- 1Department of Forest Genetics and Plant Physiology, Swedish University of Agricultural Sciences, Umeå, Sweden
- 2Institute of Biotechnology in Plant Production, Department of Agrobiotechnology, University of Natural Resources and Life Sciences Vienna, Tulln an der Donau, Austria
- 3Center for International Blood and Marrow Transplant Research, National Marrow Donor Program/Be The Match, Minneapolis, MN, United States
- 4Saatzucht Donau GmbH & CoKG, Reichersberg, Austria
- 5Department of Plant Sciences, Norwegian University of Life Sciences, Ås, Norway
- 6Institute for Crop Science and Plant Breeding, Bavarian State Research Center for Agriculture, Freising, Germany
- 7Summerland Research & Development Centre, Agriculture and Agri-Food Canada/Government of Canada, Summerland, BC, Canada
- 8Secobra Saatzucht GmbH, Moosburg an der Isar, Germany
- 9Department of Biotechnology and Plant Biology - Centre for Biotechnology and Plant Genomics - Universidad Politécnica de Madrid, Madrid, Spain
- 10Saatzucht Donau GmbH & CoKG, Probstdorf, Austria
Fusarium head blight (FHB) is a devastating disease of wheat, causing yield losses, reduced grain quality, and mycotoxin contamination. Breeding can mitigate the severity of FHB epidemics, especially with genomics-assisted methods. The mechanisms underlying resistance to FHB in wheat have been extensively studied, including phenological traits and genome-wide markers associated with FHB severity. Here, we aimed to improve genomic prediction for FHB resistance across breeding programs by incorporating FHB-correlated traits and FHB-associated loci as model covariates. We combined phenotypic data on FHB severity, anthesis date, and plant height with genome-wide marker data from five Central European winter wheat breeding programs for genome-wide association studies (GWAS) and genomic prediction. Within all populations, FHB was correlated with anthesis date and/or plant height, and a marker linked to the semi-dwarfing locus Rht-D1 was detected with GWAS for FHB. Including the Rht-D1 marker, anthesis date, and/or plant height as covariates in genomic prediction modeling improved prediction accuracy not only within populations but also in cross-population scenarios.
1 Introduction
Fusarium head blight (FHB) is a fungal disease of wheat (Triticum aestivum) caused by several species of Fusarium, including Fusarium culmorum and Fusarium graminearum (Moreno-Amores et al., 2020b). FHB can lead to yield losses due to reduced grain quality and contamination with mycotoxins, such as deoxynivalenol, which are harmful to human and animal health (Buerstmayr et al., 2020). The inheritance of resistance to FHB is quantitative and complex, controlled by many small-effect loci across the genome. Phenological and morphological traits, such as anthesis date, plant height, and anther retention, have also been implicated as passive mechanisms influencing FHB resistance (Buerstmayr et al., 2020). Notable examples are the reduced height (Rht) genes Rht-B1 and Rht-D1, which have been widely introgressed into elite wheat germplasm but have pleiotropic effects on plant height, anther retention, and FHB resistance, where lines with the semi-dwarfing allele have reduced plant height, increased anther retention, and greater susceptibility to FHB (Srinivasachary et al., 2009; Buerstmayr and Buerstmayr, 2016, 2022; He et al., 2016; Akohoue et al., 2022).
Genomics can assist breeding for resistance to FHB. For well-validated, medium- and major-effect quantitative trait loci (QTL), marker-assisted selection (MAS) can be used to introgress favorable alleles from FHB resistance QTL into desirable cultivars (Buerstmayr et al., 2020). For example, the QTL Fhb1 and Qfhs.ifa-5A, which confer type 2 and type 1 resistance, respectively, have been successfully deployed in breeding programs (Buerstmayr and Buerstmayr, 2022). However, the complex inheritance and genotype-by-environment interactions underlying resistance to FHB can reduce heritability estimates and can complicate the identification of associated single nucleotide polymorphisms (SNPs) via genome-wide association studies (GWAS) and QTL mapping (Buerstmayr et al., 2020). In addition, the process of identifying, validating, and employing MAS for one or more FHB resistance QTL is relatively slow and costly (Poland and Rutkoski, 2016). Genomic prediction/selection is a powerful and efficient tool for plant breeding, accelerating the breeding cycle and increasing genetic gain for quantitative traits, such as FHB resistance (Heffner et al., 2010; Heslot et al., 2015; Poland and Rutkoski, 2016; Crossa et al., 2017; Buerstmayr et al., 2020). In contrast to MAS, which considers the effects of individual loci, genomic prediction leverages genome-wide data on SNPs to predict breeding values for the trait(s) of interest (Poland and Rutkoski, 2016; Buerstmayr et al., 2020). One of the most widely used genomic prediction models is genomic best linear unbiased prediction (GBLUP), in which the phenotype is modeled against a relationship matrix estimated from genome-wide SNPs (Meuwissen et al., 2001; Poland and Rutkoski, 2016).
Previous studies have reported that including FHB-correlated traits, which tend to have higher heritability, or FHB-associated SNPs can improve genomic prediction accuracy for FHB resistance (Arruda et al., 2016; Larkin et al., 2020, 2021; Moreno-Amores et al., 2020b, 2020a; Zhang et al., 2021; Akohoue et al., 2022). Several studies have reported results on genomic prediction of FHB resistance within populations, but few have assessed cross-population prediction (Rutkoski et al., 2012; Jiang et al., 2015; Mirdita et al., 2015; Arruda et al., 2016; Herter et al., 2019; Liu et al., 2019; Moreno-Amores et al., 2020b; Verges et al., 2020, 2021; Larkin et al., 2020, 2021; Moreno-Amores et al., 2020a; Sneller et al., 2021; Zhang et al., 2021, 2022; Akohoue et al., 2022; Winn et al., 2023; Garcia-Abadillo et al., 2023).
We sought to leverage the wide existing knowledge on FHB for the improvement of genomic prediction of resistance to FHB across wheat breeding programs. Here, we combined phenotypic (FHB severity, anthesis date, and plant height) and genome-wide SNP data on five Central European winter wheat populations from Austrian and German seed companies, the Bavarian State Research Centre for Agriculture, and the Horizon 2020 SusCrop—ERA-NET “WheatSustain” project for GWAS and genomic prediction. We evaluated whether the inclusion of FHB-correlated traits (i.e., plant height and anthesis date) and/or the Rht-D1 SNP (identified via GWAS) as covariates in GBLUP improved prediction accuracy for FHB severity in comparison to standard GBLUP in cross-validated (within-population) and cross-population scenarios. To our knowledge, our study is the first to combine both FHB-correlated traits and FHB-associated loci in genomic prediction for FHB. In addition, we describe novel methods for harmonizing data across variable experimental conditions and breeding programs for genomic prediction of FHB within and between populations.
2 Materials and methods
2.1 Germplasm
We evaluated breeding material from five winter wheat breeding programs in this study: the WheatSustain training set (WTS) and advanced lines (F6–F8) from the breeding programs of the Bavarian State Research Center for Agriculture (LfL), Saatzucht Donau GmbH & CoKG (Probstdorf/Reichersberg, Austria; SZD), Saatzucht Josef Breun GmbH & CoKG (Herzogenaurach, Germany; BRE), and Secobra Saatzucht GmbH (Feldkirchen, Germany; SEC). The SZD population comprised 2,279 lines. From each of the German breeding programs, advanced lines from two consecutive breeding cycles were evaluated in 2020 and 2021. The 2020 and 2021 breeding cycles from BRE, LfL, and SEC comprised 446 and 543, 148 and 143, and 516 and 269 lines, respectively. Hereafter, the three German breeding programs will be cumulatively referred to as the German population (DEU). WTS comprised 230 winter wheat cultivars and breeding lines, which were chosen with the aim of capturing the genetic variation present in Central and Northern European winter wheat (Isidro y Sánchez and Akdemir, 2021; Garcia-Abadillo et al., 2023).
2.2 Phenotyping
The WheatSustain training set was evaluated across seven location-year environments, as described in a previous study (Garcia-Abadillo et al., 2023) (Supplementary Table S1). Briefly, WTS was grown in a randomized complete block design with two replications in 2020 and 2021 in Tulln an der Donau, Austria and Vollebekk, Norway and in 2020 in Reichersberg, Austria, and in non-replicated trials in Feldkirchen, Germany in 2020 and 2021. The DEU material was evaluated in non-replicated trials in Feldkirchen, Germany in 2020 and 2021. The SZD population was evaluated in Tulln an der Donau, Austria in a randomized incomplete block design with two replications per year from 2015 to 2022, with an overlap of approximately 30 lines from one year to the next and most lines evaluated in only one year.
In the Feldkirchen trials, all plots were spray inoculated with an F. culmorum conidial suspension with a concentration of 1.5 × 104 conidia/mL on 22, 27, and 30 May and on 2 and 8 June in 2020 and on 8, 12, 15, and 22 June in 2021. In the Tulln an der Donau trials, all plots were spray inoculated every other day throughout the entire anthesis period with an F. culmorum conidial suspension with a concentration of 2.5 × 104 conidia/mL, and a high level of humidity was maintained with an automated mist irrigation system for 20 h after each inoculation (Buerstmayr et al., 2011; Moreno-Amores et al., 2020a). In the Vollebekk trials, grain spawn inoculum (oat kernels infected with F. graminearum) was applied across the field at the booting stage with a density of 10 g/m2 followed by daily mist irrigation in the evening until three to four weeks after anthesis (Lu et al., 2013; Tekle et al., 2018). In Reichersberg, grain spawn inoculum (maize kernels infected with F. graminearum) was applied across the field four to five weeks before head emergence with a density of 25–30 g/m2 (Buerstmayr et al., 2011).
In all trials, the anthesis date (AD) of each plot was recorded when half of the spikes reached anthesis in the plot. In all trials, except for Tulln an der Donau 2016 and Reichersberg 2020, the plant height (PH) of each plot was measured at physiological maturity. In all Tulln an der Donau trials, Fusarium head blight severity (FHB) was scored on a percentage scale (0%–100% infection) at six time points relative to the AD of each plot (approximately 10, 14, 18, 22, 26, and 30 days post-anthesis). In the Feldkirchen 2020 trials, all plots were scored for FHB on 25 and 29 June and on 2 July. In the Feldkirchen 2021 trials, the breeding plots were scored for FHB on 24 June and on 5, 12, and 16 July, while the WTS plots were scored on 24 June and 1, 6, 12, and 18 July. In the Reichersberg 2020 trial, all plots were scored for FHB on 9 July. In the Vollebekk trials, FHB was scored on each plot once between 6 June and 1 July in 2020 and between 12 and 23 July in 2021.
2.3 Genotyping
All lines from WTS were genotyped, while a subset from the DEU and SZD breeding programs was selected for genotyping (Table 1). Genomic DNA was extracted from one-week-old seedlings and sent for genotyping at TraitGenetics GmbH (Gatersleben, Germany) (Shahinnia et al., 2022; Garcia-Abadillo et al., 2023). Lines from DEU and WTS were genotyped with the 25K Infinium iSelect array (TraitGenetics GmbH, Gatersleben, Germany), while the SZD material was genotyped with the 7K array (TraitGenetics GmbH, Gatersleben, Germany), which is a subset of the 25K array (Shahinnia et al., 2022; Garcia-Abadillo et al., 2023). The physical positions of the SNPs had been previously called against the Chinese Spring reference genome (IWGSC RefSeq v1.0) (IWGSC, 2018; Shahinnia et al., 2022). The SNP data from the 25K array for DEU had been previously filtered for polymorphism, proportion missing < 10%, and minor allele frequency (MAF) > 5% by Shahinnia et al. (2022), resulting in 17,040 SNPs for further analysis. The SZD and WTS data were filtered using the same criteria as DEU, resulting in 6,709 and 19,656 SNPs for further analysis on SZD and WTS, respectively (Supplementary Table S2).

Table 1. Number of genotypes available for genomic prediction, number of environments in which Fusarium head blight (FHB) severity was evaluated, and genomic heritability for FHB, anthesis date, and plant height within three populations.
2.4 Phenotypic analysis
Because FHB was scored differently across trials, we sought to harmonize the phenotypic data. First, we calculated the number of days between the AD and the date of each FHB observation for each plot (days to score, DTS) due to the sigmoidal relationship between the number of days since initial Fusarium infection during anthesis and FHB symptom severity (Garcia-Abadillo et al., 2023). The DTS values were then assigned to 10 time points, approximating the FHB scoring time points used in the Tulln an der Donau trials: (−1) DTS ≤ 0, (0) 0 < DTS ≤ 8, (1) 8 < DTS ≤ 12, (2) 12 < DTS ≤ 16, (3) 16 < DTS ≤ 20, (4) 20 < DTS ≤ 24, (5) 24 < DTS ≤ 28, (6) 28 < DTS ≤ 32, (7) 32 < DTS ≤ 36, and (8) DTS > 36. We then estimated the variance of the FHB observations for each time point within each trial. Garcia-Abadillo et al. (2023) previously analyzed the WTS data described here and found that using FHB data from the time point with the greatest variance in FHB was optimal for genomic prediction across trials. As such, we used FHB data from the time point with the greatest FHB variance within each trial for further analysis. With this strategy, we were able to harmonize the FHB data across trials, with the majority (98%) of the lines present in the full dataset used for further genotype–phenotype analysis (Table 1). All AD and PH data were used for further analysis.
For the SZD and WTS populations, which were evaluated in multi-environment trials, we fit the following phenotypic mixed model for each trait with the “breedR” package (Muñoz and Sanchez, 2020) in R (R Core Team, 2020):
where yijk is the FHB, AD, or PH of each plot; µ is the overall mean; Geni is the fixed effect of genotype i; Envj is the random effect of environment (year for SZD trials, location–year for WTS trials) j, Rep[Env]jk is the random effect of replication k nested within environment j, GenEnvij is the random effect of the interaction between genotype i and environment j, and ϵ is the error. Genotype best linear unbiased estimates (BLUEs) for each trait were extracted for further use in GWAS and genomic prediction.
We calculated trait correlations (r) between genotype BLUEs (SZD and WTS) or plot-level values (DEU) for FHB, AD, and PH within each population as:
where cor(trait1,trait2) is the Pearson’s correlation between trait 1 and trait 2, and h21 and h22 are the genomic heritability of trait 1 and trait 2, respectively (Sodini et al., 2018). We estimated genomic heritability (h2) for each trait within each population using the unbiased average semivariance (ASV) method (Feldmann et al., 2022). First, we fit a mixed model for each trait as:
where yi is the response vector of genotype BLUEs (SZD and WTS) or plot-level values (DEU) for FHB, AD, or PH; µ is the overall mean; Geni is the random effect of genotype i; and ϵ is the error. The variance of the genotype term was modeled as Kσ2a, where K is the realized additive genomic relationship matrix and σ2a is the estimated additive genomic variance (Yu et al., 2006; Endelman and Jannink, 2012). We scaled K with the ASV method as:
where KASV is the ASV-scaled genomic relationship matrix and j is the number of genotypes. We then extracted the genotypic (σ2g) and residual (σ2ϵ) variances to calculate .
2.5 Population structure analysis
We used TASSEL version 5.2.85 (Bradbury et al., 2007) to assess population structure across the 3,088 lines from the five breeding programs/populations. We first estimated a distance matrix for the 3,088 lines from the 7K SNP data and then conducted multidimensional scaling (MDS) analysis on the distance matrix. We extracted the eigenvalues of the first five coordinates and the corresponding coordinate values for the 3,088 lines. MDS revealed that the BRE, LfL, and SEC material clustered into one population, hereafter referred to as the German (DEU) population, which was used for further genotype–phenotype analysis.
2.6 Genome-wide association analysis
We used TASSEL version 5.2.85 (Bradbury et al., 2007) for GWAS of FHB, AD, and PH within each of the three populations. We fit the following mixed model:
where yi is the response vector of genotype BLUEs (SZD and WTS) or plot-level values (DEU) for FHB, AD, or PH; µ is the overall mean; SNPi is the fixed effect of each SNP (coded as −1, 0, 1); β is the regression coefficient for each SNP; Geni is the random effect of genotype i; and ϵ is the error. The variance of the genotype term was modeled as Kσ2a, where K is the realized additive relationship matrix and σ2a is the estimated additive genetic variance (Yu et al., 2006; Endelman and Jannink, 2012). We extracted the SNP p-values and effect estimates from each GWAS model. For multiple test correction of the SNP p-values, we conducted a false discovery rate (FDR; α=0.05) analysis for each GWAS model with the “qvalue” package (Storey, 2015) in R (R Core Team, 2020). We used the 25K SNP data for GWAS within DEU and WTS and the 7K SNP data for GWAS within SZD.
2.7 Genomic prediction modeling
We used the “remlf90” function in the “breedR” package (Muñoz and Sanchez, 2020) in R (R Core Team, 2020) for all genomic prediction modeling described hereafter. Within each population, we fit the following basic GBLUP mixed model for FHB with five-fold cross-validation with 10 replications:
where yi is the response vector of genotype BLUEs (SZD and WTS) or plot-level values (DEU) for FHB, AD, or PH; µ is the overall mean; Geni is the random effect of genotype i; and ϵ is the error. The variance of the genotype term was modeled as KASVσ2a, where KASV is the ASV-scaled realized additive relationship (kinship) matrix and σ2a is the estimated additive genetic variance (Yu et al., 2006; Endelman and Jannink, 2012; Feldmann et al., 2022). Within each fold of each replication, the values of the validation genotypes were set to missing in the yi response vector. The kinship matrix was estimated from all genotypes in the population. From each model, we estimated genomic heritability (h2g) as described previously. We extracted the genotype best linear unbiased predictors (BLUPs) from each model as the genomic estimated breeding values (GEBVs) and then calculated prediction accuracy (PA) as:
where cor(obs,pred) is the Pearson’s correlation between the observed (FHB BLUEs or plot phenotypes) and predicted (GEBVs) values of the validation genotypes and h2g is the genomic heritability.
We also fit the following “trait-assisted” GBLUP mixed model with five-fold cross-validation with 10 replications within each population:
where yi is the response vector of genotype FHB BLUEs (SZD and WTS) or plot-level FHB values (DEU), µ is the overall mean, C1-2i are the BLUEs for AD or PH of genotype i, βc1–2 are the regression coefficients for the C1–2 terms, Geni is the random effect of genotype i, and ϵ is the error. The variance of the genotype term was modeled as KASVσ2a, where KASV is the ASV-scaled realized additive relationship (kinship) matrix and σ2a is the estimated additive genetic variance (Yu et al., 2006; Endelman and Jannink, 2012; Feldmann et al., 2022). Within each population, AD and PH were modeled alone and together. Within each fold of each replication, the values of the validation genotypes were set to missing in the yi response vector. BLUEs (SZD and WTS) or plot-level values (DEU) for AD and/or PH from all genotypes in the population were included in C1i and/or C2i. The kinship matrix was estimated from all genotypes in the population. From each trait-assisted model, we extracted the genotype BLUPs and the regression coefficient(s) of the trait covariate(s) and calculated the GEBV of each genotype as
where BLUP is the genotype BLUP, βc1–2 are the regression coefficients for AD or PH, and C1–2 are the genotype BLUES (SZD and WTS) for AD or PH or plot-level AD or PH values (DEU). We calculated PA as described previously.
A SNP linked to the Rht-D1 locus was the only SNP significantly associated with FHB in GWAS in all three populations. We tested the use of the Rht-D1 SNP as a covariate in all GBLUP and trait-assisted GBLUP models previously described, within population with five-fold cross-validation with 10 replications. All terms remain unchanged for each of the previously described models, except for the inclusion of the Rht-D1 SNP as βRhtRhti, where Rhti is the fixed effect of the Rht-D1 SNP and βRht is the corresponding regression coefficient. Within each fold of each replication, the values of the validation genotypes were set to missing in the yi response vector. SNP values and BLUEs (SZD and WTS) or plot-level values (DEU) for AD and/or PH from all genotypes in the population were included in Rhti and C1i and/or C2i, respectively. The kinship matrix was estimated from all genotypes in the population. From each model, we extracted the genotype BLUPs and the regression coefficients of the trait and Rht-D1 covariates and calculated the GEBV of each genotype as
where BLUP is the genotype BLUP, βc1–2 are the regression coefficients for AD or PH and C1–2 are the genotype BLUES (SZD and WTS) for AD or PH or plot-level AD or PH values (DEU), βRht is the regression coefficient for the Rht-D1 SNP, and Rht is the SNP value (alleles coded as −1, 0, 1). We calculated PA as described previously.
We also tested all previously described GBLUP and trait-assisted GBLUP models with and without Rht-D1 between all pairs of populations. For each model and for each pair of populations, one population was modeled as the training population and the other as the validation population, and vice versa. For each between-population model, the values of the validation genotypes were set to missing in the yi response vector. Depending on the model (see previous model descriptions), SNP values and/or BLUEs (SZD and WTS) or plot-level values (DEU) for AD and/or PH from all genotypes from both the training and validation populations were included in Rhti and/or C1i and/or C2i, respectively. The kinship matrix was estimated from all genotypes from both the training and validation populations. We calculated GEBVs and PA as described previously.
3 Results
3.1 Limited population structure among breeding programs
The first and second MDS coordinates explained 28% and 23% of the total variance, respectively. MDS revealed limited population structure, with the first coordinate showing some separation between the Austrian breeding program of Saatzucht Donau (SZD) and the material from the German breeding programs of Saatzucht Breun (BRE), the Bavarian State Research Center for Agriculture (LfL), and Secobra Saatzucht (SEC) (Figure 1). BRE, LfL, and SEC were highly genetically related and clustered into one German (DEU) population. The SZD material had greater genetic diversity than the DEU population and the WheatSustain training set (WTS), as demonstrated by the variation among SZD lines on the first coordinate (Figure 1). The genetic variation present in WTS overlapped that of the Austrian and German breeding programs (Figure 1).
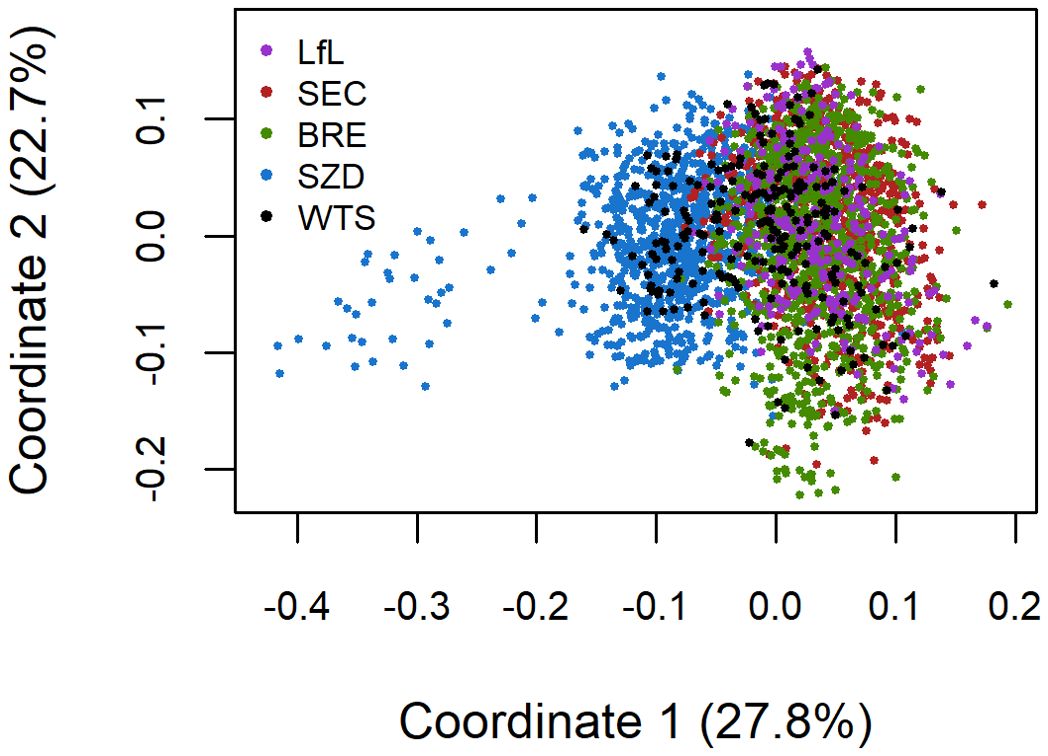
Figure 1. Scatterplot of the first two coordinates from a multidimensional scaling analysis of 3088 lines from the WheatSustain training set (WTS) and the winter wheat breeding programs of Saatzucht Josef Breun (BRE), the Bavarian State Research Center for Agriculture (LfL), Secobra Saatzucht (SEC), and Saatzucht Donau (SZD) using 7K SNP data.
3.2 Moderate to high heritability for and correlations between FHB severity, anthesis date, and plant height
Genomic heritability (h2 = 0.42–0.99) was moderate to high for all traits (Table 1). WTS had the highest heritability for FHB (h2 = 0.72) and AD (h2 = 0.99), while SZD had the highest heritability for PH (h2 = 0.53) (Table 1). Trait correlations were consistent across populations (Table 2). FHB was significantly positively correlated with AD (r = 0.42–0.64) and negatively correlated with PH (r = −[0.22–0.30]) (Table 2). AD and PH were significantly positively correlated within DEU and SZD (r = 0.38–0.53), but not within WTS (Table 2).
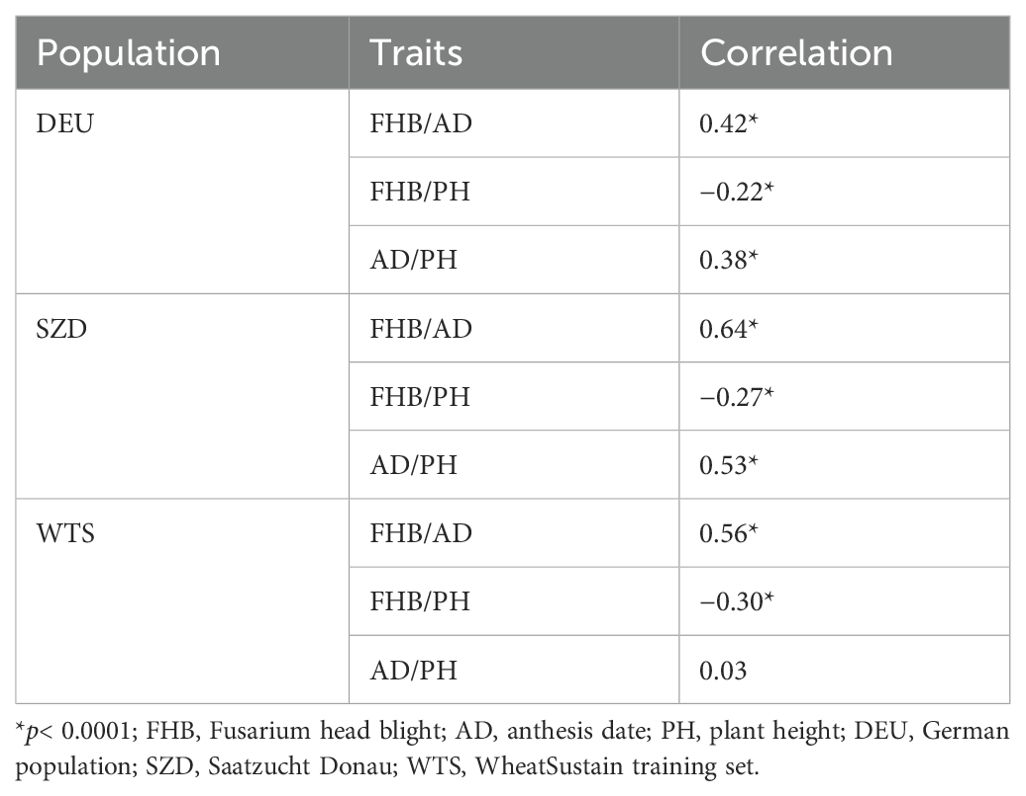
Table 2. Correlations between Fusarium head blight severity, anthesis date, and plant height within three populations.
3.3 Rht-D1 semi-dwarfing locus associated with FHB severity, anthesis date, and plant height
Within all three populations, one locus (TG0011) was significantly associated with FHB and PH (Figure 2; Table 3; Supplementary Figure S1). Two identical SNPs (TG0011a and TG0011b) underlay this locus within DEU and WTS, which were genotyped with the 25K SNP chip. Only one TG0011 SNP was available for SZD, which was genotyped with the 7K SNP subset. TG0011 is a marker linked to the Rht-D1 semi-dwarfing gene on chromosome 4D at 18,781,253 bp (Corsi et al., 2021). The wild-type allele reduced FHB by 3%–25% and increased PH by 5–10 cm, relative to the semi-dwarf allele (Table 3). Although TG0011 was significantly associated with AD only within WTS, the wild-type allele was associated with reduced AD in all populations (Table 3). The Rht-D1 wild-type and semi-dwarfing alleles were approximately equally represented in the DEU material, while the Rht-D1 wild-type allele was the major allele within SZD and WTS (Table 3).
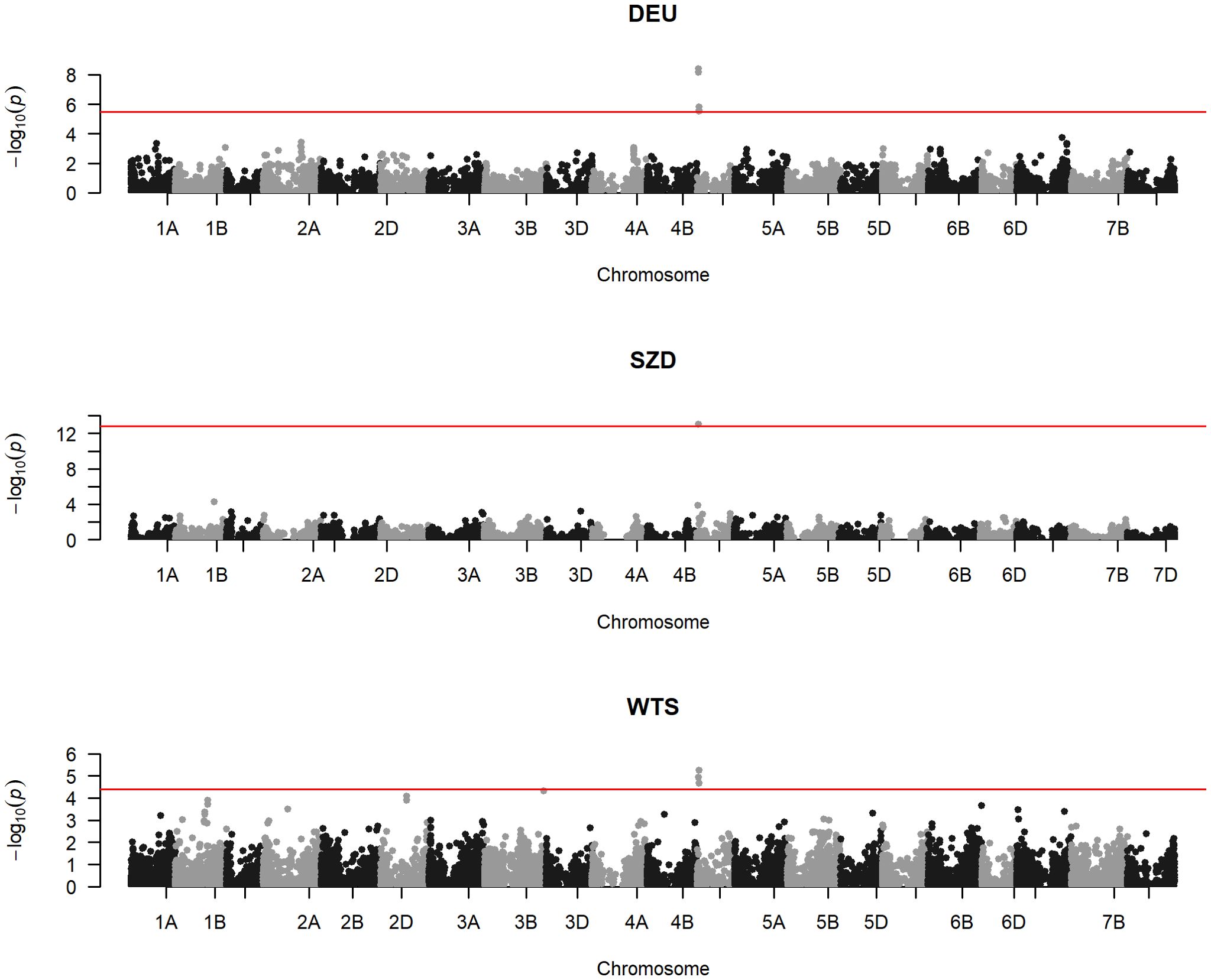
Figure 2. Manhattan plots of genome-wide association studies for Fusarium head blight severity within the German (DEU), Saatzucht Donau (SZD), and WheatSustain training set (WTS) populations, with single nucleotide polymorphism (SNP) physical positions on the x-axis, SNP −log10(p-values) on the y-axis, and red horizontal lines denoting the false discovery rate threshold for SNP significance.
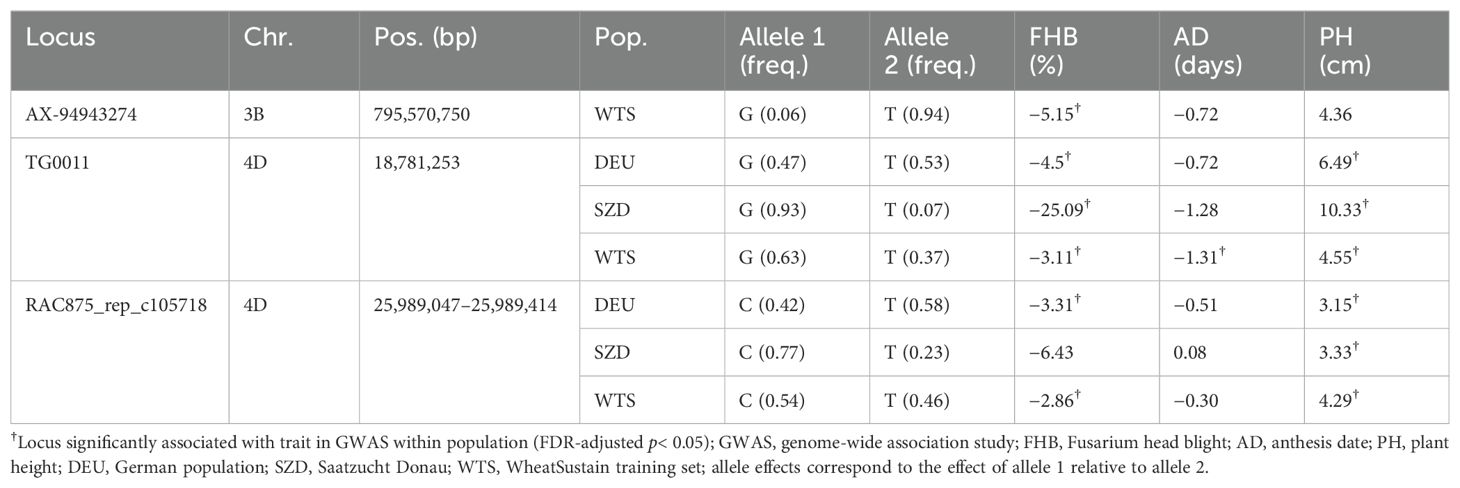
Table 3. Allele frequency and effects on Fusarium head blight (FHB) severity, anthesis date, and plant height of loci significantly associated with FHB within three populations.
Within DEU and WTS, three identical SNPs (RAC875_rep_c105718_672, RAC875_rep_c105718_585, and RAC875_rep_c105718_304) on chromosome 4D at 25,989,047–25,989,414 bp were significantly associated with FHB and PH (Figure 2; Table 3; Supplementary Figure S1). Because SZD was genotyped with fewer SNPs than DEU and WTS, only the RAC875_rep_c105718_672 SNP was available. Within SZD, RAC875_rep_c105718_672 was significantly associated with PH, but not with FHB or AD (Table 3). Similar to TG0011, these three SNPs had opposite allele effects on FHB (−[3–6]%) and PH (3–4 cm) (Table 3).
Within WTS, one SNP (AX-94943274) on chromosome 3B at 795,570,750 bp was significantly associated with FHB, but not with AD or PH (Figure 2; Table 3; Supplementary Figure S1). AX-94943274 had a moderate effect size on FHB (−6%) and a low MAF (6%) within WTS. This SNP was not included in GWAS within SZD and DEU because it was not on the 7K SNP chip used to genotype SZD and because it had been previously filtered (MAF< 5%) from DEU (Shahinnia et al., 2022).
3.4 Anthesis date, plant height, and Rht-D1 can aid genomic prediction of FHB
Cross-validated (CV) genomic prediction accuracy (PA) for FHB was high (PA = 0.69–0.96) and similar across populations (Table 4). Overall, all trait- and Rht-D1-assisted models significantly improved PA in comparison to GBLUP, except for GBLUP+PH (Table 4). AD-assisted models had lower h2 than all other models overall (Table 4). Within all populations, GBLUP+AD, GBLUP+AD+Rht, GBLUP+AD+PH, and GBLUP+AD+PH+Rht improved PA by 7%–25% compared to standard GBLUP (Table 4). Within DEU and SZD, GBLUP models including Rht-D1 and/or PH without AD did not significantly change PA compared to GBLUP (Table 4). Within DEU, GBLUP+AD and GBLUP+AD+PH did not have significantly different PA than their counterparts including Rht-D1, suggesting that AD was the key trait for improving PA (Table 4). Within SZD, GBLUP+AD+PH and GBLUP+AD+PH+Rht had 8–10% higher PA than GBLUP+AD and GBLUP+AD+Rht, indicating that modeling both AD and PH as covariates maximized PA (Table 4). Within WTS, models including Rht-D1 had marginally better PA (3%–8%) than their counterparts without Rht-D1 (Table 4). In addition, AD-assisted models, with or without PH, had the best PA within WTS, suggesting that the combination of both AD and Rht-D1 as covariates can improve prediction accuracy. Genomic heritability was consistent across models within DEU (Table 4). Within SZD and WTS, AD-assisted models yielded lower h2 than models not including AD as a covariate (Table 4). Within WTS, Rht-D1-assisted models had marginally lower h2 than their counterparts without Rht-D1 (Table 4).
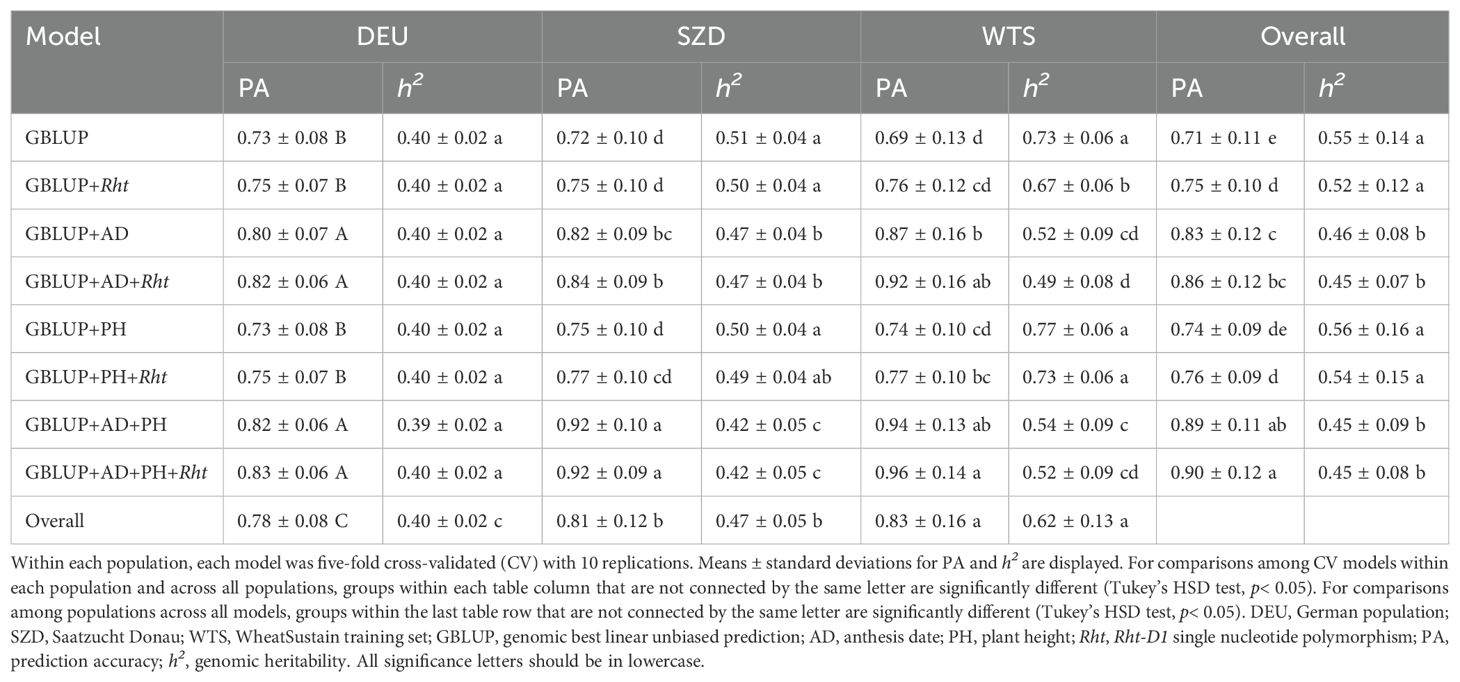
Table 4. Prediction accuracy and genomic heritability for Fusarium head blight severity of GBLUP models with and without trait and Rht-D1 covariates within three populations.
Between-population genomic prediction modeling had moderate to high accuracy for FHB (PA = 0.24–0.79) (Table 5). Including trait and/or Rht-D1 as covariates improved PA by 2%–38% over GBLUP for all pairs of populations (Table 5). AD-assisted models, with or without PH and/or Rht-D1, had the best PA for nearly all pairs of populations; the exception was the scenario where WTS was the training set and SZD was the validation set, for which PH-assisted models had the highest PA (Table 5). For three training/validation sets (DEU/WTS, SZD/DEU, and WTS/DEU), Rht-D1-assisted models had better PA than their counterparts not including Rht-D1 (Table 5). Under most scenarios, the different models and training/validation sets did not appear to impact h2 (Table 5). However, AD-assisted models yielded lower h2 under scenarios where WTS was the training population (Table 5).
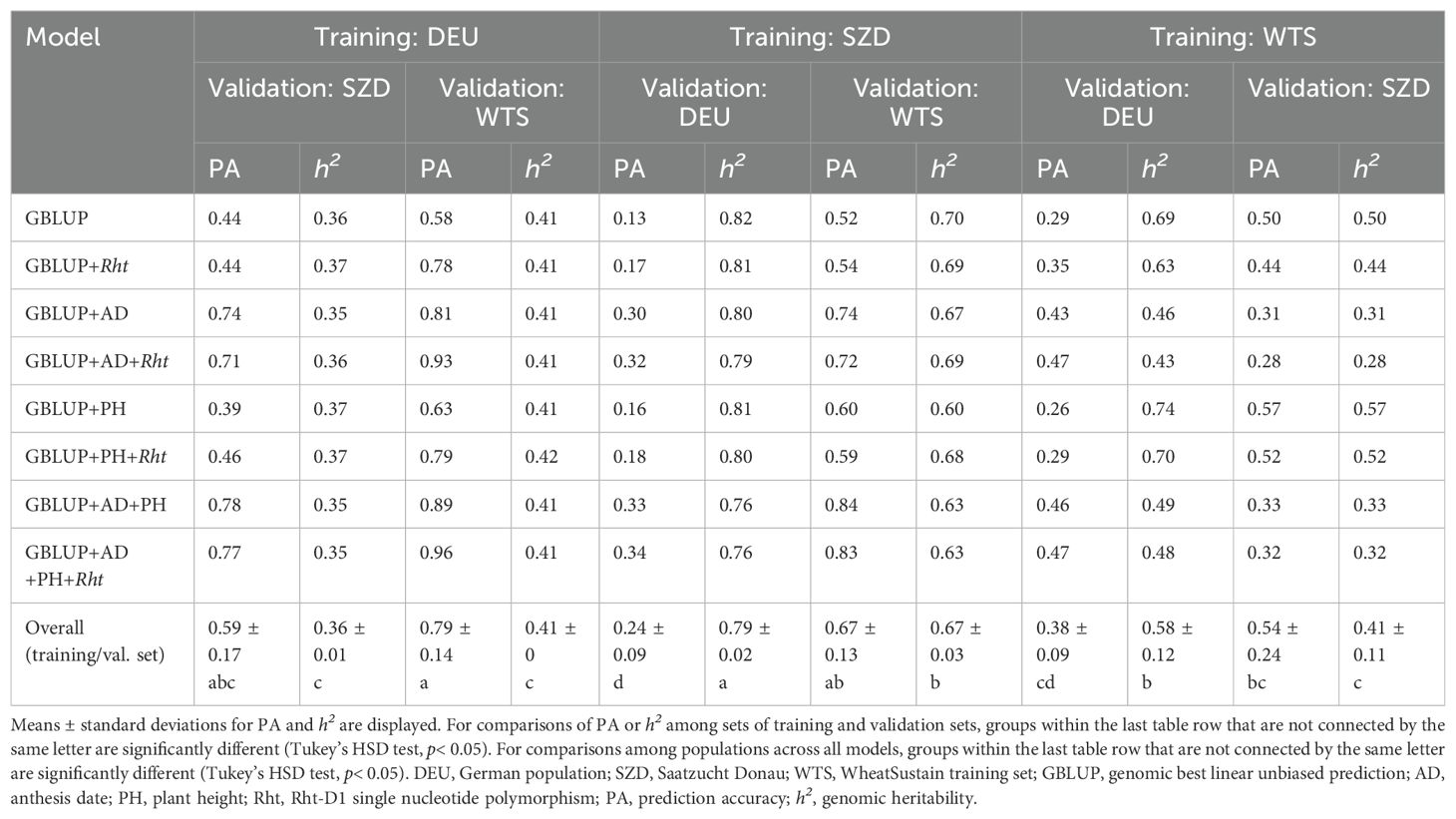
Table 5. Prediction accuracy and genomic heritability for Fusarium head blight severity of GBLUP models with and without trait and Rht-D1 covariates between three populations.
The number of genotypes varied widely among populations, with DEU (N=1991) and WTS (230) having a nearly nine-fold difference in population size (Table 1). Within population, population size was negatively correlated with PA (r = –0.14; p< 0.0001), and the smallest population (WTS) had the best PA and highest h2 overall (Table 4). Between populations, the ratio of training versus validation set size was positively correlated with PA (r = 0.65; p< 0.0001), and the largest population (DEU) had the best PA as the training set but the lowest h2 (Table 5). In addition, WTS was the best predicted validation population (PA = 0.67–0.79), while DEU had the worst PA as the validation population (PA = 0.24–0.38) under between-population genomic prediction (Table 5).
4 Discussion
Here, we evaluated the use of trait and SNP covariates in genomic prediction of FHB resistance within and between three Central European winter wheat breeding populations. Anthesis date and/or plant height were genetically correlated with FHB in all populations. The semi-dwarfing locus Rht-D1 was associated with FHB and plant height in all three populations and with anthesis date in WTS. The allele effects of the Rht-D1 SNP on the three traits matched their corresponding phenotypic correlations. The negative correlation between FHB and plant height has been reported many times, while anthesis date has been found to be both negatively and positively correlated with FHB, depending on the population and seasonal weather conditions (Mirdita et al., 2015; Buerstmayr et al., 2020; Moreno-Amores et al., 2020b, 2020a). The Rht-D1 gene has been previously shown to have pleiotropic effects on plant height and FHB resistance by several studies (Srinivasachary et al., 2009; Buerstmayr and Buerstmayr, 2016, 2022; He et al., 2016; Akohoue et al., 2022).
Cross-validated, within-population PA in our study was comparable to PA for FHB reported in previous studies using GBLUP or ridge regression BLUP, a model similar to GBLUP, in cross-validated scenarios (Rutkoski et al., 2012; Jiang et al., 2015; Mirdita et al., 2015; Arruda et al., 2016; Crossa et al., 2017; Herter et al., 2019; Liu et al., 2019; Moreno-Amores et al., 2020a, 2020b; Verges et al., 2020, 2021; Larkin et al., 2020, 2021; Sneller et al., 2021; Zhang et al., 2021, 2022; Akohoue et al., 2022; Winn et al., 2023; Garcia-Abadillo et al., 2023). We found that trait- and Rht-D1-assisted models improved prediction over standard GBLUP in all scenarios. Incorporating FHB-associated SNPs or FHB-correlated traits in genomic prediction modeling has been reported to significantly improve cross-validated PA in some previous studies, but not in others (Rutkoski et al., 2012; Arruda et al., 2016; Herter et al., 2019; Larkin et al., 2020, 2021; Moreno-Amores et al., 2020b, 2020a; Zhang et al., 2022; Garcia-Abadillo et al., 2023).
The SZD and WTS populations had higher overall cross-validated PA and h2 than DEU (Table 4). Unlike the German breeding populations, the SZD and WTS populations were evaluated under replicated, multi-environment trials. As such, the estimation of genotypic resistance in SZD and WTS was likely more precise than in the German material, leading to better genomic prediction within population. Although the three populations were not all grown in the same trials and the experimental parameters differed across environments and breeding programs, we believe that our methods were sufficient for harmonizing the phenotypic data for further cross-population analysis. We found similar levels of trait correlations, heritability, and GWAS effect estimates across the three populations, and between-population genomic prediction had moderate to high accuracy. Differences in between-population PA can result from a combination of shared genetic and environmental variance. For example, WTS was grown in the same environments as DEU and SZD, and its population structure overlapped that of the German and Austrian breeding programs, which may have led to its high PA as the validation set under between-population prediction. On the contrary, although the experimental conditions of the DEU population were generally less controlled than those of WTS and SZD, DEU was the best training set under between-population prediction scenarios.
Similar to previous reports on within- and cross-population genomic prediction for FHB, PA tended to be lower between populations than within populations (Hoffstetter et al., 2016; Schulthess et al., 2018; Moreno-Amores et al., 2020a; Verges et al., 2020; Sneller et al., 2021). Cross-population genomic prediction tends to be less accurate than within populations, as genome-wide linkage disequilibrium (LD) structure will differ among populations, ultimately changing associations between traits and markers (Buerstmayr et al., 2020; Isidro y Sánchez and Akdemir, 2021). In general, increased relatedness between the training and validation populations tends to yield higher PA for FHB resistance, which was further supported by our findings (Hoffstetter et al., 2016; Schulthess et al., 2018; Herter et al., 2019; Buerstmayr et al., 2020; Verges et al., 2020). However, genomic relatedness alone did not capture all shared variation between populations, as demonstrated by the improvement of GBLUP including anthesis date and/or the Rht-D1 SNP as covariates in most between-population scenarios. Trait correlations shared among populations are not subject to the same dynamics as LD structure, and our results indicate that FHB-correlated traits can complement genomic prediction for FHB, which may be the result of linkage between or pleiotropy at QTL for FHB resistance and FHB-correlated traits (Schulthess et al., 2018; Steiner et al., 2019; Moreno-Amores et al., 2020b, 2020a; Verges et al., 2020; Sneller et al., 2021; Zhang et al., 2022). Here, the allele effects of the Rht-D1 SNP on FHB and FHB-correlated traits were shared among populations and including Rht-D1 SNP as a covariate improved PA, suggesting that linkage phase at this locus was similar across populations (Herter et al., 2019).
Here, we sought to minimize the confounding effects of maturity on FHB severity by timing Fusarium inoculations and FHB scoring based on anthesis. However, AD was correlated with FHB, and AD-assisted models improved genomic prediction between and within populations, suggesting that our experimental procedures did not fully control the phenological relationship between anthesis timing and FHB symptom development. Previous studies found that modeling phenology and environmental patterns improved genomic prediction for FHB (Moreno-Amores et al., 2020a; Garcia-Abadillo et al., 2023), further demonstrating the importance of phenotyping phenological traits such as AD in FHB trials.
To our knowledge, our study is the first to (a) model both FHB-correlated traits and Rht-D1 together as covariates in genomic prediction for FHB resistance in a (b) harmonized dataset of diverse FHB trials from both public and private breeding programs. Although the phenotypic data generated by the partners of the WheatSustain consortium differed with respect to the number/timing of FHB observations and trial environmental parameters (location, year, and inoculation method), our methods allowed not only for the harmonization of the dataset across trials but also for moderate to high PA for FHB between breeding programs. For collaborations between breeding companies and/or public institutions, we recommend that cross-population genomic prediction for FHB resistance be aided by the agronomically important and easily measurable traits of plant height and anthesis date and by markers for semi-dwarfing genes.
Data availability statement
The datasets presented in this study can be found in online repositories. The names of the repository/repositories and accession number(s) can be found in the article/Supplementary Material.
Author contributions
LM: Conceptualization, Data curation, Formal analysis, Investigation, Methodology, Project administration, Software, Validation, Visualization, Writing – original draft, Writing – review & editing. DA: Data curation, Writing – review & editing. A-LG: Methodology, Writing – review & editing. AN: Investigation, Project administration, Writing – review & editing. VRN: Investigation, Writing – review & editing. FS: Data curation, Writing – review & editing. MS: Methodology, Writing – review & editing. LH: Funding acquisition, Resources, Writing – review & editing. JH: Investigation, Writing – review & editing. JI-S: Data curation, Funding acquisition, Resources, Writing – review & editing. HK: Investigation, Writing – review & editing. ML: Funding acquisition, Investigation, Project administration, Resources, Writing – review & editing. FL: Investigation, Project administration, Resources, Writing – review & editing. SM: Conceptualization, Data curation, Project administration, Supervision, Writing – review & editing. HB: Funding acquisition, Project administration, Resources, Supervision, Writing – review & editing.
Funding
The author(s) declare financial support was received for the research, authorship, and/or publication of this article. This work was conducted within the framework of the “WheatSustain” project (grant no. 771134), which was supported by the Horizon 2020 SusCrop ERA-NET Cofund Action, part of the Joint Programming Initiative on Agriculture, Food Security and Climate Change. Funding was provided by the Research Council of Norway (grant no. 299615), the German Federal Ministry of Education and Research (grant no. 031B0810), the Austrian Federal Ministry of Agriculture, Regions and Tourism (grant no. 101402), the Spanish Ministry of Education and Vocational Training’s Beatriz Galindo Program (grant no. BEAGAL18/00115), and the Spanish State Research Agency’s Severo Ochoa Program for Centres of Excellence in R&D (grant no. SEV-2016-0672).
Conflict of interest
Authors JH and HK were employed by the company Secobra Saatzucht GmbH. Authors FL, AN, and MS were employed by the company Saatzucht Donau GmbH & CoKG.
The remaining authors declare that the research was conducted in the absence of any commercial or financial relationships that could be construed as a potential conflict of interest.
Publisher’s note
All claims expressed in this article are solely those of the authors and do not necessarily represent those of their affiliated organizations, or those of the publisher, the editors and the reviewers. Any product that may be evaluated in this article, or claim that may be made by its manufacturer, is not guaranteed or endorsed by the publisher.
Supplementary material
The Supplementary Material for this article can be found online at: https://www.frontiersin.org/articles/10.3389/fpls.2024.1454473/full#supplementary-material
Supplementary Figure 1 | Quantile-quantile plots of the expected vs. the observed SNP p-values from GWAS of FHB severity within the German (DEU), Saatzucht Donau (SZD) and WheatSustain training set (WTS) populations.
Supplementary Figure 2 | Linkage disequilibrium plot of chromosome 4D in the German population, with R2 values in the upper diagonal and p-values in the lower diagonal. The region flanked by the significant SNPs from GWAS of FHB severity is outlined in black.
Supplementary Figure 3 | Linkage disequilibrium plot of chromosome 4D in the Saatzucht Donau population, with R2 values in the upper diagonal and p-values in the lower diagonal. The region flanked by the significant SNPs from GWAS of FHB severity is outlined in black.
Supplementary Figure 4 | Linkage disequilibrium plot of chromosome 4D in the WheatSustain training set, with R2 values in the upper diagonal and p-values in the lower diagonal. The region flanked by the significant SNPs from GWAS of FHB severity is outlined in black.
References
Akohoue, F., Koch, S., Plieske, J., Miedaner, T. (2022). Separation of the effects of two reduced height (Rht) genes and genomic background to select for less Fusarium head blight of short-strawed winter wheat (Triticum aestivum L.) varieties. Theor. Appl. Genet. 135, 4303–4326. doi: 10.1007/s00122-022-04219-4
Arruda, M. P., Lipka, A. E., Brown, P. J., Krill, A. M., Thurber, C., Brown-Guedira, G., et al. (2016). Comparing genomic selection and marker-assisted selection for Fusarium head blight resistance in wheat (Triticum aestivum L.). Mol. Breed. 36, 1–11. doi: 10.1007/s11032-016-0508-5
Bradbury, P. J., Zhang, Z., Kroon, D. E., Casstevens, T. M., Ramdoss, Y., Buckler, E. S. (2007). TASSEL: Software for association mapping of complex traits in diverse samples. Bioinformatics 23, 2633–2635. doi: 10.1093/bioinformatics/btm308
Buerstmayr, M., Buerstmayr, H. (2016). The semidwarfing alleles Rht-D1b and Rht-B1b show marked differences in their associations with anther-retention in wheat heads and with fusarium head blight susceptibility. Phytopathology 106, 1544–1552. doi: 10.1094/PHYTO-05-16-0200-R
Buerstmayr, M., Buerstmayr, H. (2022). The effect of the Rht1 haplotype on Fusarium head blight resistance in relation to type and level of background resistance and in combination with Fhb1 and Qfhs.ifa-5A. Theor. Appl. Genet. 135, 1985–1996. doi: 10.1007/s00122-022-04088-x
Buerstmayr, M., Lemmens, M., Steiner, B., Buerstmayr, H. (2011). Advanced backcross QTL mapping of resistance to fusarium head blight and plant morphological traits in a Triticum macha x T. aestivum population. Theor. Appl. Genet. 123, 293–306. doi: 10.1007/s00122-011-1584-x
Buerstmayr, M., Steiner, B., Buerstmayr, H. (2020). Breeding for Fusarium head blight resistance in wheat – Progress and challenges. Plant Breed. 139, 429–454. doi: 10.1111/pbr.12797
Corsi, B., Obinu, L., Zanella, C. M., Cutrupi, S., Day, R., Geyer, M., et al. (2021). Identification of eight QTL controlling multiple yield components in a German multi-parental wheat population, including Rht24, WAPO-A1, WAPO-B1 and genetic loci on chromosomes 5A and 6A. Theor. Appl. Genet. 134, 1435–1454. doi: 10.1007/s00122-021-03781-7
Crossa, J., Pérez-Rodríguez, P., Cuevas, J., Montesinos-López, O., Jarquín, D., de los Campos, G., et al. (2017). Genomic selection in plant breeding: methods, models, and perspectives. Trends Plant Sci. 22, 961–975. doi: 10.1016/j.tplants.2017.08.011
Endelman, J. B., Jannink, J.-L. (2012). Shrinkage estimation of the realized relationship matrix. G3 Genes Genomes Genet. 2, 1405–1413. doi: 10.1534/g3.112.004259
Feldmann, M. J., Piepho, H. P., Knapp, S. J. (2022). Average semivariance directly yields accurate estimates of the genomic variance in complex trait analyses. G3 Genes Genomes Genet. 12, jkac080. doi: 10.1093/g3journal/jkac080
Garcia-Abadillo, J., Morales, L., Buerstmayr, H., Michel, S., Lillemo, M., Holzapfel, J., et al. (2023). Alternative scoring methods of fusarium head blight resistance for genomic assisted breeding. Front. Plant Sci. 13. doi: 10.3389/fpls.2022.1057914
He, X., Singh, P. K., Dreisigacker, S., Singh, S., Lillemo, M., Duveiller, E. (2016). Dwarfing genes Rht-B1b and Rht-D1b are associated with both type I FHB susceptibility and low anther extrusion in two bread wheat populations. PLoS One 11, 1–14. doi: 10.1371/journal.pone.0162499
Heffner, E. L., Lorenz, A. J., Jannink, J. L., Sorrells, M. E. (2010). Plant breeding with genomic selection: Gain per unit time and cost. Crop Sci. 50, 1681–1690. doi: 10.2135/cropsci2009.11.0662
Herter, C. P., Erhard, H., Sonja, E., Viktor, K., Tobias, K., Miedaner, T. (2019). Accuracy of within − and among − family genomic prediction for Fusarium head blight and Septoria tritici blotch in winter wheat. Theor. Appl. Genet. 132, 1121–1135. doi: 10.1007/s00122-018-3264-6
Heslot, N., Jannink, J.-L., Sorrells, M. E. (2015). Perspectives for genomic selection applications and research in plants. Crop Sci. 55, 1–12. doi: 10.2135/cropsci2014.03.0249
Hoffstetter, A., Cabrera, A., Huang, M., Sneller, C. (2016). Optimizing training population data and validation of genomic selection for economic traits in soft winter wheat. G3 Genes Genomes Genet. 6, 2919–2928. doi: 10.1534/g3.116.032532
Isidro y Sánchez, J., Akdemir, D. (2021). Training set optimization for sparse phenotyping in genomic selection: a conceptual overview. Front. Plant Sci. 12. doi: 10.3389/fpls.2021.715910
Jiang, Y., Zhao, Y., Rodemann, B., Plieske, J., Kollers, S., Korzun, V., et al. (2015). Potential and limits to unravel the genetic architecture and predict the variation of Fusarium head blight resistance in European winter wheat (Triticum aestivum L.). Heredity. 114, 318–326. doi: 10.1038/hdy.2014.104
Larkin, D. L., Holder, A. L., Mason, R. E., Moon, D. E., Brown-Guedira, G., Price, P. P., et al. (2020). Genome-wide analysis and prediction of Fusarium head blight resistance in soft red winter wheat. Crop Sci. 60, 2882–2900. doi: 10.1002/csc2.20273
Larkin, D. L., Mason, R. E., Moon, D. E., Holder, A. L., Ward, B. P., Brown-Guedira, G. (2021). Predicting Fusarium head blight resistance for advanced trials in a soft red winter wheat breeding program with genomic selection. Front. Plant Sci. 12. doi: 10.3389/fpls.2021.715314
Liu, Y., Salsman, E., Fiedler, J. D., Hegstad, J. B., Green, A., Mergoum, M., et al. (2019). Genetic mapping and prediction analysis of FHB resistance in a hard red spring wheat breeding population. Front. Plant Sci. 10. doi: 10.3389/fpls.2019.01007
Lu, Q., Lillemo, M., Skinnes, H., He, X., Shi, J., Ji, F., et al. (2013). Anther extrusion and plant height are associated with Type I resistance to Fusarium head blight in bread wheat line “Shanghai-3/Catbird.“. Theor. Appl. Genet. 126, 317–334. doi: 10.1007/s00122-012-1981-9
Meuwissen, T. H. E., Hayes, B. J., Goddard, M. E. (2001). Prediction of total genetic value using genome-wide dense marker maps. Genetics 157, 1819–1829. doi: 10.1093/genetics/157.4.1819
Mirdita, V., He, S., Zhao, Y., Korzun, V., Bothe, R. (2015). Potential and limits of whole genome prediction of resistance to Fusarium head blight and Septoria tritici blotch in a vast Central European elite winter wheat population. Theor. Appl. Genet. 128, 2471–2481. doi: 10.1007/s00122-015-2602-1
Moreno-Amores, J., Michel, S., Löschenberger, F., Buerstmayr, H. (2020a). Dissecting the contribution of environmental influences, plant phenology, and disease resistance to improving genomic predictions for Fusarium head blight resistance in wheat. Agronomy 10, 2008. doi: 10.3390/agronomy10122008
Moreno-Amores, J., Michel, S., Miedaner, T., Longin, C. F. H., Buerstmayr, H. (2020b). Genomic predictions for Fusarium head blight resistance in a diverse durum wheat panel: an effective incorporation of plant height and heading date as covariates. Euphytica 216, 1–19. doi: 10.1007/s10681-019-2551-x
Muñoz, F., Sanchez, L. (2020). breedR: Statistical Methods for Forest Genetic Resources Analysts. Available online at: https://github.com/famuvie/breedR. (Accessed August 9, 2024).
Poland, J., Rutkoski, J. (2016). Advances and challenges in genomic selection for disease resistance. Annu. Rev. Phytopathol. 54, 79–98. doi: 10.1146/annurev-phyto-080615-100056
R Core Team (2020). R: A Language and Environment for Statistical Computing. Available online at: www.R-project.org. (Accessed August 9, 2024).
Rutkoski, J., Benson, J., Jia, Y., Brown-guedira, G., Jannink, J., Sorrells, M. (2012). Evaluation of genomic prediction methods for Fusarium head blight resistance in wheat. Plant Genome 5, 51–61. doi: 10.3835/plantgenome2012.02.0001
Schulthess, A. W., Zhao, Y., Longin, C. F. H., Reif, J. C. (2018). Advantages and limitations of multiple − trait genomic prediction for Fusarium head blight severity in hybrid wheat (Triticum aestivum L.). Theor. Appl. Genet. 131, 685–701. doi: 10.1007/s00122-017-3029-7
Shahinnia, F., Geyer, M., Schuermann, F., Rudolphi, S., Holzapfel, J., Kempf, H., et al. (2022). Genome-wide association study and genomic prediction of resistance to stripe rust in current Central and Northern European winter wheat germplasm. Theor. Appl. Genet. 135, 3583–3595. doi: 10.1007/s00122-022-04202-z
Sneller, C., Ignacio, C., Ward, B., Rutkoski, J., Mohammadi, M. (2021). Using genomic selection to leverage resources among breeding programs: consortium-based breeding. Agronomy. 11 (8), 1555. doi: 10.3390/agronomy11081555
Sodini, S. M., Kemper, K. E., Wray, N. R., Trzaskowski, M. (2018). Comparison of genotypic and phenotypic correlations: Cheverud’s conjecture in humans. Genetics 209, 941–948. doi: 10.1534/genetics.117.300630
Srinivasachary, Gosman, N., Steed, A., Hollins, T. W., Bayles, R., Jennings, P., et al. (2009). Semi-dwarfing Rht-B1 and Rht-D1 loci of wheat differ significantly in their influence on resistance to Fusarium head blight. Theor. Appl. Genet. 118, 695–702. doi: 10.1007/s00122-008-0930-0
Steiner, B., Michel, S., Maccaferri, M., Lemmens, M., Tuberosa, R., Buerstmayr, H., et al. (2019). Exploring and exploiting the genetic variation of Fusarium head blight resistance for genomic − assisted breeding in the elite durum wheat gene pool. Theor. Appl. Genet. 132, 969–988. doi: 10.1007/s00122-018-3253-9
Storey, J. D. (2015). qvalue: Q-value estimation for false discovery rate control. Available online at: https://github.com/jdstorey/qvalue. (Accessed August 9, 2024).
Tekle, S., Lillemo, M., Skinnes, H., Reitan, L., Buraas, T., Bjørnstad, Å. (2018). Screening of oat accessions for fusarium head blight resistance using spawn-inoculated field experiments. Crop Sci. 58, 143–151. doi: 10.2135/cropsci2017.04.0264
Verges, V. L., Brown-guedira, G. L., Van Sanford, D. A. (2021). Genome-wide association studies combined with genomic selection as a tool to increase Fusarium head blight resistance in wheat. Crop Breeding Genet. Genomics. 3 (4), e210007. doi: 10.20900/cbgg20210007
Verges, V. L., Lyerly, J., Dong, Y., Van Sanford, D. A. (2020). Training population design with the use of regional Fusarium head blight nurseries to predict independent breeding lines for FHB traits. Front. Plant Sci. 11. doi: 10.3389/fpls.2020.01083
Winn, Z. J., Lyerly, J. H., Brown-Guedira, G., Murphy, J. P., Mason, R. E. (2023). Utilization of a publicly available diversity panel in genomic prediction of Fusarium head blight resistance traits in wheat. Plant Genome 16, 1–18. doi: 10.1002/tpg2.20353
Yu, J., Pressoir, G., Briggs, W. H., Bi, I. V., Yamasaki, M., Doebley, J. F., et al. (2006). A unified mixed-model method for association mapping that accounts for multiple levels of relatedness. Nat. Genet. 38, 203–208. doi: 10.1038/ng1702
Zhang, J., Gill, H. S., Brar, N. K., Halder, J., Ali, S., Liu, X., et al. (2022). Genomic prediction of Fusarium head blight resistance in early stages using advanced breeding lines in hard winter wheat. Crop J. 10, 1695–1704. doi: 10.1016/j.cj.2022.03.010
Keywords: wheat, Fusarium head blight, genomic prediction, trait covariates, Rht-D1, GWAS, GBLUP
Citation: Morales L, Akdemir D, Girard A-L, Neumayer A, Reddy Nannuru VK, Shahinnia F, Stadlmeier M, Hartl L, Holzapfel J, Isidro-Sánchez J, Kempf H, Lillemo M, Löschenberger F, Michel S and Buerstmayr H (2024) Leveraging trait and QTL covariates to improve genomic prediction of resistance to Fusarium head blight in Central European winter wheat. Front. Plant Sci. 15:1454473. doi: 10.3389/fpls.2024.1454473
Received: 25 June 2024; Accepted: 16 August 2024;
Published: 04 October 2024.
Edited by:
Yusheng Zhao, Leibniz Institute of Plant Genetics and Crop Plant Research (IPK), GermanyReviewed by:
Harsimardeep Gill, University of Minnesota Twin Cities, United StatesWentao Zhang, National Research Council Canada, Canada
Copyright © 2024 Morales, Akdemir, Girard, Neumayer, Reddy Nannuru, Shahinnia, Stadlmeier, Hartl, Holzapfel, Isidro-Sánchez, Kempf, Lillemo, Löschenberger, Michel and Buerstmayr. This is an open-access article distributed under the terms of the Creative Commons Attribution License (CC BY). The use, distribution or reproduction in other forums is permitted, provided the original author(s) and the copyright owner(s) are credited and that the original publication in this journal is cited, in accordance with accepted academic practice. No use, distribution or reproduction is permitted which does not comply with these terms.
*Correspondence: Laura Morales, bGF1cmEubW9yYWxlc0BzbHUuc2U=