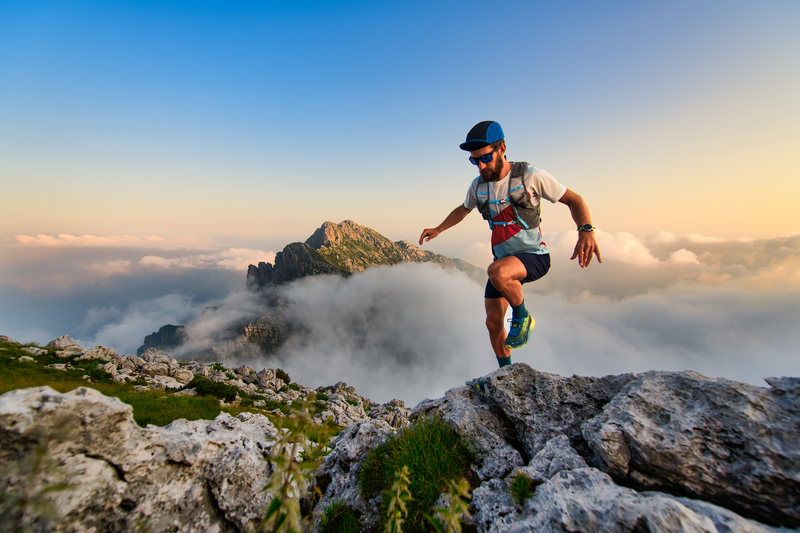
95% of researchers rate our articles as excellent or good
Learn more about the work of our research integrity team to safeguard the quality of each article we publish.
Find out more
ORIGINAL RESEARCH article
Front. Plant Sci.
Sec. Sustainable and Intelligent Phytoprotection
Volume 16 - 2025 | doi: 10.3389/fpls.2025.1548035
This article is part of the Research Topic Precision Information Identification and Integrated Control: Pest Identification, Crop Health Monitoring, and Field Management View all 14 articles
The final, formatted version of the article will be published soon.
You have multiple emails registered with Frontiers:
Please enter your email address:
If you already have an account, please login
You don't have a Frontiers account ? You can register here
Introduction: Accurate and automated yield estimation for red cluster pepper (RCP) is essential to optimize field management and resource allocation. Traditional object detection-based methods for yield estimation often suffer from time-consuming and labor-intensive annotation processes, as well as suboptimal accuracy in dense environments. To address these challenges, this paper proposes a novel multiscale feature enhancement network (MFEN) that integrates a learnable density map (LDM) for accurate RCP yield estimation.The proposed method mainly involves three key steps. First, the kernel-based density map (KDM) method was improved by integrating the Swin Transformer (ST), resulting in LDM method, which produces higher quality density maps. Then, a novel MFEN was developed to improve feature extraction from these density maps. This network combines dilation convolution, residual structures, and an attention mechanism to effectively extract features. Finally, the LDM and the MFEN were jointly trained to estimate both yield and density maps for RCP.The model achieved superior accuracy in RCP yield estimation by using LDM in conjunction with MFEN for joint training. Firstly, the integration of LDM significantly improved the accuracy of the model, with a 0.98% improvement over the previous iteration. Compared to other feature extraction networks, MFEN had the lowest mean absolute error (MAE) of 5.42, root mean square error (RMSE) of 10.37 and symmetric mean absolute percentage error (SMAPE) of 11.64%. It also achieved the highest R-squared (R ² ) value of 0.9802 on the test dataset, beating the best performing DSNet by 0.98%. Notably, despite its multi-column structure, the model has a significant advantage in terms of parameters, with only 13.08M parameters (a reduction of 3.18M compared to the classic single-column network CSRNet). This highlights the model's ability to achieve the highest accuracy while maintaining efficient deployment capabilities.The proposed method provides an robust algorithmic support for efficient and intelligent yield estimation in RCP.
Keywords: Red clustered pepper, Yield estimation, Density map generation, swin transformer, hybrid dilation convolution, Multiscale feature enhancement network
Received: 19 Dec 2024; Accepted: 18 Mar 2025.
Copyright: © 2025 Cheng, Lei, Zhu, Lu, Wang, Tao and Qin. This is an open-access article distributed under the terms of the Creative Commons Attribution License (CC BY). The use, distribution or reproduction in other forums is permitted, provided the original author(s) or licensor are credited and that the original publication in this journal is cited, in accordance with accepted academic practice. No use, distribution or reproduction is permitted which does not comply with these terms.
* Correspondence:
Xinyan Qin, College of Mechanical and Electrical Engineering, Shihezi University, Shihezi, Xinjiang Uyghur Region, China
Disclaimer: All claims expressed in this article are solely those of the authors and do not necessarily represent those of their affiliated organizations, or those of the publisher, the editors and the reviewers. Any product that may be evaluated in this article or claim that may be made by its manufacturer is not guaranteed or endorsed by the publisher.
Research integrity at Frontiers
Learn more about the work of our research integrity team to safeguard the quality of each article we publish.