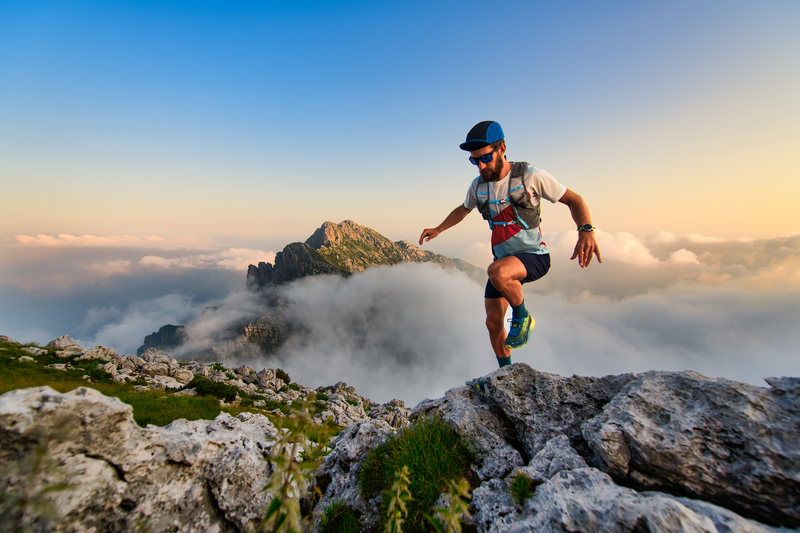
95% of researchers rate our articles as excellent or good
Learn more about the work of our research integrity team to safeguard the quality of each article we publish.
Find out more
ORIGINAL RESEARCH article
Front. Plant Sci.
Sec. Sustainable and Intelligent Phytoprotection
Volume 16 - 2025 | doi: 10.3389/fpls.2025.1530070
This article is part of the Research Topic Machine Vision and Machine Learning for Plant Phenotyping and Precision Agriculture, Volume II View all 21 articles
The final, formatted version of the article will be published soon.
You have multiple emails registered with Frontiers:
Please enter your email address:
If you already have an account, please login
You don't have a Frontiers account ? You can register here
Tomatoes are one of the most economically significant crops worldwide, with their yield and quality heavily impacted by foliar diseases. Effective detection of these diseases is essential for enhancing agricultural productivity and mitigating economic losses. Current tomato leaf disease detection methods, however, encounter challenges in extracting multi-scale features, identifying small targets, and mitigating complex background interference. The multi-scale tomato leaf disease detection model Tomato Focus-Diffusion Network (TomaFDNet) was proposed to solve the above problems. The model utilizes a multi-scale focus-diffusion network (MSFDNet) alongside an efficient parallel multi-scale convolutional module (EPMSC) to significantly enhance the extraction of multi-scale features. This combination particularly strengthens the model's capability to detect small targets amidst complex backgrounds. Experimental results show that TomaFDNet reaches a mean average precision (mAP) of 83.1% in detecting Early_blight, Late_blight, and Leaf_Mold on tomato leaves, outperforming classical object detection algorithms, including Faster R-CNN (mAP = 68.2%) and You Only Look Once (YOLO) series (v5: mAP = 75.5%, v7: mAP = 78.3%, v8: mAP = 78.9%, v9: mAP = 79%, v10: mAP = 77.5%, v11: mAP = 79.2%). Compared to the baseline YOLOv8 model, TomaFDNet achieves a 4.2% improvement in mAP, which is statistically significant (P < 0.01). These findings indicate that TomaFDNet offers a valid solution to the precise detection of tomato leaf diseases.
Keywords: deep learning, MSFDNet, Objection detection, Tomato disease, EPMSC
Received: 18 Nov 2024; Accepted: 31 Mar 2025.
Copyright: © 2025 WANG, Chen, Liang, Mou, Zhang and Hao. This is an open-access article distributed under the terms of the Creative Commons Attribution License (CC BY). The use, distribution or reproduction in other forums is permitted, provided the original author(s) or licensor are credited and that the original publication in this journal is cited, in accordance with accepted academic practice. No use, distribution or reproduction is permitted which does not comply with these terms.
* Correspondence:
Rijun WANG, Guangxi Normal University, Guilin, China
Jin Hao, Guangxi University Engineering Research Center of Agricultural and Forestry Intelligent Equipment Technology, Guilin, China
Disclaimer: All claims expressed in this article are solely those of the authors and do not necessarily represent those of their affiliated organizations, or those of the publisher, the editors and the reviewers. Any product that may be evaluated in this article or claim that may be made by its manufacturer is not guaranteed or endorsed by the publisher.
Research integrity at Frontiers
Learn more about the work of our research integrity team to safeguard the quality of each article we publish.