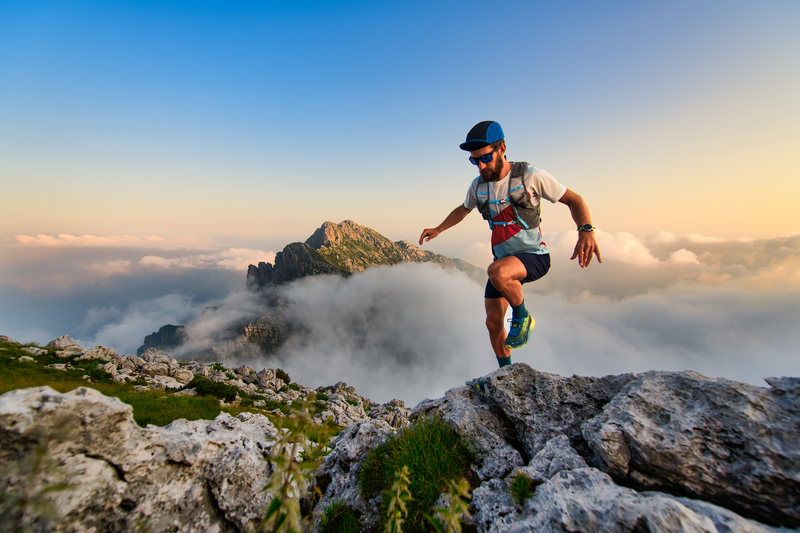
95% of researchers rate our articles as excellent or good
Learn more about the work of our research integrity team to safeguard the quality of each article we publish.
Find out more
METHODS article
Front. Plant Sci.
Sec. Sustainable and Intelligent Phytoprotection
Volume 16 - 2025 | doi: 10.3389/fpls.2025.1523633
The final, formatted version of the article will be published soon.
You have multiple emails registered with Frontiers:
Please enter your email address:
If you already have an account, please login
You don't have a Frontiers account ? You can register here
The rapid and accurate identification of soybean diseases is critical for optimizing both yield and quality. Traditional image recognition techniques face notable limitations in terms of generalization and accuracy, particularly when tasked with identifying small-scale targets or distinguishing diseases with similar characteristics in large, heterogeneous, and complex environments. To address these challenges, this study proposes the YOLOv8-DML model for soybean leaf disease recognition. Building upon YOLOv8n, this model integrates a DWR module that replaces the high-level C2f module with C2f-DWR, enhancing feature extraction across varied receptive fields. Additionally, modifications to the neck structure incorporate a Multi-scale Enhanced Feature Pyramid (MEFP), which improves detection performance across targets of varying sizes by enabling effective multi-scale information fusion. A lightweight detection head (LSCD) is further introduced to facilitate multiscale feature interactions while reducing the overall model parameter count. Lastly, the WIoUv3 loss function is employed to place greater emphasis on small targets and moderate-quality samples, thereby enhancing detection precision. Experimental results demonstrate that YOLOv8-DML achieves a mAP50 of 96.9%, marking a 1.8% improvement over the original YOLOv8 algorithm, while also achieving an 18.6% reduction in parameters. Comparative analysis with other mainstream object detection models indicates that YOLOv8-DML delivers superior overall performance, highlighting its significant potential for effective soybean leaf disease identification.
Keywords: Soybean leaf disease, attention mechanism, Multi-scale features, object detection, YOLOv8
Received: 06 Nov 2024; Accepted: 24 Feb 2025.
Copyright: © 2025 Chen, He, Xu, Lu, Qiu and Yang. This is an open-access article distributed under the terms of the Creative Commons Attribution License (CC BY). The use, distribution or reproduction in other forums is permitted, provided the original author(s) or licensor are credited and that the original publication in this journal is cited, in accordance with accepted academic practice. No use, distribution or reproduction is permitted which does not comply with these terms.
* Correspondence:
Jing Qiu, Yunnan Agricultural University, Kunming, 650201, Yunnan, China
Disclaimer: All claims expressed in this article are solely those of the authors and do not necessarily represent those of their affiliated organizations, or those of the publisher, the editors and the reviewers. Any product that may be evaluated in this article or claim that may be made by its manufacturer is not guaranteed or endorsed by the publisher.
Research integrity at Frontiers
Learn more about the work of our research integrity team to safeguard the quality of each article we publish.