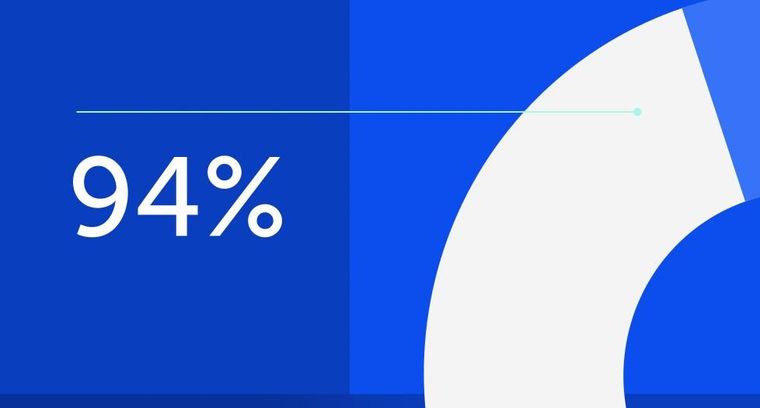
94% of researchers rate our articles as excellent or good
Learn more about the work of our research integrity team to safeguard the quality of each article we publish.
Find out more
ORIGINAL RESEARCH article
Front. Plant Sci.
Sec. Sustainable and Intelligent Phytoprotection
Volume 16 - 2025 | doi: 10.3389/fpls.2025.1543986
This article is part of the Research TopicCutting-Edge Technologies Applications in Intelligent Phytoprotection: From Precision Weed and Pest Detection to Variable Fertilization TechnologiesView all 5 articles
The final, formatted version of the article will be published soon.
Select one of your emails
You have multiple emails registered with Frontiers:
Notify me on publication
Please enter your email address:
If you already have an account, please login
You don't have a Frontiers account ? You can register here
In order to enhance the accuracy of rice leaf disease detection in complex farmland environments, and facilitate the deployment of the deep learning model onto mobile terminals for rapid real-time inference, this paper introduces a disease detection network titled YOLOv11 Multi-scale Dynamic Feature Fusion for Rice Disease Detection (YOLOv11-MSDFF-RiceD). The model adopts the concept of ParameterNet to design the FlexiC3k2Net module, which replaces the neck feature extraction network, thereby bolstering the model's feature learning capabilities without significantly increasing computational complexity. Additionally, an efficient multi-scale feature fusion module (EMFFM) is devised, improving both the computational efficiency and feature extraction capabilities of the model, while simultaneously reducing the number of parameters and memory footprint. The bounding box regression loss function, inner-WIoU, utilizes auxiliary bounding boxes and scale factors. Finally, the Dependency Graph (DepGraph) pruning model is employed to minimize the model's size, computational load, and parameter count, with only a moderate sacrifice in accuracy. Compared to the original YOLOv11nmodel, the optimized model achieves reductions in computational complexity, parameter scale, and memory usage by 50.7%, 49.6%, and 36.9%, respectively, with only a 1.7% improvement in mAP@0.5:0.9. These optimizations enable efficient deployment on resource-constrained mobile devices, making the model highly suitable for real-time disease detection in practical agricultural scenarios where hardware limitations are critical. Consequently, the improved model proposed in this paper effectively detects rice disease targets in complex environments, providing theoretical and technical support for the deployment and application of mobile terminal detection devices, such as rice disease detectors, in practical scenarios.Experimental results demonstrate that, for the disease dataset collected in natural environments, the
Keywords: Inner-WIoU, Rice disease detection, Multi-scale feature fusion, flexiC3k2Net, deep learning
Received: 12 Dec 2024; Accepted: 11 Apr 2025.
Copyright: © 2025 范, 陈 and 李. This is an open-access article distributed under the terms of the Creative Commons Attribution License (CC BY). The use, distribution or reproduction in other forums is permitted, provided the original author(s) or licensor are credited and that the original publication in this journal is cited, in accordance with accepted academic practice. No use, distribution or reproduction is permitted which does not comply with these terms.
* Correspondence:
谦 范, Yangzhou University, Yangzhou, China
斌 李, Yangzhou University, Yangzhou, China
Disclaimer: All claims expressed in this article are solely those of the authors and do not necessarily represent those of their affiliated organizations, or those of the publisher, the editors and the reviewers. Any product that may be evaluated in this article or claim that may be made by its manufacturer is not guaranteed or endorsed by the publisher.
Supplementary Material
Research integrity at Frontiers
Learn more about the work of our research integrity team to safeguard the quality of each article we publish.