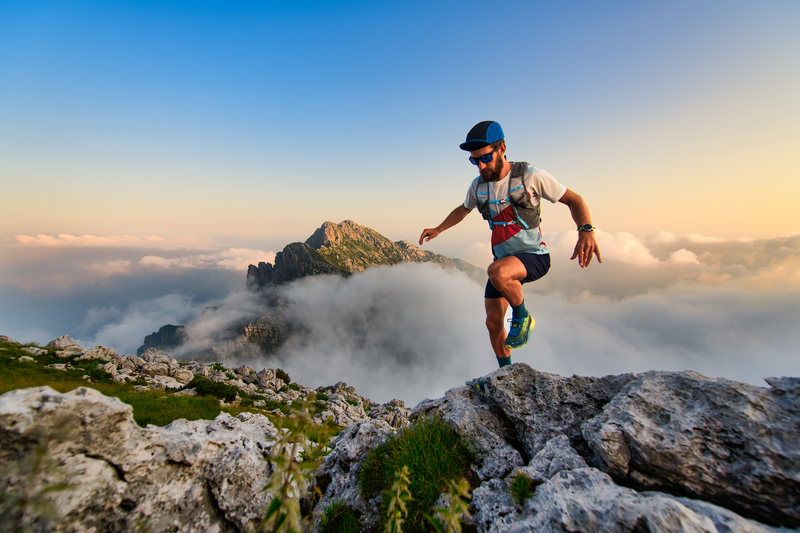
95% of researchers rate our articles as excellent or good
Learn more about the work of our research integrity team to safeguard the quality of each article we publish.
Find out more
ORIGINAL RESEARCH article
Front. Plant Sci.
Sec. Sustainable and Intelligent Phytoprotection
Volume 16 - 2025 | doi: 10.3389/fpls.2025.1545216
The final, formatted version of the article will be published soon.
You have multiple emails registered with Frontiers:
Please enter your email address:
If you already have an account, please login
You don't have a Frontiers account ? You can register here
With the continuous advancement of modern agricultural technologies, the demand for precision fruit-picking techniques has been increasing. This study addresses the challenge of accurate recognition and harvesting of winter peaches by proposing a novel recognition model based on the residual network (ResNet) architecture—WinterPeachNet—aimed at enhancing the accuracy and efficiency of winter peach detection, even in resource-constrained environments. The WinterPeachNet model achieves a comprehensive improvement in network performance by integrating depthwise separable inverted bottleneck ResNet (DIBResNet), bidirectional feature pyramid network (BiFPN) structure, GhostConv module, and the YOLOv11 detection head (v11detect). The DIBResNet module, based on the ResNet architecture, introduces an inverted bottleneck structure and depthwise separable convolution technology, enhancing the depth and quality of feature extraction while effectively reducing the model's computational complexity. The GhostConv module further improves detection accuracy by reducing the number of convolution kernels. Additionally, the BiFPN structure strengthens the model’s ability to detect objects of different sizes by fusing multi-scale feature information. The introduction of v11detect further optimizes object localization accuracy. The results show that the WinterPeachNet model achieves excellent performance in the winter peach detection task, with P = 0.996, R = 0.996, mAP50 = 0.995, and mAP50-95 = 0.964, demonstrating the model's efficiency and accuracy in the winter peach detection task. The high efficiency of the WinterPeachNet model makes it highly adaptable in resource-constrained environments, enabling effective object detection at a relatively low computational cost.
Keywords: Resnet, peach, object detection, deep learning, BiFPN
Received: 14 Dec 2024; Accepted: 24 Mar 2025.
Copyright: © 2025 Li, Li, Zhu, Zhang, Liu and Guan. This is an open-access article distributed under the terms of the Creative Commons Attribution License (CC BY). The use, distribution or reproduction in other forums is permitted, provided the original author(s) or licensor are credited and that the original publication in this journal is cited, in accordance with accepted academic practice. No use, distribution or reproduction is permitted which does not comply with these terms.
* Correspondence:
Yan Li, Faculty of Megadata and Computing, Guangdong Baiyun University, Guangzhou, China
Disclaimer: All claims expressed in this article are solely those of the authors and do not necessarily represent those of their affiliated organizations, or those of the publisher, the editors and the reviewers. Any product that may be evaluated in this article or claim that may be made by its manufacturer is not guaranteed or endorsed by the publisher.
Research integrity at Frontiers
Learn more about the work of our research integrity team to safeguard the quality of each article we publish.