- 1School of Pharmaceutical Sciences, Yunnan Key Laboratory of Pharmacology for Natural Products, and Yunnan College of Modern Biomedical Industry, Kunming Medical University, Kunming, Yunnan, China
- 2Germplasm Bank of Wild Species, Kunming Institute of Botany, Chinese Academy of Sciences, Kunming, Yunnan, China
- 3University of Chinese Academy of Sciences, Beijing, China
Gaultheria Kalm ex L. (Ericaceae), a type of evergreen shrub, known as a natural source of methyl salicylate, possesses rich germplasm resources, strong habitat adaptability, significant ornamental value, and noteworthy pharmacological activities. However, due to the paucity of whole genomic information, genetically deep research in these areas remains limited. Consequently, we intend to obtain genome data through high-throughput sequencing, gene annotation, flow cytometry, transcription factors prediction and genetic marker analysis for a representative species of this genus, with Gaultheria prostrata selected for our study. In this study, we preliminarily obtained the genome of G. prostrata through next-generation sequencing methods. Utilizing 47.94 Gb of high-quality sequence data (108.95× coverage), assembled into 114,436 scaffolds, with an N50 length of 33,667 bp. The genome size assembled by SOAPdenovo, approximately 417 Mb, corresponded closely to predictions by flow cytometry (440 Mb) and k-mer analysis (447 Mb). The genome integrity was evaluated using BUSCO with 91%. The heterozygosity ratio was 0.159%, the GC content was 38.85%, and the repetitive regions encompassed over 34.6% of the genome. A total of 26,497 protein-coding genes have been predicted and annotated across Nr, Swissprot, GO, KEGG, and Pfam databases. Among these, 14,377 and 2,387 genes received functional annotation in Nr and Swissprot, respectively; 21,895, 24,424, and 22,330 genes were similarly annotated in GO, KEGG, and Pfam. Moreover, A total of 279,785 SSRs were identified and 345,270 primers for these SSRs were designed. Within the various nucleotide types of SSRs, AG/CT and AAG/CTT constituted the predominant dinucleotide and trinucleotide repeat types in G. prostrata. In addition, 1,395 transcription factors (TFs) from 75 TF families, 462 transcription regulators (TRs) from 33 TR families and 840 protein kinase (PKs) from 118 PK families were identified in this genome. We also performed phylogenetic analyses of G. prostrata and related species, including estimation of divergence times and expansion and contraction analyses, followed by positive selection analyses of orthologous gene pairs of G. prostrata and its close relative Vaccinium corymbosum. These results provide a reference for in-depth study of genus Gaultheria, contributing to future functional and comparative genomics analyses and providing supporting data for the development of molecular markers.
Introduction
High-altitude woody plants, comprising both diploid and polyploid species, adapt through reinforced structures, deep roots, antifreeze proteins, elevated antioxidant enzyme activity, and systemic pathogen resistance, enabling them to withstand cold, UV exposure, and other environmental stresses (Nie et al., 2005; Couto and Zipfel, 2016; Ding et al., 2023a, 2023b). Like many montane woody plants, Gaultheria Kalm ex L. (Ericaceae), one of the most recently diverged taxa within Ericaceae with significant ploidy diversity, demonstrates remarkable adaptability. This genus is renowned not only for its rich germplasm resources, ornamental value, and notable pharmacological effects but also as a natural source of methyl salicylate, with strong adaptability to diverse habitats. The genus Gaultheria exhibits these traits largely due to its belonging to the Ericaceae family, renowned for its exceptional ecological adaptability, diverse symbiotic relationships, and resilience to environmental stressors. These qualities allow it to flourish and sustain biodiversity across a broad spectrum of extreme environments globally (Choudhary et al., 2021; BiologyInsights, 2024).
Notably, a lot of genome resources are still needed to reveal the complex evolutionary mechanisms of Ericaceae, but currently high-quality genome research is only focused on the two genera of Rhododendron (Yang et al., 2020) and Vaccinium (Cui et al., 2022). A large number of genome resources from other genera are urgently needed to supplement the research, especially the evolutionarily important and latest diverged Gaultheria, where there is no research on the whole genome. The analysis of Gaultheria’s evolutionary pattern mainly relies on chloroplast genomes or even nuclear gene fragments (Cheng et al., 2024). This limitation hinders the in-depth exploration of its genetic diversity, adaptive characteristics and evolutionary mechanisms. Hence, it is imperative to select representative species of the genus Gaultheria for high-throughput omics data to fill the genetic data gap of the genus, and deeply analyze the genome structure and gene expression pattern of the genus, so as to lay a solid foundation for exploring its diverse adaptation strategies. This dataset will then support broader research into the adaptive and evolutionary patterns of the Ericaceae family, further advancing knowledge in plant genomics.
A presumably diploid species within the Sympodial clade, Gaultheria prostrata, possesses the highest altitude distribution characterized by Rhododendron forests, Abies forest margins, shrublands, and rocky areas at altitudes between 4200 and 4800 meters in Himalaya-Hengduan Mountains, according to the sampling point records and reports from Flora of China (Wu et al., 2005). To be specific, G. prostrata is highly rich in methyl salicylate, accounting for about 70% of its own volatile oil content (Liu et al., 2013). This compound is not only widely used in the medical field (Li et al., 2016); more importantly, methyl salicylate’s functions as a signaling molecule, triggering systemic acquired resistance (SAR) (Chen et al., 2019b) and potentially enhancing defense abilities against environmental stresses typical of high altitudes, such as UV radiation and low temperatures, thereby likely aiding G. prostrata in adapting to high-altitude habitats. Deciphering G. prostrata’s genome will not only shed light the selection pressures on high-altitude diploid adaptive genes and their specific adaptive strategies, but also analyzes the role of its stress-resistant chemical composition in environmental adaptation.
To achieve the above goals, leveraging Next-Generation Sequencing (NGS) technology will be essential. k-mer analysis using NGS to enable access to a species’ genome size, GC content, repetition rate, and heterozygosity rates (Shan et al., 2020). In addition, this technology is also indispensable for analyzing the high-altitude adaptation mechanism of plants. For instance, a study utilized NGS technology to conduct a detailed analysis of the genomes of Roscoea alpina and Roscoea purpurea, exploring their adaptive differences at varying altitudes and revealing the genes and functions associated with high-altitude adaptation (Wang et al., 2024a). Another study employed NGS technology identifying key genes involved in Saussurea obvallata and Rheum alexandrae’s adaptation to high-altitude environments, such as DNA repair and antioxidant stress response genes (Zhang et al., 2023b). Besides, SSR (simple sequence repeat) marker is especially useful for researchers working on non-model organisms where genome resources might be limited, which helps in developing species-specific genetic markers. The combined use of NGS and SSR marker technologies will enable a comprehensive analysis of the unique high-altitude adaptation strategies of G. prostrata.
In summary, this study employed high-throughput sequencing technology to complete the sequencing, assembly, and annotation of the first genome of G. prostrata in the genus Gaultheria, providing foundational data for further exploration of its high-altitude adaptation. Subsequently, we utilized flow cytometry, genome survey analysis, and phylogenetic analysis to determine the genome size, and characteristics of this species, and to clarify their evolutionary relationships within the order Ericales. We also performed functional enrichment analysis on gene families involved in expansion/contraction and genes under positive selection. Then, we also designed several SSR markers to facilitate the construction of the genetic map of Gaultheria species. This provides important references for subsequent chromosome-level sequencing and supports in-depth research on functional genes and adaptations against stresses at high altitudes in Gaultheria species.
Materials and methods
DNA and RNA extraction from plant materials
DNA extraction
Three young leaves from an individual of G. prostrata were collected from tissue cultured plantlets at 25 ± 2°C, 16 h/8 h (light/dark) photoperiod, and 2000 lx illumination intensity. Leaf tissues were ground with liquid nitrogen and DNA was extracted using Biomarker Plant DNA Kit (Biomarker Technologies, Beijing, China). The red-boxed sample labeled “LL-2021-3,” representing G. prostrata (Supplementary Figure S1), shows a distinct band without smearing or blurring and no visible contaminants. The band intensity is appropriate, indicating that the DNA concentration is within an optimal range, which is typically indicative of high-quality DNA extraction (Sambrook and Russell, 2001). Additionally, we measured the DNA concentration using a Qubit 3.0 Fluorometer (Thermo Fisher Scientific), obtaining a result of 41 ng/µL. This indicates that the extracted DNA is of high quality and has an appropriate concentration, suitable for most molecular biology experiments (Thermo Fisher Scientific, 2014). The qualified genomic DNA is stored at -80°C for further use.
RNA extraction
Roots, stems, and leaves of G. prostrata were collected for RNA-Seq analysis, using three biological replicates for each tissue type. Immediately after harvested, samples were frozen in liquid nitrogen and stored at -80°C until extraction. Total RNA was extracted using the Trizol method (Honaas and Kahn, 2017).
Genome size and ploidy level estimation by flow cytometry
Genome size estimation
Three young leaves from each plant were collected and immediately chopped in 0.8 mL of cold nuclei isolation buffer (45 mM MgCl2·6H2O, 20 mM MOPS, 30 mM Na-Citrate, 1% (w/v) PVP 40, 0.2% (v/v) Triton X-100, 10 mM Na2EDTA, 20 μL/mL β-mercaptoethanol, pH 7.5). The homogenate was filtered and transferred into the 1.5 mL tube using 40 μm nylon mesh. Then 50 mg/mL of DNA fluorochrome propidium iodide (PI) and 50 mg/mL of RNase were added and mixed gently with the samples. Before analysis, nuclei were stained on ice for 1 h away from light with occasional shaking. Solanum lycopersicum and Zea mays B73 was selected as internal references to detect the genomic DNA contents of G. prostrata. The fluorescence intensity of the emitted light of propidium iodide was detected using a BD FACScalibur flow cytometer (Becton Dickinson, NY, USA) on a sample of stained cell nucleus suspension using a 488 nm blue light excitation (Zhang et al., 2023a).
Ploidy level estimation
Flow cytometry is also a useful tool for estimating plant ploidy levels. This entails comparing the target species’ genome size with a known ploidy sample (karyotyped) or a consistent internal standard (Here, we selected two: S. lycopersicum, Z. mays), requiring karyotyping for at least one target species (Bourge et al., 2018; Pellicer et al., 2021). To deduce the ploidy level of only one species, G. prostrata, species with established ploidy levels and consistent internal standards were selected, including G. griffithiana (2n=4x=44) (Middleton and Wilcock, 1990), G. crenulata (2n=4x=44) (Middleton and Wilcock, 1990), Vaccinium corymbosum (2n=4x=44) (Yocca et al., 2023), and V. macrocarpon (2n=2x=44) (Yocca et al., 2023).
Genome sequencing, transcriptome sequencing and genome evaluation
A genomic paired-end library with 270 bp insertions was performed on a BGISeq sequencing platform (MGI-2000) using the G. prostrata leaves. To minimize the impact of sequencing errors on the assembly, we used SOAPnuke (v1.6.5) software (Chen et al., 2018) to filter the raw sequencing data removing low quality reads with splice contamination and PCR duplication. Transcriptome sequencing is then conducted on both ends of the library using the DNBSEQ sequencing platform (BGI). The raw sequencing data undergo quality control using SOAPnuke (v1.6.5) too. During this step, reads containing adapter sequences, reads with more than 1% unknown bases (N), and low-quality reads (where more than 40% of bases have a quality score below 15) are filtered out. The result is a set of high-quality, clean data ready for downstream analysis. To estimate the genome size and heterozygosity and GC content of G. prostrata, Jellyfish (v2.1.4) software (Marcais and Kingsford, 2011) was used to analyze the k-mer depth distribution sequence of the filtered reads. Then the k-value of 21 was selected and GenomeScope2.0 software (Ranallo-Benavidez et al., 2020) was used to perform ploidy fitting to evaluate genomic characteristics. At the same time, utilizing k-mer (k = 21) histograms generated by KMC (v3.1.0) (Kokot et al., 2017), heterozygous k-mer pairs were analyzed with Smudgeplot (v0.2.5) (Ranallo-Benavidez et al., 2020) to estimate ploidy levels and infer genomic complexity. Lower (L) and upper (U) cut-off values were set based on k-mer coverage output from Genomescope 2.0, following recommendations in the Smudgeplot documentation (https://github.com/KamilSJaron/smudgeplot). k-mers falling below the lower cut-off or above the upper cut-off were discarded as errors. The lower cut-off value (L) was defined as (kcov/2) - 5, where kcov values were inferred from GenomeScope.
Genome assembly, GC depth, SSR characteristics analysis and TFs/TRs/PKs identification
The clean reads obtained by filtering in the previous step were assembled by SOAPdenovo (v2.04) software (Luo et al., 2012). Subsequently, to evaluate the completeness of the genome, we used BUSCO v5.7.1 (Benchmarking Universal Single-Copy Orthologs) tools (Simao et al., 2015) with the embryophyta_odb10 dataset (creation date: 2024-01-08), which consists of 1614 Single-Copy Orthologs from 50 species. In order to measure the sequencing bias of G. prostrata, GC content and average sequencing depth were counted. The level of GC content is important for estimating plant genome size. The average GC sequencing depth was calculated from the assembled sequences along a 10-kb non-overlapping sliding window (Zhou et al., 2013). In addition, the microsatellite identification tool (MISA) (http://pgrc.ipk-gatersle-ben.de/misa/) was used to search for SSR loci on the assembled genome (Beier et al., 2017). The criteria for identifying SSR sequences are as follows: the minimum number of nucleotides repeats for mononucleotide repeat unit is 12 times, the number for dinucleotide repeat unit is 5 times, trinucleotide is 4 times, and the number for tetranucleotide, pentanucleotide, or hexanucleotide repeat unit is 3 times (Xu et al., 2022). Considering that mononucleotide repeat unit and compound repeat unit are not suitable as candidates for SSR marker development, these SSRs have been eliminated. Therefore, the main participants in primer design are di-, tri-, tetra-, penta-, hexa- nucleotides. Then, Primer 3.0 software was used to preliminarily design the primer pairs with parameters of final product length 80–250 bp, primer size of 18–25 bp, GC content of 35–70%, and annealing temperature of 50–60°C (Wang et al., 2018). After the design was completed, reduce_ssr.py (Yang et al., 2015) was used to remove the redundancy of the designed primer pairs, and 345,270 non-redundant primer pairs were obtained (The details of these primer pairs are provided in Supplementary Table S1). Finally, genome-wide transcription factors (TFs), transcriptional regulators (TRs), and protein kinases (PKs) were identified and classified using the iTAK (v2.02) software (Zheng et al., 2016).
Gene prediction and annotation
We used RepeatModeler (v2.0.3) software (https://www.repeatmasker.org/RepeatModeler/) to construct a repetitive sequence library of G. prostrata, and used RepeatMasker (v4.1.4) software (https://www.repeatmasker.org/RepeatMasker/) to complete genomic repetitive sequence masking with the help of this repetitive sequence library. Then, we used ab initio prediction, homologous species prediction, and unigene gene prediction to predict and annotate the genes of G. prostrata. MAKER (v3.01.04) pipeline (Cantarel et al., 2008) were used for de novo prediction. It is a powerful analysis process that identifies repetitive sequences, compares ESTs and protein sequences to the genome, then performs de novo prediction using SNAP (v2006-07-28) software (Korf, 2004), Augustus (v3.3.3) software (Stanke et al., 2006), and GeneMark-ES (v4.69) software (Lomsadze et al., 2005), finally integrates the results from all three software to ensure the reliability of the results. In addition, MAKER can be trained continuously, and the initial output can be used as an input file in the algorithm for re-training the gene prediction model, thus obtaining a higher quality gene model. GeMoMa (v1.9) software (Keilwagen et al., 2018) was used to predict homologous data, and TransDecoder (v5.5.0) software (https://github.com/TransDecoder/TransDecoder/wiki) was used to predict transcription data. After completing all the above predictions and annotations, the final results were integrated with EVidenceModeler (v1.1.1) pipeline (https://github.com/EVidenceModeler/EVidenceModeler/wiki) (Altschul et al., 1990). Ultimately, we used GFAP (v3.1) (Xu et al., 2023), a program for functional annotation of plant genes, to perform GO, KEGG, Pfam, nr, and swissprot functional annotations on G. prostrata, respectively.
Phylogenomic analysis, divergence time estimation and expansion and contraction analysis
We used OrthoFinder (v.2.5.5) (Emms and Kelly, 2015) with default parameters to obtain common single-copy genes from the genomes of G. prostrata, six species from the order Ericales (Actinidia chinensis, Rhododendron williamsianum, Vaccinium corymbosum, Aegiceras corniculatum, Diospyros kaki, Camellia sinensis), one species from the family Brassicaceae (Arabidopsis thaliana), and one species from the family Poaceae (Oryza sativa). These single-copy genes were then used to reconstruct the phylogenetic tree, with O. sativa selected as the outgroup. Multiple amino acid sequence alignment was performed using Muscle (v5.1) (Edgar, 2004), followed by extraction of conserved sequences from the aligned files using Gblocks (0.91b) (Talavera and Castresana, 2007) and merging them with seqkit. ProtTest (v3.4.2) (Darriba et al., 2011) was used to predict the appropriate amino acid substitution model, identifying the JTT+I+G+F model as the best fit according to both AIC and BIC criteria. This model was then used to build the tree using RAxML (v8.2.12) (Stamatakis, 2014) with 1000 bootstrap replicates.
Divergence times were estimated using the MCMC Tree method in the PAML package (v.4.10.7) with five calibration constraints [refer to TimeTree (Kumar et al., 2017): http://www.timetree.org and Fossil calibration database (Ksepka et al., 2015): https://fossilcalibrations.org]. These included a fossil constraint for the most recent common ancestor (MRCA) of O. sativa and A. thaliana (142.1 to 163.5 million years ago, Mya); a fossil constraint for the MRCA of A. thaliana and A. corniculatum (111.4 to 123.9 Mya); a fossil constraint for the MRCA of C. sinensis and A. chinensis (82.8 to 106 Mya); a fossil constraint for the MRCA of R. williamsianum and Vaccinioideae (45.5 to 76.9 Mya); and a fossil constraint for the MRCA of V. corymbosum and G. prostrata (4.6 to 59.2 Mya).
CAFE software (v5.1.0) was used to combine the results of the previous phylogenetic analysis to screen out gene families that showed expansion or contraction (Mendes et al., 2020) with parameters set to 10 threads and a significance threshold of P = 0.05. At the same time, Chiplot (Xie et al., 2023) was used to plot pie charts of the proportion of expansion and contraction genes. Those significantly expanded and contracted gene families in G. prostrata were then subjected to functional enrichment analysis using Gene Ontology (GO) and Kyoto Encyclopedia of Genes and Genomes (KEGG) databases to elucidate their potential roles and biological functions.
The species photos were drawn by DALL-E 2 (https://openai.com/index/dall-e-2/)
Ka/Ks positive selection analysis
Initially, we employed WGD software (v1.1) to identify orthologous gene pairs between G. prostrata and its closely related species, V. corymbosum, for whose genomes were available. Subsequently, ParaAT software (v2.0) was used to align homologous sequences and convert amino acid sequences into CDS sequences (coding DNA sequences). Next, KaKs_Calculator software (v2.0) was employed to calculate Ka and Ks values. Finally, a custom Python script was used to screen genes with ω > 1 and perform GO and KEGG databases functional annotations.
Results
Genome size and ploidy level estimation by flow cytometry
Genome size estimation
The flow cytometric analysis produces a high-resolution histogram with mean values of G. prostrata with the internal references including Z. mays and S. lycopersicum. From the results, it can be seen that the internal fluorescence intensities of Z. mays and S. lycopersicum are 45.19 and 21.62, respectively. The CVs of Z. mays, S. lycopersicum and G. prostrata were 6.09%, 8.53% and 11.80%. Compared to them, the fluorescence intensity of G. prostrata is 8.99 and 10.77, respectively (Figure 1A). Besides, the DNA content of G. prostrata is 0.46 pg (1C value) or 0.92 pg (2C value), with an estimated genome size of approximately 440 Mb.
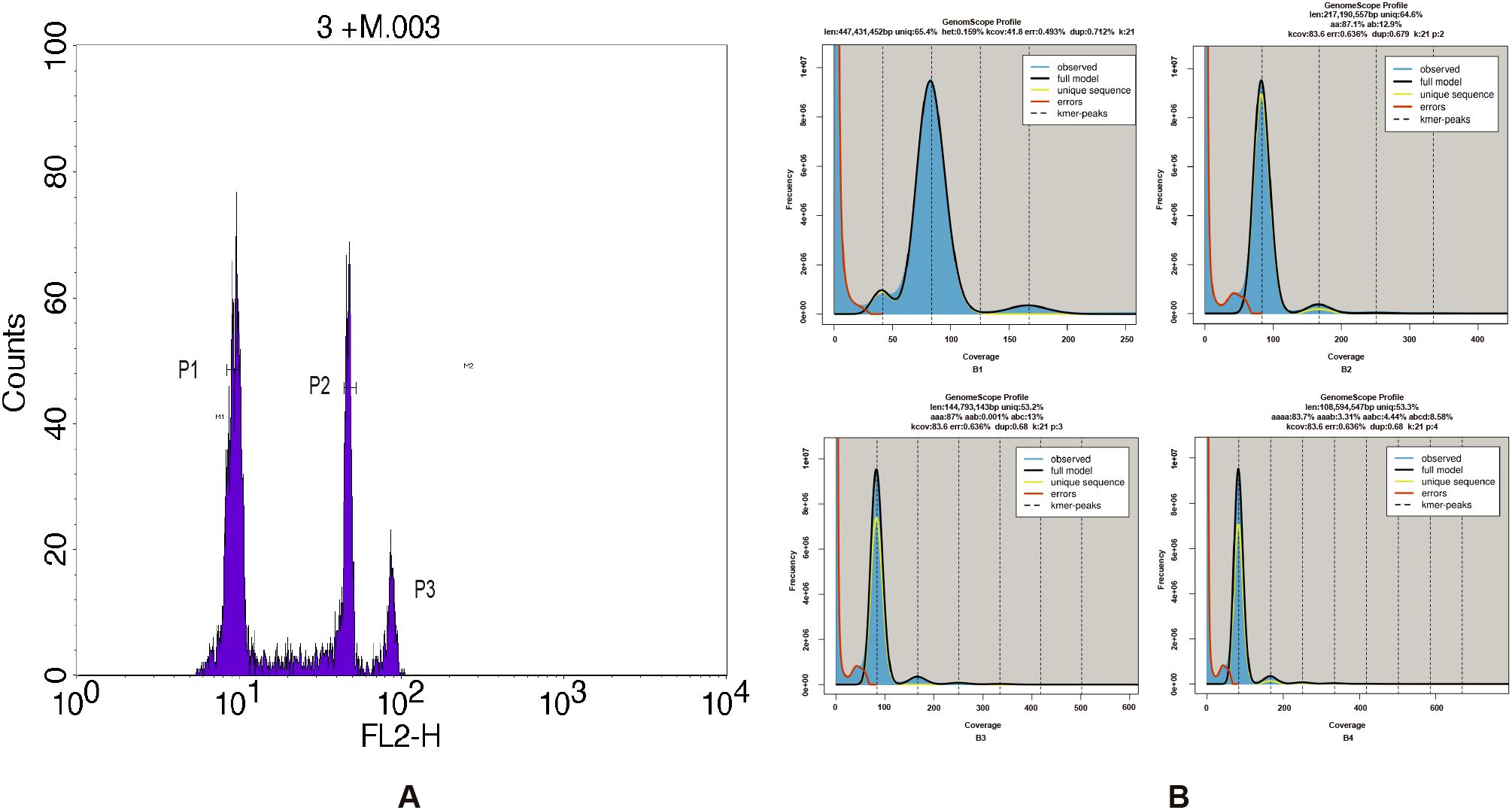
Figure 1. Flow cytometry and k-mer analysis of the Gaultheria prostrata genome. (A) Histogram of relative fluorescence intensity derived from nuclei isolated from Z. mays, S. lycopersicum and G. prostrata processed simultaneously. The horizontal axis represents the fluorescence intensity, and the vertical axis represents the number of cells corresponding to each fluorescence intensity. Peak1, Peak2, and Peak3 correspond to the fluorescence intensity of G. prostrata, S. lycopersicum, and Z. mays samples, respectively. (B) k-mer (k = 21) distribution calculated by Genomescope (B1: GenomeScope 1.0; B2–B4: ploidy fitting by GenomeScope 2.0). The observed k mer distribution is shown as blue bars. The black line models the distribution, excluding k-mer errors (red line) and extends to the maximum k-mer coverage specified in the model (yellow line). Abbreviations are as follows: ‘len’ for estimated genome length, ‘uniq’ for the unique portion of the genome (nonrepetitive elements), ‘het’ for genome heterozygosity, and ‘err’ for the sequencing error rate.
Ploidy level estimation
Tetraploid G. griffithiana and G. crenulata have DNA contents of 2.02 pg (~970 Mb) and 2.07 pg (~990 Mb), respectively. Tetraploid Vaccinium corymbosum and diploid V. macrocarpon’s genome sizes are ~980 Mb and ~500 Mb, respectively. The DNA content and genome size of tetraploid G. griffithiana and G. crenulata are about double those of G. prostrata, within the same genus. In comparison, tetraploid V. corymbosum’s genome is roughly twice, and diploid V. macrocarpon’s genome size closely matches G. prostrata’s. Therefore, G. prostrata is inferred to be a diploid species.
Genome sequencing and genome evaluation
The genome of G. prostrata was sequenced by BGISeq sequencing platform (MGI-2000). After filtering with SOAPnuke, 47.94 Gb high-quality data were obtained. The Q20 was 94.27% and the Q30 was 82.64%. Besides, sequencing depth was 108.95×. All the clean data were used for k-mer analysis (the total k-mer number was 4,257,951,935), By simulating using Jellyfish software, we performed k-mer frequency distribution analysis with K = 21. By calculating using the formula that Genome size=k-mer num/peak depth, the genome size of it was estimated at around 447Mb. Figure 1B (B1) shows the results of GenomeScope software analysis: there are three obvious peaks and the horizontal coordinates corresponding to the peaks are in a multiplicative relationship and there is a certain degree of heterozygosity and the proportion of repetitive sequences, of which the one at depth=42 is a heterozygous peak, the one at depth=83 is the main peak, and the repetitive peak is at depth=166. The heterozygous peak is shown to be at approximately half the depth of the major peak, indicating possible diploidy. A similar pattern is observed in Figure 1B (B2), supporting the inference of diploidy. Although both Figure 1B (B3) and (B4) showed one main peak and multiple other peaks, they had no clear polyploidy characteristics, which made the final ploidy inference biased towards diploidy. Besides, the proportion of repeats in the G. prostrata genome was 34.6% and the heterozygosity was 0.159% (Figure 1B), and it suggested that the genome was not a highly complex one. The ratio of heterozygous k-mer pairs in Smudgeplot also indicates that G. prostrata may be a diploid, with an average of 85% of k-mer pairs belonging to the AB type. Although there is still AAB type, it accounts for a relatively small proportion of only 15% (Figure 2A).
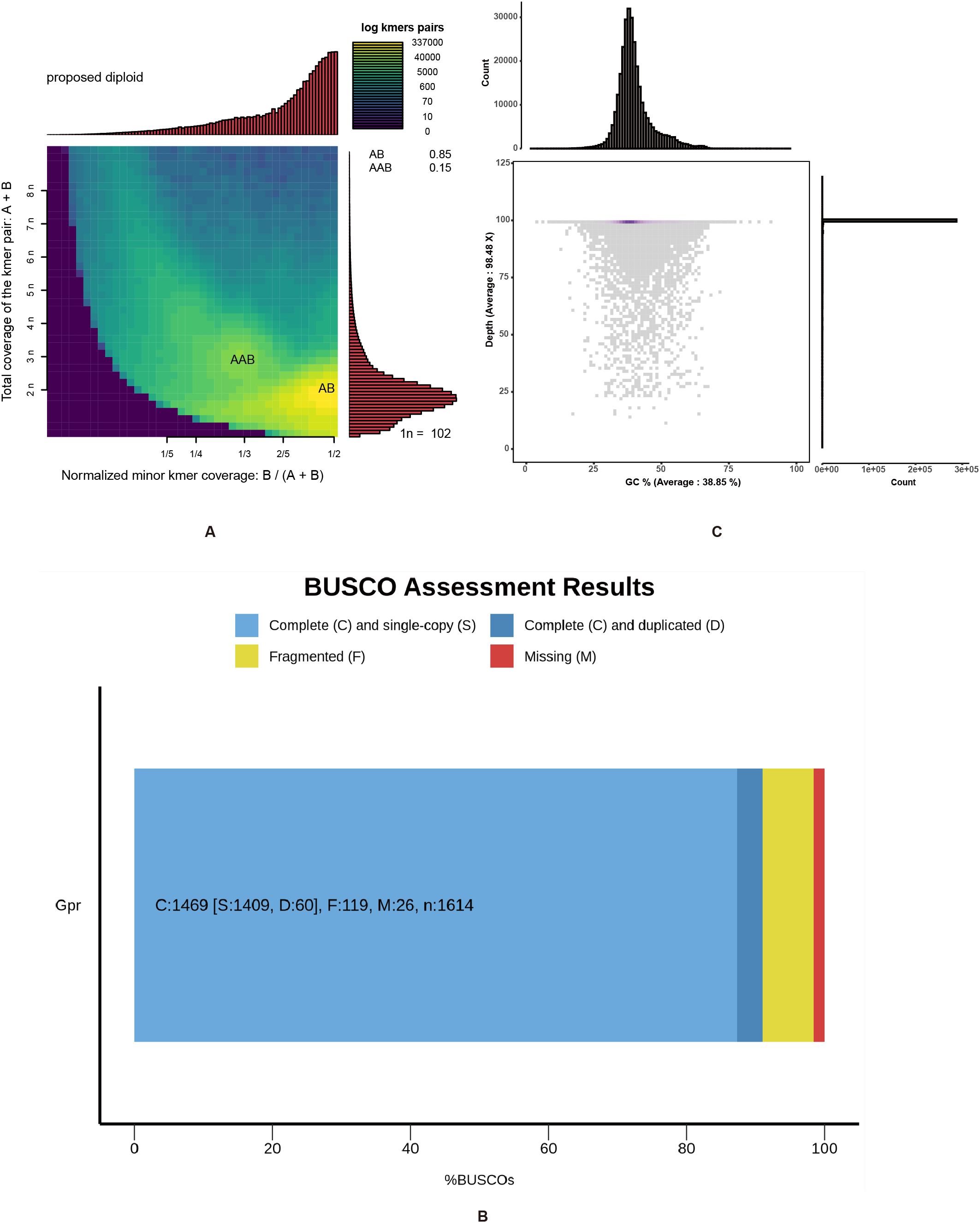
Figure 2. Smudgeplot analysis, BUSCO evaluation and GC-depth distribution of the Gaultheria prostrata genome. (A) Two-dimensional heat maps were constructed using Smudgeplot (k = 21) to illustrate the ploidy predictions from clean reads. Color intensity indicates the approximate number of k-mers per bin, ranging from purple (low) to yellow (high). Estimated ploidies are displayed in the upper left corner of each graph, along with the likelihood of various ploidy levels. The top histogram shows the frequency distribution of different minor k-mer coverage ratios (B/(A + B)). The right histogram shows the frequency distribution of different total coverages (A + B). (B) The BUSCO assessment of genome assembly completeness was conducted. The BUSCO assessment was executed in genome mode using Miniprot as the gene predictor. The x-axis represents the percentage of various types of BUSCOs, and the y-axis indicates the genome names. (C) The figure comprises two histograms and one scatter plot. The upper histogram shares the x-axis with the scatter plot, and the histogram on the right shares the y-axis with the scatter plot. The x-axis of the scatter plot represents GC content, and the y-axis represents sequencing depth; The histogram above the scatter plot displays the count of reads by varying GC contents in the G. prostrata genome; The histogram to the right of the scatter plot illustrates the counts of reads by different sequencing depths in the G. prostrata genome.
Genome assembly, GC depth, SSR characteristics analysis and TFs/TRs/PKs identification
SOAPdenovo software was used for de novo assembly with a 21-mer selection. A total of 417,467,430 scaffolds were assembled into a final genome sequence of 410 Mb with a scaffold N50 length of 33,667 bp (Table 1). The BUSCO genome completeness assessment indicates that 91.0% of the assembly is complete (C: 1,469), with 1,409 (87.3%) being complete and single-copy BUSCOs (S), 60 (3.7%) being complete and duplicated BUSCOs (D), and only 119 (7.4%) being fragmented BUSCOs (F). Out of a total of 1,614 BUSCOs searched, only 26 (2.5%) were missing (M) (Figure 2B). The number of complete (C) BUSCOs is significantly higher than the number of fragmented (F) and missing (M) BUSCOs, suggesting a high-quality genome assembly (More evaluation details are shown in Supplementary Table S2). Besides, the sequences produced by de novo assembly were similar with the genome size estimated by k-mer analysis (447 Mb) and flow cytometry (440 Mb), again indicating that the genome of G. prostrata might be a diploid with a relatively simple structure. Additionally, the GC content and average depth of G. prostrata genome were then calculated with 10-kb non-overlapping sliding window (Figure 2C). The GC content of it was 38.85%, with a mid-GC content between 30 and 50%. Meanwhile, the depth of sequencing was 98.48×, which indicated the high quality of our sequence.
A total of 279,785 SSRs were identified using the Perl script MISA, Dinucleotide repeats (41.33%) constitute the most abundant type, followed by mono- (25.22%), tetra- (12.76%), tri- (11.37%), penta- (6.69%), and hexa- (2.62%) nucleotide repeats. Among them, 463 repeat units were identified, with AG/CT (64.82%) as the predominant type among the dinucleotide repeat motifs, followed by AT/AT (20.11%), AC/GT (14.51%), and CG/CG (0.56%). The three most abundant trinucleotide motifs were AAG/CTT (27.66%), AAT/ATT (17.01%), and ACC/GGT (14.91%) (Figures 3A–D); the four most abundant tetranucleotide motifs were AAAT/ATTT (28.74%), AAAG/CTTT (15.71%), ACCC/GGGT (9.93%), and AATT/AATT (5.50%). Besides, the most abundant pentanucleotide repeats are AAAAT/ATTTT (16.09%), AAAAG/CTTTT (14.50%) and AAACC/GGTTT (8.74%). The most abundant hexanucleotide repeats are AAAAAT/ATTTTT (15.31%) AAAAAG/CTTTTT (11.45%) and AAAAAC/GTTTTT (6.17%)(Supplementary Table S3). Moreover, the frequency of repeats for different types of SSR loci predominantly ranges between 5 and 15 (Shi et al., 2016b). We plotted the frequency of SSR motif repeats (Figure 3E), revealing that the highest percentage is of dinucleotides, followed bymononucleotides, and then trinucleotides. Mononucleotides predominantly occur in the 12-15 frequency range, while dinucleotides are mostly found in the 5-10 frequency range and trinucleotides are in the 5-10 and 15 frequency range. (Supplementary Table S4)
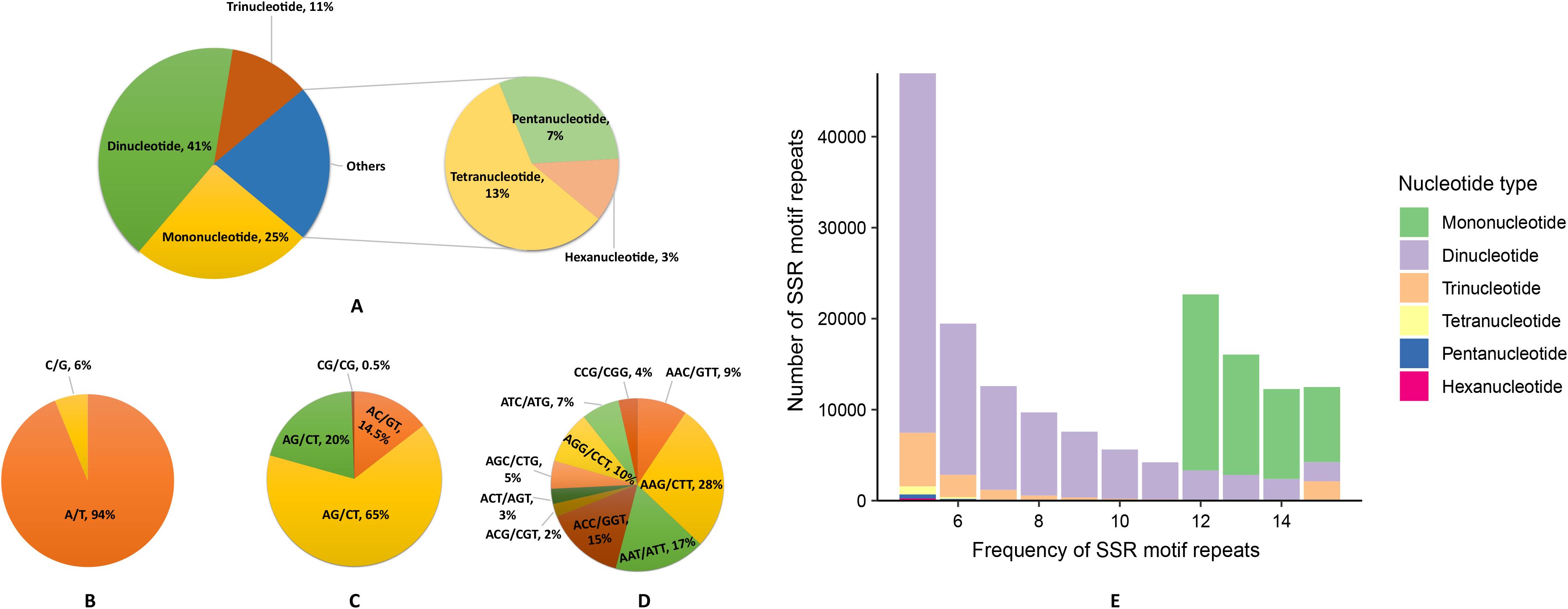
Figure 3. Characteristics and frequency of SSR motif repeats in the Gaultheria prostrata genome. (A) Percentage of different nucleotide types. (B) Percentage of different motifs in mononucleotide repeats. (C) Percentage of different motifs in dinucleotide repeats. (D) Percentage of different motifs in trinucleotide repeats. (E) Number of various SSR motif repeats in different repeat frequencies. (A, E: Various colors represent different nucleotide types; B–D: Colors differentiate nucleotide types.).
In addition, 1,395 transcription factors (TFs) from 75 TF families, 462 transcription regulators (TRs) from 33 TR families and 840 protein kinase (PKs) from 118 PK families were identified in this genome (Supplementary Tables S5, S6; Figures 4A–C):
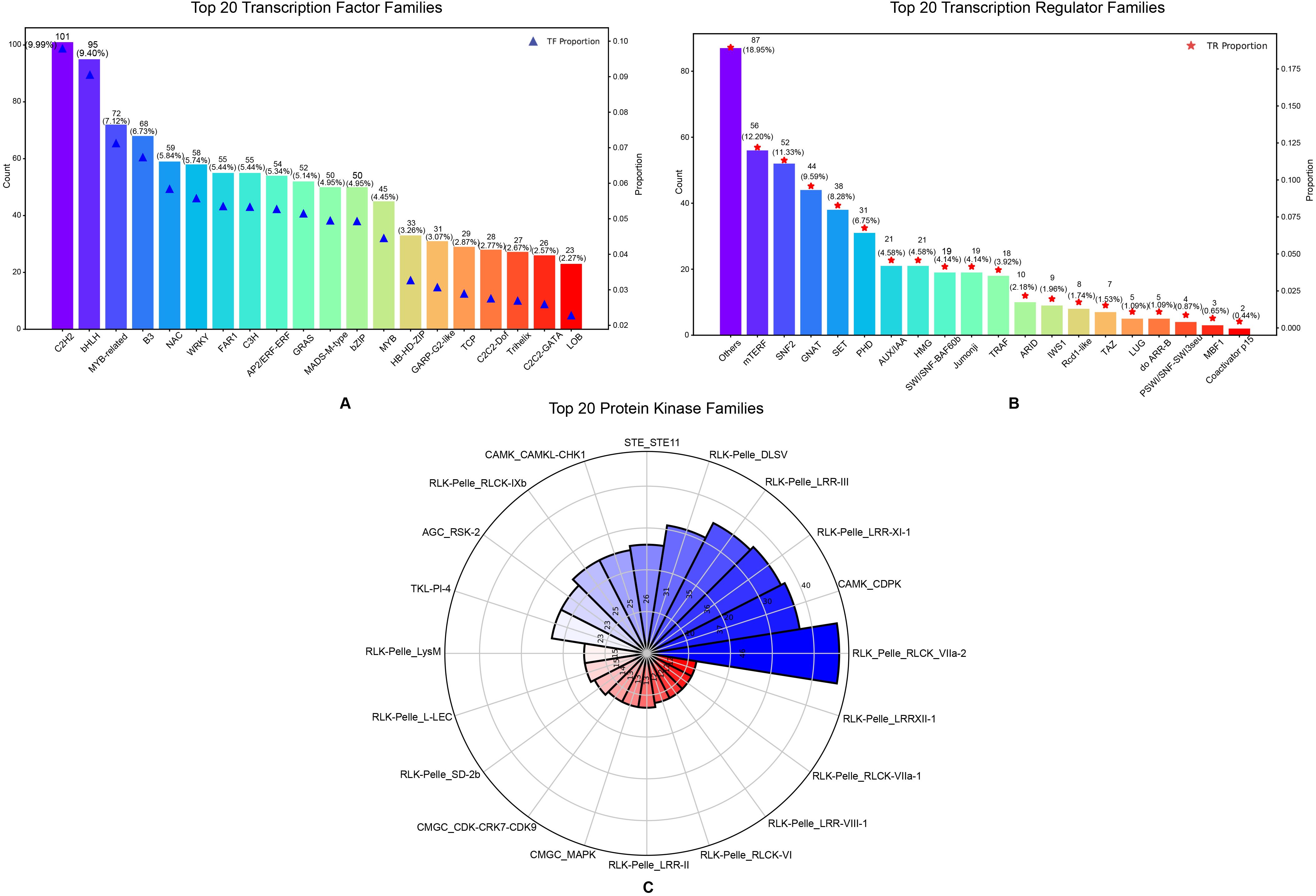
Figure 4. Top twenty TF families, TR families and PK of Gaultheria prostrata genome. (A) The bar chart illustrates the top 20 transcription factor families, classified by their counts and proportions. The x axis enumerates the various transcription factor families, while the left y-axis represents the count, and the right y-axis denotes the proportion. (B) The bar chart displays the top 20 transcription regulator families based on their counts and proportions. The x-axis lists the different transcription regulator families, while the y-axis on the left shows the count, and the y-axis on the right indicates the proportion. (C) The Nightingale Rose Plot (also known as a Radar Chart) illustrates the top 20 protein kinase families. Each sector represents a different protein kinase family, with the radius of the sector proportional to the number of members in that family. The intersection of the radial lines and the circle denotes the tick marks for the gene family count, with the number of each family also marked in the corresponding sector. (A–C) use color coding to distinguish between families.
Figure 4A shows the top 20 transcription factor families by quantity, with C2H2 being the most abundant (101 members, 9.99%), followed by bHLH (95 members, 9.40%) and MYB-related (72 members, 7.12%). Families like C2H2, B3, NAC, and WRKY are involved in environmental adaptation, while C2H2, bHLH, MYB, and others regulate growth and development (Puranik et al., 2012). Their functions span stress response, developmental regulation, and signal transduction. Figure 4B shows the distribution of the top 20 transcriptional regulator families, with “Others” comprising 87 genes (18.95%) and mTERF, SNF2, GNAT, and SET families following, ranging from 12.20% to 8.28% of the total. These regulators play key roles in gene expression and chromatin structure, where mTERF, SNF2, and HMG primarily control mitochondrial and nuclear gene regulation, while GNAT, SET, PHD, and Jumonji influence gene expression through histone modifications (Kouzarides, 2007). AUX/IAA proteins act as repressors in the auxin signaling pathway, regulating plant growth (Mockaitis and Estelle, 2008). Figure 4C shows the distribution of the top 20 protein kinase families, with RLK-Pelle_RLCK-VIIa-2 being the most abundant (46 members), followed by CAMK_CDPK (37 members) and RLK-Pelle_LRR-XI-1 (36 members). These families play key roles in plant signal transduction pathways, regulating growth, defense, and stress responses. Specifically, RLK-Pelle is involved in development and defense, CDPKs mediate stress through calcium signaling, and STE11 participates in the MAPK pathway for cell division and stress response (Gish and Clark, 2011; Schulz et al., 2013).
Gene prediction and annotation
After constructing the repetitive sequence library of G. prostrata by RepeatModeler, the G. prostrata-families.fa was identified by using ReapeatMasker, and the results showed that the repetitive sequences accounted for 45.04%, with a total length of 188,030,998 bp, of which the interspersed repeats accounted for 43.5%. Wherein, the proportion of LINE (long interspersed nuclear elements) (1.72%) and SINE (short interspersed repeated sequence) (0.75%) were lower than LTR (long terminal repeats) (11.71%) and DNA transposons (4.78%). Besides, in tandem repeat sequences, satellites accounted for 0.14%; low complexity (0.16%) and simple repeats accounted for 0.88% (Table 2). Secondly, an ab initio prediction wasmade using MAKER. A total of 599,422 genes were annotated by Augustus, 809,961 genes by SNAP, and 1,435,945 genes by Genemark. Then, homologous gene annotation was completed using GeMoMa and a total of 73,995 genes were annotated. Meanwhile, 25,020 transcriptome genes were predicted using TransDecoder. Finally, all predicted genes were integrated using EVidenceModeler and 34,909 annotated genes were obtained. Subsequently, functional gene annotation was performed using the results from EVidenceModeler integration.
A total of 26,497 protein-coding genes were predicted and annotated in Nr, Swissprot, GO, KEGG and Pfam databases using GFAP. Among these genes, 14,377 and 2,387 genes were functionally annotated on Nr and Swissprot, respectively; 21,895, 24,424 and 22,330 genes were functionally annotated on GO, KEGG and Pfam separately. (The integration annotation files for the five databases and the separate GO, KEGG, and Pfam databases are detailed in Supplementary Tables S7–S12).
The genes annotated in the GO database are categorized into three primary groups: cellular component processes (58.20%), molecular functions (25.40%), and biological processes (16.40%); these percentages indicate the proportion of all enriched genes within each category. Furthermore, within the cellular component processes, the most enriched genes were those associated with the plasma membrane (2,879 genes) and nucleus (2,682 genes); in the molecular function category, protein binding (3,076 genes) and ATP binding (2,485 genes) were predominant; and in the biological processes category, regulation of transcription, DNA-templated (1,894 genes) and defense response to nematodes (1,697 genes) were notably enriched (Figure 5). (Refer to Supplementary Tables S8, S9 for detailed functional information about specific genes.)
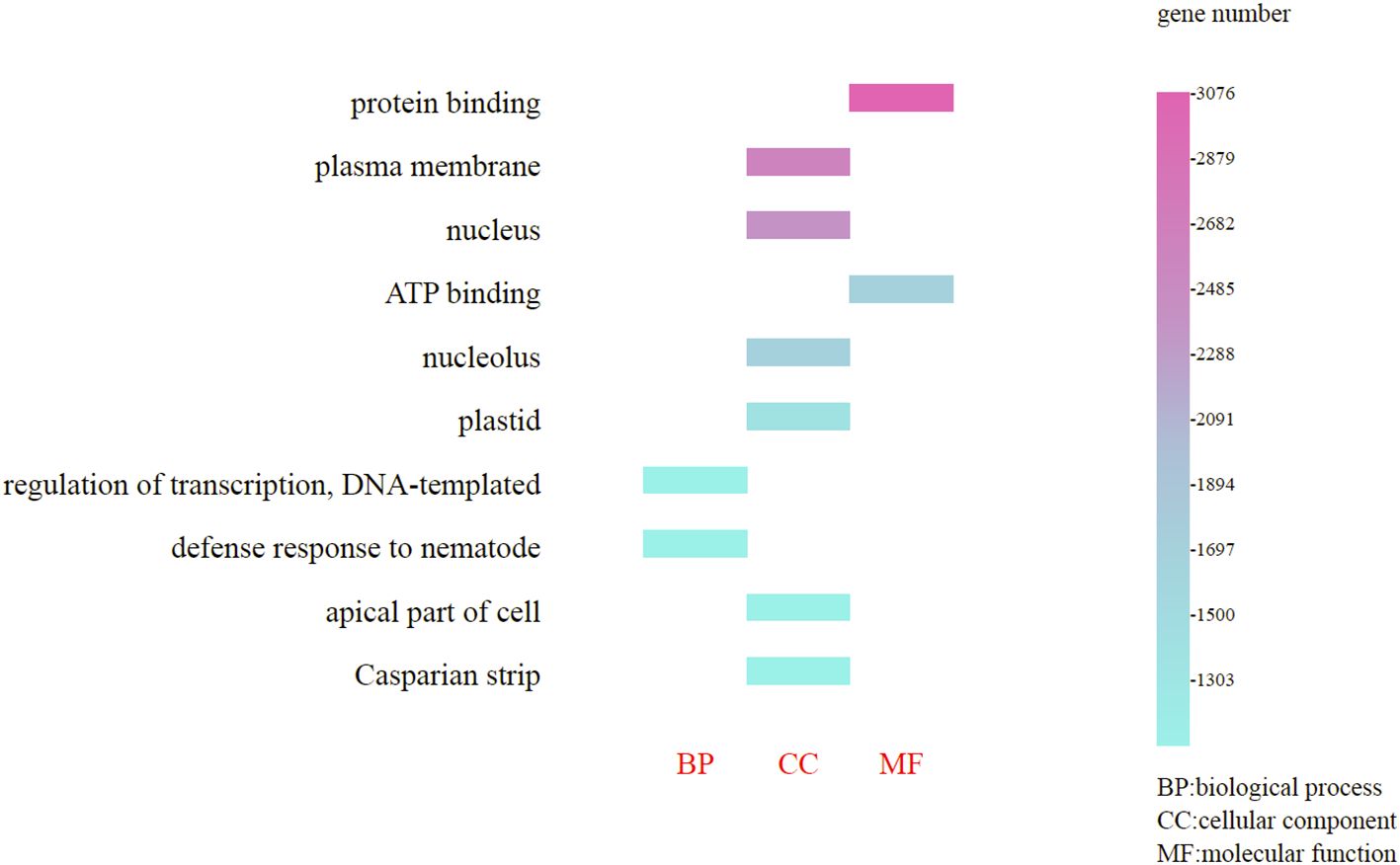
Figure 5. GO functional classification of the Gaultheria prostrata genome. The horizontal axis represents the three fundamental GO categories: biological Process (BP), cellular component (CC) and molecular function (MF); Captions on the left side of the chart correspond to specific terms for BP, CC, or MF; The vertical axis, located on the right, indicates the number of genes enriched for each term.
Moreover, the genes annotated in the KEGG database were divided into nine categories, predominantly featuring protein kinase genes associated with plant-pathogen interaction, plant hormone signal transduction and MAPK signaling pathway-plant. The largest group (16.6%) was associated with the MAPK signaling pathway, featuring protein kinases like FLS2, ERECTA, and BRI1. Plant-pathogen interaction (16.2%) included kinases such as PBS1 and BRI1, while hormone signal transduction (8.58%) involved BRI1-related kinases (Figure 6). (Refer to Supplementary Tables S10, S11 for detailed functional information about specific genes.)
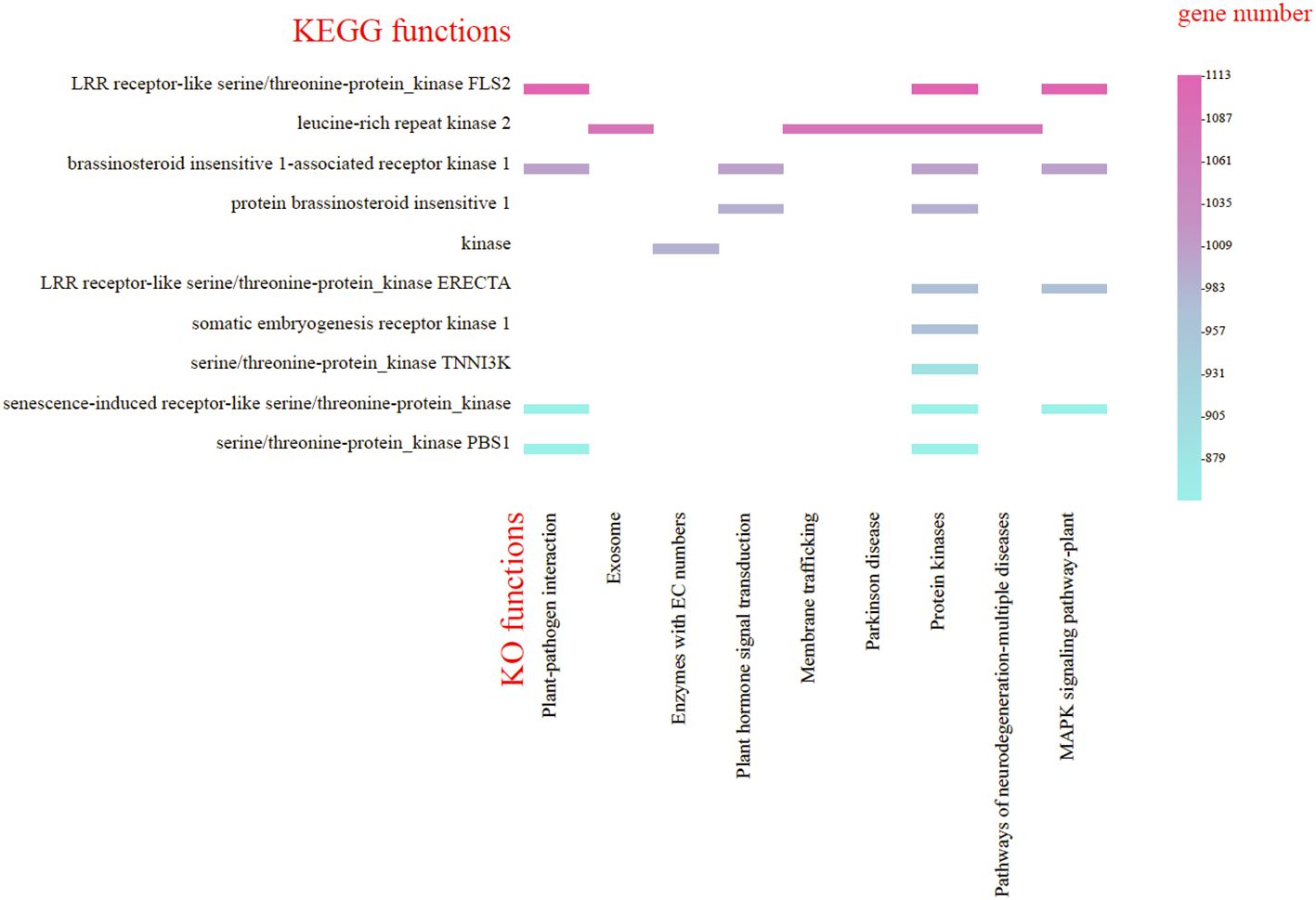
Figure 6. KEGG functional classification of the Gaultheria prostrata genome. The horizontal axis represents the highly enriched KO pathway terms; Captions on the left side of the chart to kinases involved in the KO pathway terms. The vertical axis, located on the right side of the chart, indicates the number of genes enriched for each term.
Phylogenomic analysis, divergence time estimation and expansion and contraction analysis
We conducted phylogenomic and molecular dating analyses based on 12 single-copy genes derived from the genomes of G. prostrata, six species from the order Ericales (A. chinensis, R. williamsianum, V. corymbosum, A. corniculatum, D. kaki, C. sinensis), one species from the family Brassicaceae (A. thaliana), and one species from the family Poaceae (O. sativa). G. prostrata was assigned to the subfamily Vaccinioideae within the family Ericaceae, with a strongly supported topology [((Vcor,Gpro)100,Rwil)] (Supplementary Figure S2).
Based on our selected species, the crown age of the order Ericales is estimated to be approximately 102.87 Ma (with a 95% highest posterior density (HPD) interval of 94.91–111.35 Ma) (Figure 7). This estimate is supported by molecular clock calibration studies from Bell et al. (2010); Herting et al. (2023), and Magallón and Castillo (2009), and aligns with the diversification events of other angiosperms (Magallón and Castillo, 2009; Bell et al., 2010; Herting et al., 2023). The families Primulaceae and Ebenaceae originated in the Late Cretaceous (Late Mesozoic), with the divergence time of Ebenaceae estimated at ∼92.38 Ma (95% HPD interval: 85.76–99.91 Ma). According to our species sampling, the stem age of the family Ericaceae dates back to ∼49.47 Ma (95% HPD interval: 42.84–59.06 Ma), while the divergence time between V. corymbosum and G. prostrata in the subfamily Vaccinioideae is approximately 21.48 Ma (95% HPD interval: 8.85–35.47 Ma) (Figure 7; Supplementary Figure S3).
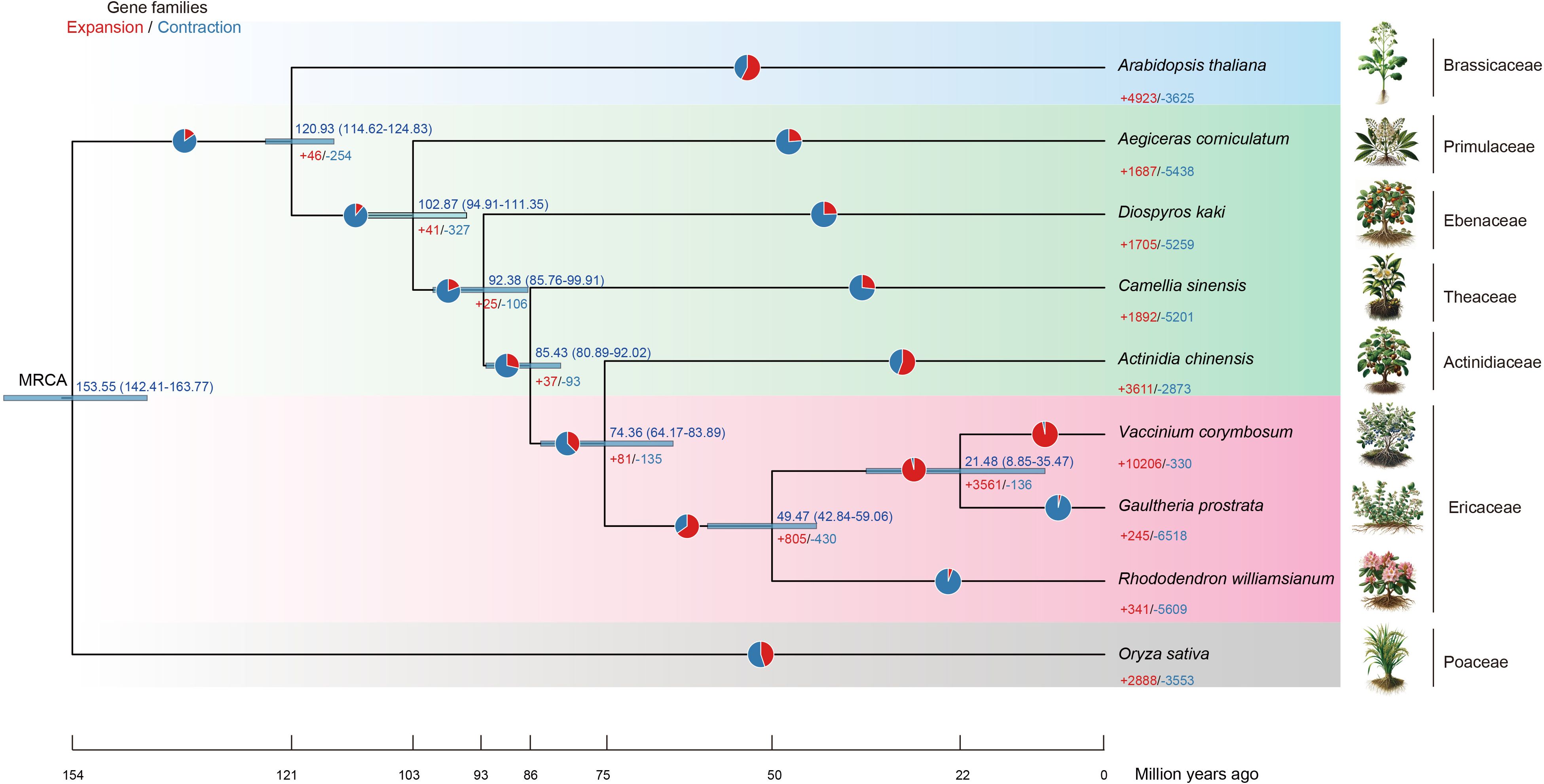
Figure 7. The time-calibrated phylogenetic tree for Ericales. The chronogram illustrates the divergence times within the order Ericales, encompassing families such as Primulaceae, Ebenaceae, Theaceae, Actinidiaceae, and Ericaceae. The species photos were drawn by DALL-E 2 (https://openai.com/index/dall-e-2/). The pie chart represents the proportion of expansion/contraction genes.
This phylogenetic tree illustrates gene families of expansion and contraction within the Ericales, focusing on G. prostrata. It shows a substantial contraction gene (-6,518 genes) with minimal expansion (+245 genes), suggesting significant gene loss or streamlining, possibly due to specific environmental adaptations. In contrast, V. corymbosum exhibits a large gene expansion (+10,266), indicating different evolutionary pressures. The broader Ericales lineage, including R. williamsianum, also shows notable gene contraction (-5,609), reflecting diverse evolutionary strategies across the order (Figure 7).
The pathways related gene expansion in G. prostrata, such as Protein phosphatases and associated proteins and MAPK signaling pathway (Supplementary Table S13), indeed play significant roles in cell signaling, pathogen defense, and substance transport, which are essential for adapting to extreme environments like high altitudes. Expansion genes support processes such as xenobiotic transmembrane transport, responses to organonitrogen compound and hormone and auxin transport (Supplementary Table S14), which contributing to adaptation to harsh conditions like low oxygen and high UV radiation. Conversely, significant gene contraction reflects an adaptive strategy to conserve resources by reducing reliance on non-essential metabolic pathways, such as Cytochrome P450, secondary metabolite synthesis, and cellular responses to light intensity (Supplementary Tables S15, S16). This reduction streamlines metabolic processes, improving efficiency and adaptation to the resource-limited and harsh conditions typical of high-altitude environments.
Ka/Ks positive selection analysis
The positively selected orthologous gene pairs of G. prostrata and V. corymbosum identified through positive selection analysis have similar functions, so only the positively selected genes for G. prostrata are discussed here as representatives. As shown in Figure 8A and Supplementary Table S17, the significantly enriched positive selection genes related to Antigen processing and presentation are prevalent or active in G. prostrata. This could suggest an adaptive immune or stress response mechanism tailored to high-altitude environments where these plants encounter unique pathogens or extreme stresses. In Figure 8B and Supplementary Table S18, pathways related to positive regulation of signaling, including osmotic stress response and signal transduction, are enriched. These enriched pathways reflect strong signaling capabilities in both species, underscoring their ability to maintain cellular functions and growth while adapting to the harsh, variable conditions of high-altitude environments. This highlights their robust stress response and signaling mechanisms, crucial for survival under extreme environmental pressures.
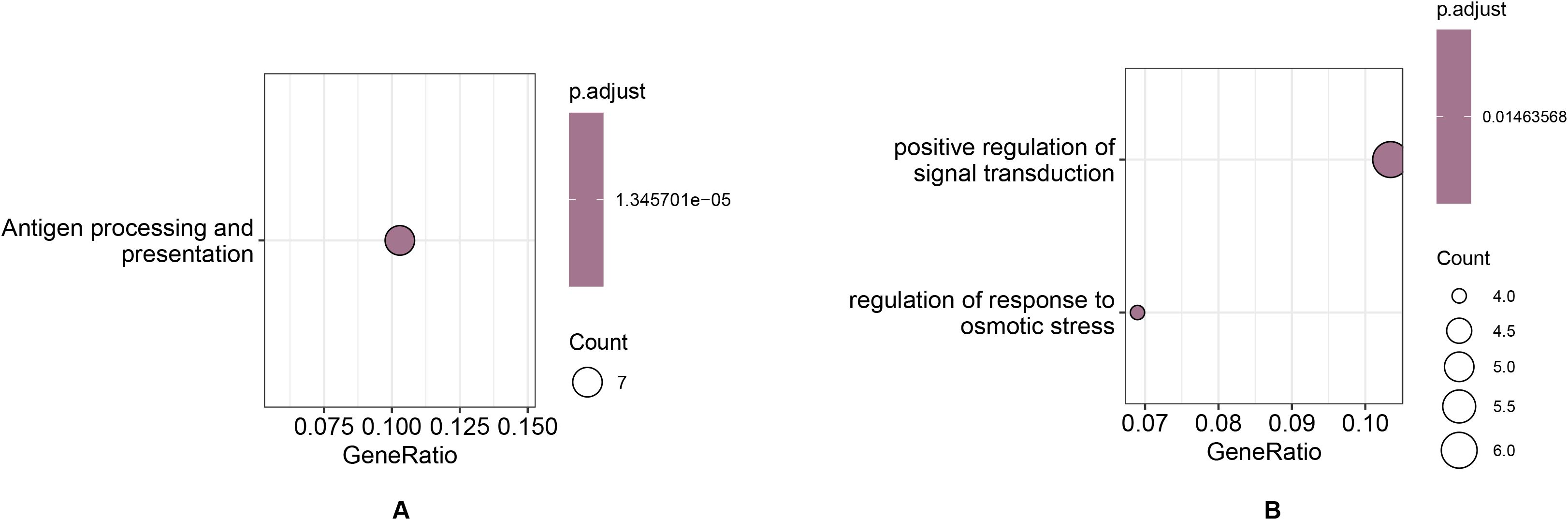
Figure 8. Functional enrichment of positive selection genes in Gaultheria prostrata related to (A) Antigen processing and presentation and (B) Positive regulation of signal transduction and Response to osmotic stress, highlighting enhanced immune and stress response mechanisms adapted to high-altitude environments.
Discussion
Woody plants are crucial for ecological protection in high-altitude habitats. However, genomic research on these plants is relatively scarce (Saikia et al., 2017). In this study, a genome sequencing draft was assembled using next-generation high-throughput sequencing for the woody plant G. prostrata genome, aiming to obtain accurate short read data, which will serve as an important reference for obtaining further third-generation data. Owing to its high accuracy and low error rate, this data has advantages in error correction and calibration of third-generation sequencing data. In-depth genomic research on G. prostrata aims to discover genes with specific functions and adaptability laying the foundation for analyzing the genetic evolutionary patterns of Gaultheria species and providing support for the conservation of genetic diversity, ecological adaptation, and functional trait evolution research of this species.
Flow cytometry is a standard method for rapid C-value acquisition and genome size prediction (Zhu et al., 2012). k-mer analysis of genome sequencing data is also used to assess characteristics such as size, heterozygosity, repetitive sequences and GC content (Zhou et al., 2023). Given the rapid advancement of NGS technology, this study integrates flow cytometry and k-mer analysis to enhance genome size prediction reliability. The genome size of G. prostrata, as determined by k-mer deep analysis (447Mb), aligns closely with the results from flow cytometry (440 Mb). Furthermore, the draft genome size for the initial assembly (410 Mb) correlates well with these predictions, thereby validating their accuracy. Numerous studies have concurrently assessed genome size using above three methods, highlighting their widespread use and significance (Pflug et al., 2020; Pfenninger et al., 2022). Besides, flow cytometry analysis reveals that the genome size of G. prostrata is nearly half of the tetraploid species G. crenulata and G. griffithiana, indicating that G. prostrata is likely a diploid species. On this basis, we conducted Smudgeplot analysis, which also further supported that G. prostrata is an AB diploid rather than a polyploid. Furtherly, cytological studies should be conducted in the future to confirm the chromosome number and verify the ploidy type. In general, the genus Gaultheria has both polyploid and diploid (Middleton’s literature suggests that the base chromosome number of the Gaultheria Kalm ex L. (Ericaceae) taxon is x=11). In the future, an in-depth exploration of the causes of polyploidy formation or diploidy maintenance in different species can be conducted based on this diploid framework.
Additionally, genome assembly quality is significantly influenced by factors such as heterozygosity and repetition rate (Bi et al., 2019). Assembly becomes challenging when heterozygosity exceeds 0.5% – 1% (Marcais and Kingsford, 2011), but G. prostrata’s heterozygosity is 0.159%, indicating simplicity. With 34.6% repetitive sequences, below the 50% threshold for complexity, G. prostrata has a low complexity genome (Sun et al., 2023). In addition, GC content distribution is important for assessing the quality of genome sequencing and the complexity of genome assembly (Singh et al., 2016). By analyzing the GC-depth distribution plot, we can determine if there’s a notable GC bias in the sequencing data (Benjamini and Speed, 2011) and identify potential bacterial contamination (Parras-Molto et al., 2018). Not only that, GC content, crucial for successful assembly, is ideal between 25%–65% (Aird et al., 2011). G. prostrata’s GC content of 38.85% is within this range, comparable to Rosa roxburghii (38.5%) and Helianthus annuus (38.9%) ( (Bi et al., 2019; Shan et al., 2020). It could be seen that the GC content of G. prostrata is at a moderate level, which has been demonstrated to be suitable for assembly. At the same time, the scaffold N50 lengths of it exceeded 30 kb (Nagarajan and Pop, 2013), and the genome completeness estimated by BUSCO using the embryophyta_odb10 dataset is 90%, indicating that the G. prostrata genome is a high-quality assembly result.
Significantly, genome size, GC content, and repetitive sequences influence genome complexity, assembly, and species adaptability. Genome size is influenced by a variety of biological factors, including the cell cycle, cell dimensions, and stress tolerance (Wan et al., 2021). In addition, in some cases, there is a correlation between genome size and plant body size (Leitch and Bennett, 2004). In some taxa, genome size correlates with body size, aiding in faster cell growth in limited resources (Greilhuber and Leitch, 2012). However, in other plants, this correlation does not hold. G. prostrata’s genome (410 Mb) is smaller than related Rhododendron species (~ 560 - 650 Mb) (Zhang et al., 2017; Soza et al., 2019; Yuan et al., 2019) and moderate among high-altitude plants (Yang et al., 2013), potentially reducing evolutionary costs (Cobo-Simon and Tamames, 2017). Besides, reduced leaf area and height help minimize heat loss and provide cold protection. Meanwhile, genome size is positively correlated with GC content and higher GC content generally confers greater thermostability and flexibility to DNA (Vinogradov, 2003). G. prostrata’s higher GC content compared to A. thaliana (Wright et al., 2002) improves DNA stability and reduces DNA curvature, aiding in high-altitude adaptation (Vinogradov, 2003). In G. prostrata, moderate GC content [compared with R. indicum (39%) and R. micranthum (40.4%) (Yuan et al., 2019; Zhou et al., 2020)] likely balances sufficient chromatin openness with the necessary flexibility for effective gene expression, thereby ensuring the stability and efficiency of physiological and metabolic processes. Moreover, repetitive sequences play a significant role in the regulation of structural genes and the control of recombination processes (Shi et al., 2016a). They enhance genomic diversity and adaptability by triggering gene recombination, mutation, and new gene formation (Shi et al., 2018). From another perspective, our study revealed that significant variations in the frequencies of various types of SSR repeats, with higher frequencies of di-, mono-, and trinucleotide repeats in G. prostrata. This result parallels findings in most plants (Shan et al., 2020; Reinar et al., 2021). The high proportion of dinucleotide SSRs in plant genomes, particularly AG/CT motifs, suggests their crucial role in genome stability, evolution, and as markers for genetic diversity due to their high mutation rates and polymorphism (Niyitanga et al., 2022). In summary, the abundance of SSRs in G. prostrata likely enhances its genetic diversity, supporting a wide array of physiological adaptations. This diversity may facilitate rapid evolution of traits, such as improved photosynthetic efficiency to withstand high UV exposure or enhanced antifreeze proteins for cold resistance (Selkoe and Toonen, 2006; Yocca et al., 2023). which is a key factor in its successful colonization and reproduction in diverse and extreme alpine environments. Overall, the unique genomic characteristics of G. prostrata, including its optimized genome size, moderate GC content, and abundant SSRs, enable it to thrive under the severe and fluctuating conditions of high-altitude environments.
Furthermore, among the TFs, TRs, and PKs we identified, the top ten genes in each category are involved in regulating environmental adaptation, responding to biotic and abiotic stresses (Zhu, 2016), and mediating light signal transduction (Chen et al., 2004). This regulation can promote the loosening of chromatin in specific gene regions, making these genes more easily transcribed (Li et al., 2007). By regulating gene expression, plants can respond quickly to environmental changes, thereby helping G. prostrata better adapt to high-altitude habitats. Then, our study identified 26,497 protein-coding genes focused on environmental stress response, notably in areas like plant-pathogen interaction and hormone signal transduction, using GFAP across multiple databases. These genes, active in transcription regulation and defense, suggest robust immune mechanisms evolved against microbial threats. These genes, active in transcription regulation and defense, suggest robust immune mechanisms evolved against microbial threats. It is noteworthy that G. prostrata exhibits a rich composition of methyl salicylate, which is also involved in stress resistance (Liu et al., 2011). Future research could explore genes regulating methyl salicylate biosynthesis in stress responses. Moreover, the MAPK signaling pathway is a critical component of the plant response to external stressors, including pathogens and abiotic stress (Cristina et al., 2010). It plays a crucial role in some plant’s response to the fluctuating environmental conditions typically found at high altitudes. The MAPK pathway is involved in regulating cellular responses to temperature fluctuations, UV radiation, and other abiotic stresses, thereby enhancing the plants’ survival and growth in these challenging environments (Liu et al., 2022; Kumar et al., 2023; Joshi et al., 2024). While the transcription regulation genes, especially those that are DNA-templated, play a central role in orchestrating a diverse array of stress responses (Jiang et al., 2017).
Lu et al.’s research indicates that the origin of Gaultheria Kalm ex L. (Ericaceae) dates back to approximately 20–25 million years ago (Lu et al., 2019), which coincides with our estimated origin for G. prostrata at 21.48 million years. This period is belong to the early Miocene epoch, when the Earth experienced significant climatic changes, including initial warming followed by cooling; the expansion of grasslands driven by increased aridity; major tectonic activities, such as the uplift of the Himalayas; and considerable biological diversification among plants and mammals (Boyer, 2023; Britannica, T.E.o.E, 2023; Paleontology, UCMP, 2023). These changes may have created new ecological opportunities or adaptive pressures for the Gaultheria plants.
The identified gene of expansion, contraction, and positive selection in G. prostrata highlight its adaptation to high-altitude environments. Gene contraction, particularly in secondary metabolite synthesis and light response pathways, suggests a streamlined metabolism for survival in resource-limited conditions, while the expansion of genes related to the MAPK signaling pathway and transmembrane transport enhances its stress response capabilities. In contrast, V. corymbosum shows substantial gene expansion, indicating different evolutionary pressures. Additionally, positive selection of genes involved in immune and osmotic stress responses further supports G. prostrata’s adaptation to extreme environments. Understanding how these genes and pathways help high-altitude plants adapt to harsh environments can provide potential applications for agriculture and ecological restoration (Schranz et al., 2012). For example, these genes can be targeted by gene editing or breeding programs to develop crops that are more resistant and environmentally adaptable (Chen et al., 2019a). This is of great significance for responding to climate change and improving crop tolerance and stability, especially for applications in agricultural marginal areas or extreme environments (Bailey-Serres et al., 2019). Although we have identified significantly enriched pathways, further functional validation and experimental studies are needed to reveal the specific roles of these genes in high altitude acclimatization. Future studies could focus on the experimental validation of the function of the genes, environmental simulation experiments, and how these genes are dynamically regulated under different environmental stresses (Burtscher et al., 2022). In addition, the synergistic effects of gene networks and multiple pathways can be explored to fully understand the complex regulatory mechanisms of G. prostrata in response to environmental changes.
In conclusion, future studies should integrate second- and third-generation sequencing for the complete genome sequencing of G. prostrata. Employing Hi-C technology will aid in understanding its chromosome organization and regulatory mechanisms, essential for utilizing its germplasm resources. T2T (Telomere-to-Telomere) technology can reveal genetic details missed by traditional sequencing, especially in complex region. Third-generation genome data will enhance comparisons and insights into Gaultheria’s genetic and evolutionary traits. In the future, integrating phylogenomic and environmental data will help reconstruct the evolutionary history of Gaultheria and reveal key biogeographic and adaptive patterns. Simultaneously, future research could also investigate those metabolites that are related to the high-altitude adaptability of G. prostrata, aim to identify characteristic metabolites, and determine whether there are some repetitive sequences or other genomic structures that respond adaptively to environmental stress.
Data availability statement
The datasets presented in this study can be found in online repositories. The names of the repository/repositories and accession number(s) can be found below: https://www.ncbi.nlm.nih.gov/, PRJNA1042922.
Author contributions
Y-JL: Writing – review & editing, Writing – original draft, Visualization, Software, Methodology, Investigation, Formal analysis, Data curation, Conceptualization. X-YD: Writing – original draft, Formal analysis, Data curation. Y-WH: Writing – original draft, Software, Formal analysis. LL: Writing – review & editing, Methodology, Funding acquisition, Conceptualization.
Funding
The author(s) declare financial support was received for the research, authorship, and/or publication of this article. This research was supported by the National Natural Science Foundation of China (31960080), Yunnan Revitalization Talent Support Program (YNWR-QNBJ-2019-172) and Reserve talents of young and middle-aged academic and technical leaders in Yunnan Province (202005AC160020).
Acknowledgments
We are grateful to Yanxia Jia, Chunxia Zeng, Jing Yang and Jixiong Yang from Kunming Institute of Botany, Chinese Academy of Sciences, and Dong Xu from Shenzhen Branch, Guangdong Laboratory of Lingnan Modern Agriculture, Genome Analysis Laboratory of the Ministry of Agriculture and Rural Affairs, Agricultural Genomics Institute at Shenzhen, Chinese Academy of Agricultural Sciences, for their kind guidance on this project. We express our gratitude to DALL-E 2 for its assistance in illustrating the species photos in the phylogenetic tree for this study.
Conflict of interest
The authors declare that the research was conducted in the absence of any commercial or financial relationships that could be construed as a potential conflict of interest.
Publisher’s note
All claims expressed in this article are solely those of the authors and do not necessarily represent those of their affiliated organizations, or those of the publisher, the editors and the reviewers. Any product that may be evaluated in this article, or claim that may be made by its manufacturer, is not guaranteed or endorsed by the publisher.
Supplementary material
The Supplementary Material for this article can be found online at: https://www.frontiersin.org/articles/10.3389/fpls.2024.1456102/full#supplementary-material
References
Aird, D., Ross, M. G., Chen, W. S., Danielsson, M., Fennell, T., Russ, C., et al. (2011). Analyzing and minimizing PCR amplification bias in Illumina sequencing libraries. Genome Biol. 12, R18. doi: 10.1186/gb-2011-12-2-r18
Altschul, S. F., Gish, W., Miller, W., Myers, E. W., Lipman, D. J. (1990). Basic local alignment search tool. J. Mol. Biol. 215, 403–410. doi: 10.1016/S0022-2836(05)80360-2
Bailey-Serres, J., Parker, J. E., Ainsworth, E. A., Oldroyd, G. E., Schroeder, J. I. (2019). Genetic strategies for improving crop yields. Nature 575, 109–118. doi: 10.1038/s41586-019-1679-0
Beier, S., Thiel, T., Munch, T., Scholz, U., Mascher, M. (2017). MISA-web: a web server for microsatellite prediction. Bioinformatics 33, 2583–2585. doi: 10.1093/bioinformatics/btx198
Bell, C. D., Soltis, D. E., Soltis, P. S. (2010). The age and diversification of the angiosperms re-revisited. Am. J. Bot. 97, 1296–1303. doi: 10.3732/ajb.0900346
Benjamini, Y., Speed, T. (2011). Estimation and correction for GC-content bias in high throughput sequencing. Technical Report No. 804, Department of Statistics, University of California, Berkeley. Available online at: https://statistics.berkeley.edu/tech-reports/804 (Accessed June 24, 2024).
Bi, Q., Zhao, Y., Cui, Y., Wang, L. (2019). Genome survey sequencing and genetic background characterization of yellow horn based on next-generation sequencing. Mol. Biol. Rep. 46, 4303–4312. doi: 10.1007/s11033-019-04884-7
BiologyInsights (2024). Adaptations and Ecological Roles of the Ericaceae Family. Available online at: https://biologyinsights.com/adaptations-and-ecological-roles-of-the-ericaceae-family/ (Accessed September 12, 2024).
Bourge, M., Brown, S. C., Siljak-Yakovlev, S. (2018). Flow cytometry as tool in plant sciences, with emphasis on genome size and ploidy level assessment. Genet. Appl. 2, 1–12. doi: 10.31383/ga.vol2iss2pp1-12
Boyer, J. (2023). Miocene Epoch (PaleoPlant). Available online at: https://www.paleoplant.org/geologic/phanerozoic/cenozoic/neogene/miocene (Accessed June 24, 2024).
Britannica, T.E.o.E (2023). Miocene Epoch (Encyclopaedia Britannica, Inc). Available online at: https://www.britannica.com/science/Miocene-Epoch (Accessed June 24, 2024).
Burtscher, M., Millet, G. P., Burtscher, J. (2022). Hypoxia conditioning for high-altitude pre-acclimatization. J. Sci. Sport Exercise 4, 331–345. doi: 10.1007/s42978-021-00150-0
Cantarel, B. L., Korf, I., Robb, S. M., Parra, G., Ross, E., Moore, B., et al. (2008). MAKER: an easy-to-use annotation pipeline designed for emerging model organism genomes. Genome Res. 18, 188–196. doi: 10.1101/gr.6743907
Chen, K., Wang, Y., Zhang, R., Zhang, H., Gao, C. (2019a). CRISPR/Cas genome editing and precision plant breeding in agriculture. Annu. Rev. Plant Biol. 70, 667–697. doi: 10.1146/annurev-arplant-050718-100049
Chen, L., Wang, W. S., Wang, T., Meng, X. F., Chen, T. T., Huang, X. X., et al. (2019b). Methyl salicylate glucosylation regulates plant defense signaling and systemic acquired resistance. Plant Physiol. 180, 2167–2181. doi: 10.1104/pp.19.00091
Chen, M., Chory, J., Fankhauser, C. (2004). Light signal transduction in higher plants. Annu. Rev. Genet. 38, 87–117. doi: 10.1146/annurev.genet.38.072902.092259
Chen, Y., Chen, Y., Shi, C., Huang, Z., Zhang, Y., Li, S., et al. (2018). SOAPnuke: a MapReduce acceleration-supported software for integrated quality control and preprocessing of high-throughput sequencing data. Gigascience 7, 1–6. doi: 10.1093/gigascience/gix120
Cheng, X. J., Fritsch, P. W., Lin, Y. J., Li, G. H., Chen, Y. Q., Zhang, M. Y., et al. (2024). The role of Pleistocene dispersal in shaping species richness of sky island wintergreens from the Himalaya-Hengduan Mountains. Mol. Phylogenet Evol. 197, 108082. doi: 10.1016/j.ympev.2024.108082
Choudhary, S., Thakur, S., Majeed, A., Bhardwaj, P. (2021). Adaptability of Rhododendrons in high altitude habitats. J. Forestry Res. 32, 449–460. doi: 10.1007/s11676-019-01081-x
Cobo-Simon, M., Tamames, J. (2017). Relating genomic characteristics to environmental preferences and ubiquity in different microbial taxa. BMC Genomics 18, 499. doi: 10.1186/s12864-017-3888-y
Couto, D., Zipfel, C. (2016). Regulation of pattern recognition receptor signalling in plants. Nat. Rev. Immunol. 16, 537–552. doi: 10.1038/nri.2016.77
Cristina, M. S., Petersen, M., Mundy, J. (2010). Mitogen-activated protein kinase signaling in plants. Annu. Rev. Plant Biol. 61, 621–649. doi: 10.1146/annurev-arplant-042809-112252
Cui, F., Ye, X., Li, X., Yang, Y., Hu, Z., Overmyer, K., et al. (2022). Chromosome-level genome assembly of the diploid blueberry Vaccinium darrowii provides insights into its subtropical adaptation and cuticle synthesis. Plant Commun. 3, 100307. doi: 10.1016/j.xplc.2022.100307
Darriba, D., Taboada, G. L., Doallo, R., Posada, D. (2011). ProtTest 3: fast selection of best-fit models of protein evolution. Bioinformatics 27, 1164–1165. doi: 10.1093/bioinformatics/btr088
Ding, H., Chen, W., Li, J., Fu, F., Li, Y., Xiao, S. (2023a). Physiological characteristics and cold resistance of five woody plants in treeline ecotone of sygera mountains. Sustainability 15, 3040. doi: 10.3390/su15043040
Ding, H., Zhang, H., Liu, B., Huang, H. (2023b). Study on mechanical properties of soil stabilization by different vegetation roots on high steep slope. Sustainability 15, 2569. doi: 10.3390/su15032569
Edgar, R. C. (2004). MUSCLE: multiple sequence alignment with high accuracy and high throughput. Nucleic Acids Res. 32, 1792–1797. doi: 10.1093/nar/gkh340
Emms, D. M., Kelly, S. (2015). OrthoFinder: solving fundamental biases in whole genome comparisons dramatically improves orthogroup inference accuracy. Genome Biol. 16, 157. doi: 10.1186/s13059-015-0721-2
Gish, L. A., Clark, S. E. (2011). The RLK/Pelle family of kinases. Plant J. 66, 117–127. doi: 10.1111/j.1365-313X.2011.04518.x
Greilhuber, J., Leitch, I. J. (2012). “Genome size and the phenotype,” in Plant genome diversity volume 2: physical structure, behaviour and evolution of plant genomes (Vienna, Austria: Springer), 323–344.
Herting, J., Schonenberger, J., Sauquet, H. (2023). Profile of a flower: How rates of morphological evolution drive floral diversification in Ericales and angiosperms. Am. J. Bot. 110, e16213. doi: 10.1002/ajb2.16213
Honaas, L., Kahn, E. (2017). A practical examination of RNA isolation methods for European pear (Pyrus communis). BMC Res. Notes 10, 1–8. doi: 10.1186/s13104-017-2564-2
Jiang, J., Ma, S., Ye, N., Jiang, M., Cao, J., Zhang, J. (2017). WRKY transcription factors in plant responses to stresses. J. Integr. Plant Biol. 59, 86–101. doi: 10.1111/jipb.12513
Joshi, S., Nath, J., Joshi, R. (2024). Genome-wide identification, characterization, and expression analysis of the MAPK gene family in Nardostachys jatamansi (D. Don) DC. In Vitro Cell. Dev. Biology-Plant 60, 222–237. doi: 10.1007/s11627-024-10430-3
Keilwagen, J., Hartung, F., Paulini, M., Twardziok, S. O., Grau, J. (2018). Combining RNA-seq data and homology-based gene prediction for plants, animals and fungi. BMC Bioinf. 19, 189. doi: 10.1186/s12859-018-2203-5
Kokot, M., Dlugosz, M., Deorowicz, S. (2017). KMC 3: counting and manipulating k-mer statistics. Bioinformatics 33, 2759–2761. doi: 10.1093/bioinformatics/btx304
Kouzarides, T. (2007). Chromatin modifications and their function. Cell 128, 693–705. doi: 10.1016/j.cell.2007.02.005
Ksepka, D. T., Parham, J. F., Allman, J. F., Benton, M. J., Carrano, M. T., Cranston, K. A., et al. (2015). The fossil calibration database-A new resource for divergence dating. Syst. Biol. 64, 853–859. doi: 10.1093/sysbio/syv025
Kumar, V., Kumar, P., Bhargava, B., Sharma, R., Irfan, M., Chandora, R. (2023). Transcriptomic and metabolomic reprogramming to explore the high-altitude adaptation of medicinal plants: a review. J. Plant Growth Regul. 42, 7315–7329. doi: 10.1007/s00344-023-11018-8
Kumar, S., Stecher, G., Suleski, M., Hedges, S. B. (2017). TimeTree: A resource for timelines, timetrees, and divergence times. Mol. Biol. Evol. 34, 1812–1819. doi: 10.1093/molbev/msx116
Leitch, I., Bennett, M. (2004). Genome downsizing in polyploid plants. Biol. J. Linn. Soc. 82, 651–663. doi: 10.1111/j.1095-8312.2004.00349.x
Li, B., Carey, M., Workman, J. L. (2007). The role of chromatin during transcription. Cell 128, 707–719. doi: 10.1016/j.cell.2007.01.015
Li, J., Yin, Y., Wang, L., Liang, P., Li, M., Liu, X., et al. (2016). Synthesis, characterization, and anti-inflammatory activities of methyl salicylate derivatives bearing piperazine moiety. Molecules 21, 1544. doi: 10.3390/molecules21111544
Liu, P.-P., von Dahl, C. C., Park, S.-W., Klessig, D. F. (2011). Interconnection between methyl salicylate and lipid-based long-distance signaling during the development of systemic acquired resistance in Arabidopsis and tobacco. Plant Physiol. 155, 1762–1768. doi: 10.1104/pp.110.171694
Liu, W. R., Qiao, W. L., Liu, Z. Z., Wang, X. H., Jiang, R., Li, S. Y., et al. (2013). Gaultheria: Phytochemical and pharmacological characteristics. Molecules 18, 12071–12108. doi: 10.3390/molecules181012071
Liu, X. W., Wang, Y. H., Shen, S. K. (2022). Transcriptomic and metabolomic analyses reveal the altitude adaptability and evolution of different-colored flowers in alpine Rhododendron species. Tree Physiol. 42, 1100–1113. doi: 10.1093/treephys/tpab160
Lomsadze, A., Ter-Hovhannisyan, V., Chernoff, Y. O., Borodovsky, M. (2005). Gene identification in novel eukaryotic genomes by self-training algorithm. Nucleic Acids Res. 33, 6494–6506. doi: 10.1093/nar/gki937
Lu, L., Fritsch, P. W., Matzke, N. J., Wang, H., Kron, K. A., Li, D. Z., et al. (2019). Why is fruit colour so variable? Phylogenetic analyses reveal relationships between fruit-colour evolution, biogeography and diversification. Global Ecol. Biogeography 28, 891–903. doi: 10.1111/geb.12900
Luo, R., Liu, B., Xie, Y., Li, Z., Huang, W., Yuan, J., et al. (2012). SOAPdenovo2: an empirically improved memory-efficient short-read de novo assembler. Gigascience 1, 18. doi: 10.1186/2047-217X-1-18
Magallón, S., Castillo, A. (2009). Angiosperm diversification through time. Am. J. Bot. 96, 349–365. doi: 10.3732/ajb.0800060
Marcais, G., Kingsford, C. (2011). A fast, lock-free approach for efficient parallel counting of occurrences of k-mers. Bioinformatics 27, 764–770. doi: 10.1093/bioinformatics/btr011
Mendes, F. K., Vanderpool, D., Fulton, B., Hahn, M. W. (2020). CAFE 5 models variation in evolutionary rates among gene families. Bioinformatics 36, 5516–5518. doi: 10.1093/bioinformatics/btaa1022
Middleton, D., Wilcock, C. (1990). Chromosome counts in Gaultheria and related genera. Edinburgh J. Bot. 47, 303–313. doi: 10.1017/S0960428600003450
Mockaitis, K., Estelle, M. (2008). Auxin receptors and plant development: a new signaling paradigm. Annu. Rev. Cell Dev. Biol. 24, 55–80. doi: 10.1146/annurev.cellbio.23.090506.123214
Nagarajan, N., Pop, M. (2013). Sequence assembly demystified. Nat. Rev. Genet. 14, 157–167. doi: 10.1038/nrg3367
Nie, Z., Wen, J., Gu, Z., Boufford, D. E., Sun, H. (2005). Polyploidy in the flora of the Hengduan Mountains hotspot, southwestern China. Ann. Missouri Botanical Garden 92, 275–306. Available online at: http://www.jstor.org/stable/3298519.
Niyitanga, S., Yao, J., Ibrahim, A. K., Afzal, M. Z., Chen, S., Zhang, L. (2022). Development of genome-wide SSR markers for physical map construction with PCR-based polymorphic SSRs in Jute (Corchorus spp.). Trop. Plant Biol. 15, 65–77. doi: 10.1007/s12042-021-09301-7
Paleontology, UCMP (2023). The Miocene Epoch (Berkeley: UCMP). Available online at: https://ucmp.berkeley.edu/tertiary/miocene.php (Accessed June 24, 2024).
Parras-Molto, M., Rodriguez-Galet, A., Suarez-Rodriguez, P., Lopez-Bueno, A. (2018). Evaluation of bias induced by viral enrichment and random amplification protocols in metagenomic surveys of saliva DNA viruses. Microbiome 6, 119. doi: 10.1186/s40168-018-0507-3
Pellicer, J., Powell, R. F., Leitch, I. J. (2021). The application of flow cytometry for estimating genome size, ploidy level endopolyploidy, and reproductive modes in plants. Methods Mol. Biol. 2222, 325–361. doi: 10.1007/978-1-0716-0997-2_17
Pfenninger, M., Schonnenbeck, P., Schell, T. (2022). ModEst: Accurate estimation of genome size from next generation sequencing data. Mol. Ecol. Resour 22, 1454–1464. doi: 10.1111/1755-0998.13570
Pflug, J. M., Holmes, V. R., Burrus, C., Johnston, J. S., Maddison, D. R. (2020). Measuring genome sizes using read-depth, k-mers, and flow cytometry: methodological comparisons in beetles (Coleoptera). G3 (Bethesda) 10, 3047–3060. doi: 10.1534/g3.120.401028
Puranik, S., Sahu, P. P., Srivastava, P. S., Prasad, M. (2012). NAC proteins: regulation and role in stress tolerance. Trends Plant Sci. 17, 369–381. doi: 10.1016/j.tplants.2012.02.004
Ranallo-Benavidez, T. R., Jaron, K. S., Schatz, M. C. (2020). GenomeScope 2.0 and Smudgeplot for reference-free profiling of polyploid genomes. Nat. Commun. 11, 1432. doi: 10.1038/s41467-020-14998-3
Reinar, W. B., Lalun, V. O., Reitan, T., Jakobsen, K. S., Butenko, M. A. (2021). Length variation in short tandem repeats affects gene expression in natural populations of Arabidopsis thaliana. Plant Cell 33, 2221–2234. doi: 10.1093/plcell/koab107
Saikia, P., Deka, J., Bharali, S., Kumar, A., Tripathi, O., Singha, L., et al. (2017). Plant diversity patterns and conservation status of eastern Himalayan forests in Arunachal Pradesh, Northeast India. For. Ecosyst. 4, 1–12. doi: 10.1186/s40663-017-0117-8
Sambrook, J., Russell, D. (2001). Molecular cloning: A laboratory manual. 3rd ed (NY: Cold Spring Harbor Laboratory Press). Cold Spring Harbor". NY.
Schranz, M. E., Mohammadin, S., Edger, P. P. (2012). Ancient whole genome duplications, novelty and diversification: the WGD Radiation Lag-Time Model. Curr. Opin. Plant Biol. 15, 147–153. doi: 10.1016/j.pbi.2012.03.011
Schulz, P., Herde, M., Romeis, T. (2013). Calcium-dependent protein kinases: hubs in plant stress signaling and development. Plant Physiol. 163, 523–530. doi: 10.1104/pp.113.222539
Selkoe, K. A., Toonen, R. J. (2006). Microsatellites for ecologists: a practical guide to using and evaluating microsatellite markers. Ecol. Lett. 9, 615–629. doi: 10.1111/j.1461-0248.2006.00889.x
Shan, T., Wu, J., Yu, D., Xie, J., Fang, Q., Zha, L., et al. (2020). Genome survey sequencing of Atractylodes lancea and identification of its SSR markers. Biosci. Rep. 40, BSR20202709. doi: 10.1042/BSR20202709
Shi, L., Yi, S., Li, Y. (2018). Genome survey sequencing of red swamp crayfish Procambarus clarkii. Mol. Biol. Rep. 45, 799–806. doi: 10.1007/s11033-018-4219-3
Shi, M., Cheng, Y., Zhang, W., Xia, X. (2016a). The evolutionary mechanism of genome size. Chin. Sci. Bull. 61, 3188–3195. doi: 10.1360/n972016-00728
Shi, X., Zhu, X., Sheng, Y., Zhuang, G., Chen, F. (2016b). Development of SSR markers based on transcriptome sequence of phoebe zhennan. Scientia Silvae Sinicae 52, 71–78. doi: 10.11707/j.1001-7488.20161109
Simao, F. A., Waterhouse, R. M., Ioannidis, P., Kriventseva, E. V., Zdobnov, E. M. (2015). BUSCO: assessing genome assembly and annotation completeness with single-copy orthologs. Bioinformatics 31, 3210–3212. doi: 10.1093/bioinformatics/btv351
Singh, R., Ming, R., Yu, Q. (2016). Comparative analysis of GC content variations in plant genomes. Trop. Plant Biol. 9, 136–149. doi: 10.1007/s12042-016-9165-4
Soza, V. L., Lindsley, D., Waalkes, A., Ramage, E., Patwardhan, R. P., Burton, J. N., et al. (2019). The Rhododendron genome and chromosomal organization provide insight into shared whole-genome duplications across the heath family (Ericaceae). Genome Biol. Evol. 11, 3353–3371. doi: 10.1093/gbe/evz245
Stamatakis, A. (2014). RAxML version 8: a tool for phylogenetic analysis and post-analysis of large phylogenies. Bioinformatics 30, 1312–1313. doi: 10.1093/bioinformatics/btu033
Stanke, M., Keller, O., Gunduz, I., Hayes, A., Waack, S., Morgenstern, B. (2006). AUGUSTUS: ab initio prediction of alternative transcripts. Nucleic Acids Res. 34, W435–W439. doi: 10.1093/nar/gkl200
Sun, X., Wang, G., Yang, J., Yu, W., Xu, J., Tang, B., et al. (2023). Whole genome evaluation analysis and preliminary Assembly of Oratosquilla oratoria (Stomatopoda: Squillidae). Mol. Biol. Rep. 50, 4165–4173. doi: 10.1007/s11033-023-08356-x
Talavera, G., Castresana, J. (2007). Improvement of phylogenies after removing divergent and ambiguously aligned blocks from protein sequence alignments. Syst. Biol. 56, 564–577. doi: 10.1080/10635150701472164
Thermo Fisher Scientific (2014). Qubit 3.0 fluorometer user guide. Available online at: https://tools.thermofisher.com/content/sfs/manuals/qubit_3_fluorometer_man.pdf.
Vinogradov, A. E. (2003). DNA helix: the importance of being GC-rich. Nucleic Acids Res. 31, 1838–1844. doi: 10.1093/nar/gkg296
Wan, H., Wei, Q., Ji, Q., Lan, H., Dai, X., Chen, W., et al. (2021). The karyotype, genome survey, and assembly of Mud artemisia (Artemisia selengensis). Mol. Biol. Rep. 48, 5897–5904. doi: 10.1007/s11033-021-06584-7
Wang, C., Yan, H., Li, J., Zhou, S., Liu, T., Zhang, X., et al. (2018). Genome survey sequencing of purple elephant grass (Pennisetum purpureum Schum ‘Zise’) and identification of its SSR markers. Mol. Breed. 38, 1–10. doi: 10.1007/s11032-018-0849-3
Wang, Y.-L., Li, L., Paudel, B. R., Zhao, J.-L. (2024a). Genomic Insights into High-Altitude Adaptation: A Comparative Analysis of Roscoea alpina and R. purpurea in the Himalayas. Int. J. Mol. Sci. 25, 2265. doi: 10.3390/ijms25042265
Wright, S. I., Lauga, B., Charlesworth, D. (2002). Rates and patterns of molecular evolution in inbred and outbred Arabidopsis. Mol. Biol. Evol. 19, 1407–1420. doi: 10.1093/oxfordjournals.molbev.a004204
Wu, Z. Y., Raven, P. H., Hong, D. Y. (2005). “Ericaceae,” in Flora of China (Beijing, China: Missouri Botanical Garden Press).
Xie, J., Chen, Y., Cai, G., Cai, R., Hu, Z., Wang, H. (2023). Tree Visualization by One Table (tvBOT): a web application for visualizing, modifying and annotating phylogenetic trees. Nucleic Acids Res. 51, W587–W592. doi: 10.1093/nar/gkad359
Xu, D., Yang, Y., Gong, D., Chen, X., Jin, K., Jiang, H., et al. (2023). GFAP: ultrafast and accurate gene functional annotation software for plants. Plant Physiol. 193, 1745–1748. doi: 10.1093/plphys/kiad393
Xu, Y. L., Shen, H. H., Du, X. Y., Lu, L. (2022). Plastome characteristics and species identification of Chinese medicinal wintergreens (Gaultheria, Ericaceae). Plant Divers. 44, 519–529. doi: 10.1016/j.pld.2022.06.002
Yang, F.-S., Nie, S., Liu, H., Shi, T.-L., Tian, X.-C., Zhou, S.-S., et al. (2020). Chromosome-level genome assembly of a parent species of widely cultivated azaleas. Nat. Commun. 11, 5269. doi: 10.1038/s41467-020-18771-4
Yang, R., Jarvis, D. E., Chen, H., Beilstein, M. A., Grimwood, J., Jenkins, J., et al. (2013). The reference genome of the halophytic plant eutrema salsugineum. Front. Plant Sci. 4. doi: 10.3389/fpls.2013.00046
Yang, T., Fang, L., Zhang, X., Hu, J., Bao, S., Hao, J., et al. (2015). High-throughput development of SSR markers from pea (Pisum sativum L.) based on next generation sequencing of a purified Chinese commercial variety. PloS One 10, e0139775. doi: 10.1371/journal.pone.0139775
Yocca, A. E., Platts, A., Alger, E., Teresi, S., Mengist, M. F., Benevenuto, J., et al. (2023). Blueberry and cranberry pangenomes as a resource for future genetic studies and breeding efforts. Hortic. Res. 10, uhad202. doi: 10.1093/hr/uhad202
Yuan, Z., Hong, Z., Jun, T., Yanfang, D., Jing, M., Dongyun, X., et al. (2019). Genome Survey of Rhododendron simsii and Rhododendron indicum. Mol. Plant Breed. 17, 4928–4935. doi: 10.13271/j.mpb.017.004928
Zhang, L., Xu, P., Cai, Y., Ma, L., Li, S., Li, S., et al. (2017). The draft genome assembly of Rhododendron delavayi Franch. var. delavayi. Gigascience 6, 1–11. doi: 10.1093/gigascience/gix076
Zhang, X., Chen, S., Zhang, Y., Xiao, Y., Qin, Y., Li, Q., et al. (2023a). Draft genome of the medicinal tea tree Melaleuca alternifolia. Mol. Biol. Rep. 50, 1545–1552. doi: 10.1007/s11033-022-08157-8
Zhang, X., Kuang, T., Dong, W., Qian, Z., Zhang, H., Landis, J. B., et al. (2023b). Genomic convergence underlying high-altitude adaptation in alpine plants. J. Integr. Plant Biol. 65, 1620–1635. doi: 10.1111/jipb.13485
Zheng, Y., Jiao, C., Sun, H., Rosli, H. G., Pombo, M. A., Zhang, P., et al. (2016). iTAK: A program for genome-wide prediction and classification of plant transcription factors, transcriptional regulators, and protein kinases. Mol. Plant 9, 1667–1670. doi: 10.1016/j.molp.2016.09.014
Zhou, W., Hu, Y., Sui, Z., Fu, F., Wang, J., Chang, L., et al. (2013). Genome survey sequencing and genetic background characterization of Gracilariopsis lemaneiformis (Rhodophyta) based on next-generation sequencing. PloS One 8, e69909. doi: 10.1371/journal.pone.0069909
Zhou, X. J., Liu, M. X., Lu, X. Y., Sun, S. S., Cheng, Y. W., Ya, H. Y. (2020). Genome survey sequencing and identification of genomic SSR markers for Rhododendron micranthum. Biosci. Rep. 40, BSR20200988. doi: 10.1042/BSR20200988
Zhou, P., Zhang, Q., Li, J., Li, F., Huang, J., Zhang, M. (2023). A first insight into the genomic background of Ilex pubescens (Aquifoliaceae) by flow cytometry and genome survey sequencing. BMC Genomics 24, 270. doi: 10.1186/s12864-023-09359-5
Zhu, D., Song, W., Yang, K., Cao, X., Gul, Y., Wang, W. (2012). Flow cytometric determination of genome size for eight commercially important fish species in China. In Vitro Cell Dev. Biol. Anim. 48, 507–517. doi: 10.1007/s11626-012-9543-7
Keywords: whole genome sequencing, gene annotation, evergreen shrubs, flow cytometry, microsatellite, SSR primers, transcription factors, phylogenetic relationships
Citation: Lin Y-J, Ding X-Y, Huang Y-W and Lu L (2024) First De Novo genome assembly and characterization of Gaultheria prostrata. Front. Plant Sci. 15:1456102. doi: 10.3389/fpls.2024.1456102
Received: 11 July 2024; Accepted: 04 October 2024;
Published: 29 October 2024.
Edited by:
Trude Schwarzacher, University of Leicester, United KingdomReviewed by:
Manosh Kumar Biswas, University of Leicester, United KingdomJana Sochorová, Academy of Sciences of the Czech Republic (ASCR), Czechia
Copyright © 2024 Lin, Ding, Huang and Lu. This is an open-access article distributed under the terms of the Creative Commons Attribution License (CC BY). The use, distribution or reproduction in other forums is permitted, provided the original author(s) and the copyright owner(s) are credited and that the original publication in this journal is cited, in accordance with accepted academic practice. No use, distribution or reproduction is permitted which does not comply with these terms.
*Correspondence: Lu Lu, bHVsdWttdUAxNjMuY29t