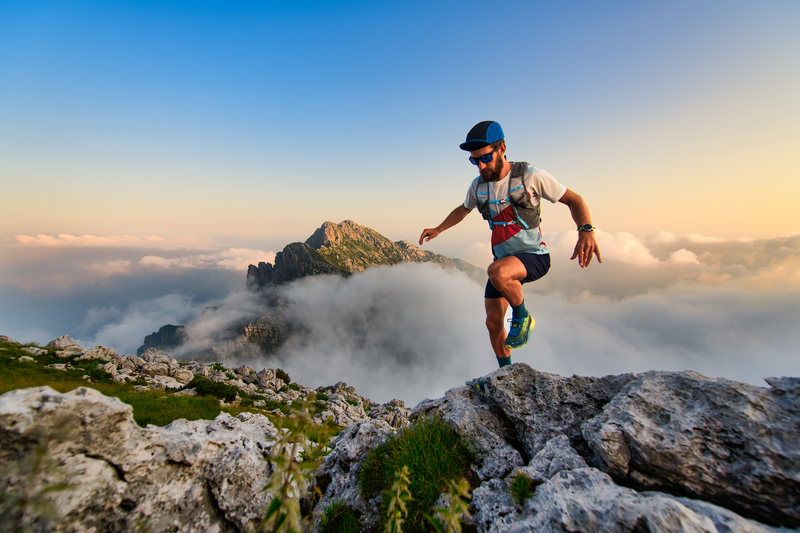
95% of researchers rate our articles as excellent or good
Learn more about the work of our research integrity team to safeguard the quality of each article we publish.
Find out more
ORIGINAL RESEARCH article
Front. Plant Sci.
Sec. Technical Advances in Plant Science
Volume 16 - 2025 | doi: 10.3389/fpls.2025.1536226
This article is part of the Research Topic Machine Vision and Machine Learning for Plant Phenotyping and Precision Agriculture, Volume II View all 21 articles
The final, formatted version of the article will be published soon.
You have multiple emails registered with Frontiers:
Please enter your email address:
If you already have an account, please login
You don't have a Frontiers account ? You can register here
Image instance segmentation is an efficient technique for plant phenotyping. However, the diverse plant types and limited availability of annotated image data in vertical farms limits the effectiveness of traditional supervised segmentation techniques. To overcome these challenges, we propose a zero-shot instance segmentation framework that integrates Grounding DINO with the Segment Anything Model (SAM). We use Vegetation Cover Aware Non-Maximum Suppression (VC-NMS), which incorporates the Normalized Crop Greenness Index (NCGI) to enhance box prompts. Additionally, similarity maps with the max distance criterion are combined to improve point prompts. Experiments show that these enhanced box and point prompts significantly outperform SAM's anything mode and Grounded SAM in zero-shot segmentation.Compared to supervised methods like YOLOv11, our approach exhibits exceptional zero-shot generalization. It achieves the best segmentation performance on two test sets, providing an effective solution to scarce annotation data in vertical farming.
Keywords: Segment Anything, Zero-shot, Instance segmentation, Prompt Augmentation, Foundation models
Received: 28 Nov 2024; Accepted: 07 Apr 2025.
Copyright: © 2025 Bao, Yang, Li and Yang. This is an open-access article distributed under the terms of the Creative Commons Attribution License (CC BY). The use, distribution or reproduction in other forums is permitted, provided the original author(s) or licensor are credited and that the original publication in this journal is cited, in accordance with accepted academic practice. No use, distribution or reproduction is permitted which does not comply with these terms.
* Correspondence:
Haichao Yang, Dali University, Dali, China
Disclaimer: All claims expressed in this article are solely those of the authors and do not necessarily represent those of their affiliated organizations, or those of the publisher, the editors and the reviewers. Any product that may be evaluated in this article or claim that may be made by its manufacturer is not guaranteed or endorsed by the publisher.
Research integrity at Frontiers
Learn more about the work of our research integrity team to safeguard the quality of each article we publish.