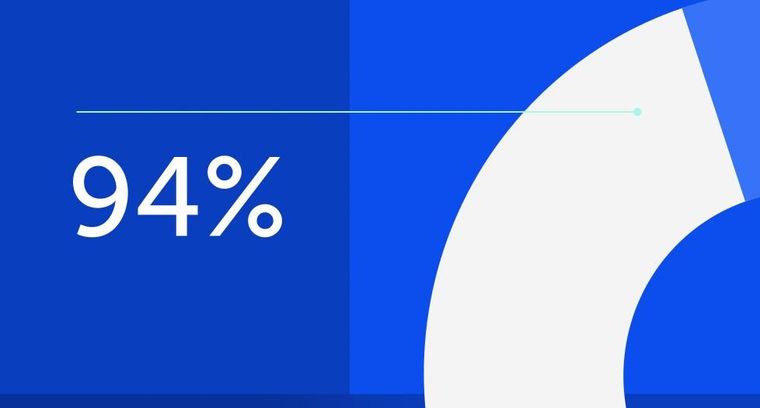
94% of researchers rate our articles as excellent or good
Learn more about the work of our research integrity team to safeguard the quality of each article we publish.
Find out more
ORIGINAL RESEARCH article
Front. Plant Sci.
Sec. Sustainable and Intelligent Phytoprotection
Volume 16 - 2025 | doi: 10.3389/fpls.2025.1541982
This article is part of the Research TopicPlant Pest and Disease Model Forecasting: Enhancing Precise and Data-Driven Agricultural PracticesView all 13 articles
The final, formatted version of the article will be published soon.
Select one of your emails
You have multiple emails registered with Frontiers:
Notify me on publication
Please enter your email address:
If you already have an account, please login
You don't have a Frontiers account ? You can register here
The temporal and spatial irregularity of plant diseases results in insufficient image data for certain diseases, challenging traditional deep learning methods that rely on large amounts of manually annotated data for training. Few-shot learning has emerged as an effective solution to this problem. This paper proposes a method based on the Feature Adaptation Score (FAS) metric, which calculates the FAS for each feature layer in the Swin-TransformerV2 structure. By leveraging the strict positive correlation between FAS scores and test accuracy, we can identify the Swin-Transformer V2-F6 network structure suitable for few-shot plant disease classification without training the network. Furthermore, based on this network structure, we designed the Plant Disease Feature Calibration (PDFC) algorithm, which uses extracted features from the PlantVillage dataset to calibrate features from other datasets. Experiments demonstrate that the combination of the Swin-Transformer V2-F6 network structure and the PDFC algorithm significantly improves the accuracy of few-shot plant disease classification, surpassing existing state-of-the-art models. Our research provides an efficient and accurate solution for few-shot plant disease classification, offering significant practical value.
Keywords: deep learning, Few-shot learning, Plant disease classification, Feature calibration, image classification
Received: 09 Dec 2024; Accepted: 21 Apr 2025.
Copyright: © 2025 Li, Yin, Li, Zhang and Xu. This is an open-access article distributed under the terms of the Creative Commons Attribution License (CC BY). The use, distribution or reproduction in other forums is permitted, provided the original author(s) or licensor are credited and that the original publication in this journal is cited, in accordance with accepted academic practice. No use, distribution or reproduction is permitted which does not comply with these terms.
* Correspondence: Zhendong Yin, Harbin Institute of Technology, Harbin, China
Disclaimer: All claims expressed in this article are solely those of the authors and do not necessarily represent those of their affiliated organizations, or those of the publisher, the editors and the reviewers. Any product that may be evaluated in this article or claim that may be made by its manufacturer is not guaranteed or endorsed by the publisher.
Supplementary Material
Research integrity at Frontiers
Learn more about the work of our research integrity team to safeguard the quality of each article we publish.