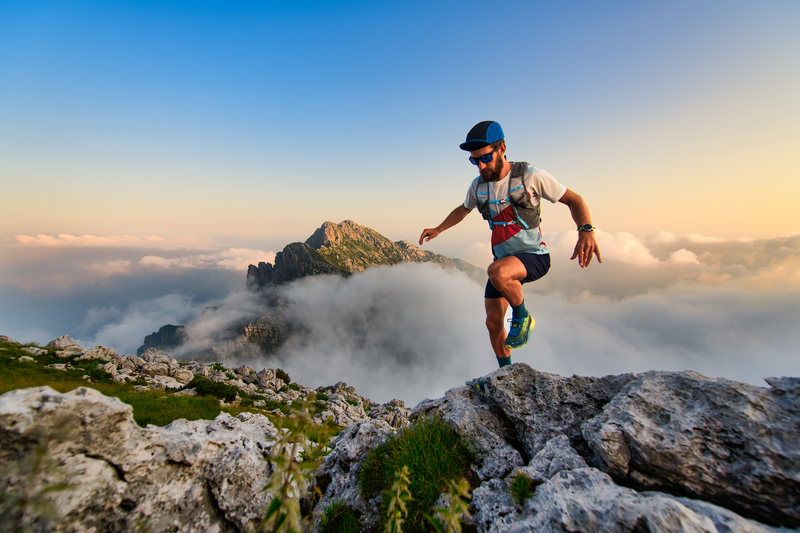
95% of researchers rate our articles as excellent or good
Learn more about the work of our research integrity team to safeguard the quality of each article we publish.
Find out more
ORIGINAL RESEARCH article
Front. Plant Sci.
Sec. Sustainable and Intelligent Phytoprotection
Volume 16 - 2025 | doi: 10.3389/fpls.2025.1513041
The final, formatted version of the article will be published soon.
You have multiple emails registered with Frontiers:
Please enter your email address:
If you already have an account, please login
You don't have a Frontiers account ? You can register here
Wheat is a crucial global food crop but is highly vulnerable to stripe rust, a pervasive and de-structive disease that is challenging to control. The severity classification of wheat stripe rust is helpful to accurately assess the severity of the disease, so as to formulate effective prevention and control measures and reduce yield loss. By identifying the disease in a timely manner, farmers can optimize management strategies and improve the efficiency and stability of wheat production. Effectively recognizing and classifying the severity of wheat stripe rust using artificial intelligence presents significant challenges due to variable image quality, subtle differences between severity levels, and limited model interpretability. To address these challenges, we propose a novel Double-Branch feature fusion neural network, named CCMVT, for classifying the severity of wheat stripe rust. Our model integrates two vi-sion transformers at different scales to fuse image patches of varying sizes, enabling more ro-bust feature extraction. To reduce the computational burden of the Double-Branch Transformer, we utilize Decomposed Manhattan self-attention, which incorporates explicit spatial prior information. Addi-tionally, we designed a Cross-Attention-based token fusion module to facilitate effective infor-mation exchange between branches.To further enhance the model's performance, we employ Latent Diffusion Models (LDMs) for data augmentation, generating highquality training sam-ples that improve the model's generalization and accuracy. Our results show that CCMVT achieves a state-of-the-art accuracy of 91.38%, outperforming existing mainstream methods. Ex-tensive experiments further validate the model's generalization capabilities. In conclusion, our method not only provides valuable technical support for agricultural production but also paves the way for broader applications of artificial intelligence in agriculture.The Wheat is one of the world's most vital food crops, serving as a major food source for many people globally [1]. Proper planting and advanced nutrient management can significantly increase wheat production [2]. However, wheat production is often impacted by various factors, including diseases, pests, and climate change [3]. Among these, diseases are a major threat, particularly wheat rust, which can lead to yield losses exceeding 50% [4]. Wheat rusts include stripe
Keywords: stripe rust of wheat, double-branch, vision Transformer, attention mechanism, diffusion model
Received: 29 Oct 2024; Accepted: 24 Mar 2025.
Copyright: © 2025 Yuan, Cheng and Yang. This is an open-access article distributed under the terms of the Creative Commons Attribution License (CC BY). The use, distribution or reproduction in other forums is permitted, provided the original author(s) or licensor are credited and that the original publication in this journal is cited, in accordance with accepted academic practice. No use, distribution or reproduction is permitted which does not comply with these terms.
* Correspondence:
Dianqing Yang, Keimyung University, Daegu, 704-701, Daegu, Republic of Korea
Disclaimer: All claims expressed in this article are solely those of the authors and do not necessarily represent those of their affiliated organizations, or those of the publisher, the editors and the reviewers. Any product that may be evaluated in this article or claim that may be made by its manufacturer is not guaranteed or endorsed by the publisher.
Research integrity at Frontiers
Learn more about the work of our research integrity team to safeguard the quality of each article we publish.