- 1Department of Plant and Soil Sciences, Oklahoma State University, Stillwater, OK, United States
- 2Department of Statistics, Kansas State University, Manhattan, KS, United States
- 3Department of Agronomy, Kansas State University, Manhattan, KS, United States
Increasing wheat (Triticum aestivum L.) yield and grain protein concentration (GPC) without excessive nitrogen (N) inputs requires understanding the genotypic variations in N accumulation, partitioning, and utilization strategies. This study evaluated whether high protein genotypes exhibit increased N accumulation (herein also expressed as N nutrition index, NNI) and partitioning (including remobilization from vegetative organs) compared to low-protein genotypes under low and high N conditions. Four winter wheat genotypes with similar yields but contrasting GPC were examined under two N rates (0 and 120 kg N ha-1) across two environments and four growing seasons in Oklahoma, US. As expected, the high-protein genotypes Doublestop CL+ (Dob) and Green Hammer (Grn) had greater GPC than the medium- (Gallagher, Gal) and low-protein genotypes (Iba), without any difference in grain yield. Total plant N accumulation at maturity showed diminishing increases for greater grain yield, and low-protein genotype showed greater N utilization efficiency (NUtE) than high-protein genotypes. The high-protein genotype Grn tended to achieve higher GPC by increasing total N uptake, while Dob exhibited a tendency towards higher N partitioning to grain (NHI). The allometric relationship between total N accumulation and biomass remained unchanged for both high- and low-protein genotypes. The N remobilization patterns differed between high- and low-protein genotypes. As N conditions improved, the proportional contributions of remobilized N from leaves tended to increase, while contributions from stems and chaff tended to decrease or remained unchanged for high-protein genotypes. This study highlights the importance of both N uptake capacity and efficient N partitioning to the grain as critical traits for realizing wheat’s dual goals of higher yield and protein. Leaf N remobilization plays a critical role during grain filling, sustaining plant N status and contributing to protein levels. The higher NUtE observed in the low-protein genotype Iba likely contributed to its lower GPC, emphasizing the trade-off between NUtE and GPC. The physiological strategies employed by high-protein genotypes, such as genotype Grn’s tendency for increased N uptake and Dob’s efficient N partitioning, provide a foundation for future breeding efforts aimed at developing resource-efficient and nutritionally superior wheat genotypes capable of achieving both increased yield and protein.
Introduction
Wheat (Triticum aestivum L.) is the most widely cultivated staple cereal crop, providing calories to 20% of the world’s population and serving as a vital source of human nutrition (FAO, 2019; Padhy et al., 2024). Given its growing global demand and importance for food security, improving grain yield and grain protein concentration (GPC) is crucial to meet the rising production needs and the nutritional quality of wheat (Sendhil et al., 2022; Teng et al., 2022). However, simultaneously enhancing grain yield and GPC is challenging due to their trade-off (Michel et al., 2019). This trade-off occurs because increasing both grain yield (a C-based compound) and GPC (an N compound) are competing metabolic processes for energy (Munier-Jolain and Salon, 2005). Furthermore, the dilution theory suggests that as grain yield increases, protein concentration decreases due to the dilution of N in the grain (i.e., source limitation) (Whitfield and Smith, 1992; Acreche and Slafer, 2009). However, this negative relationship between yield and GPC can be mitigated or even reversed through improved crop management practices and genotype selection (Zhang et al., 2017; Pan et al., 2020). It has been postulated that selecting genotypes with high total N uptake at maturity, N harvest index (NHI, ratio of grain N to total N uptake), N accumulation during grain filling (PostN) or N remobilization from vegetative organs to the grain (RemN) are associated with ability of genotypes to reach adequate yield while maintaining or improving its GPC (Bogard et al., 2010; Cormier et al., 2016; Fortunato et al., 2023). However, earlier research has reported inconsistent associations between NHI, PostN, RemN, and GPC under different environmental conditions. For instance, in wheat genotypes with different protein levels cultivated under dryland conditions, Desai and Bhatia (1978) and Chen et al. (2023) found a weak relationship between NHI and GPC. In general, RemN has contributed from 60 to 95% to grain N at maturity as compared to PostN, which has provided a 35 to 55% contribution to grain N accumulation (Palta and Fillery, 1995; Kichey et al., 2007). However, these contributions may differ depending on the environment, genotype, and management techniques (Taulemesse et al., 2015). Given these inconsistencies, evaluating the physiological mechanisms responsible for reducing the yield-protein trade-off is very important. Understanding these mechanisms may provide insights into the drivers of genotypic variability in GPC and help breeding programs identify wheat genotypes that can achieve high yield and GPC.
Although the literature is vast in evaluating the contribution of RemN to GPC (Barbottin et al., 2005; Barraclough et al., 2010; Gaju et al., 2014; Chen et al., 2015; Kaur et al., 2015), it lacks an understanding of the relative contributions of N remobilization from different vegetative organs (e.g., leaves, stems, chaff) to the grain in modern genotypes. Moreover, the negative relationship between N utilization efficiency (ratio of grain yield and total N uptake, NUtE) and GPC highlights the difficulty of breeding and managing crops to maximize grain yield and GPC concurrently (Gaju et al., 2014; Thompson et al., 2022). N utilization efficiency measures the capacity of genotypes to make use of the N accumulated by the plant for grain or biomass production (Moll et al., 1982; De Oliveira Silva et al., 2020; Lebedev et al., 2021; Biradar et al., 2024). Thus, examining the dynamics of NUtE may offer important insights into the physiological mechanisms controlling the yield-protein trade-off.
The Nitrogen Nutrition Index (NNI), defined as the ratio between the actual plant N concentration and the critical N concentration required for maximum growth, serves as a valuable indicator of crop N status throughout the growing season (Lemaire et al., 2008; Sadras and Lemaire, 2014). Thus, evaluating the association of physiological traits and NNI (e.g., NNI and yield components, Rodriguez et al., 2024), could provide crucial insights into the plant’s nitrogen nutritional state, allowing for a more nuanced understanding of nitrogen dynamics in relation to yield and protein formation.
Our study aims to provide a comprehensive understanding of the physiological mechanisms underlying genotypic differences in GPC under high and low N conditions in dryland environments to ultimately inform breeding strategies and crop management practices to develop genotypes capable of optimizing both yield and protein concentration. Specifically, our objectives were to (a) evaluate whether high-protein genotypes exhibit increased N accumulation and partitioning as compared to medium-low protein genotypes under high and low N availability and (b) investigate whether the relationship between the proportional contributions of N remobilization from each vegetative organ to the grain and NNI differs between high and low protein genotypes.
Material and methods
General experiment information
A non-irrigated research study was conducted in two locations for four years (i.e., eight site-years) in Oklahoma. The experiments were established at the Oklahoma State University Research Station in Stillwater (36°08’24.9’N, 9705’37.0”W) and the Cimarron Valley Research Station in Perkins (35°59’25.2”N, 97°02’41.2”W) during 2019-2020, 2020-2021, 2021-2022 and 2022-2023 wheat growing seasons. The soil type for the experiment in Stillwater was Port silt loam (Fine-silty, mixed, superactive, thermic Cumulic Haplustolls) (USDA/NRCS soil taxonomy). The soil type for the experiment carried out in Perkins was the Teller series (fine loamy, mixed, active, thermic Udic Argiustolls) and Konawa series (fine loamy, mixed, active, thermic Ultic Haplustalfs). Weather information was acquired daily from planting to harvest (i.e., from early October to the end of June) from automated weather stations operated by the Mesonet Oklahoma weather network, proximately located to the research sites (Table 1).
The field trials in Perkins were performed under conventional tillage, implemented in the fall before wheat sowing, and under a no-tillage system in Stillwater. The experiments were sown using a Great Plains Not-till drill (3P605NT) with a seeding rate of two million seeds ha-1. Plots were 10.6 m long and 1.5 m wide, with seven rows 0.19 m apart. At sowing, 47 kg ha-1 of ammonium polyphosphate (10-34-0) was applied along the seed furrow in all plots. Diseases, insects, and weeds were chemically controlled as needed. Soil fertility was evaluated at the time of sowing and after harvesting for each site-year (Table 2). At sowing, 15 soil cores were obtained at 0-6 inches and 6-12 inches depth across the field and combined to represent a composite sample using hand probes. Two weeks after harvest, soil samples were collected from each individual plot at the same depths (0-15 cm and 15-30 cm) using hand probes. Samples were analyzed at the Oklahoma State University’s Soil, Water, and Forage Analytical Laboratory (SWFAL) to analyze pH, N, P, K, Mg, Ca, and SO4 (Table 2).
Experimental design and treatment structure
The experiment was arranged in a randomized complete block design (RCBD) with a 2x4 factorial treatment structure (i.e., two N rates and four genotypes) and four replications. The N rates evaluated were zero N (0N) application, which represented the conditions with only the residual soil N pool, and 120 kg N ha-1 (120N) of urea (46-0-0) broadcasted in the fall, which represented conditions with an appropriate fertilizer application for the region (Zhang et al., 2017). The four winter wheat genotypes evaluated were Iba with Plant Variety Protection Act (PVP, 201300135), released in 2012; Gallagher (Gal) (PI 667569; PV 201300134), released in 2012; Doublestop CL+ (Dob) (PVP 201400228), released in 2013; and Green Hammer (Grn) (PVP 201900171), released in 2018. These genotypes were selected due to their adaptability to the environments explored in this study, their similar yield levels, and differences in grain protein concentration (GPC). Iba represented a low protein genotype, Gal a medium protein genotype, and Dob and Grn high protein genotypes (De Oliveira Silva et al., 2023).
Aboveground plant biomass harvesting, partitioning, and total N analysis
Aboveground biomass was collected from one linear meter of a middle row at anthesis (Z59) and physiological maturity (Z92) at four replications. In the first and second growing seasons (i.e., 2019-2020 and 2020-2021), aboveground biomass was collected by the average growth stage of all genotypes. In the following two growing seasons (i.e., 2021-2022 and 2022-2023), samples were collected based on the growth stage of each genotype. Plants were cut with an electric clipper as close to the soil surface as possible at each growth stage. Plants were partitioned into leaf, stem, and spike at anthesis and leaf, stem, grain, and chaff at physiological maturity. Then, samples were placed in a dryer set at 65°C for seven days. The dry weight of each plant organ was recorded, and plant samples were sent to the OSU Soil, Water and Forage Analytical Laboratory for total N analysis using a LECO CN Combustion Analyzer and an Elementa CN Combustion Analyzer. Grain yield was estimated from the aboveground biomass samples collected at physiological maturity.
Plant N uptake was calculated by multiplying the aboveground biomass of each plant organ by its N concentration (%) (Ciampitti and Vyn, 2013), as
Grain protein concentration (GPC) was calculated by multiplying the grain N concentration (%) by the conversion factor 5.7 (i.e., 5.7 units of protein per unit of N) (Sosulski and Imafidon, 1990), as
The Nitrogen Harvest Index (NHI, %) was estimated as the ratio of grain N uptake to whole plant N uptake at physiological maturity (Ciampitti and Vyn, 2012), as
Post-flowering N uptake (PostN, kg ha-1) was calculated as the whole plant N uptake at physiological maturity minus whole plant N uptake at anthesis, as
Remobilized N (RemN, kg ha-1) was calculated as whole plant N uptake at the anthesis stage minus stover N uptake (i.e., stem + leaf + chaff) at the physiological maturity stage, as
Nitrogen remobilized from individual vegetative organs (organ Rem N, kg ha-1) was calculated as the difference between the N uptake of each vegetative organ (i.e., stem, leaf, and spike) at anthesis and N uptake in the same organs at maturity (Ortez et al., 2019), as
Nitrogen remobilized from all vegetative organs from anthesis to maturity (Sum RemNVeg) was calculated as the sum of the remobilized N in the three vegetative plant organs (i.e., stem, leaf, and chaff) (Ortez et al., 2019), as
The contribution of N remobilization from each vegetative plant organ (i.e., stem, leaf, or chaff) was estimated as relative to the total vegetative N remobilization (Ortez et al., 2019), as
The actual plant N concentration (Na, %) was estimated by dividing the whole plant N uptake (Na, %) by whole plant biomass at anthesis, as
Critical N concentration (Nc, %) was determined using the critical dilution curve model for wheat from Justes et al. (1994), where 5.35% was the whole plant N concentration, when biomass was between 1.5 to 12 Mg ha-1 and -0.442 the dilution coefficient (i.e., rate of decrease in whole plant N concentration as the biomass increases), as
The Nitrogen Nutrition Index (NNI) (Equation 11) was estimated by dividing the actual N concentration of whole plant (Na, %) at anthesis (Equation 9) by the critical N concentration (Nc, %) (Equation 10), as
Statistical analysis
Linear mixed models were applied for evaluating the treatment effect on the traits measured. For each trait at each location, we fitted the model i.e., we considered genotype, N rate, and their interactions as fixed effects, and blocks nested within each year as random effects. The models can be generally described as
where yijkl is the trait being studied observed for the ith genotype, jth N treatment, kth year and lth block, µ is the overall mean, is the effect of the ith genotype, is the effect of the jth N treatment, is the interaction between the ith genotype and jth N treatment, is the random effect of the kth year, is the random effect of the lth block in the kth year, and is the residual. All three u, v, and are assumed iid and independent among each other.
For the remobilized N and its contribution to grain N accumulation, we fit the model
where yijklm is the trait being studied observed for the ith genotype, jth N treatment, kth year, lth block at the mth location, µ is the overall mean, is the effect of the ith genotype, is the effect of the jth N treatment, , , are the two-way interactions, is the three way interaction, is the random effect of the lth year, is the random effect of the mth block in the lth year, and is the residual. All three u, v, and are assumed iid and independent among each other. We performed an ANOVA using the models outlined in Equation 12 for the major traits (i.e., yield, GPC, plant biomass, plant N uptake). Next, we compared mean differences between treatments (i.e., combinations of N treatment and genotype) within locations using the Tukey adjustments and a significance level of 0.05.
To describe the relationship between grain yield vs plant N uptake at maturity, total biomass vs N uptake at maturity, and grain N accumulation vs N uptake at maturity, we compared simple linear regression (i.e., intercept and slope) to quadratic, and cubic. All models were fitted using R software. Linear mixed models were fitted using the R packages “lme4” (Bates et al., 2015) and “lmerTest” (Kuznetsova et al., 2017), and multiple comparisons were done with the “multcomp” package in R (Hothorn et al., 2008).
Results
Examining grain yield, grain protein concentration, and NUtE trends in winter wheat genotypes
This study evaluated the ability of genotypes to produce grain yield while minimizing the trade-off with GPC by evaluating four winter wheat genotypes (selected for their known similar yield levels and varying GPC), two N rates (0N and 120N), two locations, and four growing seasons (from 2019-2020 to 2022-2023) resulting in a total of 256 observations. The results were reported for each site across years (i.e., individual sites were analyzed separately) independently.
The grain yield values ranged from 769 to 8005 kg ha-1 with an estimated standard deviation of 1246 kg ha-1, and GPC values ranged from 8 to 16% with a standard deviation of 1.5% points across all sources of variation evaluated (i.e., genotypes, N rate, and site-years). As expected, the genotypes tested in this study showed similar yield levels across N rates in both sites (Figure 1A) and different GPC at the high N rate in both sites (Figure 1B). Iba had the lowest GPC, Gal had a medium GPC, and Dob and Grn with higher GPC values. The high protein genotypes Dob and Grn had greater mean GPCs of 11.4% and 11.6%, respectively, than the low protein genotype Iba, with a mean GPC of 10.3% at the 120N in both sites. They also had greater GPC than the medium protein genotype Gal, with a mean GPC equal 10.6% in Stillwater. A quadratic model better illustrated the relationship between grain yield and total N accumulation at maturity (slope = NUtE) than a linear model (Supplementary Table S1). The model showed a quadratic rise towards a maximum yield value, indicating that increases in total N accumulation at maturity resulted in greater yield up to a certain level, after which yield gains diminished as N accumulation continued to rise (Figure 1C, Supplementary Figure S1). Thus, there was an overall positive and quadratic relationship between grain yield and total N accumulation at maturity, with 87% (for Perkins) and 89% (for Stillwater) of the variation in grain yield due to N rate, genotypes, and growing seasons explained by differences in total N accumulation at maturity (Figure 1C). In both sites, the association between grain yield and total N accumulation was investigated to quantify the N Utilization Efficiency (NUtE) of genotypes differing in GPC. This analysis further revealed that increases in yield via increases in NUtE are possible in high grain yield and N accumulation scenarios. Furthermore, in Stillwater, 70% of each high-protein genotypes Dob and Grn data points fell below the trendline, while 54% of the low-protein genotype Iba fell at or above the trendline, specially under low N uptake levels, indicating lower NUtE for high protein genotypes. In Perkins, 54% of each high-protein genotype Grn and Dob datapoints were below the trendline, while 66% of the low-protein genotype Iba were at or above the regression line, indicating a greater NUtE for Iba in comparison to the high-protein genotypes (Supplementary Figure S2). Our results suggest that the high NUtE of the low protein genotype Iba may have penalized its GPC, and that the high protein genotypes Dob and Grn seemed to use different pathways to achieve GPC. The higher protein from the genotype Dob did not come from greater N uptake, while it could have for Grn.
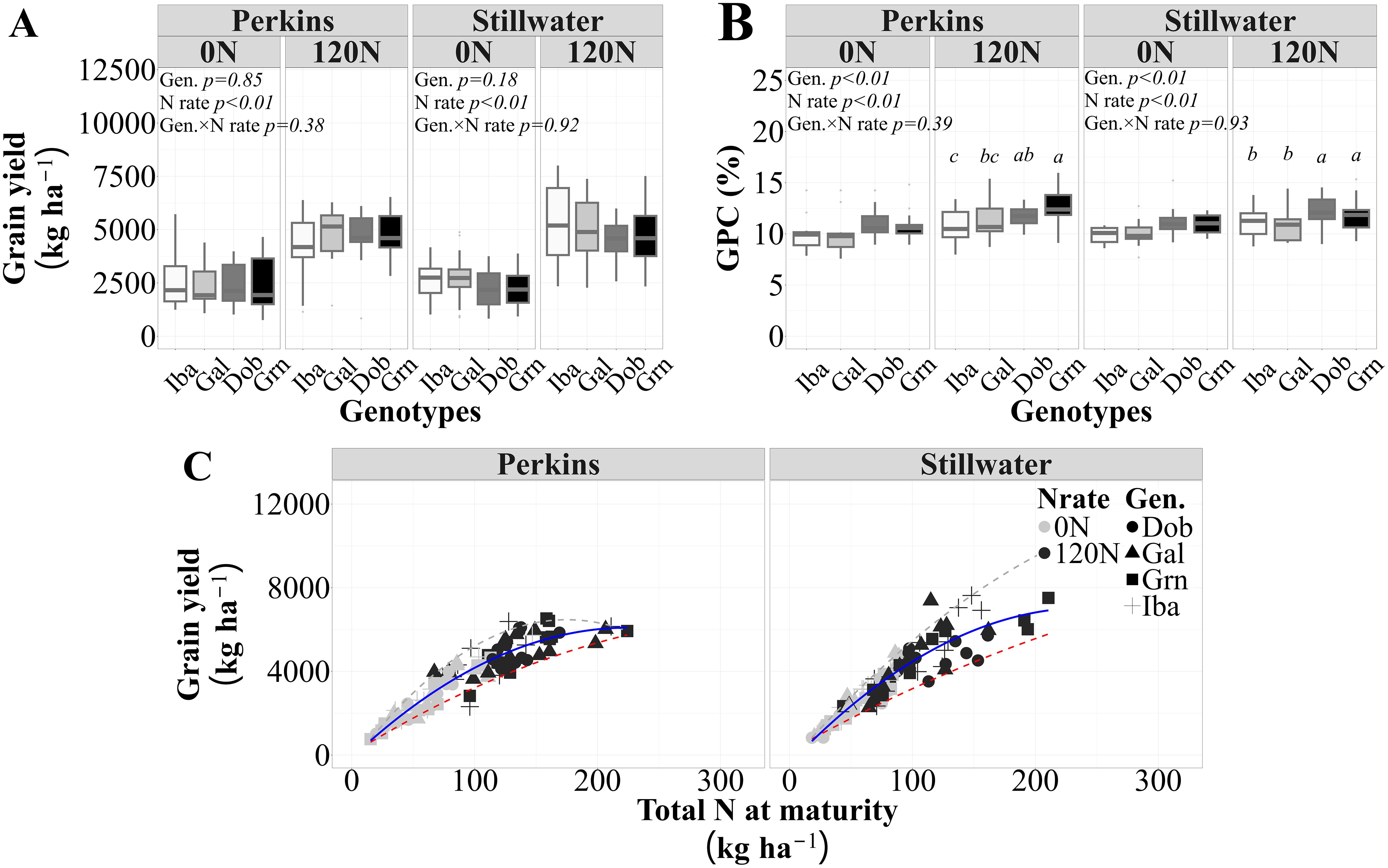
Figure 1. Mean grain yield (A), grain protein concentration (B) for each genotype, N rate and site on average of four growing seasons, and the relationship between grain yield and total N accumulation at maturity (C) for each site across two N rates (0N and 120N), four genotypes, and four growing seasons (n=96 observations). The solid lines represent the selected models for this relationship (Perkins, y = 0.12x2+ 55x-130, R2 = 0.83; Stillwater, y = -0.11x2 + 62x -418, R2=0.84), and the dashed lines are the 5% and 95% quantiles (minimum and maximum N utilization efficiency (i.e., NUtE, grain yield to whole plant N uptake ratio), respectively. The statistical analysis shown in (B) indicates that mean values with different letters are statistically different at p<0.05.
Total N and biomass accumulation in genotypes with different protein levels
We evaluated whether the high protein genotypes showed increased N and biomass accumulation compared to the low protein genotypes, and whether their accumulation dynamics would change when they were evaluated under low or high N conditions. Nitrogen rate increased biomass and N uptake of all genotypes, but the accumulation patterns between genotypes remained consistent under both low and high N conditions (Supplementary Tables S4, S5). A strong positive association was observed between total N accumulation and biomass accumulation at maturity for both sites (Figure 2; Supplementary Figure S3). A linear model better illustrated the relationship between total N and biomass accumulation at maturity (Supplementary Table S2). At both sites, increases in total biomass were related to a corresponding increase in total N accumulation at maturity, irrespective of genotype GPC levels. Based on the r2 of this relationship, 83% (Perkins) and 77% (Stillwater) of the variation in N accumulation due to N rate, genotypes, and growing seasons were explained by changes in biomass at physiological maturity. Hence, the stoichiometry of whole plant N uptake and biomass did not change for genotypes with high and low GPC.
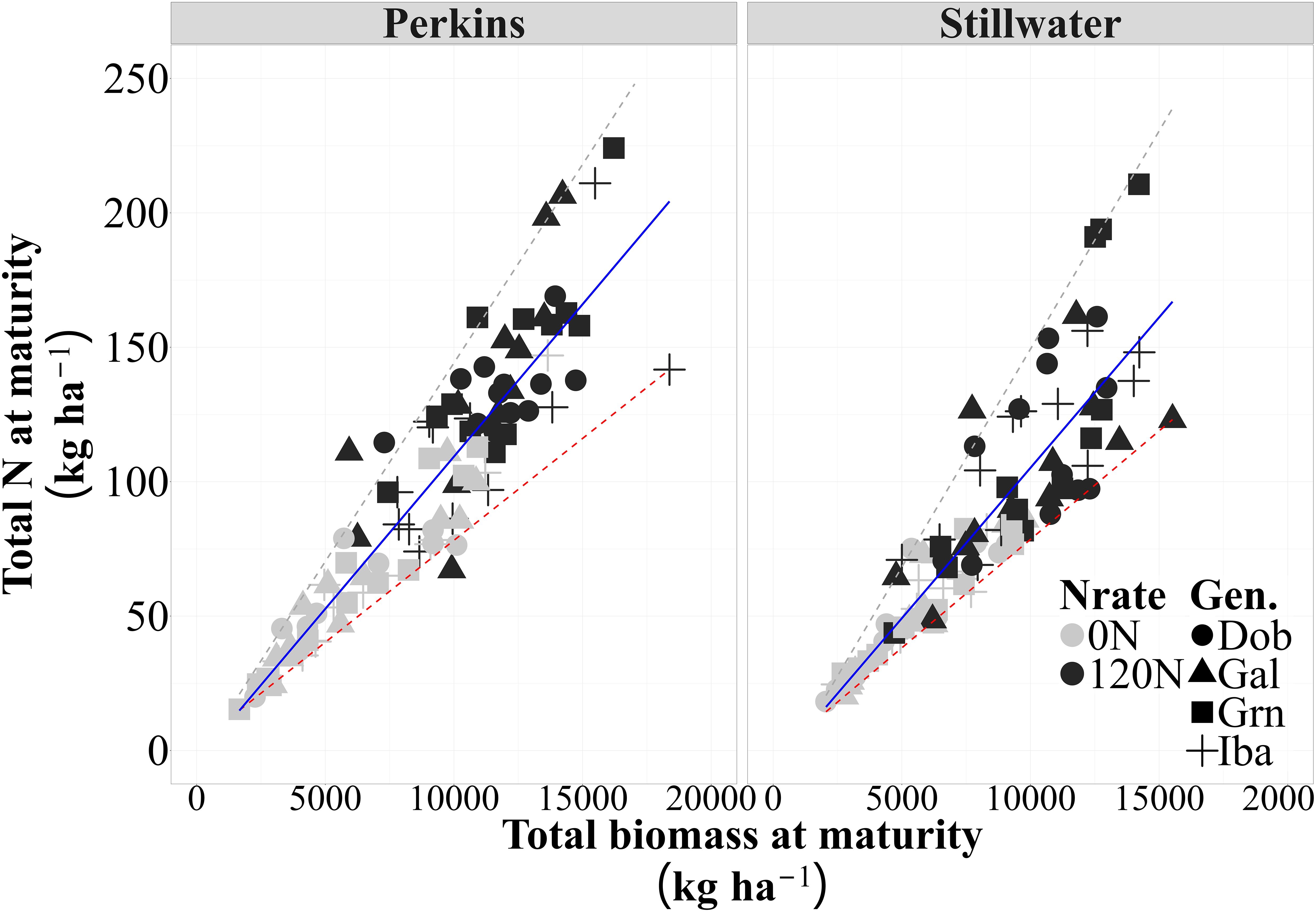
Figure 2. Relationship between total N and biomass accumulation at maturity for each site across four genotypes, two N rates (0N and 120N), and three growing seasons (2020, 2021, 2023) (n=96 observations) (Perkins, y = 0.011x-3.98, R2= 0.83, p<0.01 and Stillwater, y = 0.012x-10.9, R2=0.77, p<0.01). The solid lines represent the selected models to describe this relationship, and the dashed lines are the 5% and 95% quantiles (minimum and maximum, respectively).
Nitrogen partitioning to the grain
There was a strong and positive association between grain N accumulation and total plant N at maturity in both sites (p<0.01), with approximately 96 and 95% of the variation in grain N accumulation across all sources of variation (i.e., N rate, genotypes, and growing seasons) explained by differences in total N accumulation at maturity in both sites (Figure 3, Supplementary Figure S4). This strong relationship demonstrates that genotypes with higher total N accumulation tended to partition a more significant proportion of their acquired N towards grain and that some genotypic variation may occur. For instance, in Perkins, 50% of the datapoints for the low protein genotype Iba were below the trendline while the 72% of the datapoints for the high protein genotype Dob were at or above the trendline, indicating a greater NHI for Dob as compared to Iba (Figure 3; Supplementary Figure S5). Based on the slope of this relationship, the average NHI was 63% for Perkins and 67% for Stillwater. The consistency of these strong relationships in both sites (R2>0.90 and p<0.05) indicated that the greater NHI observed in the high-protein genotype is relatively stable and less influenced by environmental factors or N availability levels. Therefore, the strong partitioning capability of some high-protein genotypes enables them to maintain high GPC levels while achieving competitive yields. This supports the hypothesis that high-protein genotypes may exhibit increased N partitioning to the grain, irrespective of N availability.
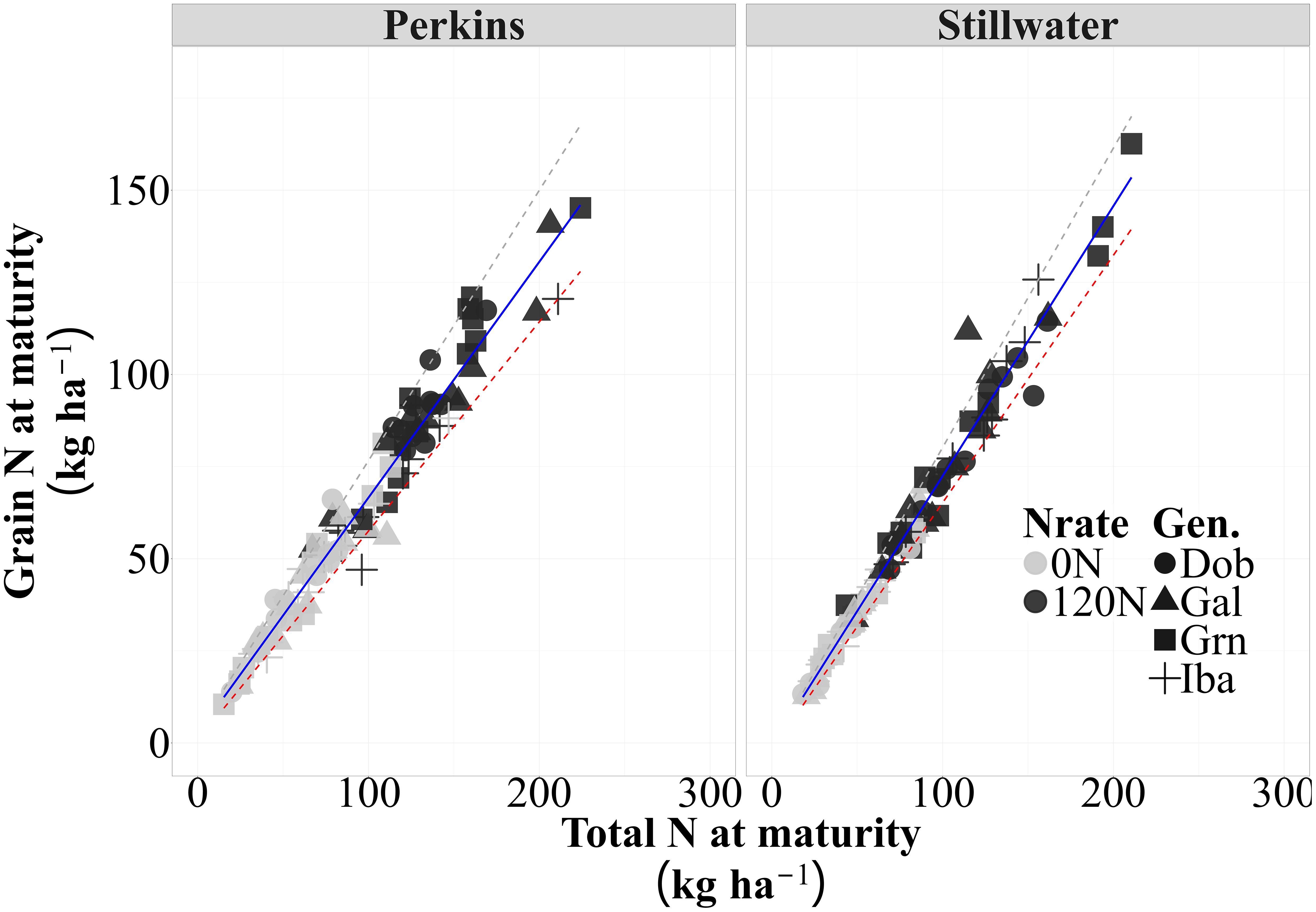
Figure 3. The relationship between grain N and total N accumulation (i.e., slope = N Harvest Index [NHI]) for each site across four genotypes, two N rates (0N and 120N) and three growing seasons (2020, 2021, 2023) (n=96) (Perkins, y = 0.64x+2.6, R2 = 0.96; Stillwater, y = 0.77x+3.09, R2=0.98). The solid lines are best-fitted functions for this relationship, and the dashed lines are the 5% and 95% quantiles (minimum and maximum, respectively).
Grain N sources: Post-flowering N accumulation (PostN), N remobilization to the grain (RemN), remobilized N from individual vegetative organs (RemNorgans) from anthesis to maturity
High and low protein genotypes did not change their PostN uptake and N remobilization dynamics when tested under low and high N rate (Table 3). While there were no statistical differences between genotypes and their interaction with the N rate, the 120N treatment increased the N remobilized from individual vegetative organs in both sites (p<0.01) (Table 3; Supplementary Table S7). Thus, genotypes with different GPC levels had similar N remobilization from individual vegetative organs and the sum of all vegetative organs. When comparing the RemN of plant organs averaged over genotypes and growing seasons, the stem remobilized more N from anthesis to maturity than the chaff and was not statistically different from leaf in both sites (Figure 4).
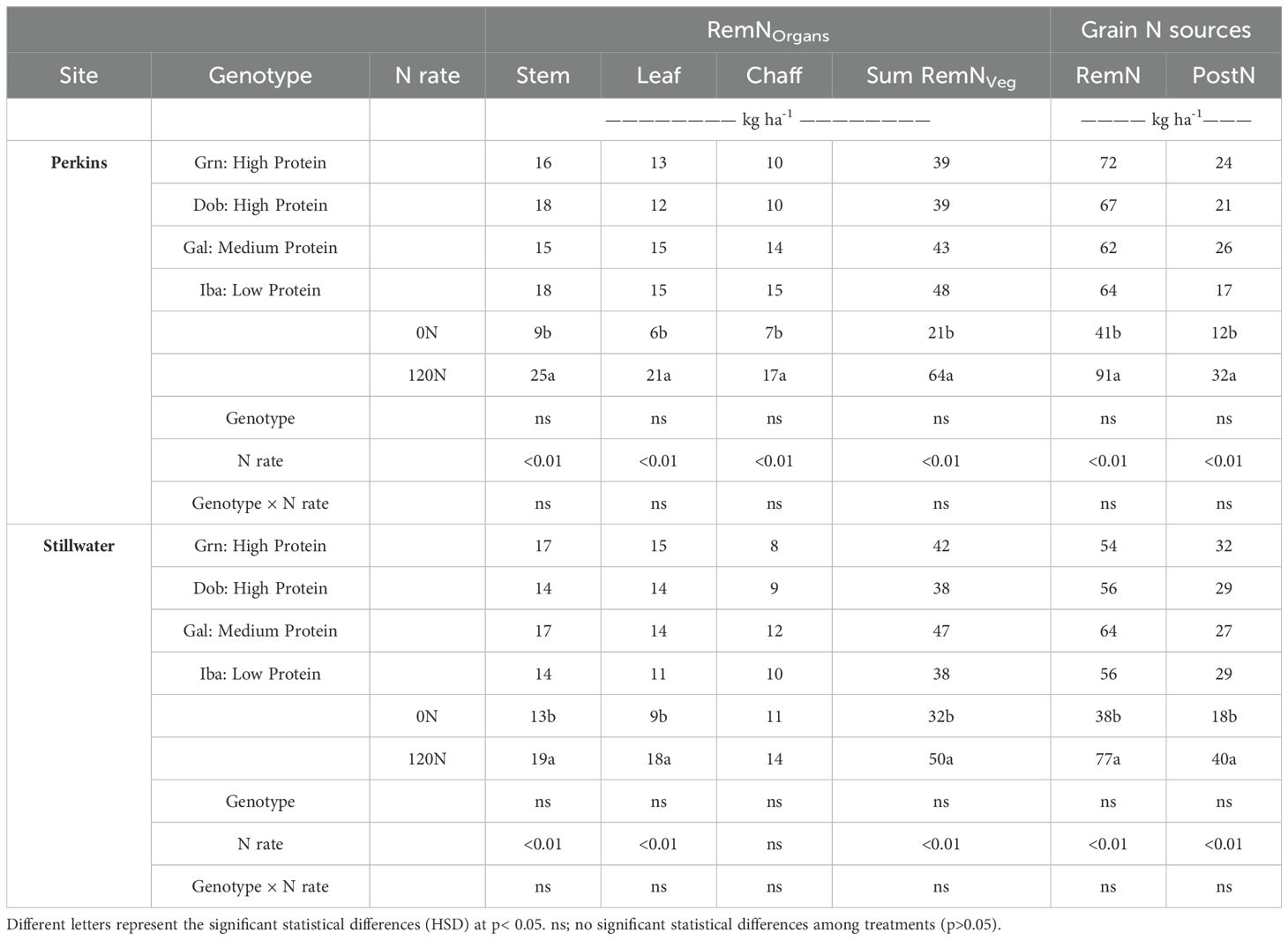
Table 3. Nitrogen remobilization from vegetative organs from anthesis to maturity (plant component N uptake at anthesis minus plant component N uptake at maturity, RemNOrgans), the sum of N remobilization from all vegetative organs (Sum RemNVeg), whole plant N remobilization (RemN; whole plant N uptake at anthesis minus stover N uptake at maturity) and post-flowering N uptake (PostN, whole plant N uptake at maturity minus whole plant N uptake at anthesis) for each genotype, N rate, and site averaged over four growing seasons.
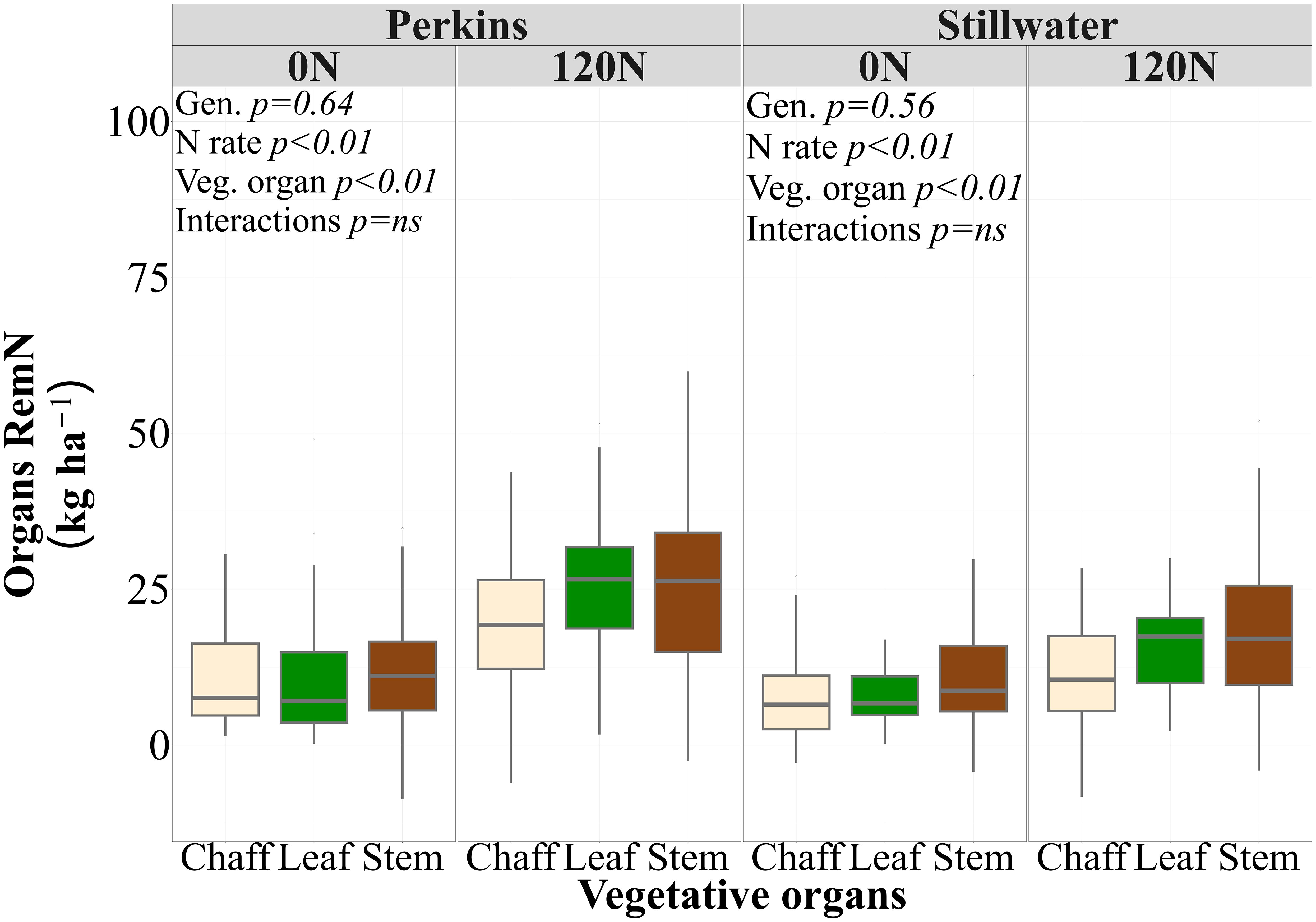
Figure 4. Nitrogen remobilization of each vegetative organ (Organs RemN, kg ha-1) at two N rates (0N and 120N) and each site (Perkins and Stillwater) averaged across four genotypes and three growing seasons (2020, 2021, 2023) (n=96). Different letters represent statistical difference among plant organs on average of N rates, genotypes, and growing seasons at p< 0.05.
Relationship between the proportional contribution of RemN of each organ and NNI
There was a positive relationship between the proportional contribution of RemNleaf to the Sum RemNVeg (%) and NNI, indicating that under favorable N conditions (higher NNI), a more significant proportion of the Sum RemNVeg was contributed by the leaves relative to other organs (Figure 5A; Supplementary Figure S6). The high-protein genotypes (Grn and Dob) exhibited a higher proportional contribution of RemNleaf compared to the low-protein genotype (Iba), as evidenced by the 84% of data points above the trend line for high-protein genotypes. This pattern was consistent across both environments, but slightly more evident at the Perkins site, where the high-protein genotype Grn showed greater leaf N uptake at anthesis than the medium-low protein genotypes Gal and Iba (Supplementary Table S5). This suggests that genotypes with higher GPC tend to allocate a significant fraction of their Sum RemNVeg from leaves to support N accumulation during grain filling period (hypothesis 2). Contrarily, the proportional contribution of RemNstem to Sum RemNVeg exhibited a very weak and negative correlation with NNI (Perkins: r² = 0.02, Stillwater: r² = 0.03) at both sites (Figure 5B; Supplementary Figure S7). This implies that as N availability improved, the relative contribution of RemNstem decreased, perhaps, due to a shift in N allocation towards other organs, possibly leaf, for optimizing grain protein formation. Notably, the high protein genotypes Grn and Dob exhibited a more pronounced decrease in contribution RemNstem as NNI increased compared to low and medium protein genotypes, as evidenced by their data points above the trend line. This finding suggests potential strategy high protein genotypes employ to prioritize grain N accumulation over vegetative biomass. Similarly, examining the relationship between the contribution of RemNchaff to Sum RemNVeg and NNI, a weak and negative correlation was observed in both Perkins and Stillwater sites (Perkins: r² = 0.13, Stillwater: r² = 0.10) (Figure 5C; Supplementary Figure S8). This negative association indicates that the chaff contributed a lower proportion of the Sum RemNVeg under more favorable N conditions. Notably, while low and medium protein genotypes (Iba and Gal) showed a more pronounced reduction in the proportional contribution of RemNchaff, the high protein genotypes (Grn and Dob) exhibited a distinct pattern. The high-protein genotypes exhibited a distinct pattern in N remobilization under varying NNI conditions. As NNI increased, these genotypes showed a lower proportional contribution of RemNchaff to total remobilized N, with their data points falling below the trend line, especially at higher NNI values. Simultaneously, they demonstrated a higher proportional contribution of RemNleaves. This pattern indicates that under improved N status, high-protein genotypes prioritized N remobilization from leaves over chaff, potentially as a strategy to enhance GPC while maintaining efficient overall N use.
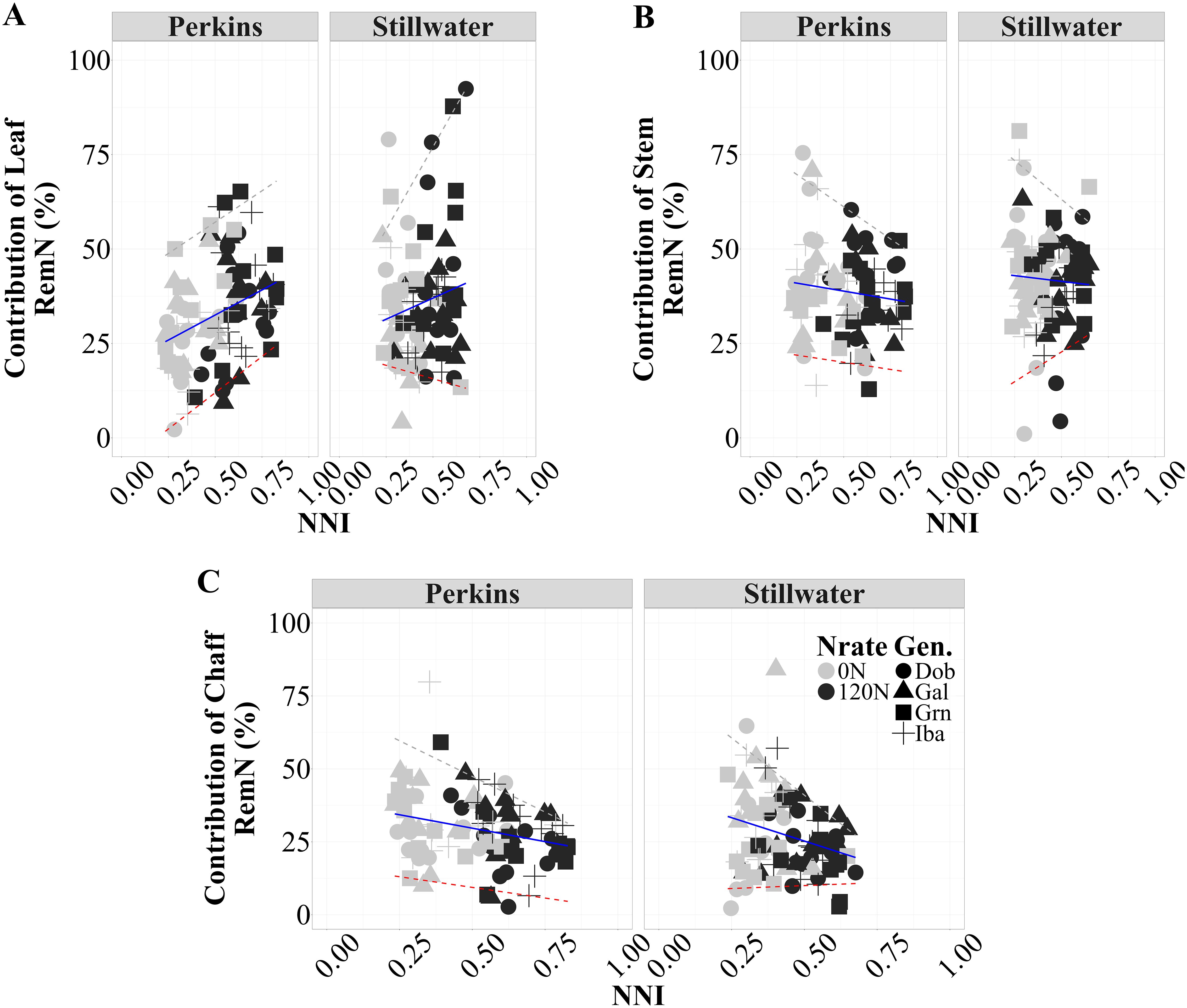
Figure 5. Relationship between contribution RemNorgan to Sum RemNVeg and NNI for each site-year across two N rates (0N and 120N) and three growing seasons (2020, 2021, 2023) (n=96). For leaf, Perkins RemNleaf vs NNI: 26.5 x + 19.3, R2 = 0.12 and Stillwater RemNleaf vs NNI: 22.4 x + 25.8, R2 = 0.03. For stem, Perkins RemNstem vs NNI: 6.04 x – 44.5, R2 < 0.02 and Stillwater RemNstem vs NNI: 6.04 x – 44.5, R2 < 0.01. For chaff, Perkins RemNchaff vs NNI: 18.4 x – 38.9, R2 = 0.07 and Stillwater RemNchaff vs NNI: 32.1 x – 41.3, R2 =0.07. The solid lines are best-fitted functions for this relationship, and the dashed lines are the 5% and 95% quantiles (minimum and maximum, respectively).
Discussion
Relationship between grain protein concentration and yield overview
The relationship between GPC and yield in wheat has been extensively studied due to its economic and end-use importance. Multiple studies have been conducted to explore the factors contributing to their negative relationship (Sieling and Kage, 2021; Tanin et al., 2022; Donaire et al., 2023), but further discussion is needed on the N uptake dynamics of genotypes with similar yield levels and different grain protein levels in dryland environments. Generally, the negative relationship between GPC and grain yield arises from competition for energy between C and N compounds in the plant, and N dilution in the grain as grain yield increases (Acreche and Slafer, 2009). The latter occurs when yield gains occur under limited N conditions (Munier‐Jolain and Salon, 2005). As demonstrated in our research and previous studies, genotypes differ in their magnitude of trade-off between GPC and yield (Cox et al., 1986; Bogard et al., 2010). Thus, understanding the physiological traits that enable genotypes to maintain or improve yield with minimal impact on GPC is relevant for the hard red wheat industry.
Genotypes performance under low and high N rates
Wheat genotypes can perform differently under low and high N conditions. Cormier et al. (2016) found that certain wheat genotypes exhibited better tolerance to N deficiency, maintaining higher biomass and grain yield under low N conditions. However, in our study, genotypes did not differ in any of the traits evaluated under low N. This discrepancy between our findings could be attributed to several factors, including the severity of N stress in our experiment, which may have been more extreme than in other studies, potentially masking genetic variation. Gaju et al. (2014) observed that under severe N stress, relationships between N uptake, remobilization, and yield components changed compared to conditions of mild N stress or high N availability. Furthermore, genotype and environment interactions play a crucial role in the expression of N use efficiency (NUE) traits. Teng et al. (2022) reported that genetic factors accounted for a more significant portion of the variation in GPC in wheat. Gaju et al. (2011, 2014) observed that wheat genotypes differed in their ability to maintain GPC under varying N supply, with some genotypes maintaining higher grain N concentrations through more efficient N remobilization from vegetative tissues. Our study corroborates with previous findings regarding varied genotypic responses under high N conditions, particularly regarding yield and GPC. This is consistent with Bogard et al. (2010), who reported that high N conditions allowed for continued N uptake during grain filling, resulting in increased grain protein accumulation for some genotypes. Barraclough et al. (2010) found that high-yielding wheat genotypes often had lower GPC, particularly under high N supply. Regarding the differences in grain N sources under low and high N rates, Kichey et al. (2007) observed that under high N conditions, some genotypes showed increased post-anthesis N uptake, which contributed more significantly to grain N accumulation compared to N remobilization from vegetative tissues. This observation does not align with our results, where N remobilization increased as compared to post-anthesis N uptake under high N conditions.
The greater NUtE exhibited by low protein genotype Iba aligns with the concept of a physiological tradeoff between NUtE and GPC which is similar to findings of previous studies (Ivić et al., 2021; Teng et al., 2022; Thompson et al., 2022). Future research efforts should focus on identifying physiological traits and management strategies to optimize both NUtE and GPC to maintain or increase yields, reduce environmental nitrogen loss, and achieve desirable protein levels while considering economic factors.
Total biomass and N accumulation at physiological maturity
Variations in GPC among genotypes may be driven by differences in other traits or N partitioning rather than total biomass. Dier et al. (2022) suggested that greater GPC in some genotypes may originate from their ability to partition N in the grain more efficiently. The subtle difference observed in Grn, however, suggests that some high-protein genotypes may indeed utilize increased N uptake as a strategy to enhance GPC. This finding underscores the complexity of the mechanisms underlying GPC variations and highlights the importance of considering both general trends and trait-specific strategies when breeding for improved grain quality.
Nitrogen partitioning to the grain
Plants with greater total N accumulation at maturity tend to allocate more N to grain, as consistently observed in wheat across various studies (Barraclough et al., 2010; De Oliveira Silva et al., 2020). The relationship between grain N uptake and total N uptake, as reflected in the NHI observed in our study, aligns with and extends previous research findings. Consistent with studies by Montemurro et al. (2006) and Rahimizadeh et al. (2010), we found that NHI plays a critical role in influencing GPC across diverse wheat genotypes and environments. Our results revealed that high-protein genotypes (Grn and Dob) demonstrated higher NHI compared to the low-protein genotype (Iba), supporting our hypothesis that high-protein genotypes exhibit increased N partitioning to the grain relative to low-protein genotypes. A higher NHI indicates that more of the absorbed N is being utilized for grain protein production, potentially reducing the amount of excess nitrogen left in crop residues or lost to the environment (Kirika, 2021). This efficient use of N can contribute to reducing environmental nitrogen pollution, a major concern in agricultural systems.
Grain N pathways: post-flowering N accumulation and N remobilization to the grain
While numerous studies have investigated management strategies and traits that could help in enhancing both GPC and grain yield without substituting one for the other (Dhillon et al., 2020; Lollato et al., 2021; Kartseva et al., 2023), no one has investigated the different remobilization strategies of genotypes with high and low protein under zero and high N conditions nor have they detailed the organ-specific contributions to N remobilization in modern wheat varieties. Across all genotypes, RemNleaf and RemNstem emerged as the primary contributors to grain N, followed by RemNchaff. This aligns with previous research showing stems and leaves as significant sources of RemN during grain-filling (Masoni et al., 2007; Egle et al., 2015). Interestingly, the effect of the N rate on RemNchaff varied depending on the environment, suggesting that environmental or management factors may influence its contribution (Dordas, 2009). Thus, the lack of significant differences among genotypes for PostN and RemN traits suggests they responded similarly to environmental conditions, underscoring the importance of N management in optimizing grain yield and GPC. These findings indicate that N availability, rather than genotypic differences, is the primary driver of these processes across all genotypes studied.
Proportional contribution organ RemN to total RemN and their relationship with NNI
Consistently with previous research (Kichey et al., 2007), leaf N remobilization plays a critical role during grain filling to sustain plant N status and attain protein levels. Similar findings from studies by Barraclough et al. (2010) and Gaju et al. (2011, 2014) also posited that in wheat, remobilizing N from the leaves during grain filling determines the ultimate grain N concentration. Conversely, the negative correlation between RemNstem contribution and NNI at both sites, suggesting a shift in nitrogen allocation as availability increases, consistent with findings by Barraclough et al. (2010). High-protein genotypes exhibited a more pronounced decrease in RemNstem contribution with increasing NNI, suggesting a distinct physiological strategy that may favor grain protein accumulation over vegetative growth (Triboi and Triboi-Blondel, 2002). Similarly, RemNchaff decreased as NNI increased, with high-protein genotypes showing lower RemNchaff but higher NNI values. These results support our hypothesis that N remobilization patterns differ between high and low protein genotypes, aligning with Pask et al. (2012) and Gaju et al. (2014) proposition that high-GPC genotypes may have evolved efficient N remobilization mechanisms.
The enhanced leaf N remobilization observed in high-protein genotypes under increased NNI is particularly noteworthy, corroborating the importance of leaf senescence and N remobilization in determining final GPC (Masclaux-Daubresse et al., 2010). These differential N partitioning strategies, especially the prioritization of leaf N remobilization in high-protein genotypes, likely contribute to their ability to maintain elevated GPC while responding to N availability conditions (Cormier et al., 2016). These findings have important implications for breeding programs aimed at developing wheat varieties with improved nitrogen use efficiency and high GPC. However, future research should consider how these N partitioning strategies may be modulated under different environmental stresses, particularly in the context of climate change and increasing water scarcity in wheat-growing regions.
Conclusion
Our study addresses the challenge of understanding nitrogen dynamics in wheat by investigating whether modern high-protein genotypes exhibited increased N accumulation (also expressed as N nutrition index, NNI) and partitioning (including remobilization from vegetative organs) compared to low-protein genotypes under low and high N conditions.
Our results support the hypothesis that N remobilization patterns differ between high- and low-protein genotypes. Leaf N remobilization played a critical role during grain filling, sustaining plant N status and contributing to protein levels. High-protein genotypes demonstrated a prioritization of N remobilization from leaves as N conditions improved, likely contributing to their ability to maintain elevated GPC. Specifically, Grn tended to achieve high protein by increasing total N uptake, while Dob exhibited a trend toward higher N partitioning to grain (NHI). These differential N partitioning strategies support the hypothesis that high-protein genotypes exhibit increased N partitioning to the grain compared to low-protein genotypes. The greater N utilization efficiency (NUtE) observed in the genotype Iba likely contributed to its lower GPC, emphasizing the trade-off between NUtE and GPC. Future research should further elucidate the genetic and physiological mechanisms underlying these N partitioning strategies in different organs during the entire growing season in high-protein genotypes. This knowledge could lead to more targeted breeding efforts and management practices that balance the competing demands of high yield, high GPC, and efficient N use in wheat production.
Data availability statement
The original contributions presented in the study are included in the article/Supplementary Material. Further inquiries can be directed to the corresponding author.
Author contributions
SA: Data curation, Formal analysis, Investigation, Visualization, Writing – original draft. JL: Visualization, Writing – review & editing. BC: Conceptualization, Methodology, Writing – review & editing. BA: Conceptualization, Writing – review & editing. IC: Conceptualization, Visualization, Writing – review & editing. AO: Conceptualization, Data curation, Funding acquisition, Investigation, Methodology, Project administration, Resources, Supervision, Validation, Visualization, Writing – review & editing.
Funding
The author(s) declare that financial support was received for the research, authorship, and/or publication of this article. This research was partially funded by the Oklahoma Wheat Commission and the Oklahoma Wheat Research Foundation (OSU internal grant number 2-544560).
Acknowledgments
The authors thank Tyler Lynch, the Senior Agriculturalist of the Small Grains Program, and all past small grains lab assistants for their assistance in managing field trials and data collection.
Conflict of interest
The authors declare that the research was conducted in the absence of any commercial or financial relationships that could be construed as a potential conflict of interest.
Publisher’s note
All claims expressed in this article are solely those of the authors and do not necessarily represent those of their affiliated organizations, or those of the publisher, the editors and the reviewers. Any product that may be evaluated in this article, or claim that may be made by its manufacturer, is not guaranteed or endorsed by the publisher.
Supplementary material
The Supplementary Material for this article can be found online at: https://www.frontiersin.org/articles/10.3389/fpls.2024.1493901/full#supplementary-material
References
Acreche, M. M., Slafer, G. A. (2009). Grain weight, radiation interception, and use efficiency as affected by sink-strength in Mediterranean wheats released from 1940 to 2005. Field Crops Res. 110, 98–105. doi: 10.1016/j.fcr.2008.07.006
Barbottin, A., Lecomte, C., Bouchard, C., Jeuffroy, M. H. (2005). Nitrogen remobilization during grain filling in wheat: genotypic and environmental effects. Crop Sci. 45, 1141–1150. doi: 10.2135/cropsci2003.0361
Barraclough, P. B., Howarth, J. R., Jones, J., Lopez-Bellido, R., Parmar, S., Shepherd, C. E., et al. (2010). Nitrogen efficiency of wheat: genotypic and environmental variation and prospects for improvement. Eur. J. Agron. 33, 1–11. doi: 10.1016/j.eja.2010.01.005
Bates, D., Mächler, M., Bolker, B., Walker, S. (2015). Fitting linear mixed-effects models using lme4. J. Stat. Softw. 67, 1–48. doi: 10.18637/jss.v067.i01
Biradar, S. S., Patil, M. K., Desai, S. A., Singh, S. K., Naik, V. R., Lamani, K., et al. (2024). Nitrogen use efficiency in bread wheat: Genetic variation and prospects for improvement. PloS One 19, e0294755. doi: 10.1371/journal.pone.0294755
Bogard, M., Allard, V., Brancourt-Hulmel, M., Heumez, E., MacHet, J. M., Jeuffroy, M. H., et al. (2010). Deviation from the grain protein concentration-grain yield negative relationship is highly correlated to post-anthesis N uptake in winter wheat. J. Exp. Bot. 61, 4303–4312. doi: 10.1093/jxb/erq238
Chen, Y., Chen, H., Chen, R., Yang, H., Zheng, T., Huang, X., et al. (2023). The impacts of nitrogen accumulation, translocation, and photosynthesis on simultaneous improvements in the grain yield and gluten quality of dryland wheat. Agronomy 13, 1283. doi: 10.3390/agronomy13051283
Chen, Y., Xiao, C., Wu, D., Xia, T., Chen, Q., Chen, F., et al. (2015). Effects of nitrogen application rate on grain yield and grain nitrogen concentration in two maize hybrids with contrasting nitrogen remobilization efficiency. Eur. J. Agron. 62, 79–89. doi: 10.1016/j.eja.2014.09.008
Ciampitti, I. A., Vyn, T. J. (2013). Grain nitrogen source changes over time in maize: a review. Crop Sci. 53, 366–377. doi: 10.2135/cropsci2012.07.0439
Cormier, F., Foulkes, J., Hirel, B., Gouache, D., Moënne-Loccoz, Y., Le Gouis, J. (2016). Breeding for increased nitrogen-use efficiency: a review for wheat (T. aestivum L.). Plant Breed. 135, 255–278. doi: 10.1111/pbr.2016.135.issue-3
Cox, M. C., Qualset, C. O., Rains, D. W. (1986). Genetic variation for nitrogen assimilation and translocation in wheat. III. Nitrogen translocation in relation to grain yield and protein. Crop Sci. 26, 737–740. doi: 10.2135/cropsci1985.0011183X002500030003x
De Oliveira Silva, A., Carver, B., Aoun, M., Lynch, T., Cyrineu, I. M., Abiola, S. O. (2023). 2022-2023 Small Grains Variety Performance Tests (Oklahoma State, United States of America: Oklahoma Cooperative Extension Service). CR-2143.
De Oliveira Silva, A., Ciampitti, I. A., Slafer, G. A., Lollato, R. P. (2020). Nitrogen utilization efficiency in wheat: A global perspective. Eur. J. Agron. 114, 126008. doi: 10.1016/j.eja.2020.126008
Desai, R. M., Bhatia, C. R. (1978). Nitrogen uptake and nitrogen harvest index in durum wheat cultivars varying in their grain protein concentration. Euphytica 27, 561–566. doi: 10.1007/BF00043182
Dhillon, J., Eickhoff, E., Aula, L., Omara, P., Weymeyer, G., Nambi, E., et al. (2020). Nitrogen management impact on winter wheat grain yield and estimated plant nitrogen loss. Agron. J. 112, 564–577. doi: 10.1002/agj2.v112.1
Dier, M., Hüsken, A., Mikolajewski, S., Langenkämper, G., Zörb, C. (2022). Analyzing a saturation effect of nitrogen fertilization on baking volume and grain protein concentration in wheat. Agriculture 13, 20. doi: 10.3390/agriculture13010020
Donaire, G., Vanzetti, L. S., Conde, M. B., Bainotti, C., Mir, L., Borrás, L., et al. (2023). Dissecting genetic loci of yield, yield components, and protein content in bread wheat nested association mapping population. Euphytica 219, 65. doi: 10.1007/s10681-023-03195-0
Dordas, C. (2009). Dry matter, nitrogen and phosphorus accumulation, partitioning and remobilization as affected by N and P fertilization and source–sink relations. Eur. J. Agron. 30, 129–139. doi: 10.1016/j.eja.2008.09.001
Egle, K., Beschow, H., Merbach, W. (2015). Nitrogen allocation in barley: relationships between amino acid transport and storage protein synthesis during grain filling. Can. J. Plant Sci. 95, 451–459. doi: 10.4141/cjps2013-324
FAO (2019). FAO-STAT: Commodities by Country. Available online at: http://www.fao.org/faostat/en/rankings/countries_by_commodity (Accessed 15 April, 2024).
Fortunato, S., Nigro, D., Lasorella, C., Marcotuli, I., Gadaleta, A., de Pinto, M. C. (2023). The role of glutamine synthetase (GS) and glutamate synthase (GOGAT) in the improvement of nitrogen use efficiency in cereals. Biomolecules 13, 1771. doi: 10.3390/biom13121771
Gaju, O., Allard, V., Martre, P., Le Gouis, J., Moreau, D., Bogard, M., et al. (2014). Nitrogen partitioning and remobilization in relation to leaf senescence, grain yield and grain nitrogen concentration in wheat cultivars. Field Crops Res. 155, 213–223. doi: 10.1016/j.fcr.2013.09.003
Gaju, O., Allard, V., Martre, P., Snape, J. W., Heumez, E., Le Gouis, J. (2011). Identification of traits to improve the nitrogen-use efficiency of wheat genotypes. Field Crop Res. 123, 139–152. doi: 10.1016/j.fcr.2011.05.010
Hothorn, T., Bretz, F., Westfall, P. (2008). Simultaneous inference in general parametric models. Biometr. J. 50, 346–363. doi: 10.1002/bimj.200810425
Ivić, M., Grljušić, S., Plavšin, I., Dvojković, K., Lovrić, A., Rajković, B., et al. (2021). Variation for nitrogen use efficiency traits in wheat under contrasting nitrogen treatments in South-Eastern Europe. Front. Plant Sci. 12, 682333. doi: 10.3389/fpls.2021.682333
Justes, E., Mary, B., Meynard, J. M., Machet, J. M., Thelier-Huché, L. (1994). Determination of a critical nitrogen dilution curve for winter wheat crops. Ann. Bot. 74, 397–407. doi: 10.1006/anbo.1994.1133
Kartseva, T., Alqudah, A. M., Aleksandrov, V., Alomari, D. Z., Doneva, D., Arif, M. A. R., et al. (2023). Nutritional genomic approach for improving grain protein content in wheat. Foods 12, 1399. doi: 10.3390/foods12071399
Kaur, G., Asthir, B., Bains, N. S., Farooq, M. (2015). Nitrogen nutrition, its assimilation and remobilization in diverse wheat genotypes. Int. J. Agric. Biol. 17 (3), 531–538. doi: 10.17957/IJAB/17.3.14.539
Kichey, T., Hirel, B., Heumez, E., Dubois, F., Le Gouis, J. (2007). In winter wheat (Triticum aestivum L.), post-anthesis nitrogen uptake and remobilisation to the grain correlates with agronomic traits and nitrogen physiological markers. Field Crop Res. 102, 22–32. doi: 10.1016/j.fcr.2007.01.002
Kirika, M. W. (2021). Understanding Nitrogen Uptake, Partitioning and Remobilization to Improve Grain Protein Content in Wheat. Thesis (Ph.D.) University of Adelaide, School of Agriculture, Food and Wine, Australia. Available online at: https://digital.library.adelaide.edu.au/items/bf20d3ba-24b1-4794-827a-e662b9d50c38.
Kuznetsova, A., Brockhoff, P. B., Christensen, R. H. B. (2017). lmerTest package: tests in linear mixed effects models. J. Stat. softw. 82 (13). doi: 10.18637/jss.v082.i13
Lebedev, V. G., Popova, A. A., Shestibratov, K. A. (2021). Genetic engineering and genome editing for improving nitrogen use efficiency in plants. Cells 10, 3303. doi: 10.3390/cells10123303
Lemaire, G., Jeuffroy, M. H., Gastal, F. (2008). Diagnosis tool for plant and crop N status in vegetative stage: Theory and practices for crop N management. Eur. J. Agron. 28, 614–624. doi: 10.1016/j.eja.2008.01.005
Lollato, R. P., Jaenisch, B. R., Silva, S. R. (2021). Genotype-specific nitrogen uptake dynamics and fertilizer management explain contrasting wheat protein concentrations. Crop Sci. 61, 2048–2066. doi: 10.1002/csc2.20442
Masclaux-Daubresse, C., Daniel-Vedele, F., Dechorgnat, J., Chardon, F., Gaufichon, L., Suzuki, A. (2010). Nitrogen uptake, assimilation and remobilization in plants: challenges for sustainable and productive agriculture. Ann. Bot. 105, 1141–1157. doi: 10.1093/aob/mcq028
Masoni, A., Ercoli, L., Mariotti, M., Arduini, I. (2007). Post-anthesis accumulation and remobilization of dry matter, nitrogen and phosphorus in durum wheat as affected by soil type. Eur. J. Agron. 26, 179–186. doi: 10.1016/j.eja.2006.09.006
Michel, S., Löschenberger, F., Ametz, C., Pachler, B., Sparry, E., Bürstmayr, H. (2019). Simultaneous selection for grain yield and protein content in genomics-assisted wheat breeding. Theor. Appl. Genet. 132, 1745–1760. doi: 10.1007/s00122-019-03312-5
Moll, R. H., Kamprath, E. J., Jackson, W. A. (1982). Analysis and interpretation of factors which contribute to efficiency of nitrogen utilization. Agron. J. 74, 562–564. doi: 10.2134/agronj1982.00021962007400030037x
Montemurro, F., Maiorana, M., Ferri, D., Convertini, G. (2006). Nitrogen indicators, uptake and utilization efficiency in a maize and barley rotation cropped at different levels and sources of N fertilization. Field Crops Res. 99, 114–124. doi: 10.1016/j.fcr.2006.04.001
Munier-Jolain, N. G., Salon, C. (2005). Are the carbon costs of seed production related to quantitative and qualitative performance? An appraisal for legumes and other crops. Plant Cell Environ. 28, 1388–1395. doi: 10.1111/j.1365-3040.2005.01371.x
Ortez, O. A., Tamagno, S., Salvagiotti, F., Prasad, P. V., Ciampitti, I. A. (2019). Soybean nitrogen sources and demand during the seed-filling period. Agron. J. 111, 1779–1787. doi: 10.2134/agronj2018.10.0656
Padhy, A. K., Kaur, P., Singh, S., Kashyap, L., Sharma, A. (2024). Colored wheat and derived products: Key to global nutritional security. Crit. Rev. Food Sci. Nutr. 64, 1894–1910. doi: 10.1080/10408398.2022.2119366
Palta, J. A., Fillery, I. R. P. (1995). N application enhances remobilization and reduces losses of pre-anthesis N in wheat grown on a duplex soil. Aust. J. Agric. Res. 46, 519–531. doi: 10.1071/AR9950519
Pan, W. L., Kidwell, K. K., McCracken, V. A., Bolton, R. P., Allen, M. (2020). Economically optimal wheat yield, protein and nitrogen use component responses to varying N supply and genotype. Front. Plant Sci. 10, 1790. doi: 10.3389/fpls.2019.01790
Pask, A. J. D., Sylvester-Bradley, R., Jamieson, P. D., Foulkes, M. J. (2012). Quantifying how winter wheat crops accumulate and use nitrogen reserves during growth. Field Crops Res. 126, 104–118. doi: 10.1016/j.fcr.2011.09.021
Rahimizadeh, M., Kashani, A., Zare-Feizabadi, A., Koocheki, A. R., Nassiri-Mahallati, M. (2010). Nitrogen use efficiency of wheat as affected by preceding crop, application rate of nitrogen and crop residues. Aust. J. Crop Sci. 4, 363–368. doi: 10.3316/informit.414715022907778
Rodriguez, I. M., Lacasa, J., Lemaire, G., Zhao, B., Ata-Ul-Karim, S. T., Ciampitti, I. A. (2024). Crop nitrogen status and yield formation: A cross-species comparison for maize, rice, and wheat field crops. Field Crops Res. 316, 109515. doi: 10.1016/j.fcr.2024.109515
Sadras, V. O., Lemaire, G. (2014). Quantifying crop nitrogen status for comparisons of agronomic practices and genotypes. Field Crops Res. 164, 54–64. doi: 10.1016/j.fcr.2014.05.006
Sendhil, R., Kumari, B., Khandoker, S., Jalali, S., Acharya, K. K., Gopalareddy, K., et al. (2022). “Wheat in Asia: trends, challenges and research priorities,” in New Horizons in Wheat and Barley Research: Global Trends, Breeding and Quality Enhancement (Springer Singapore, Singapore), 33–61.
Sieling, K., Kage, H. (2021). Apparent fertilizer N recovery and the relationship between grain yield and grain protein concentration of different winter wheat varieties in a long-term field trial. Eur. J. Agron. 124, 126246. doi: 10.1016/j.eja.2021.126246
Sosulski, F. W., Imafidon, G. I. (1990). Amino acid composition and nitrogen-to-protein conversion factors for animal and plant foods. J. Agric. Food Chem. 38, 1351–1356. doi: 10.1021/jf00096a011
Tanin, M. J., Sharma, A., Saini, D. K., Singh, S., Kashyap, L., Srivastava, P., et al. (2022). Ascertaining yield and grain protein content stability in wheat genotypes having the Gpc-B1 gene using univariate, multivariate, and correlation analysis. Front. Genet. 13, 1001904. doi: 10.3389/fgene.2022.1001904
Taulemesse, F., Le Gouis, J., Gouache, D., Gibon, Y., Allard, V. (2015). Post-flowering nitrate uptake in wheat is controlled by N status at flowering, with a putative major role of root nitrate transporter NRT2. 1. PloS One 10, e0120291. doi: 10.1371/journal.pone.0120291
Teng, W., He, X., Tong, Y. (2022). Genetic control of efficient nitrogen use for high yield and grain protein concentration in wheat: A Review. Plants 11, 492. doi: 10.3390/plants11040492
Thompson, M., Okamoto, M., Martin, A., Seneweera, S. (2022). Grain protein concentration at elevated [CO2] is determined by genotype dependent variations in nitrogen remobilisation and nitrogen utilisation efficiency in wheat. Plant Physiol. Biochem. 192, 120–128. doi: 10.1016/j.plaphy.2022.10.003
Triboi, E., Triboi-Blondel, A. M. (2002). Productivity and grain or seed composition: a new approach to an old problem. Eur. J. Agron. 16, 163–186. doi: 10.1016/S1161-0301(01)00146-0
Whitfield, D. M., Smith, C. J. (1992). Nitrogen uptake, water use, grain yield and protein content in wheat. Field Crops Res. 29, 1–14. doi: 10.1016/0378-4290(92)90071-G
Keywords: nitrogen use efficiency, nutrient partitioning, crop physiology, genotype selection, plant nutrition, breeding strategies, agronomic practices, N remobilization patterns
Citation: Abiola SO, Lacasa J, Carver BF, Arnall BD, Ciampitti IA and de Oliveira Silva A (2024) Nitrogen uptake dynamics of high and low protein wheat genotypes. Front. Plant Sci. 15:1493901. doi: 10.3389/fpls.2024.1493901
Received: 09 September 2024; Accepted: 26 November 2024;
Published: 16 December 2024.
Edited by:
Elias Kaiser, Wageningen University and Research, NetherlandsReviewed by:
Francesco Sunseri, Mediterranea University of Reggio Calabria, ItalyJoão Ricardo Bachega Feijó Rosa, RB Genetics & Statistics Consulting, Brazil
Copyright © 2024 Abiola, Lacasa, Carver, Arnall, Ciampitti and de Oliveira Silva. This is an open-access article distributed under the terms of the Creative Commons Attribution License (CC BY). The use, distribution or reproduction in other forums is permitted, provided the original author(s) and the copyright owner(s) are credited and that the original publication in this journal is cited, in accordance with accepted academic practice. No use, distribution or reproduction is permitted which does not comply with these terms.
*Correspondence: Amanda de Oliveira Silva, c2lsdmFhQG9rc3RhdGUuZWR1