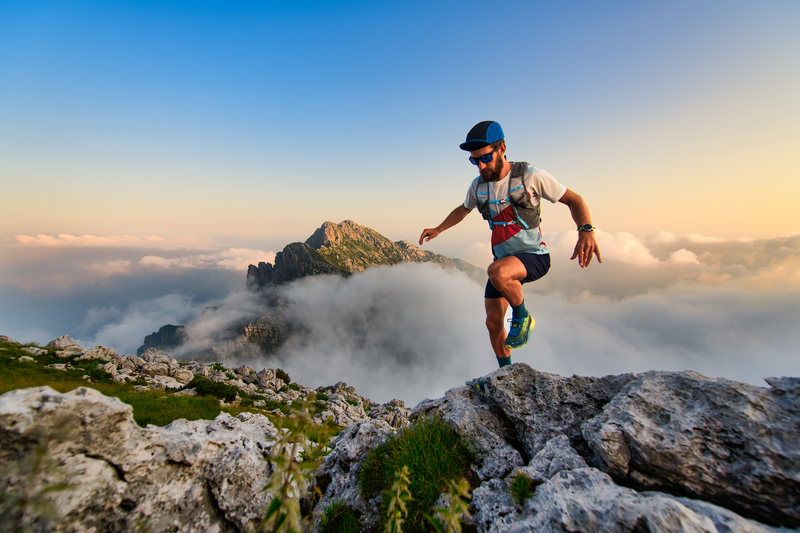
94% of researchers rate our articles as excellent or good
Learn more about the work of our research integrity team to safeguard the quality of each article we publish.
Find out more
ORIGINAL RESEARCH article
Front. Plant Sci.
Sec. Technical Advances in Plant Science
Volume 16 - 2025 | doi: 10.3389/fpls.2025.1541266
This article is part of the Research Topic Machine Vision and Machine Learning for Plant Phenotyping and Precision Agriculture, Volume II View all 7 articles
The final, formatted version of the article will be published soon.
You have multiple emails registered with Frontiers:
Please enter your email address:
If you already have an account, please login
You don't have a Frontiers account ? You can register here
The timely and accurate detection of apple flowers is crucial for assessing the growth status of fruit trees, predicting peak blooming dates, and early estimating apple yields. However, challenges such as variable lighting conditions, complex growth environments, occlusion of apple flowers, clustered flowers and significant morphological variations, impede precise detection. To overcome these challenges, an improved YO-AFD method based on YOLOv8 for apple flower detection was proposed. First, to enable adaptive focus on features across different scales, a new attention module, ISAT, which integrated the Inverted Residual Mobile Block (IRMB) with the Spatial and Channel Synergistic Attention (SCSA) module was designed. This module was then incorporated into the C2f module within the network's neck, forming the C2f-IS module, to enhance the model's ability to extract critical features and fuse features across scales. Additionally, to balance attention between simple and challenging targets, a regression loss function based on Focaler Intersection over Union (FIoU) was used for loss function calculation. Experimental results showed that the YO-AFD model accurately detected both simple and challenging apple flowers, including small, occluded, and morphologically diverse flowers. The YO-AFD model achieved an F1 score of 88.6%, mAP50 of 94.1%, and mAP50-95 of 55.3%, with a model size of 6.5 MB and an average detection speed of 5.3 ms per image. The proposed YO-AFD method outperforms five comparative models, demonstrating its effectiveness and accuracy in real-time apple flower detection. With its lightweight design and high accuracy, this method offers a promising solution for developing portable apple flower detection systems.
Keywords: Apple flower, object detection, deep learning, YO-AFD, attention mechanism
Received: 07 Dec 2024; Accepted: 21 Feb 2025.
Copyright: © 2025 Wang, Wang and Song. This is an open-access article distributed under the terms of the Creative Commons Attribution License (CC BY). The use, distribution or reproduction in other forums is permitted, provided the original author(s) or licensor are credited and that the original publication in this journal is cited, in accordance with accepted academic practice. No use, distribution or reproduction is permitted which does not comply with these terms.
* Correspondence:
Bo Wang, Xi'an Jiaotong University, Xi'an, 710049, China
Disclaimer: All claims expressed in this article are solely those of the authors and do not necessarily represent those of their affiliated organizations, or those of the publisher, the editors and the reviewers. Any product that may be evaluated in this article or claim that may be made by its manufacturer is not guaranteed or endorsed by the publisher.
Research integrity at Frontiers
Learn more about the work of our research integrity team to safeguard the quality of each article we publish.