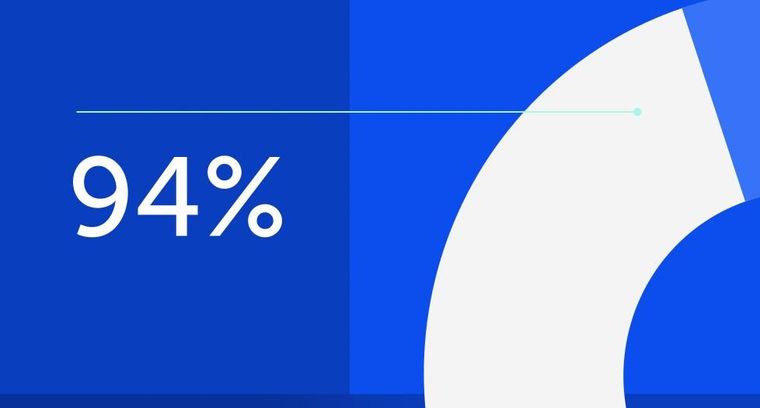
94% of researchers rate our articles as excellent or good
Learn more about the work of our research integrity team to safeguard the quality of each article we publish.
Find out more
ORIGINAL RESEARCH article
Front. Plant Sci.
Sec. Sustainable and Intelligent Phytoprotection
Volume 16 - 2025 | doi: 10.3389/fpls.2025.1532138
This article is part of the Research TopicAccurate Measurement and Dynamic Monitoring of Forest ParametersView all 5 articles
The final, formatted version of the article will be published soon.
Select one of your emails
You have multiple emails registered with Frontiers:
Notify me on publication
Please enter your email address:
If you already have an account, please login
You don't have a Frontiers account ? You can register here
Chinese fir (Cunninghamia lanceolata) is a key afforestation and timber species in southern China, and accurate estimation of its stand biomass is essential for forest resource assessment, ecological industry development, and ecosystem management.However, conventional biomass prediction methods often suffer from limited accuracy and efficiency, necessitating the development of more robust modeling approaches.This study utilized data from 154 forest stands in Guangdong Province to develop biomass regression models incorporating random effects and dummy variables, based on airborne LiDAR-derived metrics. Among the 41 highly correlated LiDAR variables assessed, only two-the 5% cumulative height percentile and the leaf area index-were retained in the final model. The results demonstrated that the logistic mixed-effects model was most effective for estimating leaf biomass, while the empirical mixed-effects model was better suited for other biomass components. Nonlinear models outperformed linear models, with the nonlinear mixed-effects model incorporating stand age as a random effect achieving the highest predictive accuracy. Furthermore, machine learning techniques further enhanced model performance (R²=0.855~0.939). Validation with independent test samples confirmed the reliability and robustness of the nonlinear mixed-effects model. This study highlights the effectiveness of airborne LiDAR data in enabling efficient and precise stand biomass estimation and underscores the significant influence of stand developmental stages on biomass modeling. The findings provide a rigorous and scalable methodological framework for large-scale artificial forest biomass estimation, offering valuable insights for forest resource monitoring, ecological industry development, and ecosystem management strategies.
Keywords: airborne LiDAR, forest biomass, Regression modeling, age grouping, dummy variable modeling
Received: 21 Nov 2024; Accepted: 14 Apr 2025.
Copyright: © 2025 Zhu, Huang, Liu, Bai, Yang, Ye, Wang, Sharma and Fu. This is an open-access article distributed under the terms of the Creative Commons Attribution License (CC BY). The use, distribution or reproduction in other forums is permitted, provided the original author(s) or licensor are credited and that the original publication in this journal is cited, in accordance with accepted academic practice. No use, distribution or reproduction is permitted which does not comply with these terms.
* Correspondence: Liyong Fu, Institute of Forest Resource Information Techniques, Chinese Academy of Forestry, Haidian, China
Disclaimer: All claims expressed in this article are solely those of the authors and do not necessarily represent those of their affiliated organizations, or those of the publisher, the editors and the reviewers. Any product that may be evaluated in this article or claim that may be made by its manufacturer is not guaranteed or endorsed by the publisher.
Supplementary Material
Research integrity at Frontiers
Learn more about the work of our research integrity team to safeguard the quality of each article we publish.