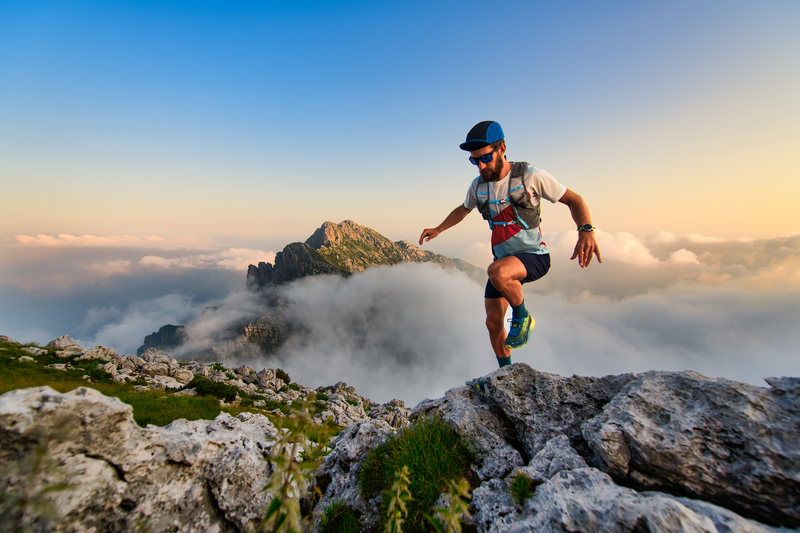
95% of researchers rate our articles as excellent or good
Learn more about the work of our research integrity team to safeguard the quality of each article we publish.
Find out more
ORIGINAL RESEARCH article
Front. Plant Sci.
Sec. Sustainable and Intelligent Phytoprotection
Volume 16 - 2025 | doi: 10.3389/fpls.2025.1515105
This article is part of the Research Topic Plant Pest and Disease Model Forecasting: Enhancing Precise and Data-Driven Agricultural Practices View all 10 articles
The final, formatted version of the article will be published soon.
You have multiple emails registered with Frontiers:
Please enter your email address:
If you already have an account, please login
You don't have a Frontiers account ? You can register here
Pepper leaf segmentation plays a pivotal role in monitoring pepper leaf diseases across diverse backgrounds and ensuring healthy pepper growth. However, existing Transformer-based segmentation methods grapple with computational inefficiency, excessive parameterization, and inadequate utilization of edge information. To address these challenges, this study introduces an Adaptive Multi-Scale MLP (AMS-MLP) framework. This framework integrates the Multi-Path Aggregation Module (MPAM) and the Multi-Scale Context Relation Mask Module (MCRD) to refine object boundaries in pepper leaf segmentation. The AMS-MLP includes an encoder, an Adaptive Multi-Scale MLP (AM-MLP) module, and a decoder. The encoder's MPAM fuses five-scale features for accurate boundary extraction. The AM-MLP splits features into global and local branches, with an adaptive attention mechanism balancing them. The decoder enhances boundary feature extraction using MCRD. To validate the proposed method, extensive experiments were conducted on three pepper leaf datasets with varying backgrounds. Resultsdemonstrate mean Intersection over Union (mIoU) scores of 97.39%, 96.91%, and 97.91%, and F1 scores of 98.29%, 97.86%, and 98.51% across the datasets, respectively. Comparative analysis with U-Net and state-of-the-art models reveals that the proposed method significantly improves the accuracy and efficiency of pepper leaf image segmentation.
Keywords: Pepper leaf segmentation, Multi-scale MLP, Multi-path aggregation module, Context relation mask module, Adaptive attention mechanism
Received: 22 Oct 2024; Accepted: 17 Mar 2025.
Copyright: © 2025 Fang, Liu, Zhang, Hu, Gu and Fu. This is an open-access article distributed under the terms of the Creative Commons Attribution License (CC BY). The use, distribution or reproduction in other forums is permitted, provided the original author(s) or licensor are credited and that the original publication in this journal is cited, in accordance with accepted academic practice. No use, distribution or reproduction is permitted which does not comply with these terms.
* Correspondence:
Youyao Fu, Taizhou University, Taizhou, China
Disclaimer: All claims expressed in this article are solely those of the authors and do not necessarily represent those of their affiliated organizations, or those of the publisher, the editors and the reviewers. Any product that may be evaluated in this article or claim that may be made by its manufacturer is not guaranteed or endorsed by the publisher.
Research integrity at Frontiers
Learn more about the work of our research integrity team to safeguard the quality of each article we publish.