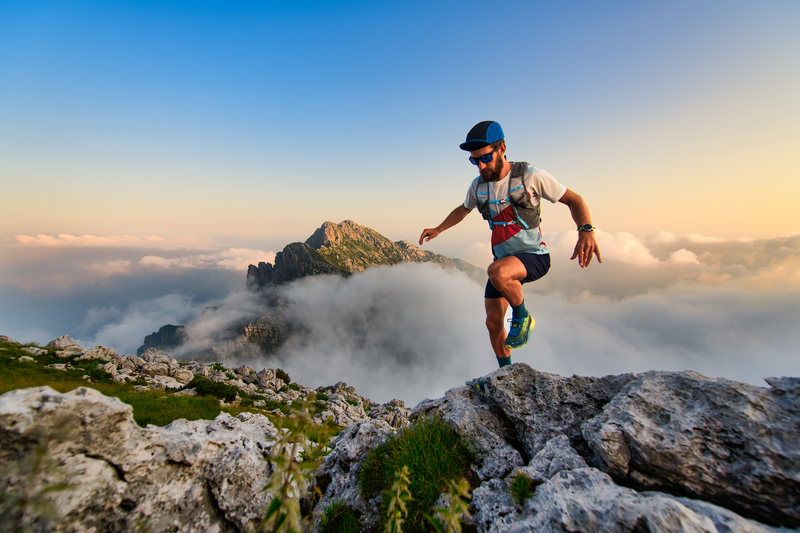
95% of researchers rate our articles as excellent or good
Learn more about the work of our research integrity team to safeguard the quality of each article we publish.
Find out more
ORIGINAL RESEARCH article
Front. Plant Sci.
Sec. Technical Advances in Plant Science
Volume 16 - 2025 | doi: 10.3389/fpls.2025.1503256
The final, formatted version of the article will be published soon.
You have multiple emails registered with Frontiers:
Please enter your email address:
If you already have an account, please login
You don't have a Frontiers account ? You can register here
In order to meet the urgent need of fruit contour information for robot precision picking in complex field environments (such as light changes, occlusion and fruit overlap, etc.), this paper proposes an improved YOLOv8s-seg method for tomato instance segmentation, named ACP-Tomato-Seg. The method proposes two innovative modules: the Adaptive and Oriented Feature Refinement module (AOFRM) and the Custom Multi-scale Pooling module (CMPRD) with Rsiduals and Depth. By deformable convolution and multi-directional asymmetric convolution, the AOFRM module adaptively extracts the shape and direction features of tomatoes to solve the problems of occlusion and overlap. The CMPRD module uses the pooling kernels of self-defined size to extract multi-scale features, which enhances the model's ability to distinguish tomatoes of different sizes and maturity levels. In addition, this paper also introduces a partial self-attention module (PSA), which combines channel attention and spatial attention mechanism to capture global context information, improve the model's ability to focus on the target region and extract details. To verify the validity of the method, a dataset of 1061 images of large and small tomatoes was constructed, covering six ripened categories of large and small tomatoes. The experimental results show that compared with the original YOLOv8s-seg model, the performance of ACP-TomatoSeg model is significantly improved. In the bounding box task, mAP50 and MAP50-95 are improved by 5.6% and 8.3%, respectively, In the mask task, mAP50 and MAP50-95 increased by 5.8% and 8.5%, respectively. Furthermore, additional validation on the public strawberry instance segmentation dataset (StrawDI_Db1) indicates that ACP-Tomato-Seg not only exhibits superior performance but also significantly outperforms existing comparative methods in key metrics. This validates its commendable generalization ability and robustness. The method showcases its superiority in tomato maturity detection and fruit segmentation, thus providing an effective approach to achieving precise picking.
Keywords: Tomato Instance Segmentation, Complex Field Environments, Adaptive feature extraction, Multi-scale features, Self-attention mechanism, ACP-Tomato-Seg
Received: 28 Sep 2024; Accepted: 07 Apr 2025.
Copyright: © 2025 Wei, Sun, Luo, Ni, Chen, You, Mu and Gong. This is an open-access article distributed under the terms of the Creative Commons Attribution License (CC BY). The use, distribution or reproduction in other forums is permitted, provided the original author(s) or licensor are credited and that the original publication in this journal is cited, in accordance with accepted academic practice. No use, distribution or reproduction is permitted which does not comply with these terms.
* Correspondence:
Yu Sun, College of Information Technology, Jilin Agricultural University, Changchun, China
Ye Mu, College of Information Technology, Jilin Agricultural University, Changchun, China
He Gong, College of Information Technology, Jilin Agricultural University, Changchun, China
Disclaimer: All claims expressed in this article are solely those of the authors and do not necessarily represent those of their affiliated organizations, or those of the publisher, the editors and the reviewers. Any product that may be evaluated in this article or claim that may be made by its manufacturer is not guaranteed or endorsed by the publisher.
Research integrity at Frontiers
Learn more about the work of our research integrity team to safeguard the quality of each article we publish.