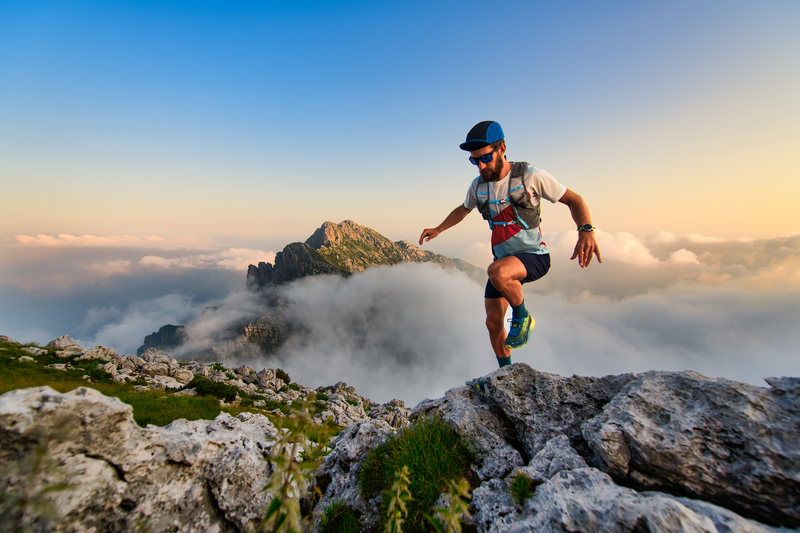
94% of researchers rate our articles as excellent or good
Learn more about the work of our research integrity team to safeguard the quality of each article we publish.
Find out more
ORIGINAL RESEARCH article
Front. Plant Sci.
Sec. Plant Breeding
Volume 16 - 2025 | doi: 10.3389/fpls.2025.1511693
This article is part of the Research Topic Breeding Approaches to Improve Woody Plants’ Resistance to Pests and Diseases View all 8 articles
The final, formatted version of the article will be published soon.
You have multiple emails registered with Frontiers:
Please enter your email address:
If you already have an account, please login
You don't have a Frontiers account ? You can register here
Endangered species can achieve population growth through utilization. Juglans mandshurica is an endangered species, which can be used in gardens and street trees.To avoid population degradation caused by long-term nursery cultivation, we need to introduce high-quality wild sources of germplasm for hybridization. In the past, when the selection of strains was carried out, attention was often paid to the performance of different traits of each strain. The strains with advantages in many more traits were selected as the target. In this paper, we proposed that excellent strains should be selected based on the needs of managers. Therefore, we constructed a complex index composed of insect resistance and growth amount, which was concerned by plantation managers, for the selection of excellent strains. Its availability was confirmed as well.We cultivated 16 wild-sourced J. mandshurica strains in a homogeneous garden and carried out experiments for 3 years. We measured 28 functional traits. Through collinearity diagnostics, 15 functional traits in 4 dimensions (morphology, leaf economy, stoichiometry and reproduction) were selected for analysis and construction of complex index. The influence of environmental factors on traits was excluded by comparing the trait matrix calculated based on Euclidean distance with the geographical distance matrix. Studies showed that, excellent strains (No. 15 from Dazeshan) selected based on the key complex index may not be outstanding in each trait, but have a more balanced performance among the trade-offs of trait combinations. We also explored the visualization of this key complex index by correlated with leaf carbon content (its ecologically relevant trait), so as to realize rapid and early selection of J. mandshurica strains by using LCC (an easily measurable trait). To construct key complex index, appropriate functional traits should be selected according to the needs of managers or different species. The measurable traits with clear ecological links with complex index should be selected as "agents" to realize visualization of complex index.
Keywords: J. mandshurica, measurable trait, Strain optimization, Management needs, insect resistance
Received: 15 Oct 2024; Accepted: 24 Feb 2025.
Copyright: © 2025 Xu, Han, Liu, Pang, Guo, Zhou and Zhang. This is an open-access article distributed under the terms of the Creative Commons Attribution License (CC BY). The use, distribution or reproduction in other forums is permitted, provided the original author(s) or licensor are credited and that the original publication in this journal is cited, in accordance with accepted academic practice. No use, distribution or reproduction is permitted which does not comply with these terms.
* Correspondence:
Ping Zhang, School of Life Sciences, Ludong University, Yantai, China
Disclaimer: All claims expressed in this article are solely those of the authors and do not necessarily represent those of their affiliated organizations, or those of the publisher, the editors and the reviewers. Any product that may be evaluated in this article or claim that may be made by its manufacturer is not guaranteed or endorsed by the publisher.
Research integrity at Frontiers
Learn more about the work of our research integrity team to safeguard the quality of each article we publish.