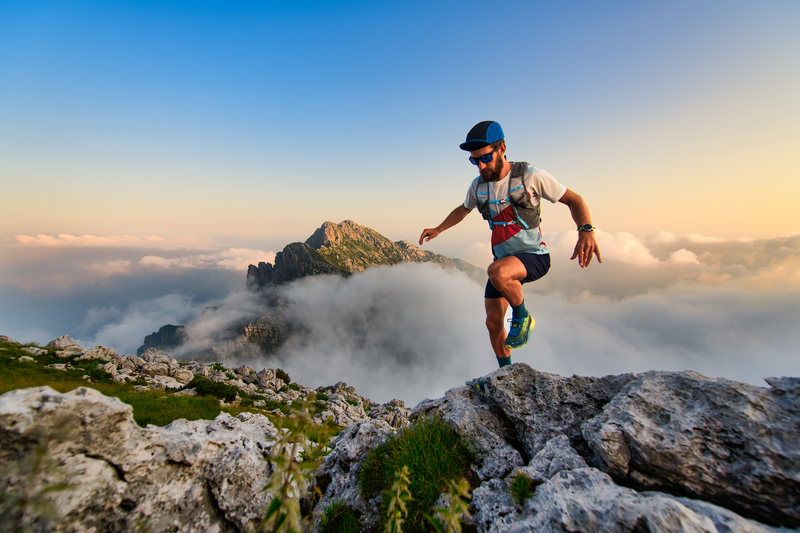
95% of researchers rate our articles as excellent or good
Learn more about the work of our research integrity team to safeguard the quality of each article we publish.
Find out more
METHODS article
Front. Plant Sci.
Sec. Sustainable and Intelligent Phytoprotection
Volume 16 - 2025 | doi: 10.3389/fpls.2025.1524630
This article is part of the Research Topic Machine Vision and Machine Learning for Plant Phenotyping and Precision Agriculture, Volume II View all 20 articles
The final, formatted version of the article will be published soon.
You have multiple emails registered with Frontiers:
Please enter your email address:
If you already have an account, please login
You don't have a Frontiers account ? You can register here
The agricultural sector faces persistent threats from plant diseases and pests, with Tuta absoluta posing a severe risk to tomato farming by causing up to 100% crop loss. Timely pest detection is essential for effective intervention, yet traditional methods remain labor-intensive and inefficient. Recent advancements in deep learning offer promising solutions, with YOLOv8 emerging as a leading real-time detection model due to its speed and accuracy, outperforming previous models in on-field deployment. This study focuses on the early detection of Tuta absoluta-induced tomato leaf diseases in Nigeria, the largest tomato producer in Sub-Saharan Africa. The first major contribution is the annotation of a dataset (TomatoEbola), which consists of 326 images and 784 annotations collected from three Nigerian farms and is now publicly available. The second key contribution is the proposal of a transfer learning-based approach to evaluate YOLOv8's performance in detecting Tuta absoluta. Experimental results highlight the model’s effectiveness, with a mean average precision of up to 0.737, outperforming other state-of-the-art methods that achieve less than 0.69, demonstrating its capability for real-world deployment. These findings suggest that AI-driven solutions like YOLOv8 could play a pivotal role in reducing agricultural losses and enhancing food security.
Keywords: Artificial intelligence in agriculture, Dataset, detection, Tuta absoluta, Tomato leaf diseases, YOLOv8
Received: 07 Nov 2024; Accepted: 31 Mar 2025.
Copyright: © 2025 Shehu, Ackley, Marvellous, Eteng, Sharif and Kusetogullari. This is an open-access article distributed under the terms of the Creative Commons Attribution License (CC BY). The use, distribution or reproduction in other forums is permitted, provided the original author(s) or licensor are credited and that the original publication in this journal is cited, in accordance with accepted academic practice. No use, distribution or reproduction is permitted which does not comply with these terms.
* Correspondence:
Harisu Abdullahi Shehu, Victoria University of Wellington, Wellington, New Zealand
Disclaimer: All claims expressed in this article are solely those of the authors and do not necessarily represent those of their affiliated organizations, or those of the publisher, the editors and the reviewers. Any product that may be evaluated in this article or claim that may be made by its manufacturer is not guaranteed or endorsed by the publisher.
Research integrity at Frontiers
Learn more about the work of our research integrity team to safeguard the quality of each article we publish.