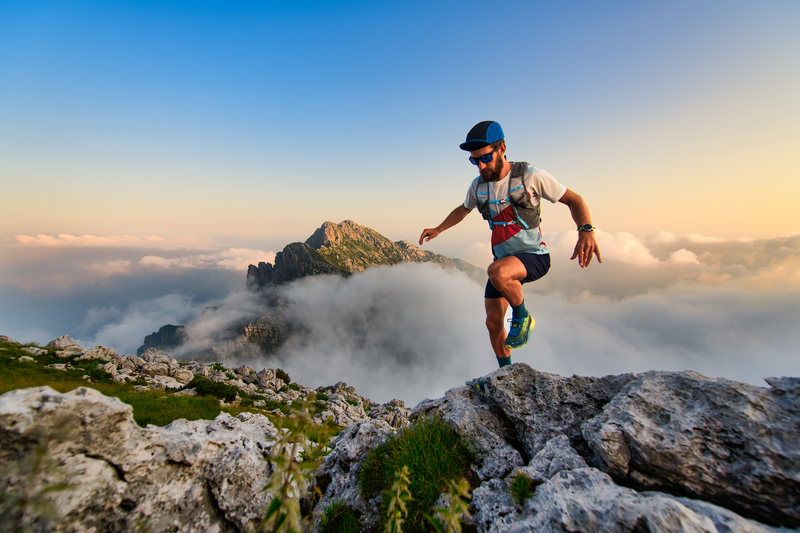
95% of researchers rate our articles as excellent or good
Learn more about the work of our research integrity team to safeguard the quality of each article we publish.
Find out more
ORIGINAL RESEARCH article
Front. Plant Sci.
Sec. Technical Advances in Plant Science
Volume 16 - 2025 | doi: 10.3389/fpls.2025.1499909
This article is part of the Research Topic Harnessing Machine Learning to Decode Plant-Microbiome Dynamics for Sustainable Agriculture View all 16 articles
The final, formatted version of the article will be published soon.
You have multiple emails registered with Frontiers:
Please enter your email address:
If you already have an account, please login
You don't have a Frontiers account ? You can register here
Potatoes are a vital global product, and prompt identification of foliar diseases is imperative for sustaining healthy yields. Computer vision is essential in precision agriculture, facilitating automated disease diagnosis and decision-making through real-time data. Inconsistent data in uncontrolled contexts undermines classic image classification techniques, hindering precise illness detection. We present a novel model that integrates EfficientNet-LITE for enhanced feature extraction with KE-SVM Optimization for effective classification. KE-SVM Optimization cross-references misclassified instances with correct classifications across kernels, iteratively refining the confusion matrix to improve accuracy across all classes. EfficientNet-LITE improves the model's emphasis on pertinent features through Channel Attention (CA) and 1-D Local Binary Pattern (LBP), while preserving computational economy with a reduced model size of 12.46 MB, fewer parameters at 3.11M, and a diminished FLOP count of 359.69 MFLOPs. Before optimization, the SVM classifier attained an accuracy of 79.38% on uncontrolled data and 99.07% on laboratory-controlled data. Following the implementation of KE-SVM Optimization, accuracy increased to 87.82% for uncontrolled data and 99.54% for laboratory-controlled data. The model's efficiency and improved accuracy render it especially appropriate for settings with constrained computational resources, such as mobile or edge devices, offering substantial practical advantages for precision agriculture.
Keywords: EfficientNet-LITE, KE-SVM Optimization, Channel attention, 1-D Local Binary Pattern, Sobel Edge augmentation
Received: 22 Sep 2024; Accepted: 27 Mar 2025.
Copyright: © 2025 G and V. This is an open-access article distributed under the terms of the Creative Commons Attribution License (CC BY). The use, distribution or reproduction in other forums is permitted, provided the original author(s) or licensor are credited and that the original publication in this journal is cited, in accordance with accepted academic practice. No use, distribution or reproduction is permitted which does not comply with these terms.
* Correspondence:
Sangar G, SRM Institute of Science and Technology, Vadapalani Campus, Chennai, India
Disclaimer: All claims expressed in this article are solely those of the authors and do not necessarily represent those of their affiliated organizations, or those of the publisher, the editors and the reviewers. Any product that may be evaluated in this article or claim that may be made by its manufacturer is not guaranteed or endorsed by the publisher.
Research integrity at Frontiers
Learn more about the work of our research integrity team to safeguard the quality of each article we publish.