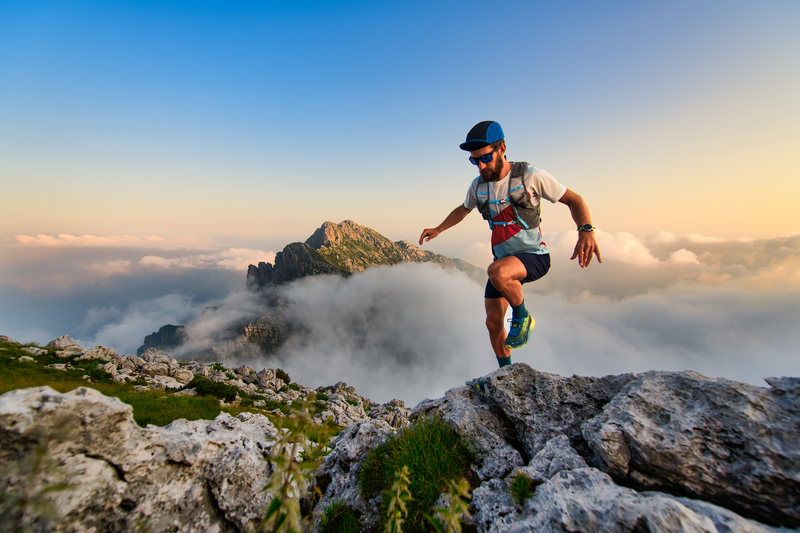
95% of researchers rate our articles as excellent or good
Learn more about the work of our research integrity team to safeguard the quality of each article we publish.
Find out more
EDITORIAL article
Front. Plant Sci. , 04 December 2024
Sec. Sustainable and Intelligent Phytoprotection
Volume 15 - 2024 | https://doi.org/10.3389/fpls.2024.1518814
This article is part of the Research Topic Pests and Diseases Monitoring and Forecasting Algorithms, Technologies, and Applications View all 19 articles
Editorial on the Research Topic
Pests and diseases monitoring and forecasting algorithms, technologies, and applications
In the face of growing challenges in agriculture due to pests and diseases, the need for advanced monitoring and forecasting techniques has become increasingly critical. Climate change, global trade, and the adaptation of pests to traditional control methods have further complicated this landscape. This Research Topic offers a collection of studies highlighting the latest advancements in pest and disease monitoring, focusing on the development and application of innovative algorithms, technologies, and practical solutions to mitigate the impact of these threats on agriculture.
Recent advances in deep learning, such as fast Fourier Convolutional Networks, have shown promise in distinguishing between similar symptoms like wheat yellow rust and nitrogen deficiency using Sentinel-2 time series data (Shi et al.). These techniques underscore the power of modern AI to refine diagnostic accuracy, which is crucial for early intervention and targeted management. Similarly, the spatial ensemble model has been employed to assess the potential risk zones of Pierce’s disease across Europe, integrating multiple data sources to offer more reliable predictions for pest management (Yoon et al.).
Within controlled environments, greenhouse-based pest monitoring has seen significant improvements due to the implementation of deep learning and machine vision (Zhang et al.). Automatic identification systems are now capable of real-time recognition of pests, thanks to the deployment of sophisticated neural networks. The integration of UAV technology with deep learning also extends pest monitoring capabilities to broader agricultural landscapes. For instance, studies on Brandt’s vole detection and counting via UAV-based systems exemplify the ability to efficiently monitor field conditions (Wu et al.), while multispectral imaging from UAVs provides detailed nutritional assessments, such as potassium levels in potato plants (Ma et al.).
Machine learning architectures have also been developed to handle complex diagnostic tasks in challenging environments (Liu et al.). Techniques like the multi-scale double-branch GAN-ResNet for rice pest identification demonstrate the application of advanced algorithms in complex scenarios, including those with variable backgrounds (Hu et al.). Other lightweight deep learning models, such as MS-Net, are designed to be both accurate and efficient, focusing on optimizing computational resources without compromising precision (Quan et al.).
The fusion of multispectral and hyperspectral data has shown great potential in early disease detection across different crop types and ecosystems. The MSGF-GLP method, for example, utilizes visible and hyperspectral data to identify stressed vegetation, enhancing early detection capabilities (Zhou et al.). These approaches highlight the increasing role of spectral data in disease management, offering more nuanced insights into plant health (Huang et al.).
Field-based applications of these technologies have also made considerable strides. Studies on the penetration of fog droplets in fruit tree canopies (Sun et al.) reveal the multifactorial elements affecting pesticide delivery efficiency. These findings are crucial for improving precision agriculture, allowing targeted interventions that minimize pesticide use while maximizing coverage. Lightweight models like the enhanced CNN (Dai et al.) for pepper leaf disease recognition showcase how AI can be applied to specific crops, even in complex agricultural settings. Similarly, research on weed identification in soybean fields using lightweight segmentation models such as DCSAnet demonstrates (Yu et al.) the application of optimized AI architectures in practical field conditions.
The rise of mobile applications powered by AI, such as GranoScan (Dainelli et al.) for in-field wheat threat identification, reflects the growing trend of democratizing technology for farmers. These tools provide accessible and accurate diagnostic capabilities, empowering agricultural stakeholders with real-time data. Likewise, the development of UAV spraying systems (Liu et al.) that account for pest activity patterns, such as thrips during the cotton flowering period, illustrates the synergy between automated technologies and pest behavior research. Additionally, a risk-based regionalization approach has been proposed for the area-wide management of HLB vectors in the Mediterranean Basin (Galvan et al.), offering a strategic perspective to mitigate the spread of disease.
The Research Topic also addresses challenges associated with AI applications in pest monitoring. Issues such as complex environments, small object detection, and the variability of natural conditions continue to test the limits of current technologies. Innovations like the Skip DETR model (Liu et al.), which integrates skip connections for small object detection, and the adaptive filtering fusion method for pest recognition, indicate ongoing efforts to overcome these obstacles (Chen et al.).
In summary, this Research Topic offers a comprehensive overview of current innovations in pest and disease monitoring. The articles included emphasize the growing role of AI, machine learning, and advanced imaging technologies in modern agriculture. Together, these studies not only demonstrate the effectiveness of cutting-edge solutions but also underline the importance of continued collaboration across disciplines to address the evolving challenges in pest and disease management.
YD: Conceptualization, Methodology, Supervision, Validation, Writing – original draft, Writing – review & editing. WH: Conceptualization, Methodology, Resources, Writing – review & editing. KL: Data curation, Investigation, Supervision, Validation, Writing – review & editing. LH: Data curation, Formal analysis, Methodology, Validation, Writing – review & editing. GL: Conceptualization, Methodology, Validation, Writing – review & editing. JZ: Data curation, Formal analysis, Methodology, Validation, Writing – review & editing.
The authors declare that the research was conducted in the absence of any commercial or financial relationships that could be construed as a potential conflict of interest.
The author(s) declared that they were an editorial board member of Frontiers, at the time of submission. This had no impact on the peer review process and the final decision.
All claims expressed in this article are solely those of the authors and do not necessarily represent those of their affiliated organizations, or those of the publisher, the editors and the reviewers. Any product that may be evaluated in this article, or claim that may be made by its manufacturer, is not guaranteed or endorsed by the publisher.
Keywords: pest and disease monitoring, AI and machine learning, UAV technology, multispectral and hyperspectral data, precision agriculture
Citation: Dong Y, Huang W, Lin K, Han L, Laneve G and Zhang J (2024) Editorial: Pests and diseases monitoring and forecasting algorithms, technologies, and applications. Front. Plant Sci. 15:1518814. doi: 10.3389/fpls.2024.1518814
Received: 29 October 2024; Accepted: 18 November 2024;
Published: 04 December 2024.
Edited and Reviewed by:
Lei Shu, Nanjing Agricultural University, ChinaCopyright © 2024 Dong, Huang, Lin, Han, Laneve and Zhang. This is an open-access article distributed under the terms of the Creative Commons Attribution License (CC BY). The use, distribution or reproduction in other forums is permitted, provided the original author(s) and the copyright owner(s) are credited and that the original publication in this journal is cited, in accordance with accepted academic practice. No use, distribution or reproduction is permitted which does not comply with these terms.
*Correspondence: Yingying Dong, ZG9uZ3l5QGFpcmNhcy5hYy5jbg==
Disclaimer: All claims expressed in this article are solely those of the authors and do not necessarily represent those of their affiliated organizations, or those of the publisher, the editors and the reviewers. Any product that may be evaluated in this article or claim that may be made by its manufacturer is not guaranteed or endorsed by the publisher.
Research integrity at Frontiers
Learn more about the work of our research integrity team to safeguard the quality of each article we publish.