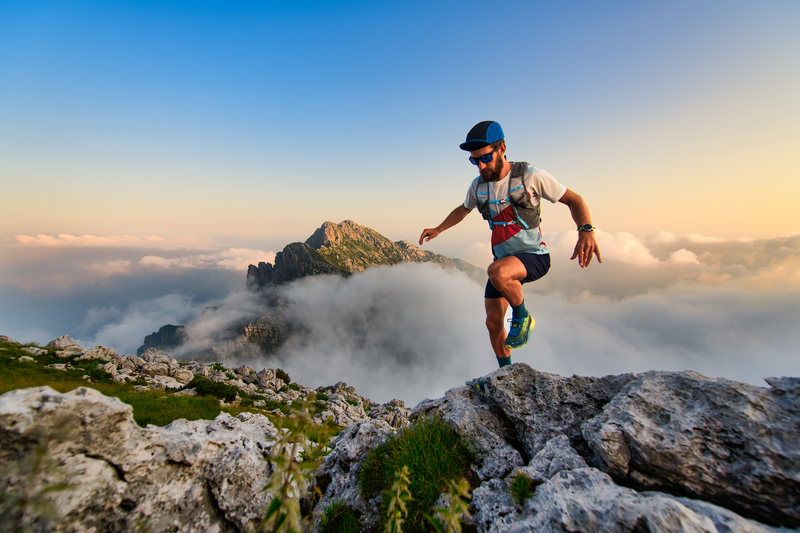
94% of researchers rate our articles as excellent or good
Learn more about the work of our research integrity team to safeguard the quality of each article we publish.
Find out more
ORIGINAL RESEARCH article
Front. Plant Sci.
Sec. Plant Metabolism and Chemodiversity
Volume 16 - 2025 | doi: 10.3389/fpls.2025.1533187
The final, formatted version of the article will be published soon.
You have multiple emails registered with Frontiers:
Please enter your email address:
If you already have an account, please login
You don't have a Frontiers account ? You can register here
Macadamia integrifolia, a perennial evergreen crop valued for its nutritious nuts, also exhibits a diverse range of inflorescence colors that possess both ornamental and biological significance. Despite the economic importance of macadamia, the molecular mechanisms regulating flower coloration remain understudied. This study employed a combination of metabolomic and biochemical approaches to analyze metabolites present in inflorescences from 11 Macadamia cultivars, representing distinct color phenotypes. A total of 787 metabolites were identified through the use of ultra-high-performance liquid chromatography–tandem mass spectrometry (UPLC–MS/MS), the majority of which were phenolic acids, flavonoids, and flavonols. Principal component analysis and clustering yielded a classification of the samples into three major flower color groups. The differential metabolites were found to be enriched in pathways such as flavonoid, flavonol, and phenylpropanoid biosynthesis, which have been demonstrated to be key contributors to color variation. Moreover, weighted gene co-expression network analysis (WGCNA) identified metabolite modules that were strongly associated with specific flower colors. This revealed that key compounds, including kaempferol, quercetin derivatives, and anthocyanins, were the primary drivers of pigmentation. This study provides a comprehensive framework for understanding the genetic, biochemical, and environmental factors influencing macadamia flower color. These findings contribute to the theoretical understanding of macadamia reproductive biology and have practical implications for molecular breeding, ornamental enhancement, and optimizing pollinator attraction to improve crop yield and ecological sustainability.
Keywords: Macadamia integrifolia, Inflorescences Color, Widely targeted metabolomics, WGCNA, Flavonoids
Received: 23 Nov 2024; Accepted: 11 Mar 2025.
Copyright: © 2025 Gong, Zhang, Ma, Li, Li, Wu, Li and Tao. This is an open-access article distributed under the terms of the Creative Commons Attribution License (CC BY). The use, distribution or reproduction in other forums is permitted, provided the original author(s) or licensor are credited and that the original publication in this journal is cited, in accordance with accepted academic practice. No use, distribution or reproduction is permitted which does not comply with these terms.
* Correspondence:
Liang Tao, Yunnan Institute of Tropical Crops, Xishuangbanna, China
Disclaimer: All claims expressed in this article are solely those of the authors and do not necessarily represent those of their affiliated organizations, or those of the publisher, the editors and the reviewers. Any product that may be evaluated in this article or claim that may be made by its manufacturer is not guaranteed or endorsed by the publisher.
Research integrity at Frontiers
Learn more about the work of our research integrity team to safeguard the quality of each article we publish.