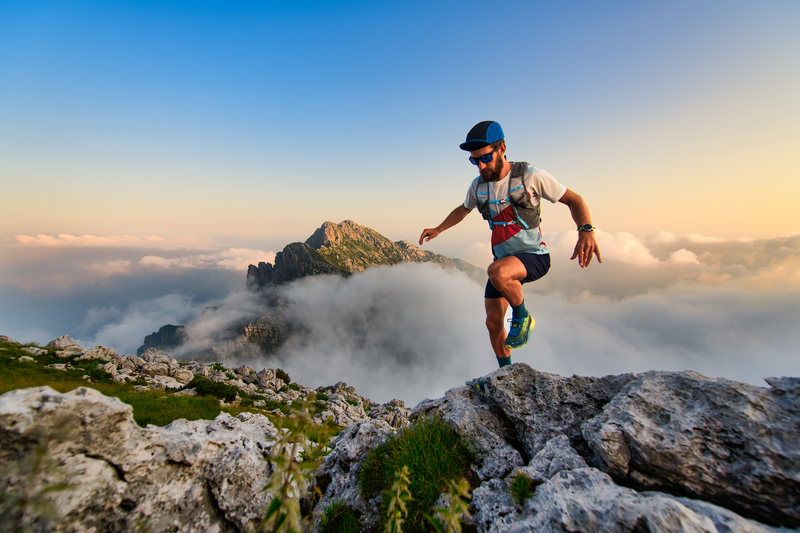
94% of researchers rate our articles as excellent or good
Learn more about the work of our research integrity team to safeguard the quality of each article we publish.
Find out more
ORIGINAL RESEARCH article
Front. Plant Sci.
Sec. Sustainable and Intelligent Phytoprotection
Volume 16 - 2025 | doi: 10.3389/fpls.2025.1545875
This article is part of the Research Topic Precision Information Identification and Integrated Control: Pest Identification, Crop Health Monitoring, and Field Management View all 10 articles
The final, formatted version of the article will be published soon.
You have multiple emails registered with Frontiers:
Please enter your email address:
If you already have an account, please login
You don't have a Frontiers account ? You can register here
Automated detection of apple leaf diseases is crucial for predicting and preventing losses and for enhancing apple yields.However, in complex natural environments, factors such as light variations, shading from branches and leaves, and overlapping disease spots often result in reduced accuracy in detecting apple diseases.To address the challenges of detecting small-target diseases on apple leaves in complex backgrounds and difficulty in mobile deployment, we propose an enhanced lightweight model, ELM-YOLOv8n.To mitigate the high consumption of computational resources in real-time deployment of existing models, we integrate the Fasternet Block into the C2f of the backbone network and neck network, effectively reducing the parameter count and the computational load of the model.In order to enhance the network's anti-interference ability in complex backgrounds and its capacity to differentiate between similar diseases, we incorporate an Efficient Multi-Scale Attention (EMA) within the deep structure of the network for in-depth feature extraction.Additionally, we design a detail-enhanced shared convolutional scaling detection head (DESCS-DH) to enable the model to effectively capture edge information of diseases and address issues such as poor performance in object detection across different scales.Finally, we employ the NWD loss function to replace the CIoU loss function, allowing the model to locate and identify small targets more accurately and further enhance its robustness, thereby facilitating rapid and precise identification of apple leaf diseases.Experimental results demonstrate ELM-YOLOv8n's effectiveness, achieving 94.0% of F1 value and 96.7% of mAP50 value-a significant improvement over YOLOv8n. Furthermore, the parameter count and computational load are reduced by 44.8% and 39.5%, respectively.The ELM-YOLOv8n model is better suited for deployment on mobile devices while maintaining high accuracy.
Keywords: Orchard environments, Disease detection, deep learning, ELM-YOLOv8n, DESCS-DH
Received: 16 Dec 2024; Accepted: 12 Feb 2025.
Copyright: © 2025 Wang, Sang, Xu, Gao, Han and Liu. This is an open-access article distributed under the terms of the Creative Commons Attribution License (CC BY). The use, distribution or reproduction in other forums is permitted, provided the original author(s) or licensor are credited and that the original publication in this journal is cited, in accordance with accepted academic practice. No use, distribution or reproduction is permitted which does not comply with these terms.
* Correspondence:
Wenjie Sang, College of Intelligent Equipment, Shandong University of Science and Technology, Taian, China
Disclaimer: All claims expressed in this article are solely those of the authors and do not necessarily represent those of their affiliated organizations, or those of the publisher, the editors and the reviewers. Any product that may be evaluated in this article or claim that may be made by its manufacturer is not guaranteed or endorsed by the publisher.
Research integrity at Frontiers
Learn more about the work of our research integrity team to safeguard the quality of each article we publish.