- 1International Maize and Wheat Improvement Center (CIMMYT), World Agroforestry Centre (ICRAF), United Nations Avenue, Nairobi, Kenya
- 2West Africa Centre for Crop Improvement (WACCI), University of Ghana, Accra, Ghana
- 3Maize Improvement Program (MIP), The Council for Scientific and Industrial Research (CSIR)-Savanna Agricultural Research Institute, Tamale, Ghana
Drought is a major constraint on maize (Zea mays L.) production and productivity in Sub-Saharan Africa (SSA). The increase in frequency and severity of drought, driven by climate change, is expected to worsen in the future. These occurrences are likely to adversely affect maize production and productivity, threatening the economic and social stability of millions of smallholder farmers. Understanding the genetics of hybrid performance under drought stress is crucial for designing breeding strategies to develop high-yielding hybrids. This study aimed to (i) evaluate the performance of three-way cross hybrids developed from elite inbred lines, including several drought-tolerant lines, using a line-by-tester mating design, and (ii) estimate the general combining ability (GCA) and specific combining ability (SCA) effects of the tropical maize inbred lines under managed drought and optimum conditions. A total of 265 maize inbred lines from the CIMMYT global maize breeding program were used as parents and crossed to six single cross testers to generate 795 testcross hybrids. These hybrids, along with six commercial hybrids as a check, were evaluated under managed drought and optimum conditions. Significant (p < 0.001) variations were observed among genotypes and genotypes-by-environment interactions (GEIs) for grain yield and other traits. There was a preponderance of GCA variance (lines and tester) over SCA variance, indicating that additive effects were more important in determining grain yield and other key traits under both managed drought and optimum conditions. Ten inbred lines (S2_8, S10_1, S6_4, S10_14, S2_14, S10_15, S8_7, S2_3, S8_15, and S13_5) with desirable GCA effects for grain yield and other traits were identified. Fourteen testcross hybrids were identified with high grain yield and desirable agronomic traits under both drought and optimum conditions. The identified lines and hybrids are useful sources to be used in breeding and deploying as stress-tolerant hybrids. High correlations observed between observed and GCA-predicted hybrid performance suggest the possibility to evaluate more hybrids with fixed resources. The study demonstrates that it is feasible to obtain high-yielding and drought-tolerant lines and hybrids. These testcross hybrids should undergo rigorous on-farm trials to ensure consistent performance before commercialization and release. Deploying these hybrids could help in mitigating the effects of drought stress in SSA and contribute to improved maize productivity in the region.
Introduction
Maize (Zea mays L.) is the most widely consumed cereal crop and is grown on more than 40 million hectares of arable land in Sub-Saharan Africa (SSA) (Cairns et al., 2021). Maize contributes more than 30% of the daily calorie intake (varying from 50 to 30 g/person/day) (Prasanna et al., 2020b; Erenstein et al., 2022). Millions of households in SSA are dependent on maize for food and nutritional security and for providing income for securing their basic necessities of life (Ekpa et al., 2018; Santpoort, 2020; Poole et al., 2021; Food and Agriculture Organization (FAO), 2022).
Currently, maize yields in SSA are extremely low and exhibit significant variability, with an average yield ranging between 1 and 3 t/ha (Prasanna et al., 2022), which is significantly lower than the global average of 5 t/ha. The variability in maize yields in the maize-producing regions of SSA is attributed to a combination of biotic and abiotic stresses (Cairns and Prasanna, 2018; Prasanna et al., 2021; Ndlovu et al., 2022). With frequent occurring drought in the region being a key limiting abiotic factor, its negative impact makes it quite challenging to fully unlock the maximum yield potential of the maize (Pinho et al., 2022). Drought is expected to increase in both frequency and severity, which further exacerbates the declining yields in maize production zones in SSA, due to the continued pressure of climate change, rising temperatures, and irregular rainfall distribution (Cairns et al., 2021; Nurmberg et al., 2022; Cooper and Messina, 2023). Approximately 40% of the “maize belt” in SSA encounters periodic drought, leading to significant yield losses ranging between 10% and 25%. An additional 25% of the area is severely impacted by recurrent drought, which directly contributes to substantial harvest yield losses of up to 50% (Fisher et al., 2015). Recent studies have shown that drought stress is expected to inflict maize yield losses of up to 1.7% for each degree day spent above 30°C (Zhao et al., 2017; Ray et al., 2019; Prasanna et al., 2021).
The magnitude of drought-induced yield loss in maize varies from 30% to 90%, which largely depends on the maize growth stage, type of variety, and the duration/intensity of water deficit (Mcmillen et al., 2022; Prasanna et al., 2020a; Sheoran et al., 2022). It is therefore not surprising that smallholder farmers in SSA have high preference and demand for drought-tolerant maize varieties for cultivation. Farmers and other actors in the maize value chain consider drought tolerance as a “must-have” trait and has become a key breeding objective for most maize breeding program in SSA (Beyene et al., 2021).
The International Maize and Wheat Improvement Center (CIMMYT) in collaboration with national agriculture research organizations developed and released a wide range of high-yielding drought-tolerant maize inbred lines, hybrids, and open pollinated varieties for different maturity groups and adaptations in SSA. These contributed to significant genetic gain for grain yield (GY) under managed drought (32.5 kg ha−1 year−1) and random drought stress (22.7 kg ha−1 year−1) conditions (Badu-Apraku and Fakorede, 2017; Masuka et al., 2017; Prasanna et al., 2020a; Prasanna et al., 2022). Although significant gains in breeding for drought-tolerant varieties have been achieved, these gains are inadequate to meet future demand due to population growth, global climate change with unpredictable rainfall pattern, and rising temperature, particularly in SSA (Masuka et al., 2017; Cairns and Prasanna, 2018; Barbosa et al., 2021). Therefore, genetic improvement and breeding for improved tolerance in tropical maize germplasm are highly relevant strategies to continue to guarantee food security, reduce smallholder farmers’ vulnerability to drought, and mitigate against climate change.
Development of drought-tolerant maize varieties largely depends on the availability of genetic variation and carefully selecting appropriate parental lines that show high performance per se and can transmit favorable genes/alleles to their progeny. For the effective and judicious use of elite maize germplasm, basic genetic information about the breeding value of a new set of inbred lines can help to accelerate the development of high-yielding cultivars with desirable traits (e.g., drought tolerance). This is critical in establishing an appropriate breeding plan or strategy that can be employed to effectively improve the development of productive hybrids with improved drought tolerance.
Combining ability is the ability of parental lines to effectively pass on genes/alleles to their progenies. Genetic mating designs including diallel mating designs (Griffing, 1956); North Carolina design I, II, and III (Comstock and Robinson, 1948); and line-by-tester mating design (Hallauer et al., 2010) are commonly used for the evaluation of the combining ability of new inbred lines and for providing key information about the genetic control of desirable traits. Among these mating designs, the line-by-tester design involves crossing few known testers to a large number of inbred lines that allow one to estimate the lines’ combining ability and breeding value (Acquaah, 2012). Early-stage testcross evaluation using the line-by-tester design enables the identification of inbred lines that are good combiners with high or low per se performance, which save time and money by helping breeders to discard poor combiners at the early stage of testing in breeding.
Over the years, numerous studies have been conducted to evaluate maize inbred lines for their combining ability, breeding values, per se performance, and gene activity under drought and optimum conditions (Betrán et al., 2003b; Makumbi et al., 2011; Oyekunle, 2014; Badu-Apraku et al., 2015; Trachsel et al., 2016; Beyene et al., 2017; Osuman et al., 2022; Sang et al., 2022; Matongera et al., 2023). Using the line-by-tester design, Oluwaseun et al. (2022) and Ertiro et al. (2017) reported that additive gene activity was more important in regulating GY and other traits under drought stress. In contrast, Annor et al. (2019), using the line-by-tester design, reported that non-additive gene effects were more important than additive gene action in controlling GY under drought stress. The conflicting results on gene activity controlling yield and other traits under drought conditions can be attributed to variations in the genetic background of inbred lines and the environment conditions in which the lines are evaluated. Therefore, it is crucial for breeders to determine the combining ability of a new set of maize inbred lines and their gene action derived from populations of diverse sources. This enables the selection of superior inbred lines that contribute desirable alleles/genes to their crosses, which can be used as donors in genetic improvement for drought tolerance and the production of highly productive hybrids. In eastern and southern Africa, CIMMYT is developing improved drought-tolerant and other multiple stress-resilient, high-yielding potential inbred lines, which helps to achieve high genetic gain under smallholder farming conditions. Understanding the genetic potential of newly developed inbred lines in hybrid combinations is critical for their effective utilization. Therefore, combining ability analysis of the newly developed elite drought-tolerant inbred lines is a vital tool to identify and select the most desirable inbred lines for the development of high-yielding and disease-resistant hybrids adapted to the target environments in the region. The objectives of this study were to (i) evaluate the performance of three-way cross hybrids under drought and optimum conditions and (ii) estimate the GCA and SCA effects of a new set of tropical maize inbred lines for GY and other agronomic traits under managed drought and optimum conditions.
Materials and methods
Plant materials and hybridization
A total of 265 new inbred lines derived from CIMMYT’s east Africa mid-altitude medium maturity breeding program (EA-PP1) were used in the present study. The selected 265 lines were developed from a set of elite drought donor lines in an elite-by-elite line combination. Specific drought donors like LapostaSequia-C7-F71, LapostaSequia-C7-F78, LapostaSequia-C7-F103, LapostaSequia-C7-F180, CML386, and DTPWC9 were used to develop these lines. In addition to drought tolerance, lines with good GCA and per se performance for high yield potential as well as resistance to several foliar diseases were also included in this study. An incomplete line-by-tester design (Kempthorne, 1957) was used to generate 795 testcrosses by crossing 265 lines to six single-cross testers. A total of 93 lines belong to CIMMYT heterotic group A while the remaining 172 lines belong to heterotic group B. All lines were crossed to three testers from opposite heterotic groups (Supplementary Table S1). Pollen from each line was used to manually pollinate the single cross testers. The testcrosses were formed during the short rainy season (October–March) in the Kiboko maize research station in Kenya. The inbred lines were selected through rigorous phenotypic evaluations in the breeding nurseries and screened for foliar diseases. The testers used in the study are good combiners and are used as a female parent to adapt the three-way hybrid to mid-altitude environments. Detailed information on the pedigree of the inbred line is presented in Supplementary Table S1.
Experimental design and trial management
A total of 795 testcrosses were evaluated in a multilocation trial under optimum and managed drought conditions. The experiments were interconnected by six commercial hybrid checks (DK8031, H513, PH3253, Pioneer 3253, DH04, and WH505). An Alpha (0,1) lattice experimental design with two replications was used. Each genotype was planted in two-row plots of 5 m in length, spaced at 0.75 m between rows and 0.25 m between hills. Each hill was planted with two seeds and later thinned to one plant per hill at 3 weeks after seedling emergence to adjust the final plant population to 53,333 plants/ha. Basal fertilizer application was performed at planting using di-ammonium phosphate (DAP) fertilizer at the rate of 60 kg N and 60 kg P2O5 per hectare. Six weeks after emergence, all experiments were top dressed with nitrogen fertilizer at the rate of 60 kg N/ha. All the experiments were kept weed-free by manual weeding and herbicide control.
The testcrosses were evaluated in Kenya at four locations under optimum management (Kakamega, Kiboko, Kirinyaga, and Shikutza) and at three locations (Kiboko, Homabay, and Mtwapa) under drought stress conditions (Table 1). Drought stress experiments were conducted in the off season where it does not rain during cropping period. The drought stress fields are irrigated with a drip irrigation system. Irrigation was applied twice weekly for 3 to 5 h depending on the potential evapotranspiration (Trachsel et al., 2016). Irrigation of the trials was stopped at ~750 GDD after planting (2 weeks before the anticipated date of flowering) to induce drought stress (Trachsel et al., 2016; Ertiro et al., 2017). The well-watered or optimum trials were conducted in the main season under rainfed conditions. The trials were also supplemented with irrigation throughout the growth cycle to avoid drought stress. The management of drought stress experiment and phenotyping were according to the procedures outlined in the drought phenotyping protocols by CIMMYT (Zaman-Allah et al., 2016; Zaidi, 2019).
Data collection
Data were collected per plot for each experiment under managed drought and optimum conditions for days to 50% anthesis (AD, as number of days after planting when 50% of the plants per plot shed pollen), days to 50% silking (SD, as number of days after planting when 50% of the plants per plot show silks), anthesis–silking interval (ASI) (as the difference between AD and SD), plant height (PH, measured as the length in centimeters from the base of a plant to the insertion of the first tassel branch of the same plant for 10 representative plants per plot), and ear height (EH, measured as the length in centimeters from the base of a plant to the internode of the top ear of the same plant for 10 representative plants per plot). At harvest, field weight [weight of dehusked ears (cobs) in kilograms per plot] and grain moisture (MOI, measured using a moisture meter on grain sampled from the center of five representative ears per plot) were recorded. GY was calculated using the field weight of ears per plot and a shelling percentage of 80, and adjusted to a moisture content of 12.5%. For drought trials, all trait measurements were performed according to the procedures outlined in the drought phenotyping protocols by CIMMYT (Zaman-Allah et al., 2016; Zaidi, 2019).
Phenotypic data analysis
The restricted maximum likelihood method was utilized to conduct analysis of variance for each trial as well as a combined analysis across environment years using the META-R statistical package (Alvarado et al., 2020). The following linear mixed model was used:
where is the observed trait of interest; is the overall mean; is the effect of environment; is the effect of replication within environment; is the effect of block within environment; is the effect of replication within environment; is the effect of block within replication in environment; is the effect of the genotype; is the genotype by environment interaction and is effect of the residual error. Residuals are assumed as independent and identically distributed, ). Heritability in the broad sense (Hallauer et al., 2010) was computed as:
where , and is the genotype variance, genotype-by-environment interaction variance, and residual variance, respectively. The number of replications and environments is denoted by r and e, respectively. The correlation between traits under managed drought and optimum conditions was calculated using the “cor” function in R (R Core Team, 2023). A correlation heatmap and the distribution of phenotypic values of the traits were generated using the ggplot2 R package.
Line-by-tester mating design analysis
Line-by-tester analysis was conducted by excluding the checks from the analysis. The total variance of the testcrosses values was partitioned into the GCA variance due to the line and tester as well as the specific combining ability variance (SCA) due to line × tester (Trachsel et al., 2016; Liu et al., 2021). Data were analyzed using the AGD-R version 4 software (Rodríguez et al., 2015). Analyses were performed for within (Equation 1) and across locations (Equation 2) using the joint linear mixed model as:
where is the observed value, is the overall mean; is the effect of replication; is the effect of incomplete block within replication; is the GCA effect of the line; is the GCA effect of the tester; is the SCA effect of the line by tester, and is the residual effect assumed to be independent and identically distributed as ).
where is the response value, is the overall mean; is the effect of environment; is the effect of replication within environment; is the effect of incomplete block within replication in environment; is the GCA effect of the line; is the GCA effect of the tester; is the SCA effect of the line by tester; is the GCA effect of line interacting with environment; is the GCA effect of tester interacting with environment; is the SCA effect of line by tester interacting with environment and is the residual effect assumed to be independent and identically distributed, ).
The effects of environment and replication within the environment were considered fixed, while line, tester, line × tester, and their corresponding interaction with environment and block within environment within replication were considered random. The restricted maximum likelihood procedure was used to estimate all variance components (Rodríguez et al., 2015). Tests of the significance of the variance component estimates and model comparison were performed using the likelihood ratio test (Stram and Lee, 1994; Giampaoli and Singer, 2009).
Estimation of GCA and SCA effects
The estimates of the GCA effects for line, tester, and SCA effects of the line-by-tester interaction were estimated according to the procedure outlined by Singh and Chaudhary (1985). To test the significance of GCA and SCA at the 5% level of significance, the values of GCA for each line and tester and the SCA values of each hybrid were divided by their corresponding standard error, to compute their respective t-values. The calculated t-values were then compared with the tabular t-values at their corresponding error degree of freedom (Singh and Chaudhary, 1985). To assess the relative significance of GCA and SCA effects, Baker’s ratio was estimated using the variance of GCA of lines and testers and SCA based on the following formula:
where is the GCA variance of lines or tester and is the SCA line × tester variance. The closer the ratio to unity, the greater the predictability of a hybrid performance based on GCA effects alone (Baker, 1978).
The proportional contribution of total genetic variance due to lines, tester, and the interaction between lines and tester was computed as suggested by Singh and Chaudhary (1985). Pearson’s correlation of testcross hybrid performance (TCP) with the sum of GCA effects of both the parents r(GCA, TCP) using a leave-one-hybrid-out cross-validation was explained in detail by Schrag et al. (2009). All analyses were performed using the ASReml-R software version 3.0 (Butler et al., 2009).
Results
Analysis of variance under drought and optimum conditions
Individual location analyses for GY under managed drought and optimum conditions revealed significant (p < 0.05) genotypic variance and the heritability estimates ranged from 0.17 to 0.73 (Supplementary Table S2). Results of combined analysis across locations under optimum and managed drought conditions are shown in Table 2. The analysis showed highly significant (p < 0.01) genotypic variance among the testcrosses for all the traits indicating that genetic variation among testcross hybrids was abundant. The ratio of genotype to genotype-by-environment interaction (GEI) variance for GY was higher in the optimum condition compared to managed drought stress condition, suggesting that GEI interaction was greater under drought conditions. The GEI revealed a highly significant (p < 0.01) variance for GY, AD, SD, ASI, PH, and EH under optimum and drought conditions. The coefficients of variation for all the traits under optimum and drought conditions were below 25% except for ASI. The estimates of broad-sense heritability for all traits were relatively higher under optimum conditions (0.53 to 0.86) than under drought stress conditions (0.25 to 0.85). The low experimental coefficient of variation and high broad-sense heritability for most of the traits reflected good experimental accuracy. A wide range of phenotypic variation was observed among the testcrosses for all the traits under drought and optimum conditions (Figure 1). Drought stress considerably reduced GY, AD, and SD compared to optimum. Furthermore, drought stress prolonged the interval between AD and SD in the testcrosses. Plant height was reduced by drought stress compared to the optimum condition.
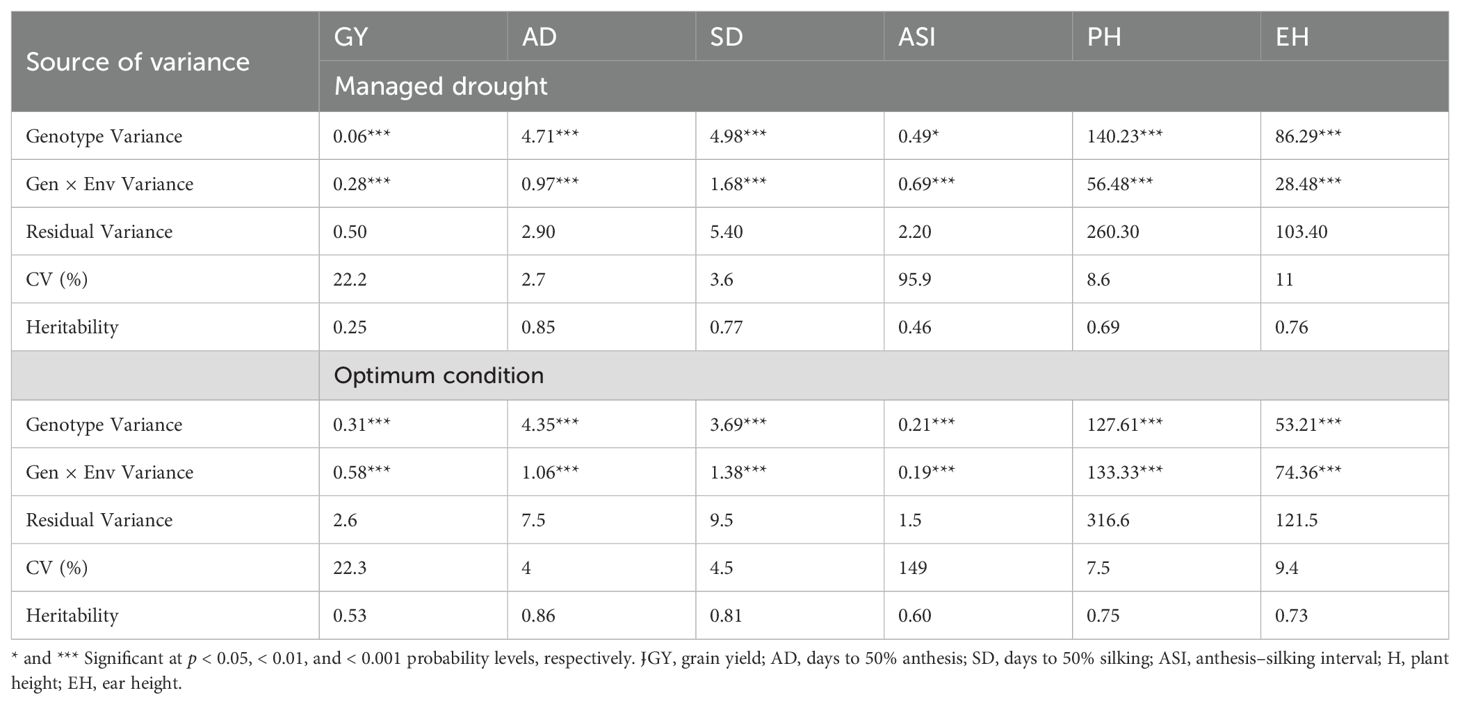
Table 2. Variance component, coefficient of variation, heritability of grain yield, and other traits under optimum and drought conditions.
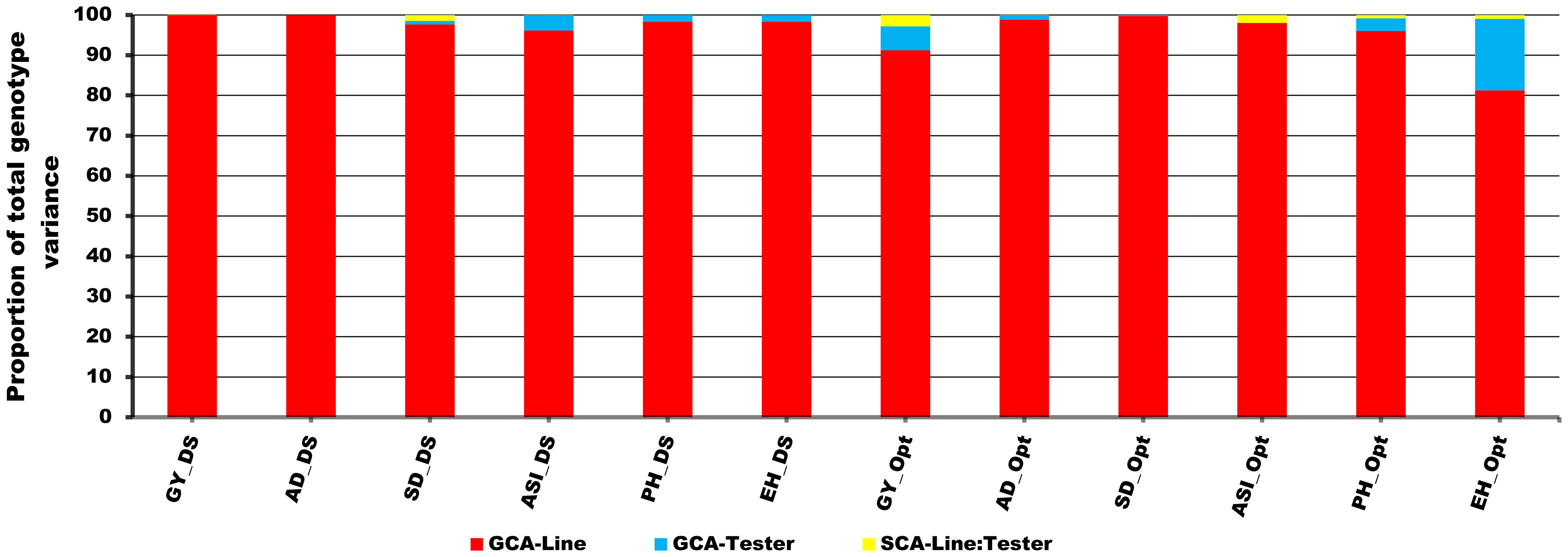
Figure 1. Frequency distribution of grain yield, anthesis date, silking date, anthesis–silking interval, plant height, and ear height recorded under managed drought and optimum conditions. Blue color denotes traits measured under drought stress and orange color denotes trait performance under optimum condition.
Performance of testcrosses
The mean performance of GY and other agronomic traits for the 20 selected best testcross hybrids and six commercial checks under managed drought and optimum conditions is presented in Tables 3 and 4, respectively. Under drought stress, GY averaged 2.15 t/ha, with a range of 0.41 to 4.89 t/ha. In contrast, under optimum conditions, the average GY was significantly higher at 7.39 t/ha, ranging from 3.95 to 9.77 t/ha. Under drought stress, it took 58 to 79 days (average, 67 days) to reach 50% anthesis (AD). Under optimum conditions, AD averaged 68 days, ranging from 62 to 74 days. For silking (SD), it took 60 to 79 days (average, 70 days) under drought stress and 60 to 72 days (average, 66 days) under optimum conditions. The ASI averaged 2.8 days (range, −2 to 10 days) under drought stress and 1 day (range, −1.4 to 3.1 days) under optimum conditions (Tables 3, 4). However, under optimum conditions (Table 3), PH varied from 172 to 265 cm with a mean of 226.4 cm under optimum conditions. Under managed drought conditions, it ranged from 153.2 to 263.1 cm with an average of 214.7 cm. Generally, plant and ear height were taller under optimum conditions than under drought stress conditions (Tables 3, 4).
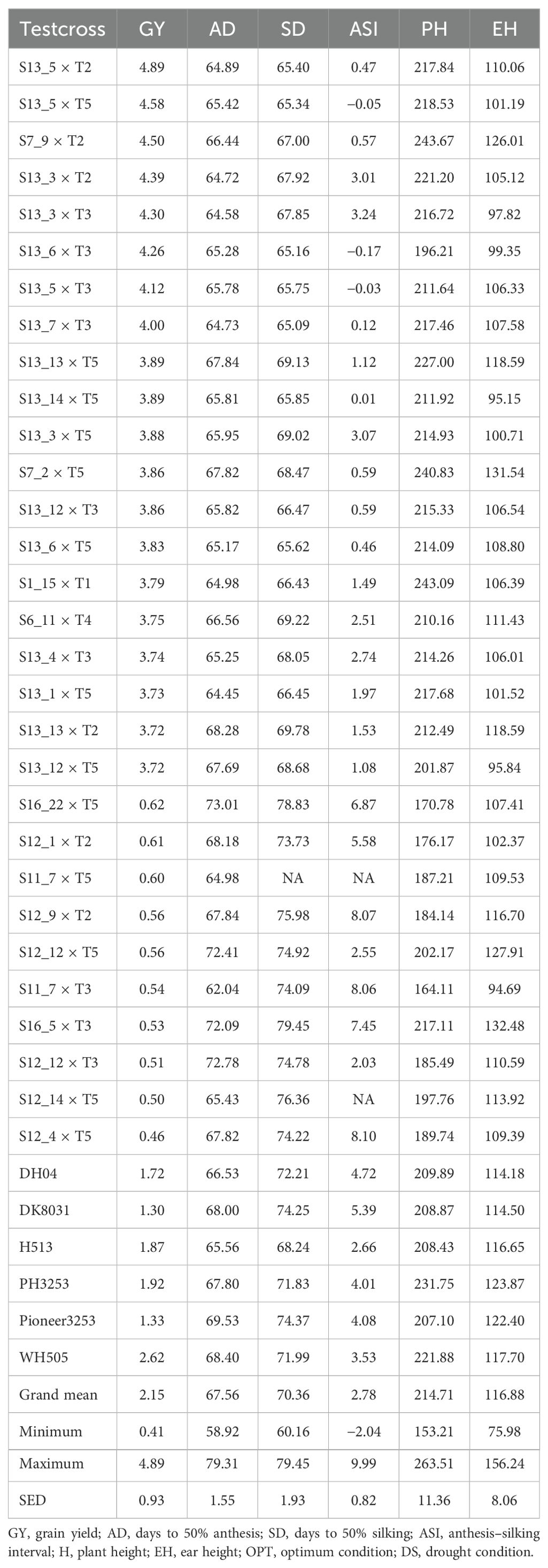
Table 3. Mean performance of grain yield and other agronomic traits in 30 testcross hybrids (20 best and 10 worst) plus six checks tested under drought.
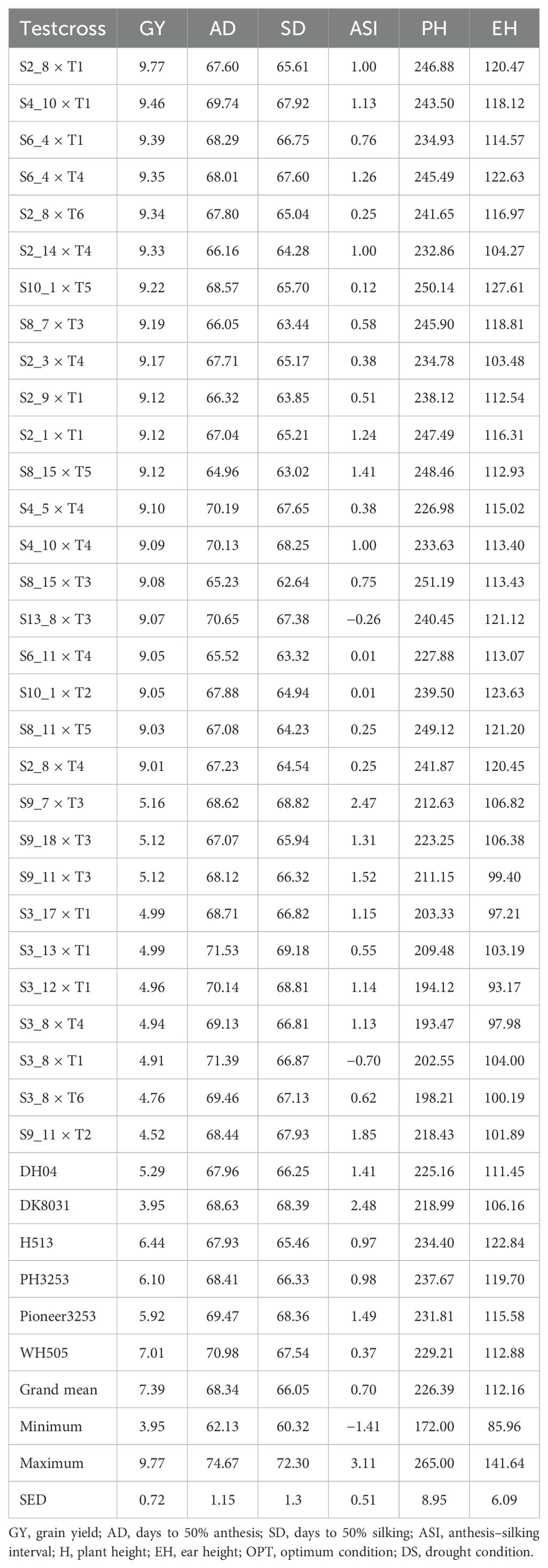
Table 4. Mean performance of grain yield and other agronomic traits in 30 testcross hybrids (20 best and 10 worst) plus six checks tested under optimum conditions.
Under managed drought conditions, testcrosses S13_5 × T2 (4.9 t/ha), S13_5 × T5 (4.6 t/ha), S7_9 × T2 (4.50 t/ha), S13_3 × T2 (4.4 t/ha), and S13_3 × T3 (4.3 t/ha) are among the top-performing hybrids, with GY outperforming the best commercial check (WH505; Table 3). The yield performance of the best testcross hybrid under managed drought conditions was almost double that of the best commercial check (Table 3). Under optimum conditions, the best testcross hybrid (S2_8 × T1) outyielded the best commercial hybrid by 2.7 tons (Table 4). Overall, drought stress reduced the GY by 20%. Under the optimum condition (Table 4), testcross S2_8 × T1 (9.8 t/ha), S4_10 × T1 (9.46 t/ha), S6_4 × T1 (9.39 t/ha), S6_4 × T4 (9.35 t/ha), S2_8 × T6 (9.34 t/ha), and S2_14 × T4 (9.3 t/ha) are the best-performing hybrids. All the 20 best hybrids outyielded the best commercial check (WH505).
Relationship between traits under drought and optimum conditions
Insights into the relationships among traits is crucial for breeders when selecting suitable traits to incorporate in selection criteria. Significant (p < 0.001) coefficients of phenotypic correlation were observed among most traits across drought and optimum conditions (Figures 2A–C). Significant (p < 0.001) and positive correlations were identified between GY and PH and EH. PH and EH implied that selection improvement of these traits under optimum conditions could lead to an increase in GY (Figure 2B). Furthermore, significant (p < 0.001) and negative correlations observed between GY and AD, SD and ASI, and ASI with PH, EH, and AD under optimum conditions implied that that these traits are reliable selection indices for yield improvement in optimum conditions. A significant (p < 0.001) and negative correlation was observed between GY and AD_DS, SD_DS, and ASI_DS under drought stress. This implied that selection of GY alone selection may not be effective.
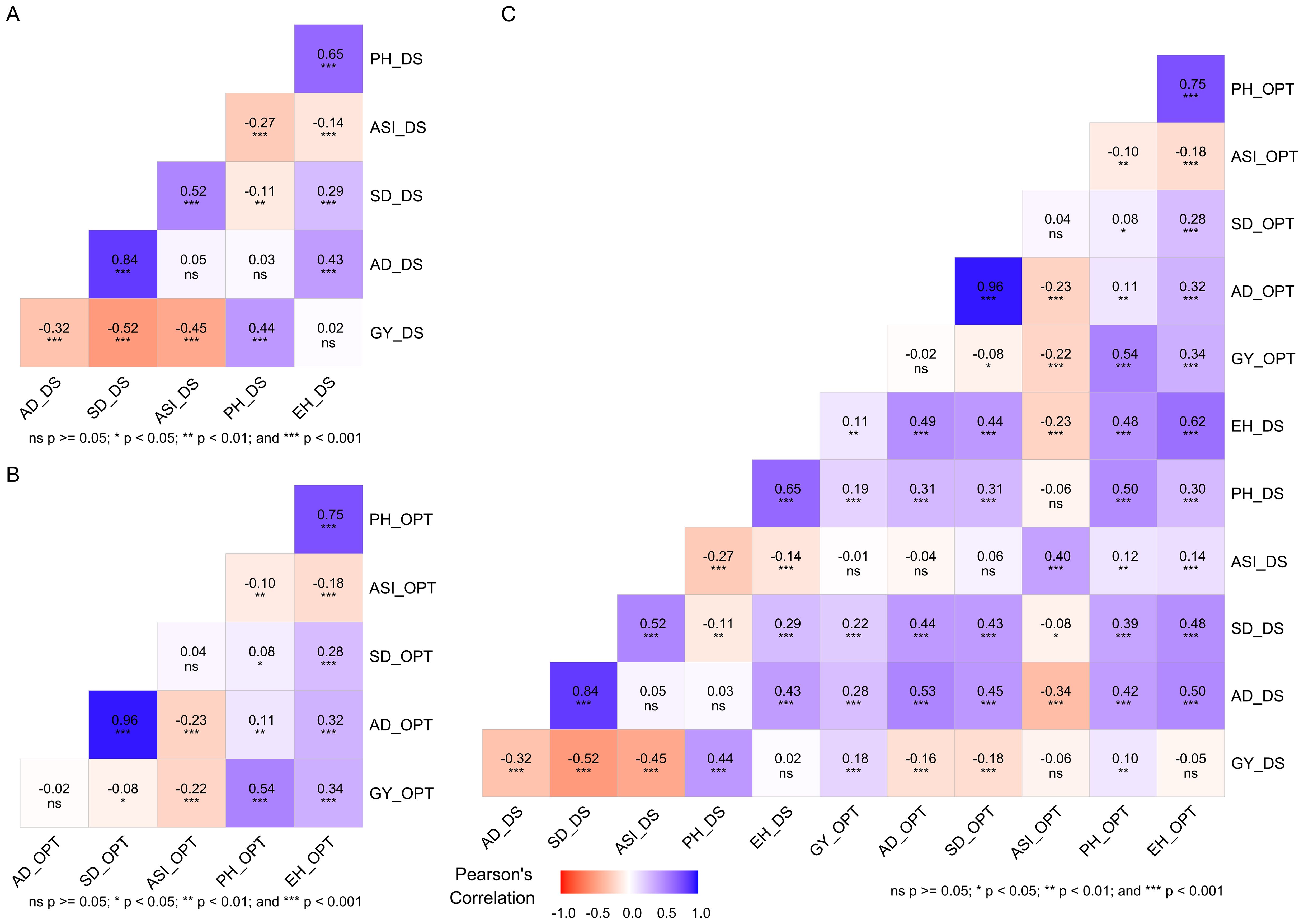
Figure 2. Pearson’s correlation between grain yield and other traits measured under drought stress (A), under optimum condition (B), and under both drought and optimum conditions (C). The correlation level is color-coded according to the color key indicated on the scale. Correlations with *, **, and *** were significant at 0.05, 0.01, and 0.001 levels, respectively. AD, days to 50% anthesis; ASI, anthesis to silking interval; SD, silking date; GY, grain yield; PH, plant height; EH, ear height; DS, drought stress; OPT, optimum.
Analyses of GCA and SCA variance
The genotypes and GEI variance components were significant at the 0.05% probability level for all traits under OPT and DS conditions (Table 5). The GCA variance for lines and testers were highly significant (p < 0.001) for majority of the traits under both DS and OPT conditions, indicating high genetic variation among these genotypes. In contrast, GCA variance for testers was not significant for GY under DS conditions. The SCA variance for line × tester interactions was not significant for all the traits under DS and OPT conditions (Table 5). Variances for line × environment and tester × environment interactions were significant (p < 0.001) for most of the traits under DS and OPT conditions. However, tester-by-environment interaction variances were not significant for GY, PH, and EH under DS conditions. SCA variances were not significant for most of the traits under DS and OPT conditions (Table 5). Baker’s ratio (Tables 5, 6) was closer to or equal to 1 for all the traits under DS and OPT conditions.
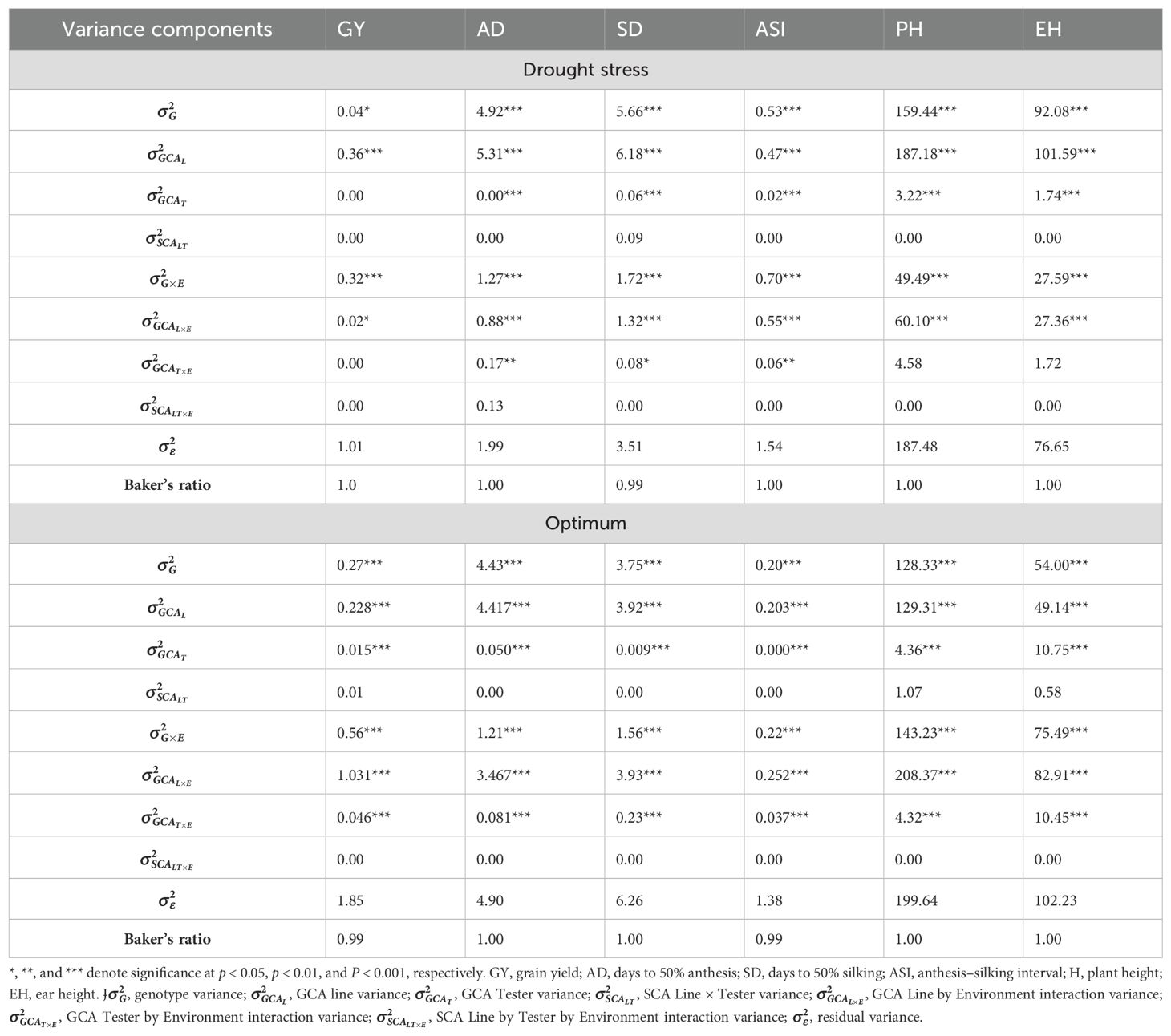
Table 5. Partition of variance components for line by tester and Baker’s ratio of grain yield and other traits evaluated under drought and optimum conditions.
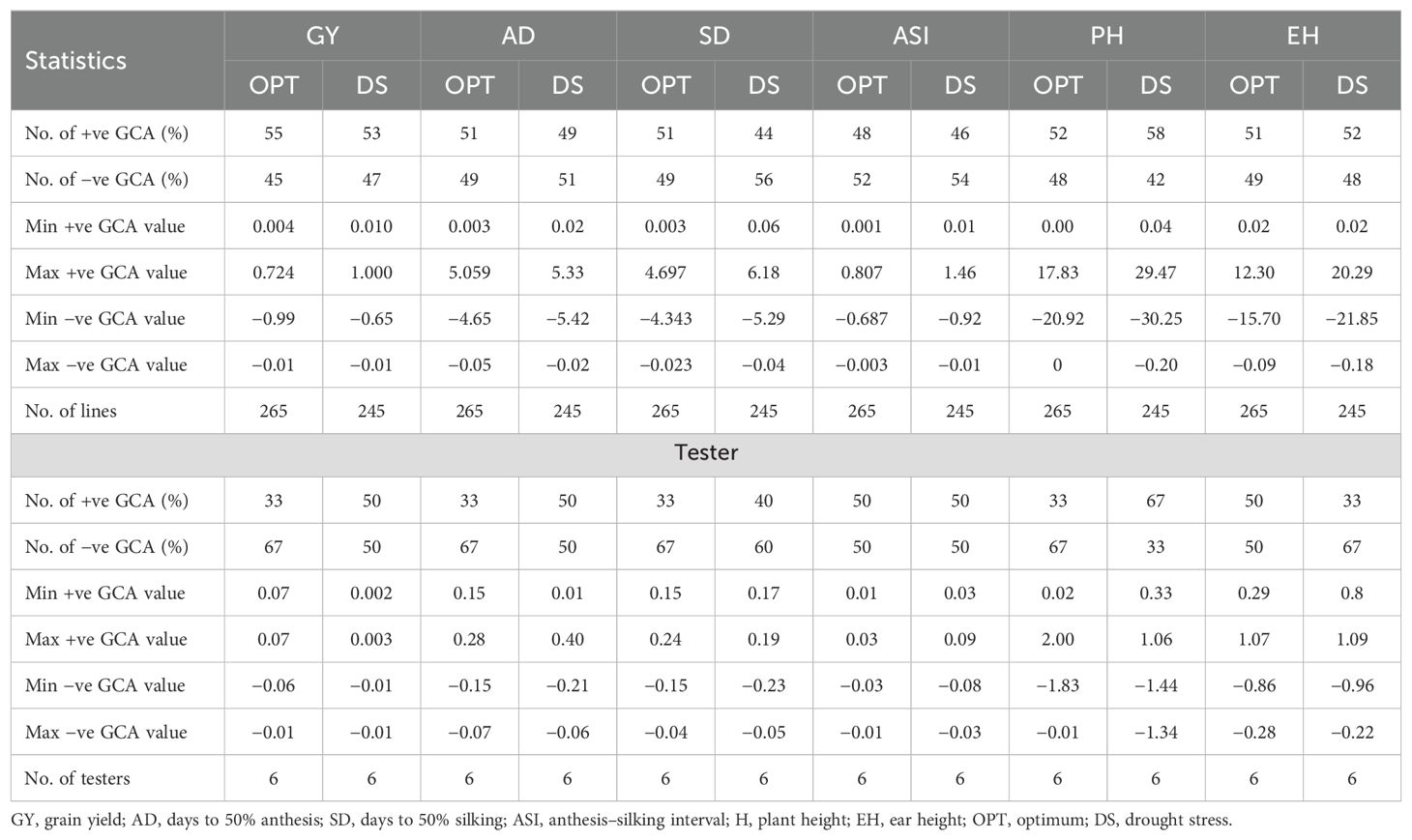
Table 6. Summary statistics of GCA estimates of lines and testers for grain yield and other agronomic traits evaluated in multiple locations under drought and optimum conditions.
The proportional contribution due to GCA variance of lines to the total genotypic variance varied from 81% to 100% under DS and OPT conditions (Figure 3). In contrast, the contribution of GCA variance of tester to total genotypic variance ranged from 0% to 4% under DS and from 0% to 18% under OPT conditions (Figure 3). Similarly, the SCA variance varied from 0% to 1% under DS compared to 0% to 3% under OPT conditions. Overall, proportional contribution due to GCA variance of lines was by far larger than the contribution due to tester and the interaction between line and tester variance.
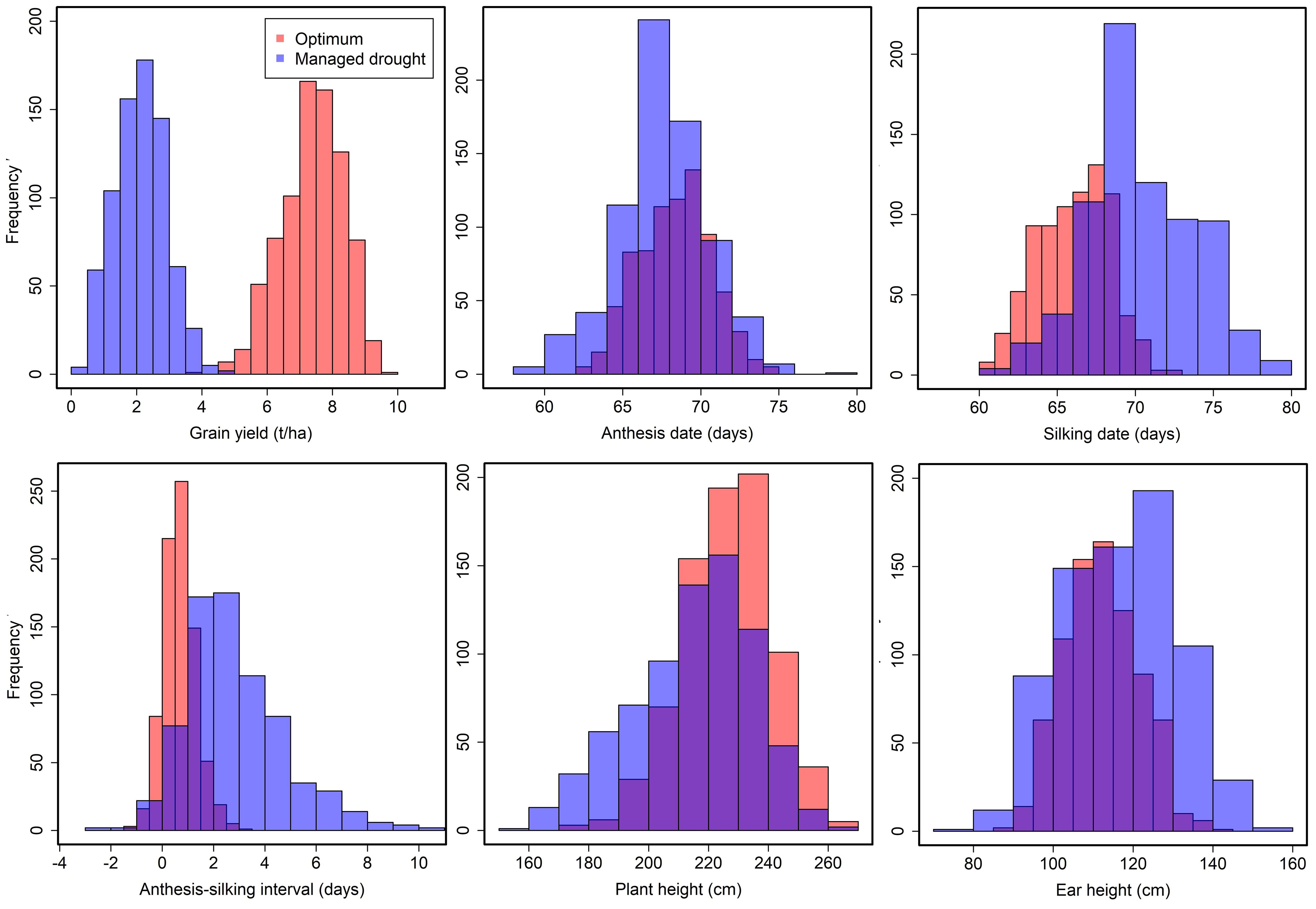
Figure 3. Proportional contribution of GCA-Line, GCA-Tester, and SCA-Line by Tester interaction to total genotype variance of testcrosses for GY and other secondary traits under drought (DS), optimum (Opt), and across all environments (ACR) conditions. The lower bar denoted by red color indicates the GCA-Line; the middle bar denoted by sea blue represents the GCA-Tester, and the upper bar denoted by yellow indicates the SCA-Line by Tester interaction.
Estimates of GCA and SCA effects under DS and OPT
The summary statistics of GCA effects of lines and testers for GY and other traits under DS and OPT conditions is shown in Figure 4 and in Tables 6 and 7. The values of GCA estimates varied among the lines and testers for all the traits. Under DS conditions (Table 5), the GCA effect of GY varied from −0.65 to 1.00 with 53% and 47% of lines found to be positive and negative effects, respectively. Lines S13_5, S13_6, S13_12, S6_11, S7_2, S2_8, S8_7, S2_7, S8_8, S2_15, and S8_19 were positive with high GCA effects for GY (Figure 3). Tester T5 had the highest GCA effect, whereas tester T4 had the lowest GCA effects for GY. The GCA effects for other traits are also shown in Tables 6 and 7.
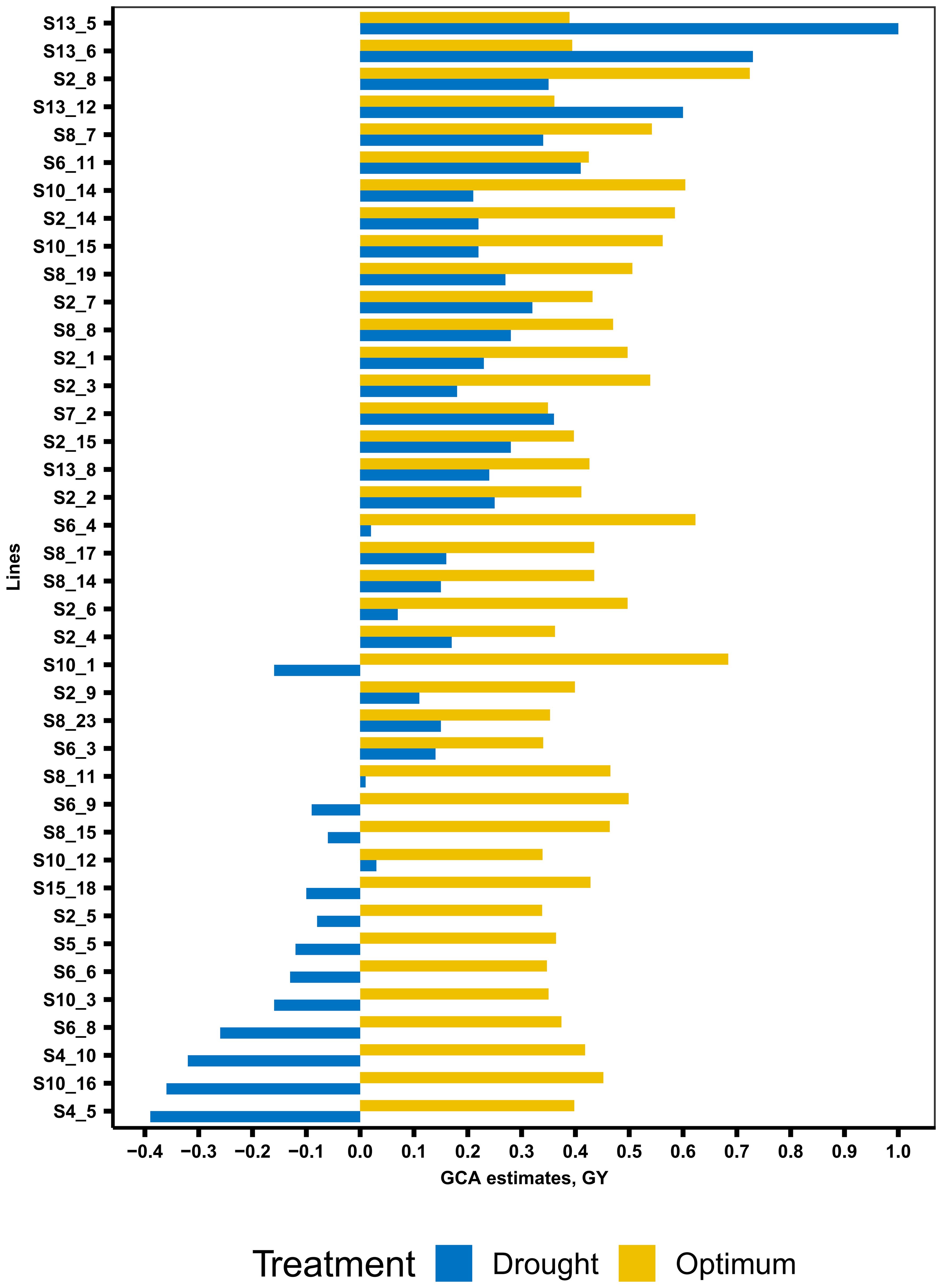
Figure 4. General combining ability estimates of 40 selected best lines for grain yield across managed drought and optimum conditions.
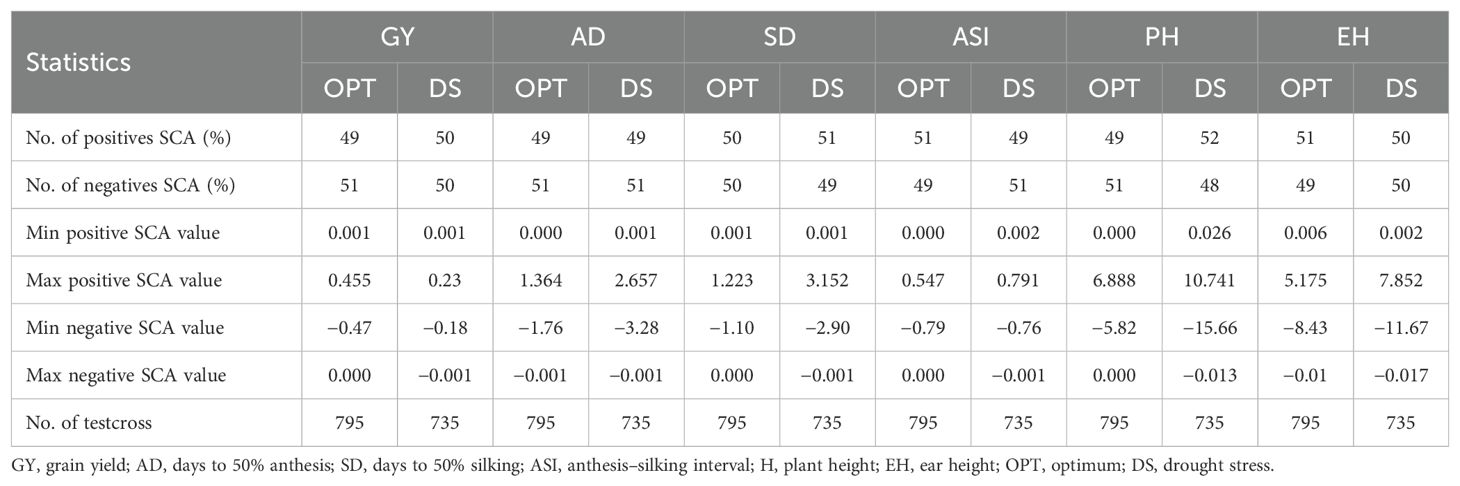
Table 7. Summary statistics of SCA values for grain yield and other agronomic traits evaluated in multiple locations under drought and optimum conditions.
The GCA effects of ASI ranged from −0.92 (S13_5) to 1.46 (S10_8), while tester GCA effects varied from −0.08 to 0.09 (Table 7). More than half of the lines and testers showed a negative GCA effect for ASI. Under OPT conditions (Figure 3; Table 6), GCA effects varied considerably among the lines and testers. The GCA effects for GY ranged from −0.01 to 0.72. Out of the total lines, 55% of the lines showed positive GCA effects for GY, whereas 45% of the lines displayed negative GCA effects for GY. Line S2_8 recorded the highest GCA effects for GY followed by lines S10_1, S6_4, S10_14, S2_14, S10_15, S8_7, S2_3, S8_19, and S6_9. Testers T1 and T5 showed a positive and high GCA effect for GY. Across DS and OPT management conditions (Figure 4; Tables 6, 8), lines S2_8, S10_1, S6_4, S10_14, S2_14, S10_15, S8_7, S2_3, S8_15, and S13_5 consistently exhibited a high and positive GCA effect for GY and a high and negative GCA effect for AD, SD, ASI, PH, and EH.
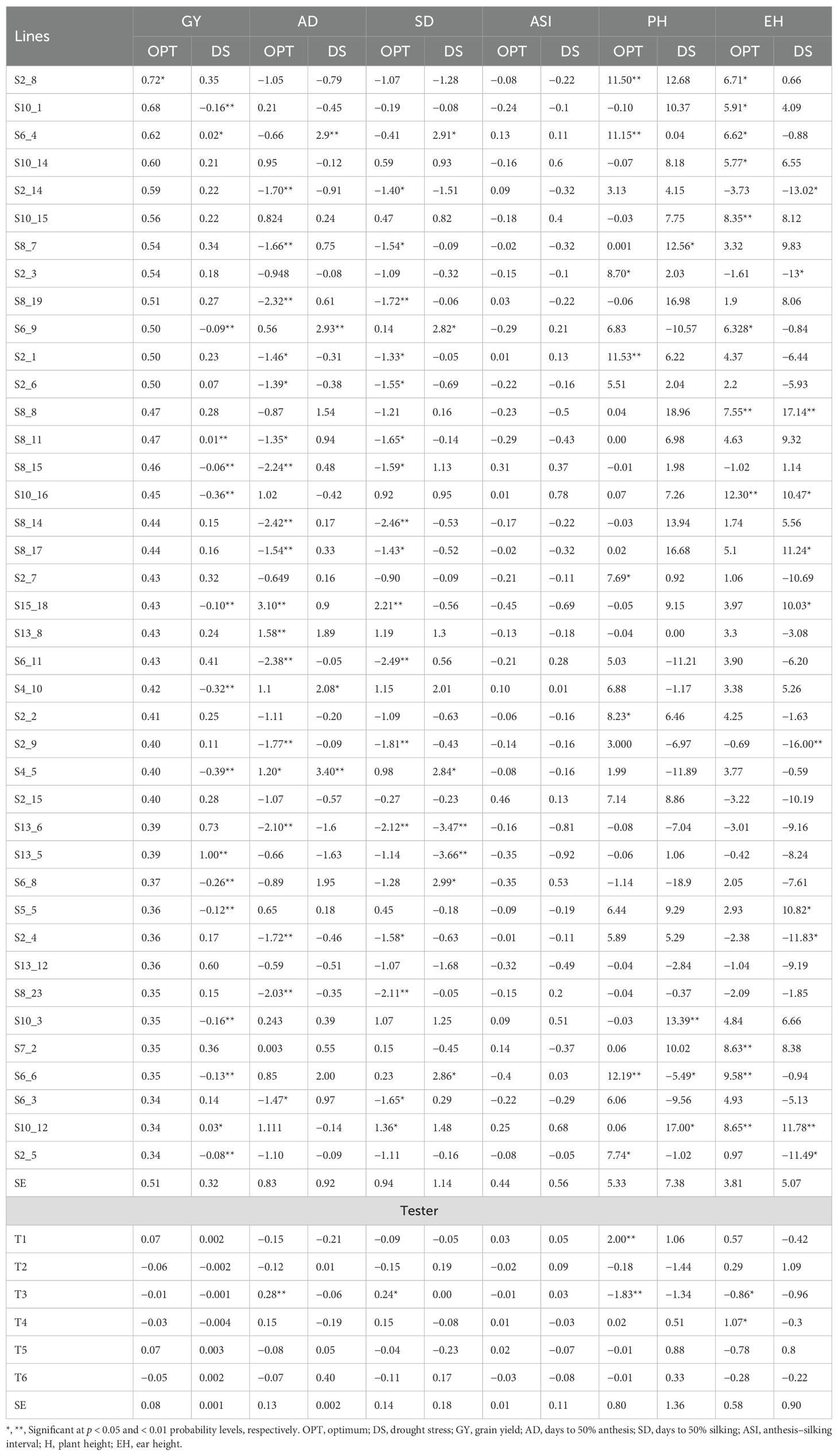
Table 8. GCA estimates of selected best 40 lines and six testers for grain yield and other agronomic traits evaluated in multiple locations under drought and optimum conditions.
The summary statistics of SCA effects on selected testcross hybrids for all the traits evaluated under DS and OPT conditions is presented in Tables 7 and 9. Across all the management conditions, some of the selected testcross hybrids showed consistently high and positive SCA effects for GY and a high negative SCA effect for other traits. This indicates that these testcross hybrids are good specific combiners for GY and possess desirable plant architectural characteristics including shorter number of days to anthesis and silking, reduced period between anthesis and silking, shorter plant stature, and lower ear placement. Prediction correlation between the observed testcross hybrid performance and the GCA-predicted hybrid performance was high for GY with r = 0.95 under OPT and r = 0.90 under DS conditions (Figure 5). Prediction correlations were also high for AD and PH under both OPT and managed DS conditions.
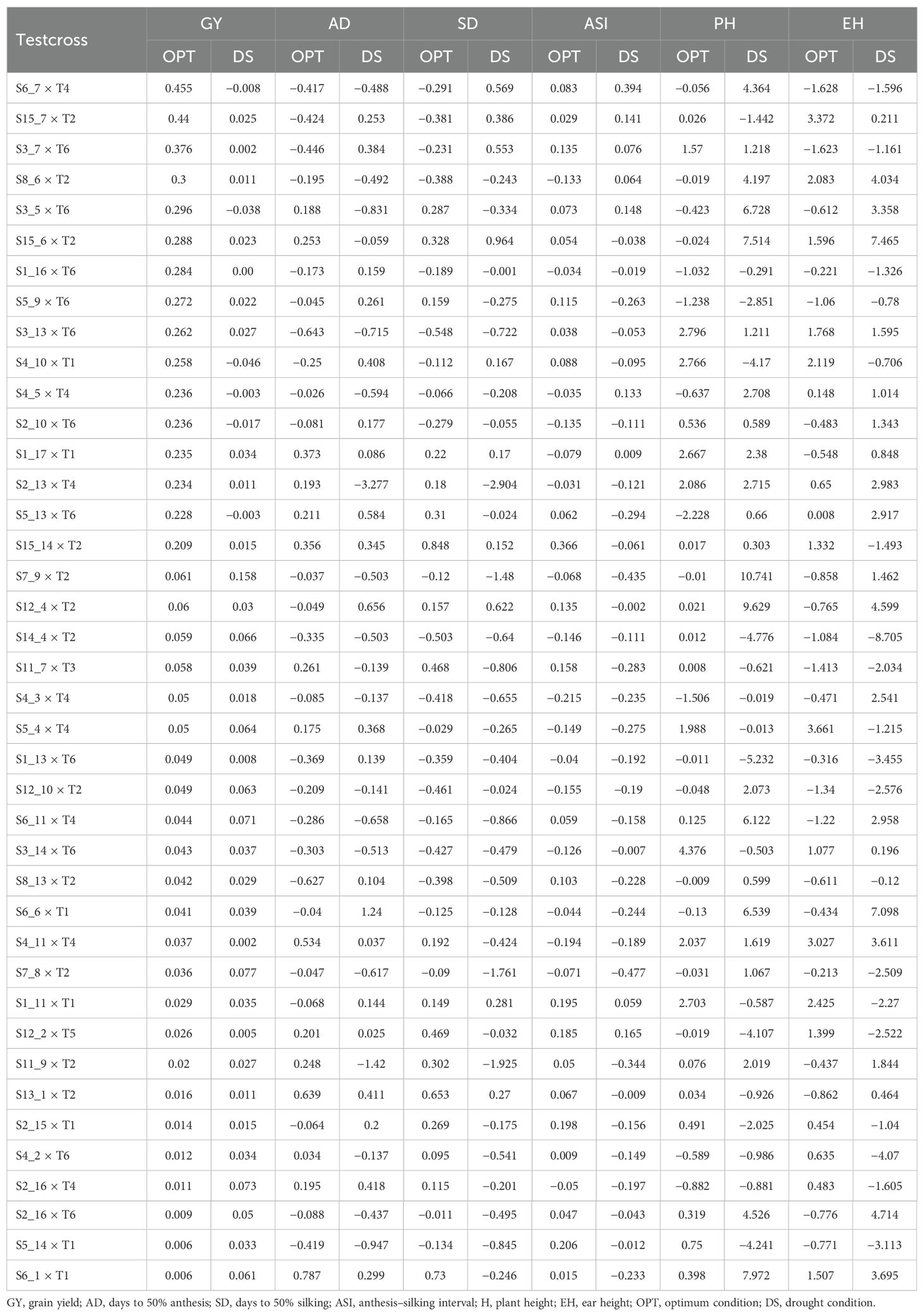
Table 9. SCA estimates of selected best 40 lines-by-tester combinations for grain yield and other agronomic traits evaluated in multiple locations under drought and optimum conditions.
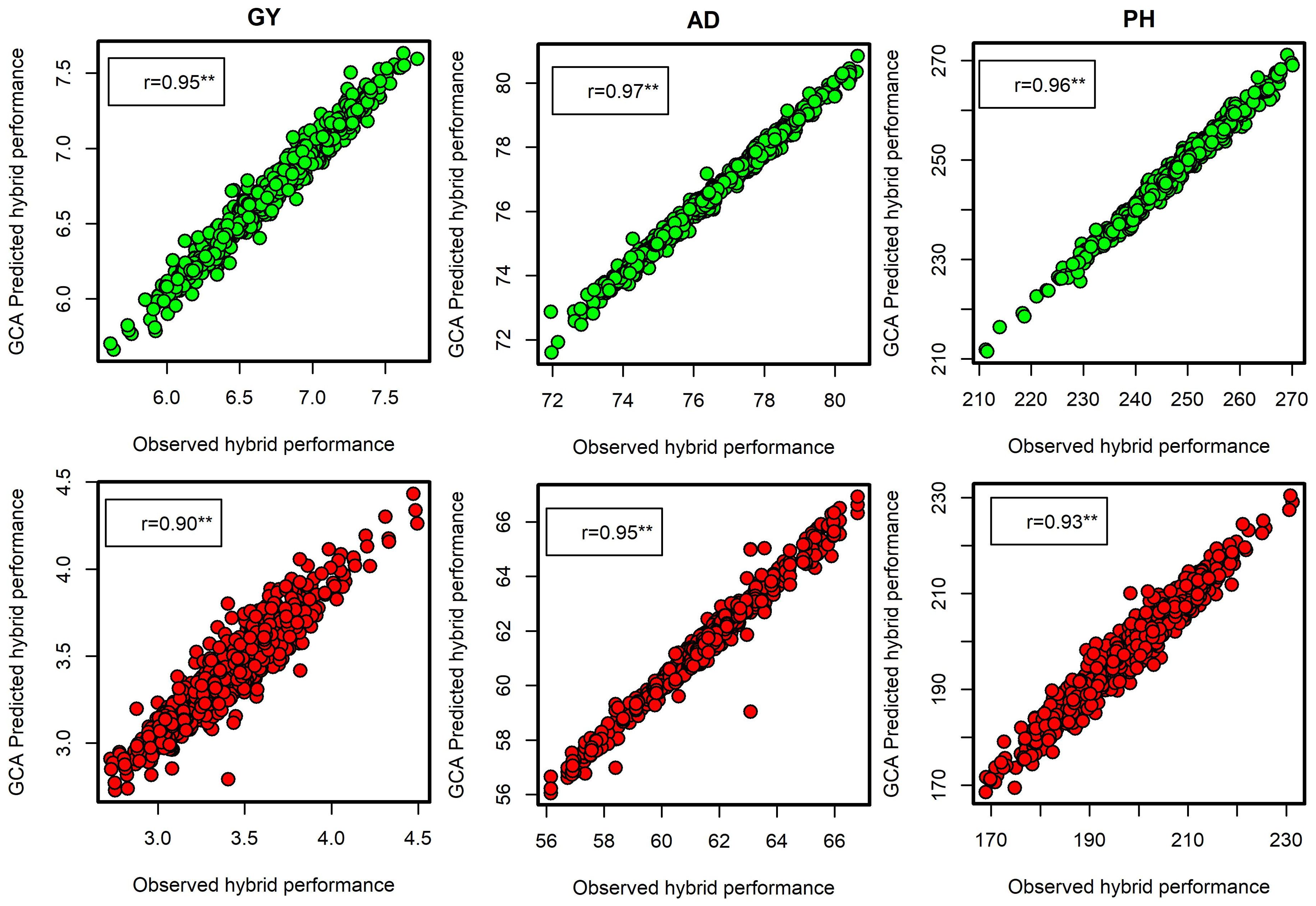
Figure 5. Leave-one-hybrid-out cross-validated r values between general combining ability (GCA)-based predicted testcross hybrid performance and observed hybrid performance for grain yield, anthesis date, and plant height evaluated under optimum (green dots) and managed drought conditions (red dots).
Discussion
Drought stress is one of the main factors for yield losses in maize production. Yield improvement under DS management has become a “must have trait” in tropical maize breeding programs in SSA. In this study, the significant genotype variability among the testcross hybrids for GY and other agronomic traits under DS and optimum conditions indicated that trait improvements could be achieved through selection. Therefore, these parental lines could further be exploited for yield improvement under OPT and DS conditions (Ertiro et al., 2017; Santos et al., 2019).
The present study showed that environment and GEI variance components were significant for GY and other agronomic traits, suggesting that hybrids responded differentially across both DS and OPT environments. These results agree with those previous findings by Sserumaga et al. (2016, 2021) and Sorsa et al. (2023). The significant GEI effects on the expression of a trait can obscure the correlation between genotype and phenotype values, leading to reduced selection response (Yan, 2014). This implies that the ability to identify high-yielding and consistently performing hybrids across DS and OPT growing environments will be hampered by the high GEI variance. Therefore, rigorous testing of hybrids across multiple locations and over several years is essential before hybrids can be recommended for cultivar release. This result is in agreement with the findings of several studies (Sserumaga et al., 2016; Annor et al., 2019; Sorsa et al., 2023; Ndlovu et al., 2024, 2021).
High genotypic variance and moderate to high broad-sense heritability estimates were observed for AD, SD, ASI, EH, and PH under drought and optimum. However, heritability for GY was low under drought. The low heritability of GY observed under the drought experiment suggests that secondary traits exhibiting high heritability may enhance the effectiveness of selection responses (Lu et al., 2011; Rezende et al., 2020). These results are in agreement with previous studies that reported lower genotypic variances and heritabilities for GY under stressed conditions (Badu-Apraku and Fakorede, 2017; Banziger et al., 1997; Beyene et al., 2013).
The increased mean value of ASI observed under DS, the significant yield reduction and increased AD and SD, and decreased PH relative to the OPT condition implied that the intensity of DS was sufficient enough to discriminate among testcross hybrids for drought tolerance (Sang et al., 2022). Previous studies have demonstrated that a significant decrease in GY, ranging from 20% to 30%, is a clear indication of severe DS (Edmeades et al., 1999, 2015; Sang et al., 2022). The observed decrease in GY in the current study is in agreement with previous studies reported by Trachsel et al. (2016); Ertiro et al. (2017); Annor et al. (2020), and Matongera et al. (2023). A study conducted by Ertiro et al. (2017) on testcross performance for their combining ability under DS and low nitrogen reported a 50% yield reduction and an increase in ASI by 149% with a 1% decrease in PH. The reduction of GY under DS can be attributed to increased ASI leading to high yield reduction among the testcross hybrids. DS inhibits silk development, thus delaying the silking process, leading to increased period between anthesis and silking resulting in higher rates of ear and kernel abortion, which can cause ear barrenness, leading to GY reduction (Bänziger et al., 2000).
Direct selection of testcross hybrids for GY alone under DS is considered inefficient due to the observed low heritability and low genetic variance (Ertiro et al., 2017; Kim et al., 2017). On the other hand, ASI can be used as a secondary trait for indirect selection of genotypes with improved drought tolerance and high GY in maize breeding (Bänziger et al., 2000; Betrán et al., 2003a; Cooper et al., 2014). The mean performance of GY and other traits in the 30 selected testcross hybrids was generally higher than the mean performance of the best commercial checks across DS and OPT conditions. This suggested that the testcross possesses high mean performance for drought tolerance. Similar observations were reported in previous studies (Sserumaga et al., 2016; Ertiro et al., 2017; Matongera et al., 2023).
Understanding the trait correlations across DS and OPT conditions can provide valuable insights for making informed decisions in breeding for drought tolerance (Badu-Apraku et al., 2012; Ziyomo and Bernardo, 2013). It is possible to improve positively correlated traits by focusing on the improvement of one easily measurable trait. According to Bänziger et al. (2000), simultaneous selection of drought tolerance and GY under DS can be efficiently implemented by prioritizing secondary traits that are both easily measurable and highly correlated with GY under managed drought conditions. The presence of significant negative association between GY and flowering traits (AD, SD, and ASI) under DS and OPT conditions suggested that these traits have indirect effects on GY across the management conditions. These results are in agreement with the findings of previous studies (Badu-Apraku et al., 2012; Ertiro et al., 2017, 2022; Talabi et al., 2017; Oyekunle and Badu-Apraku, 2018; Matongera et al., 2023).
The prevalence of GCA (line and tester) variance over SCA for GY and other agronomic traits within and across management conditions implied that additive gene action for the inheritance of these traits was higher than nonadditive gene effects. These findings corroborate previous results reported by Betrán et al. (2003b), Makumbi et al. (2011); Amegbor et al. (2017); Ertiro et al. (2017), and Oluwaseun et al. (2022). The current study results also contradict those of de Souza et al. (2009) and Annor et al. (2019) who reported that nonadditive effects predominantly governed GY and other secondary traits under DS conditions. The difference in the outcomes of the current study compared to previous studies can likely be attributed to the differences in the germplasms utilized.
In the present study, the Baker’s variance ratio showed that there is a high probability of predicting testcross hybrid performance based on GCA variance (line or tester) alone under DS and OPT conditions. The ratios closer to or equal to one implied that the traits are governed predominantly by additive gene action and that there is a high likelihood of predicting testcross hybrid performance based on only GCA effects. This is well supported by the observed high correlations between testcross hybrid performance and GCA-predicted hybrid performance for GY, AD, and PH under both OPT and DS conditions (Figure 5). This further suggested that additive effects had a more significant impact on all the traits than non-additive effects. The results of this study corroborates the findings of Amegbor et al. (2017) and Oluwaseun et al. (2022).
The estimates of combining ability effects hold great significance as they reveal the inherent ability of a set of genotypes to effectively pass on desirable genes to their offspring. This valuable information aids in making informed decisions regarding genetic selection and enhancement (Hallauer et al., 2010). According to several researchers (Makumbi et al., 2011; Ertiro et al., 2017; Annor et al., 2019), inbred lines possessing favorable GCA effects for GY and secondary traits are highly valuable in breeding programs. These lines can be utilized (i) as parental lines and incorporated into recurrent selection programs to enhance the overall genetic potential of future generation, (ii) as excellent parent candidates for developing synthetics if necessary, and (iii) for recycling to maintain their superior genetic attributes within breeding populations. Generally, inbred lines with a high GCA can be utilized as excellent broad-based testers. In this regard, inbred lines S2_8, S10_1, S6_4, S10_14, S2_14, S10_15, S8_7, S2_3, S8_15, and S13_5 consistently exhibited high and desirable GCA effects for GY and other agronomic traits (Table 8). Similarly, tester 5 exhibited high GCA effects for GY and other secondary traits. Lines with negative GCA effects for AD, SD, and ASI are suitable for exploiting desirable genes for developing early maturity cultivars with short period between male and female flowering, a key measure of drought tolerance. High negative GCA effects for PH and EH suggest that these lines possess shorter plant and ear height genes, which could be selected as parental lines for exploiting lodging resistance. The high and desirable GCA effects shown by inbred lines suggested that they possess necessary sources of genes/alleles for high GY, earliness, shorter ASI, reduced PH, and lower ear placement that could potentially be transmitted to offspring through breeding.
The following testcross combinations consistently showed a high positive SCA effect for GY and a high negative SCA effect for other traits across both DS and OPT conditions: S7_9 × T2, S7_8 × T2, S2_16 × T4, S6_11 × T4, S12_10 × T2, S6_1 × T1, S3_13 × T6, S15_7 × T2, S15_6 × T2, S5_9 × T6, S4_3 × T4, S8_6 × T2, S1_13 × T6, and S3_7 × T6. These findings suggested that the testcrosses are excellent candidates for improving GY with desirable agronomic traits under both drought and optimum conditions. The observed high correlation between testcross hybrid performance and GCA-predicted hybrid performance for GY, AD, and PH under both optimum and DS conditions indicates the predominance of GCA effects (Figure 5). Furthermore, this result gives an opportunity for breeders to test a greater number of lines without increasing the number of plots and resources even for both optimum and DS conditions.
Conclusion
A line × tester experiment of 765 testcross hybrids and six commercial checks was evaluated under managed OPT and DS conditions. In the present study, we observed significant genetic variations among the testcross hybrids for GY and other agronomic traits under both OPT and DS conditions, which supports the possibility of genetic improvement through selection. Combining ability estimates and Baker’s ratio indicate a preponderance of additive gene action over non-additive gene action. Therefore, significant progress can be achieved through recurrent selection. Ten inbred lines (S2_8, S10_1, S6_4, S10_14, S2_14, S10_15, S8_7, S2_3, S8_15, and S13_5) consistently showed a high and desirable GCA effect for GY and other traits across DS and OPT conditions. These lines could be used as donors for the improvement of drought tolerance or for introgression of their favorable alleles into other elite lines through recurrent selection. Fourteen testcross hybrids (S7_9 × T2, S7_8 × T2, S2_16 × T4, S6_11 × T4, S12_10 × T2, S6_1 × T1, S3_13 × T6, S15_7 × T2, S15_6 × T2, S5_9 × T6, S4_3 × T4, S8_6 × T2, S1_13 × T6, and S3_7 × T6) were identified with high yield potential and with other desirable agronomic traits under DS and OPT conditions. These testcross hybrids should be further evaluated extensively under on-farm conditions, which mimic the real smallholder farmers’ conditions and confirm their consistent performance before releasing for commercialization.
Data availability statement
The datasets presented in this study can be found in online repositories. The names of the repository/repositories and accession number(s) can be found in the article/Supplementary Material.
Author contributions
KM: Data curation, Formal analysis, Methodology, Software, Validation, Visualization, Writing – original draft, Writing – review & editing. YB: Conceptualization, Methodology, Resources, Validation, Writing – review & editing. VC: Data curation, Validation, Visualization, Writing – review & editing. PT: Project administration, Resources, Supervision, Writing – review & editing. ED: Investigation, Supervision, Writing – review & editing. BI: Data curation, Validation, Writing – review & editing. IA: Data curation, Formal analysis, Software, Writing – review & editing. AC: Data curation, Formal analysis, Software, Writing – review & editing. BP: Conceptualization, Funding acquisition, Project administration, Resources, Writing – review & editing. MG: Conceptualization, Investigation, Methodology, Supervision, Visualization, Writing – review & editing.
Funding
The author(s) declare financial support was received for the research, authorship, and/or publication of this article. The research was supported by the Bill and Melinda Gates Foundation (BMGF), Foundation for Food and Agriculture Research (FFAR), and the United States Agency for International Development (USAID) through AG2MW (Accelerating Genetic Gains in Maize and Wheat for Improved Livelihoods, BMGF Investment ID INV-003439), the Stress Tolerant Maize for Africa (STMA, BMGF Grant # OPP1134248) project, and the CGIAR Research Program on Maize (MAIZE). Our sincere thanks to CIMMYT technicians in Kenya. This research was part of the PhD thesis of the first author, funded by the WACCI ACE Impact project at the West Africa Centre for Crop Improvement (WACCI), University of Ghana, Legon, Ghana.
Acknowledgments
The authors are grateful to the International Maize and Wheat Improvement Center (CIMMYT) and partner scientists and technicians who generated the germplasm and designed and conducted the experiments that we used to explore our objectives.
Conflict of interest
The authors declare that the research was conducted in the absence of any commercial or financial relationships that could be construed as a potential conflict of interest.
Publisher’s note
All claims expressed in this article are solely those of the authors and do not necessarily represent those of their affiliated organizations, or those of the publisher, the editors and the reviewers. Any product that may be evaluated in this article, or claim that may be made by its manufacturer, is not guaranteed or endorsed by the publisher.
Supplementary material
The Supplementary Material for this article can be found online at: https://www.frontiersin.org/articles/10.3389/fpls.2024.1471041/full#supplementary-material
References
Acquaah, G. (2012). Principles of Plant Genetics and Breeding, 2nd ed. (Chichester: Wiley-Blackwell, John Wiley & Sons). doi: 10.1002/9781118313718
Alvarado, G., Rodríguez, F. M., Pacheco, A., Burgueño, J., Crossa, J., Vargas, M., et al. (2020). META-R: A software to analyze data from multi-environment plant breeding trials. Crop J. 8, 745–756. doi: 10.1016/j.cj.2020.03.010
Amegbor, I. K., Badu-Apraku, B., Annor, B. (2017). Combining ability and heterotic patterns of extra-early maturing white maize inbreds with genes from Zea diploperennis under multiple environments. Euphytica 213, 1–16. doi: 10.1007/s10681-016-1823-y
Annor, B., Badu-apraku, B., Nyadanu, D., Akromah, R., Fakorede, M. A. B. (2019). Testcross performance and combining ability of early maturing maize inbreds under multiple-stress environments. Sci. Rep. 9, 13809. doi: 10.1038/s41598-019-50345-3
Annor, B., Badu-Apraku, B., Nyadanu, D., Akromah, R., Fakorede, M. A. B. (2020). Identifying heterotic groups and testers for hybrid development in early maturing yellow maize (Zea mays) for sub-Saharan Africa. Plant Breed. 139, 708–716. doi: 10.1111/PBR.12822
Badu-Apraku, B., Akinwale, R. O., Franco, J., Oyekunle, M. (2012). Assessment of reliability of secondary traits in selecting for improved grain yield in drought and low-nitrogen environments. Crop Sci. 52, 2050–2062. doi: 10.2135/cropsci2011.12.0629
Badu-Apraku, B., Fakorede, M. A. B. (2017). “Breeding Maize for Drought Tolerance,” in Advances in Genetic Enhancement of Early and Extra-Early Maize for Sub-Saharan Africa. eds. Badu-Apraku, B., Fakorede, M. A. B. (Cham: Springer, Cham, Switzerland), 287–309. doi: 10.1007/978-3-319-64852-1_11
Badu-Apraku, B., Fakorede, M. A. B., Gedil, M., Talabi, A. O., Annor, B., Oyekunle, M., et al. (2015). Heterotic responses among crosses of IITA and CIMMYT early white maize inbred lines under multiple stress environments. Euphytica. 206, 245–262. doi: 10.1007/s10681-015-1506-0
Baker, R. J. (1978). Issues in diallel analysis. Crop Sci. 18, 533–536. doi: 10.2135/CROPSCI1978.0011183X001800040001X
Bänziger, M., Betrán, F. J., Lafitte, H. R. (1997). Efficiency of high-nitrogen selection environments for improving maize for low-nitrogen target environments. Crop Sci. 37, 1103–1109. doi: 10.2135/cropsci1997.0011183X003700040012x
Bänziger, M., Edmeades, G. O., Beck, D., Bellon, M. (2000). Breeding for drought and nitrogen stress tolerance in maize: from theory to practice. Mexico D.F.: CIMMYT, 1–68.
Barbosa, P. A. M., Fritsche-Neto, R., Andrade, M. C., Petroli, C. D., Burgueño, J., Galli, G., et al. (2021). Introgression of maize diversity for drought tolerance: subtropical maize landraces as source of new positive variants. Front. Plant Sci. 12. doi: 10.3389/fpls.2021.691211
Betrán, F. J., Beck, D., Bänziger, M., Edmeades, G. O. (2003b). Secondary traits in parental inbreds and hybrids under stress and non-stress environments in tropical maize. Field Crops Res. 83, 51–65. doi: 10.1016/S0378-4290(03)00061-3
Betrán, F. J., Ribaut, J. M., Beck, D., Gonzalez De León, D. (2003a). Genetic diversity, specific combining ability, and heterosis in tropical maize under stress and nonstress environments. Crop Sci. 43, 807–817. doi: 10.2135/cropsci2003.7970
Beyene, Y., Gowda, M., Pérez-Rodríguez, P., Olsen, M., Robbins, K. R., Burgueño, J., et al. (2021). Application of genomic selection at the early stage of breeding pipeline in tropical maize. Front. Plant Sci. 12. doi: 10.3389/FPLS.2021.685488/BIBTEX
Beyene, Y., Gowda, M., Suresh, L. M., Mugo, S., Olsen, M., Oikeh, S. O., et al. (2017). Genetic analysis of tropical maize inbred lines for resistance to maize lethal necrosis disease. Euphytica 213, 224. doi: 10.1007/s10681-017-2012-3
Beyene, Y., Mugo, S., Semagn, K., Asea, G., Trevisan, W., Tarekegne, A., et al. (2013). Genetic distance among doubled haploid maize lines and their testcross performance under drought stress and non-stress conditions. Euphytica 192, 379–392. doi: 10.1007/s10681-013-0867-5
Butler, D. G., Cullis, B. R., Gilmour, A. R., Gogel, B. J. (2009). ASReml-R reference manual (Brisbane: The State of Queensland, Department of Primary Industries and Fisheries).
Cairns, J. E., Chamberlin, J., Rutsaert, P., Voss, R. C., Ndhlela, T., Magorokosho, C. (2021). Challenges for sustainable maize production of smallholder farmers in sub-Saharan Africa. J. Cereal Sci. 101, 103274. doi: 10.1016/j.jcs.2021.103274
Cairns, J. E., Prasanna, B. M. (2018). Developing and deploying climate-resilient maize varieties in the developing world. Curr. Opin. Plant Biol. 45, 226–230. doi: 10.1016/j.pbi.2018.05.004
Comstock, R. E., Robinson, H. F. (1948). The components of genetic variance in populations of biparental progenies and their use in estimating the average degree of dominance. Biometrics 4, 254. doi: 10.2307/3001412
Cooper, M., Gho, C., Leafgren, R., Tang, T., Messina, C. (2014). Breeding drought-tolerant maize hybrids for the US corn-belt: Discovery to product. J. Exp. Bot. 65, 6191–6204. doi: 10.1093/jxb/eru064
Cooper, M., Messina, C. D. (2023). Breeding crops for drought-affected environments and improved climate resilience. Plant Cell 35, 162–186. doi: 10.1093/PLCELL/KOAC321
de Souza, L. V., Miranda, G. V., Cardoso Galvão, J. C., Moreira Guimarães, L. J., dos Santos, I. C. (2009). Combining ability of maize grain yield under different levels of environmental stress. Pesquisa Agropecuaria Bras. 44, 1297–1303. doi: 10.1590/s0100-204x2009001000013
Edmeades, G. O., Bolaños, J., Chapman, S. C., Lafitte, H. R., Bänziger, M. (1999). Selection improves drought tolerance in tropical maize populations: I. Gains in biomass, grain yield, harvest index. Crop Sci. 39, 1306–1315. doi: 10.2135/cropsci1999.3951306x
Edmeades, G. O., Bolanos, J., Elings, A., Ribaut, J. M., Banziger, M., Westgate, M. E. (2015). “The role and regulation of the anthesis-silking interval in maize,” in CSSA Special Publications, eds. Westgate, M., Boote, K., Knievel, D., Kiniry, J. (Madison, WI, USA: Crop Science Society of America and American Society of Agronomy), 43–73. doi: 10.2135/cssaspecpub29.c4
Ekpa, O., Palacios-Rojas, N., Kruseman, G., Fogliano, V., Linnemann, A. R. (2018). Sub-Saharan African maize-based foods: Technological perspectives to increase the food and nutrition security impacts of maize breeding programmes. Global Food Secur. 17, 48–56. doi: 10.1016/j.gfs.2018.03.007
Erenstein, O., Jaleta, M., Sonder, K., Mottaleb, K., Prasanna, B. M. (2022). Global maize production, consumption and trade: trends and R&D implications. Food Secur. 14, 1295–1319. doi: 10.1007/s12571-022-01288-7
Ertiro, B. T., Beyene, Y., Das, B., Mugo, S., Olsen, M., Oikeh, S., et al. (2017). Combining ability and testcross performance of drought-tolerant maize inbred lines under stress and non-stress environments in Kenya. Plant Breed. = Z. Fur Pflanzenzuchtung 136, 197–205. doi: 10.1111/PBR.12464
Ertiro, B. T., Das, B., Kosgei, T., Tesfaye, A. T., Labuschagne, M. T., Worku, M. R., et al. (2022). Relationship between grain yield and quality traits under optimum and low-nitrogen stress environments in tropical maize. Agronomy 12, 197–205. doi: 10.3390/agronomy12020438
Food and Agriculture Organization (FAO). (2022). Cereal supply and demand balances for sub-Saharan African countries. (FAO). doi: 10.4060/cb2333en
Fisher, M., Abate, T., Lunduka, R. W., Asnake, W., Alemayehu, Y., Madulu, R. B. (2015). Drought tolerant maize for farmer adaptation to drought in sub-Saharan Africa: Determinants of adoption in eastern and southern Africa. Climatic Change 133, 283–299. doi: 10.1007/s10584-015-1459-2
Giampaoli, V., Singer, J. M. (2009). Likelihood ratio tests for variance components in linear mixed models. J. Stat. Plann. Inference 139, 1435–1448. doi: 10.1016/j.jspi.2008.06.016
Griffing, B. (1956). Concept of general and specific combining ability in relation to diallel crossing systems. Aust. J. Biol. Sci. 9, 463–493. doi: 10.1071/bi9560463
Hallauer, A. R., Carena, M. J., Miranda Filho, J. B. (2010). Quantitative genetics in maize breeding, Ed. 3. New York ; London: Springer.
Kim, H. C., Moon, J.-C., Kim, J. Y., Song, K., Kim, K.-H., Lee, B.-M. (2017). Evaluation of drought tolerance using anthesis-silking interval in maize. Korean J. Crop Sci. 62, 24–31. doi: 10.7740/kjcs.2016.62.1.024
Liu, X., Hu, X., Li, K., Liu, Z., Wu, Y., Feng, G., et al. (2021). Identifying quantitative trait loci for the general combining ability of yield-relevant traits in maize. Breed. Sci. 71, 217–228. doi: 10.1270/jsbbs.20008
Lu, Y., Hao, Z., Xie, C., Crossa, J., Araus, J.-L., Gao, S., et al. (2011). Large-scale screening for maize drought resistance using multiple selection criteria evaluated under water-stressed and well-watered environments. Field Crops Res. 124, 37–45. doi: 10.1016/j.fcr.2011.06.003
Makumbi, D., Betra, J. F., Ba, M. (2011). Combining ability, heterosis and genetic diversity in tropical maize (Zea mays L.) under stress and non-stress conditions. Euphytica 180, 143–162. doi: 10.1007/s10681-010-0334-5
Masuka, B., Atlin, G. N., Olsen, M., Magorokosho, C., Labuschagne, M., Crossa, J., et al. (2017). Gains in maize genetic improvement in Eastern and Southern Africa: I. CIMMYT hybrid breeding pipeline. Crop Sci. 57, 168–179. doi: 10.2135/CROPSCI2016.05.0343
Matongera, N., Ndhlela, T., van Biljon, A., Kamutando, C. N., Labuschagne, M. (2023). Combining ability and testcross performance of multi-nutrient maize under stress and non-stress environments. Front. Plant Sci. 14. doi: 10.3389/fpls.2023.1070302
Mcmillen, M. S., Mahama, A. A., Sibiya, J., Lübberstedt, T., Suza, W. P. (2022). Improving drought tolerance in maize: Tools and techniques. Front. Genet. 13, 1001001. doi: 10.3389/fgene.2022.1001001
Ndlovu, N., Gowda, M., Beyene, Y., Chaikam, V., Nzuve, F. M., Makumbi, D., et al. (2024). Genomic loci associated with grain yield under well-watered and water-stressed conditions in multiple bi-parental maize populations. Front. Sustain. Food Syst. 8, 1391989. doi: 10.3389/FSUFS.2024.1391989/FULL
Ndlovu, N., Spillane, C., McKeown, P. C., Cairns, J. E., Das, B., Gowda, M. (2022). Genome-wide association studies of grain yield and quality traits under optimum and low-nitrogen stress in tropical maize (Zea mays L.). Theor. Appl. Genet. 135, 4351–4370. doi: 10.1007/S00122-022-04224-7/FIGURES/4
Nurmberg, P. L., Brito, A., Zimmer, C. M., Schwantes, D. O., Mantovani, E. E., Fátima, B., et al. (2022). A commercial breeding perspective of maize improvement for drought stress tolerance. Revista Brasileira de Milho e Sorgo 21. doi: 10.18512/rbms2022v21e1277
Oluwaseun, O., Badu-Apraku, B., Adebayo, M., Abubakar, A. M. (2022). Combining ability and performance of extra-early maturing provitamin a maize inbreds and derived hybrids in multiple environments. Plants 11, 964. doi: 10.3390/plants11070964
Osuman, A. S., Badu-Apraku, B., Ifie, B. E., Nelimor, C., Tongoona, P., Obeng-Bio, E., et al. (2022). Combining ability and heterotic patterns of tropical early-maturing maize inbred lines under individual and combined heat and drought environments. Plants 11, 1365. doi: 10.3390/plants11101365
Oyekunle, M. (2014). Genetic analysis of grain yield and other traits of early- maturing maize inbreds under drought and well-watered conditions. J. Agron. Crop Sci. 200, 92–107. doi: 10.1111/jac.12049
Oyekunle, M., Badu-Apraku, B. (2018). Assessment of interrelationships among grain yield and secondary traits of early-maturing maize inbred lines under drought and well-watered conditions. Maydica 63, 1–10.
Pinho, R. G. V., Vieira Silva, E. V., De Oliveira, T. L. (2022). Challenges of maize breeding under tropical conditions of brazil. Revista Brasileira de Milho e Sorgo, 21, 101976. doi: 10.18512/rbms2022vol21e1258
Poole, N., Donovan, J., Erenstein, O. (2021). Viewpoint: Agri-nutrition research: Revisiting the contribution of maize and wheat to human nutrition and health. Food Policy 100, 101976. doi: 10.1016/J.FOODPOL.2020.101976
Prasanna, B. M., Burgueño, J., Beyene, Y., Makumbi, D., Asea, G., Woyengo, V., et al. (2022). Genetic trends in CIMMYT’s tropical maize breeding pipelines. Sci. Rep. 12, 1–13. doi: 10.1038/s41598-022-24536-4
Prasanna, B. M., Cairns, J. E., Zaidi, P. H., Beyene, Y., Makumbi, D., Gowda, M., et al. (2021). Beat the stress: breeding for climate resilience in maize for the tropical rainfed environments. Theor. Appl. Genet. 134, 1729–1752. doi: 10.1007/s00122-021-03773-7
Prasanna, B. M., Nair, S. K., Babu, R., Gowda, M., Zhang, X., Xu, Y., et al. (2020a). “Increasing genetic gains in maize in stress-prone environments of the tropics,” in Genomic Designing of Climate-Smart Cereal Crops, ed. Kole, C. (Cham: Springer International Publishing), 97–132. doi: 10.1007/978-3-319-93381-8_3
Prasanna, B. M., Palacios-Rojas, N., Hossain, F., Muthusamy, V., Menkir, A., Dhliwayo, T., et al. (2020b). Molecular breeding for nutritionally enriched maize: status and prospects. Front. Genet. 10. doi: 10.3389/fgene.2019.01392
R Core Team (2023). R: A language and environment for statistical computing (Vienna, Austria: R Foundation for Statistical Computing). Available online at: https://www.R-project.org/.
Ray, D. K., West, P. C., Clark, M., Gerber, J. S., Prishchepov, A. V., Chatterjee, S. (2019). Climate change has likely already affected global food production. PloS One 14, 1–18. doi: 10.1371/journal.pone.0217148
Rezende, W. S., Beyene, Y., Mugo, S., Ndou, E., Gowda, M., Sserumaga, J. P., et al. (2020). Performance and yield stability of maize hybrids in stress-prone environments in eastern Africa. Crop J. 8, 107–118. doi: 10.1016/j.cj.2019.08.001
Rodríguez, F., Alvarado, G., Pacheco, Á., Crossa, J., Burgueño, J. (2015). “Analysis of genetic designs with r for windows (AGD-r) version 3.0,“ in Biometrics and statistical unit (El Batań, Mexico: CIMMYT).
Sang, Z.q., Zhang, Z.q., Yang, Y.x., Li, Z.w., Liu, X.g., Xu, Y., et al. (2022). Heterosis and heterotic patterns of maize germplasm revealed by a multiple-hybrid population under well-watered and drought-stressed conditions. J. Integr. Agric. 21, 2477–2491. doi: 10.1016/J.JIA.2022.07.006
Santos, W., Beyene, Y., Mugo, S., Ndou, E., Gowda, M., Pyton, J., et al. (2019). Performance and yield stability of maize hybrids in stress-prone environments in eastern Africa ☆. Crop J. 8, 107–118. doi: 10.1016/j.cj.2019.08.001
Santpoort, R. (2020). THE drivers of maize area expansion in sub-Saharan Africa. How policies to boost maize production overlook the interests of smallholder farmers. Land 9, 68. doi: 10.3390/land9030068
Schrag, T. A., Möhring, J., Maurer, H. P., Dhillon, B. S., Melchinger, A. E., Piepho, H. P., et al. (2009). Molecular marker-based prediction of hybrid performance in maize using unbalanced data from multiple experiments with factorial crosses. Theor. Appl. Genet. 118, 741–751. doi: 10.1007/s00122-008-0934-9
Sheoran, S., Kaur, Y., Kumar, S., Shukla, S., Rakshit, S., Kumar, R. (2022). Recent advances for drought stress tolerance in maize (Zea mays L.): present status and future prospects. Front. Plant Sci. 13. doi: 10.3389/fpls.2022.872566
Singh, R. K., Chaudhary, B. D. (1985). Biometrical Method in Quantitative Genetics Analysis New Delhi: Kalyani Publishers.
Sorsa, Z., Mohammed, W., Wegary, D., Tarkegne, A. (2023). Performances of three-way cross hybrids over their respective single crosses and related heterosis of maize (Zea mays L.) hybrids evaluated in Ethiopia. Heliyon 9, e15513. doi: 10.1016/J.HELIYON.2023.E15513
Sserumaga, J. P., Makumbi, D., Oikeh, S. O., Otim, M., Machida, L., Anani, B. Y., et al. (2021). Evaluation of early-generation tropical maize testcrosses for grain-yield potential and weevil (Sitophilus zeamais Motschulsky) resistance. Crop Prot. 139, 105384. doi: 10.1016/j.cropro.2020.105384
Sserumaga, J. P., Oikeh, S. O., Mugo, S., Asea, G., Otim, M., Beyene, Y., et al. (2016). Genotype by environment interactions and agronomic performance of doubled haploids testcross maize (Zea mays L.) hybrids. Euphytica 207, 353–365. doi: 10.1007/s10681-015-1549-2
Stram, D. O., Lee, J. W. (1994). Variance components testing in the longitudinal mixed effects model. Biometrics 50, 1171. doi: 10.2307/2533455
Talabi, A. O., Badu-Apraku, B., Fakorede, M. A. B. (2017). Genetic variances and relationship among traits of an early maturing maize population under drought-stress and low nitrogen environments. Crop Sci. 57, 681–692. doi: 10.2135/cropsci2016.03.0177
Trachsel, S., Leyva, M., Lopez, M., Suarez, E. A., Mendoza, A., Montiel, N. G., et al. (2016). Identification of tropical maize germplasm with tolerance to drought, nitrogen deficiency, and combined heat and drought stresses. Crop Sci. 56, 3031–3045. doi: 10.2135/cropsci2016.03.0182
Yan, W. (2014). Crop Variety Trials: Data Management and Analysis. Hoboken, NJ: John Wiley & Sons, Blackwell. doi: 10.1002/9781118688571
Zaman-Allah, M., Zaidi, P. H., Trachsel, S., Cairns, J. E., Vinayan, M. T., Seetharam, K. (2016). Phenotyping For Abiotic Stress Tolerance In Maize: Drought Stress India: CIMMYT.
Zhao, C., Liu, B., Piao, S., Wang, X., Lobell, D. B., Huang, Y., et al. (2017). Temperature increase reduces global yields of major crops in four independent estimates. Proc. Natl. Acad. Sci. United States America 114, 9326–9331. doi: 10.1073/pnas.1701762114
Keywords: drought, grain yield, general combining ability, line-by-tester design, specific combining ability, maize
Citation: Manigben KA, Beyene Y, Chaikam V, Tongoona PB, Danquah EY, Ifie BE, Aleri I, Chavangi A, Prasanna BM and Gowda M (2024) Testcross performance and combining ability of intermediate maturing drought tolerant maize inbred lines in Sub-Saharan Africa. Front. Plant Sci. 15:1471041. doi: 10.3389/fpls.2024.1471041
Received: 26 July 2024; Accepted: 30 October 2024;
Published: 28 November 2024.
Edited by:
Leonardo Velasco, Spanish National Research Council (CSIC), SpainReviewed by:
Edmore Gasura, University of Zimbabwe, ZimbabwePeng Jiao, Jilin Agricultural University, China
Copyright © 2024 Manigben, Beyene, Chaikam, Tongoona, Danquah, Ifie, Aleri, Chavangi, Prasanna and Gowda. This is an open-access article distributed under the terms of the Creative Commons Attribution License (CC BY). The use, distribution or reproduction in other forums is permitted, provided the original author(s) and the copyright owner(s) are credited and that the original publication in this journal is cited, in accordance with accepted academic practice. No use, distribution or reproduction is permitted which does not comply with these terms.
*Correspondence: Manje Gowda, bS5nb3dkYUBjZ2lhci5vcmc=; Yoseph Beyene, eS5iZXllbmVAY2dpYXIub3Jn