registered with Frontiers:
Notify me on publication
Please enter your email address:
If you already have an account, please login
You don't have a Frontiers account ? You can register here
Global climate change and ecological degradation highlight the urgency of dealing with agricultural waste and ecological restoration. Traditional pollutant monitoring and ecological restoration methods face challenges in accuracy and adaptability, especially when dealing with complex environmental data. This paper proposes the Bio-DANN model, which combines biogeochemical models and deep learning techniques to improve the accuracy of pollutant monitoring and ecological restoration prediction. The model uses deep neural networks (DNNs) and attention mechanisms to process multidimensional environmental data in various agricultural and ecological scenarios in real time. Experimental results based on Open Soil Data and NEON datasets show that Bio-DANN performs well in pollutant prediction, with mean square errors (MSE) of 0.012 and 0.018, root mean square errors (RMSE) of 0.109 and 0.134, and accuracy of 0.92 and 0.90, respectively. In terms of ecological restoration assessment, Bio-DANN achieved ∆F and PIPGR of 0.15 and 18%, and 0.20 and 22%, respectively, and H' values of 1.5 and 1.7, which are better than other models. Bio-DANN provides a promising technical solution for environmental protection, resource recovery and sustainable agriculture, especially showing significant potential in pollutant monitoring, soil health assessment and ecological restoration evaluation.
Keywords: 3D Reconstruction, Landscape restoration, hybrid method, point cloud, ecological integrity, attention mechanism, Graph networks
Received: 23 Dec 2024; Accepted: 11 Apr 2025.
Copyright: © 2025 Guo, Lin and Xiao. This is an open-access article distributed under the terms of the Creative Commons Attribution License (CC BY). The use, distribution or reproduction in other forums is permitted, provided the original author(s) or licensor are credited and that the original publication in this journal is cited, in accordance with accepted academic practice. No use, distribution or reproduction is permitted which does not comply with these terms.
* Correspondence: Jinsong Guo, Guangdong Ocean University, Zhanjiang, China
Disclaimer: All claims expressed in this article are solely those of the authors and do not necessarily represent those of their affiliated organizations, or those of the publisher, the editors and the reviewers. Any product that may be evaluated in this article or claim that may be made by its manufacturer is not guaranteed or endorsed by the publisher.
Supplementary Material
Research integrity at Frontiers
Learn more about the work of our research integrity team to safeguard the quality of each article we publish.