- 1Department of Microbiology, Abdul Wali Khan University Mardan, Mardan, Khyber Pakhtunkhwa, Pakistan
- 2Centre of Biotechnology and Microbiology, University of Swat, Swat, Khyber Pakhtunkhwa, Pakistan
- 3Department of Environmental Sciences, Kohat University of Science and Technology, Kohat, Khyber Pakhtunkhwa, Pakistan
- 4Department of Microbiology, Hazara University, Hazara, Khyber Pakhtunkhwa, Pakistan
- 5Department of Biology, University of Haripur, Haripur, Khyber Pakhtunkhwa, Pakistan
Taphrina deformans is a plant-pathogenic fungus and a responsible agent for causing peach leaf curl disease. Taphrina deformans affects peach fruit production and contributes to global economic losses. Commercial fungicides may provide temporary relief; however, their overuse resulted in adverse environmental consequences as well as led to drug-resistant strains of T. deformans. Therefore, the discovery of novel drug targets for the future synthesis of antifungal drugs against Taphrina deformans is needed. Here we studied Taphrina deformans by computational proteomics approaches. The whole genome and proteome of T. deformans were subjected to subtractive proteomics, high-throughput virtual screening, and molecular dynamic simulations. We employed subtractive proteomics analysis of 4,659 proteins extracted from UniProtKB database; after filtering out homologous and non-essential proteins, we identified 189 essential ones, including nine that participated in the crucial metabolic pathways of the pathogen. These proteins were categorized as nuclear (n = 116), cytoplasmic (n = 37), and membrane (n = 36). Of those essential proteins, glutamate–cysteine ligase (GCL) emerged as one promising target due to its essential function for glutathione biosynthesis process which facilitates T. deformans survival and pathogenicity. To validate GCL as an antifungal target, virtual screening and molecular docking studies with various commercial fungicides were carried out to better characterize GCL as a drug target. The data showed strong binding affinities for polyoxin D, fluoxastrobin, trifloxystrobin, and azoxystrobin within the active site of GCL. Polyoxin D showed a strong affinity when the measured docking score was at -7.34 kcal/mol, while molecular dynamics simulations confirmed stable interactions (three hydrogen bonds, two hydrophobic bonds, and one salt bridge interaction), supporting our findings that GCL represents an excellent target for antifungal drug development efforts. The results showed that GCL, as an innovative target for future fungicide designs to combat T. deformans infections, provides an avenue toward creating more effective peach leaf curl disease treatments while mitigating environmental harm caused by its current use.
1 Introduction
Taphrina deformans (T. deformans), a prevalent plant pathogen, infects peach trees, causing the very well-known peach leaf curl disease (PLCD). Peach leaf curl is a globally important disease in which nectarines, peaches, and sometimes other stone fruits’ leaves are affected, such as those of apricots and almonds (Pecknold, 2015b). The fungus T. deformans is phylogenetically classified in subphylum Taphrinomycotina, a basal lineage of the phylum Ascomycota that includes fission yeast and members of the genus Pneumocystis. The genus T. deformans is one of the most extensively studied species because it is the most widespread plant pathogen, infecting the cultivars of nectarine and peach (Cissé et al., 2013). PLCD causes $2.5 to $3 million losses annually in the United States (Chester, 1947). In northern Italy, it can affect 60% to 90% of shoots, representing an important threat to the host tree (Kern and Naef-Roth, 1975). The severity of the disease as well as the resulting economic impact on yield can vary based on the microclimate as well as the different resistance responses of selected cultivars (Rossi et al., 2007).
The fungus induces a fast and spontaneous proliferation of the developing cells at the leaf margins, resulting in a curled, puckered, and blurred shape. The color of the leaves also varies, from variants of yellow and green to brown, purple, and pink. On the surface of the leaves, spores are formed as leaves mature, causing a dusty look (Kurtzman et al., 2011). T. deformans enter the leaves’ cells through the stomata (Svetaz et al., 2017). The mycelial growth of fungus in the stomata and interstitial spaces of leaves effects the metabolic activity of the plant (Kolattukudy, 1985). In comparison, the interface between the leaf cell wall and fungus is changed to facilitate fungal nutrition (Bassi et al., 1984). During the infection, changes in the cell host anatomy were also identified (Giordani et al., 2013). When cold and moist conditions are persistent during bud growth, the probability of extreme leaf curl outbreaks is high (Giosuè et al., 2000).
Pathogenicity in Taphrina deformans, the causal agent of peach leaf curl, is a multifaceted process that involves a complex interplay of genes and metabolic pathways. These virulence genes belong to cutinase activity, fat metabolism, and other oxidative stresses, favoring the pathogenesis of T. deformans in peach plants. The genes enable the fungus to adapt and thrive within its host environment. Research indicates that the synthesis of chitin, a critical component of the fungal cell wall, is essential to maintain cell integrity and facilitate successful infection. Genes that regulate chitin production and modification are therefore pivotal in the pathogenicity of this fungus (Tsai et al., 2014). In terms of fungal management, several fungicides are employed to control T. deformans. Remarkably, certified bio-fungicides like polyoxin D target chitin synthase directly compromise the fungus’ ability to maintain its cell wall structure. Additionally, strobilurin fungicides such as fluoxastrobin, trifloxystrobin, and azoxystrobin inhibit the mitochondrial cytochrome bc1 complex, disrupting ATP production and ultimately leading to fungal cell death. These mechanisms highlight the importance of targeting both structural components, like the fungal cell wall, and energy production pathways in the development of effective fungicides against T. deformans (Tsai et al., 2014).
The genome of T. deformans has been sequenced, revealing approximately 5,735 protein-coding genes, many of which are implicated in plant cell wall degradation, secondary metabolism, and the biosynthesis of plant hormones, all of which contribute to its virulence and pathogenicity. In addition, the presence of drug detoxification enzymes in its genome suggests that T. deformans can develop resistance to fungicides, complicating control efforts (Cissé et al., 2013). Knowing the genetic and biochemical pathways involved in the pathogenicity of T. deformans is crucial for the development of targeted management strategies and novel fungicides that can effectively mitigate the impact of this significant agricultural pathogen (Maniatis et al., 2024).
Understanding the molecular basis of pathogens that threaten agriculture, like Taphrina deformans, and their resistance mechanisms against fungicides is crucial in combatting fungal pathogens. Widely utilized strategies include a single spray in early spring that can effectively combat the disease due to the monocyclic nature of this pathogen (Fitzpatrick, 1934). Spraying fungicides, such as Chlorothalonil, Bordeaux, and copper-based products, is able to prevent PLCD by killing fungal spores, thus preventing the spread of infection (Gogorcena Aoiz et al., 2020; La Torre et al., 2018). The fungicides are sprayed twice a year in spring and autumn; however, fall season is mostly deemed suitable for effective control (Thomidis et al., 2018). The excessive use of fungicides has adverse effects on the environment. Furthermore, fungal pathogens developed resistance against commercial pesticides (Zhang et al., 2015). Currently, the scientific community is trying to discover and develop new drug and drug targets in fungal pathogens. In this study, we have identified novel drug targets in T. deformans and characterized them with commercial fungicide using in silico methodology.
Furthermore, due to the extensive and long-term use of fungicides, the pathogens develop resistance against registered ones (Zhang et al., 2015). Therefore, the use of new fungicides against novel targets, which can emerge as effective alternatives to the fungicides currently used, is important. Our research is focused on glutamate–cysteine ligase (GCL), an essential enzyme involved in glutathione biosynthesis that plays an essential role in managing oxidative stress—an integral factor of pathogen viability directly influencing its ability to infect and damage the host plant. Traditional fungicides like polyoxin D and azoxystrobin target this essential protein, disrupting essential metabolic pathways. In this study, a more detailed examination of this interaction is being undertaken in order to validate GCL as a potential new drug target, potentially leading to more effective fungicidal strategies.
To identify the drug target, the proteome of T. deformans was subjected to subtractive proteome analysis; the non-homologous and essential proteins (uncharacterized) were identified against the Prunus persica proteome. Moreover, virtual screening and molecular dynamics (MD) simulations, which are efficient computational biology strategies, were used to identify inhibitory compounds and reaffirm their stability. We identified glutamate–cysteine ligase (GCL) as a target for antifungal drugs by virtual screening, molecular docking simulations, and protein–protein and protein–drug interactions. To our knowledge, this is the first report that shows GCL as a target for future drug development against T. deformans. The docking results show strong binding and stability of the GCL active site with polyoxin D, fluoxastrobin, trifloxystrobin, azoxystrobin, vincozolin, and propiconazole. These results validate that GCL could be a novel target to control the growth of T. deformans and PLCD.
2 Materials and methods
2.1 Retrieval and identification of uncharacterized proteins
The whole protein contents of T. deformans were downloaded from the UniprotKB database (https://www.uniprot.org/) that comprised of 4,659 protein sequences (Consortium, 2019). The uncharacterized proteins of T. deformans were identified for further analysis. The graphical workflow of methodology is illustrated in Figure 1. A list of the tools used in the study is provided in Supplementary Table S1.
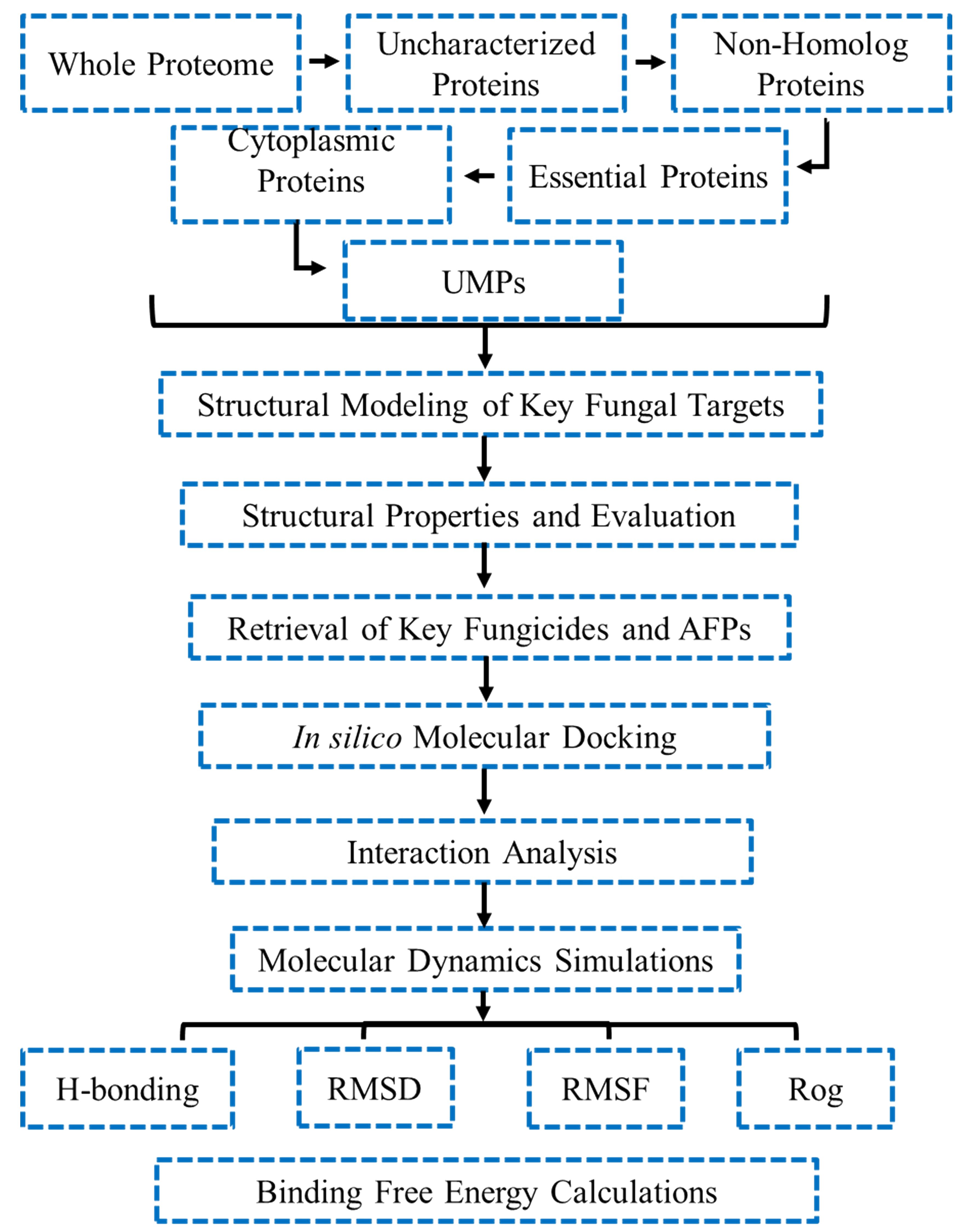
Figure 1. Detailed methodological workflow of the study. UMP, unique metabolic pathways; RMSD, root mean square deviation; RMSF, root mean square fluctuation; ROG, radius of gyration.
2.2 Identification of paralogous sequences
The selected uncharacterized proteins were subjected to the Cluster Database at High Identity with Tolerance CD-HIT server, a cluster database at high identity with tolerance (http://weizhong-lab.ucsd.edu/cdhit-web-server/cgi-bin/index.cgi) for the identification and subsequent removal of paralogous proteins. Paralogous proteins are duplicates that arise during evolution (Li and Godzik, 2006). Redundant proteins that are duplicated or repeated proteins that are irrelevant were considered as targets for a potential fungicide. Generally referred to as paralogous, these proteins have no specific target-based details, so it was convenient to exclude them prior to analysis (Ahmad et al., 2022). For the CD-HIT analysis, a threshold value of 80% sequence identity was deemed to be paralogous in nature (Li and Godzik et al., 2006).
2.3 Non−homology analysis against Prunus persica
The non-paralogous proteins were searched for non-homology against the whole proteome of Prunus persica. NCBI-BLASTp search (https://blast.ncbi.nlm.nih.gov/Blast.cgi?PAGE=Proteins) against the proteome of Prunus persica was carried out with an e-value 0.0001 and bit score threshold >100 for the identification of proteins that are non-homologous to the host (Gul et al., 2020). It is an essential step in subtractive proteomics analysis that ensures that the fungicide will not damage the host since the target protein has no similarity to the host proteins.
2.4 Identification of essential proteins
The non-homologs were subjected to the identification of essential proteins that play a key role in the survival of the pathogen by searching the Database of Essential genes (http://www.essentialgene.org/). The e-value was set to 0.0001 and bit score >100 to classify the essential proteins (Zhang et al., 2004).
2.5 Sub−cellular localization
The non-homologous and essential uncharacterized proteins are divided into two categories depending on protein localization: cytoplasmic proteins that can better serve as candidates for fungicide target and non-cytoplasmic proteins. Localization of the essential proteins was performed by using CELLO2GO server (http://cello.life.nctu.edu.tw/cello2go/) (Yu et al., 2006). CELLO2GO employs the BLAST algorithm to find homologous sequences, which are GO annotations in a search database used in the work modified from UniProtKB/SwissProt. The cytoplasmic proteins were set forth for further analysis. The confidence score for the target proteins was set from 0 to 1, where 0 indicates low clustering and 1 corresponds to the likelihood of presence in the desired cluster.
2.6 Pathway analysis
Pathway analysis was carried out for selected cytoplasmic proteins using KEGG database. To identify the metabolic pathway of non-homologous proteins in T. deformans, Kyoto Encyclopedia of Genes and Genomes (KEGG) or Genome Database (https://www.genome.jp/kegg/kaas/) was used (Moriya et al., 2007). Similarly, a metabolic pathway study of the host was also conducted. A manual comparison was carried out using the KAAS server for qualitative insights into the similarity of pathogen–host metabolic pathways. Finally, proteins have been distinguished according to their corresponding role in the metabolic pathways present only in the pathogen and absent in the host; the workflow of this study is shown in Figure 2.
2.7 Homology modeling
The 3D model is not accessible within the Protein Data Bank (PDB) for the hypothetical protein due to the lack of clarity in the descriptions of conserved areas. Thus, homology modeling was used to construct this 3D model. Phyre2 server was utilized for homology modeling of the new target protein for fungicides (Kelley et al., 2015). The concept of homology modeling is to create 3D models of protein structures through referring to the templates of relatives of the same family. The Phyre2 server is based on the creation of 3D models of proteins that evaluate proteins based on template models of particular protein families, the structures of which nuclear magnetic resonance (NMR) spectroscopy or X-ray is able to solve in a laboratory environment. Phyre2 employed sophisticated remote homology detection techniques to create 3D models.
2.7.1 Tertiary structure prediction and validation of glutamate–cysteine ligase
The primary amino acid sequence of glutamate–cysteine ligase (GCL), consisting of 647 amino acids, was subjected to tertiary structure prediction using the Phyre2 web-based modeling server. The sequence was input into Phyre2, and structural modeling was carried out by utilizing multiple templates (Saccharomyces cerevisiae, Salmonella Typhimurium, and Escherichia coli). The modeled structure was generated with 90% confidence level, indicating reliable structural accuracy. The COFACTOR server was used to predict the active site of the modeled protein according to Kelley et al. (2015).
2.8 Validation of protein model
The Phyre2-constructed model was subjected to 3D protein model validation. ERRAT and Ramachandran plot evaluation are widely used for structural assessment. Evaluation of the protein models was carried out to determine if the model is correctly configured (Khan et al., 2019, 2022). In general, structure validations evaluate the acceptable and not acceptable conformations of the models. The validation of most structures seeks to track the R-value and resolution as a greater resolution indicates a higher precision of molecular structures.
ProSA (Protein Structure Analysis) is a widely used method comprising a diverse user base and is mainly used to analyze and validate the predicted protein mode (Wiederstein and Sippl, 2007). ProSA specifies the analysis of X-ray and NMR spectroscopy. The errors in protein structure were recognized by the server to identify the regions and promote the method of interpretation. Procheck carries out protein model validation through the Ramachandran plot (Laskowski et al., 1993). The analysis was carried out to classify the amino acids of the protein models within the preferred and disallowed regions. The high-quality model should show a cumulative score of more than 90% for amino acids in the centralized and approved regions. The ERRAT plot was utilized to verify the protein models by producing the “overall performance factor” of the non-bonded atomic interactions (Dym et al., 2012). This step evaluates the protein 3D model generated utilizing homology modeling as well as the higher score model representing high-quality models.
2.9 High-throughput virtual screening
Fungicides were virtually screened using AutoDock Vina against the binding sites of a protein. The identified binding sites have features about the degree of exposure, enclosure, hydrogen bonding, size, tightness, hydrophobicity, linking site points, and hydrophilic nature. Firstly, the complete database was screened using AutoDock Vina, and then finally, to confirm the final hits for the best scoring compounds, induced-fit docking (IFD) was done through 64 exhaustiveness. For IFD AutoDockFR, AutoDock with flexible receptors (ADFR) was done (Ravindranath et al., 2015). It utilizes the AutoDock4 scoring characteristic to lower the internal electricity of the receptor. It also handles the side chain of the receptor conformational optimization of up to 14 unique facet chains that enhance the docking rate. AutoDockFR has better accuracy over AutoDock Vina in skip-validation docking, and the speed of docking is much more efficient.
2.10 Molecular dynamics simulation of protein–ligand complexes
In order to perform all-atoms MD simulation, Amber18 package was used for top hits from in-house fungicide database (Case et al., 2005). The topologies of drug small molecules were predicted using an antechamber module, while for complex simulations, the Amber general force field (GAFF) and ff14SB force fields were employed. A TIP3P container of water and Na+ counter ions have been used to solvate and neutralize each device eventually. The energy minimization of systems was carried out in two stages, followed by heating and equilibration. The Particle Mesh Ewald (PME) algorithm is used to quantify lengthy-range electrostatic interactions (Price and Brooks III, 2004). A 1.4-nm cutoff value was set for Van der Waals interactions as well as for columbic interactions of short range. Langevin thermostat was employed to temperature constant at 300 K, whereas for pressure control, Berendsen barostat was considered. A time step of 2fs and total simulation time of 50 ns for each complex were performed. The dynamics, stability, and other features of the ligand–protein complexes were evaluated by using CPPTRAJ and PTRAJ within AMBER software suite (Roe and Cheatham III, 2013).
2.11 Calculations of binding free energy
For all of the protein–ligand complexes, the binding free energy was calculated using the MMPBSA.PY script (Python-based script used to perform molecular mechanics Poisson–Boltzmann surface area) by considering 500 snapshots. This method is widely used to estimate the total binding energy (TBE) of various ligands, as referenced in several studies. Binding free energy is computed using the equations below (Hou et al., 2012; Miller III et al., 2012; Sun et al., 2014b; Chen et al., 2016). Different studies broadly use this unfastened energy calculation approach to estimate the TBE of various ligands (Wang et al., 2018).
ΔGbind: overall free binding energy—the energy change associated with the binding of a ligand to a receptor,
ΔGcomplex: free energy of the complex or the energy state of the receptor–ligand complex
ΔGreceptor: free energy of the receptor or the energy state of the receptor alone (without the ligand)
ΔGligand: free energy of the ligand or the energy state of the ligand alone (without the receptor)
Equation 2 was used to calculate the specific energy term contribution to the total free energy:
G: total free energy
Gbond: bonding energy (or bond stretching energy)
Gele: electrostatic energy (or Coulomb energy)—energy due to electrostatic interactions between charged particles
GvdW: van der Waals energy (or dispersion energy)
Gpol: polarization energy—energy associated with the polarization of molecules (e.g., induced dipoles)
Gnpol: non-polar energy (or hydrophobic energy)
This equation accounts for bonded interactions, electrostatic energy, van der Waals forces, polar solvation energy, and non-polar solvation energy, thus providing a comprehensive view of the binding interactions involved.
3 Results
3.1 Retrieval and identification of uncharacterized proteins
The complete proteome of Taphrina deformans (Proteome ID: UP000013776) comprised 4,659 proteins—1,581 of which were identified as uncharacterized proteins. The molecular functions of more than 30% of proteins remain unknown in most organisms; such proteins are referred to as hypothetical or uncharacterized proteins. The functional characterization of such proteins can help us to consider their functions in various metabolic pathways essential for the survival of the pathogen and to classify previously novel fungicide targets in plant pathogens (Shahid et al., 2016). There are several bioinformatics services available for the functional classification of uncharacterized proteins, like databases and software. The functions of uncharacterized proteins in different microbial pathogens have been successfully annotated using these resources, including Borrelia burgdorferi (Khan et al., 2016), Chlamydia trachomatis (Naqvi et al., 2017)], Haemophilus influenza (Shahbaaz et al., 2013), and Vibrio cholerae (Islam et al., 2015), and can be utilized to identify potential fungicide candidates in T. deformans. The subtractive proteomics analysis is graphically illustrated in Figure 2.
3.2 Identification of non-paralogous proteins
By importing the FASTA format of uncharacterized proteins into the CD-HIT server, the non-paralogous proteins were identified. This server implements greedy incremental algorithms to remove redundancy and cluster protein sequences. The proteins were sorted depending on a chosen sequence identity cutoff depending on the requirement. The sequence identity of 80% was chosen as a cutoff to preserve a rigid parameter in this analysis. The entire proteome was clustered, and proteins with 80% identity were identified and analyzed as paralogous. After the CD-HIT suite study, no duplicates or paralogs with more than 80% identity were found (Figure 2). The proteins were set forth for identification and subsequent removal of proteins similar to the host.
3.3 Non−homology analysis against Prunus persica
Uncharacterized proteins were subjected to NCBI-BLASTp search against Prunus persica proteome, with a threshold e-value of 0.0001, to identify the non-homologous sequence. The homologous sequences were identified and ultimately excluded from the study. Only those sequences specific to the pathogen and can be regarded as potential fungicide targets were selected after excluding the homologous sequences. In this step, 1,262 uncharacterized non-homologous proteins were identified (Figure 2).
3.4 Essential proteins for T. deformans survival
The non-homologous proteins of T. deformans against plant proteome was further treated for the identification of essential genes in T. deformans. From the non-homologous sequences, essential proteins were separated with the Blastp technique against the eukaryotic set of the Database of Essential Gene (DEG). We identified a total of n = 244 critical proteins (Figure 2). A gene product’s practical contribution to an organism’s survival and pathogenicity determines how crucial it is. The output of the sequence from the last phase was locally aligned against the Database of Essential Gene in order to determine essentiality (van Vlijmen et al., 2017). Protein alignments linked to an anticipated value of less than 0.0001 were deemed to be more significant hits. Crucial proteins are essential to pathogen survival and could be viewed as a first step toward developing new fungicide targets.
3.5 Subcellular localization
The subcellular locations of these proteins were identified by utilizing the CELLO2GO server. The overall prediction revealed n = 116 proteins in the nuclear region, 37 proteins in the cytoplasm, and 36 proteins in the plasma membrane. Cytoplasmic proteins have a strong possibility of being a suitable fungicide target. The complete results of the CELLO2GO prediction are provided in the pie chart (Figure 3).
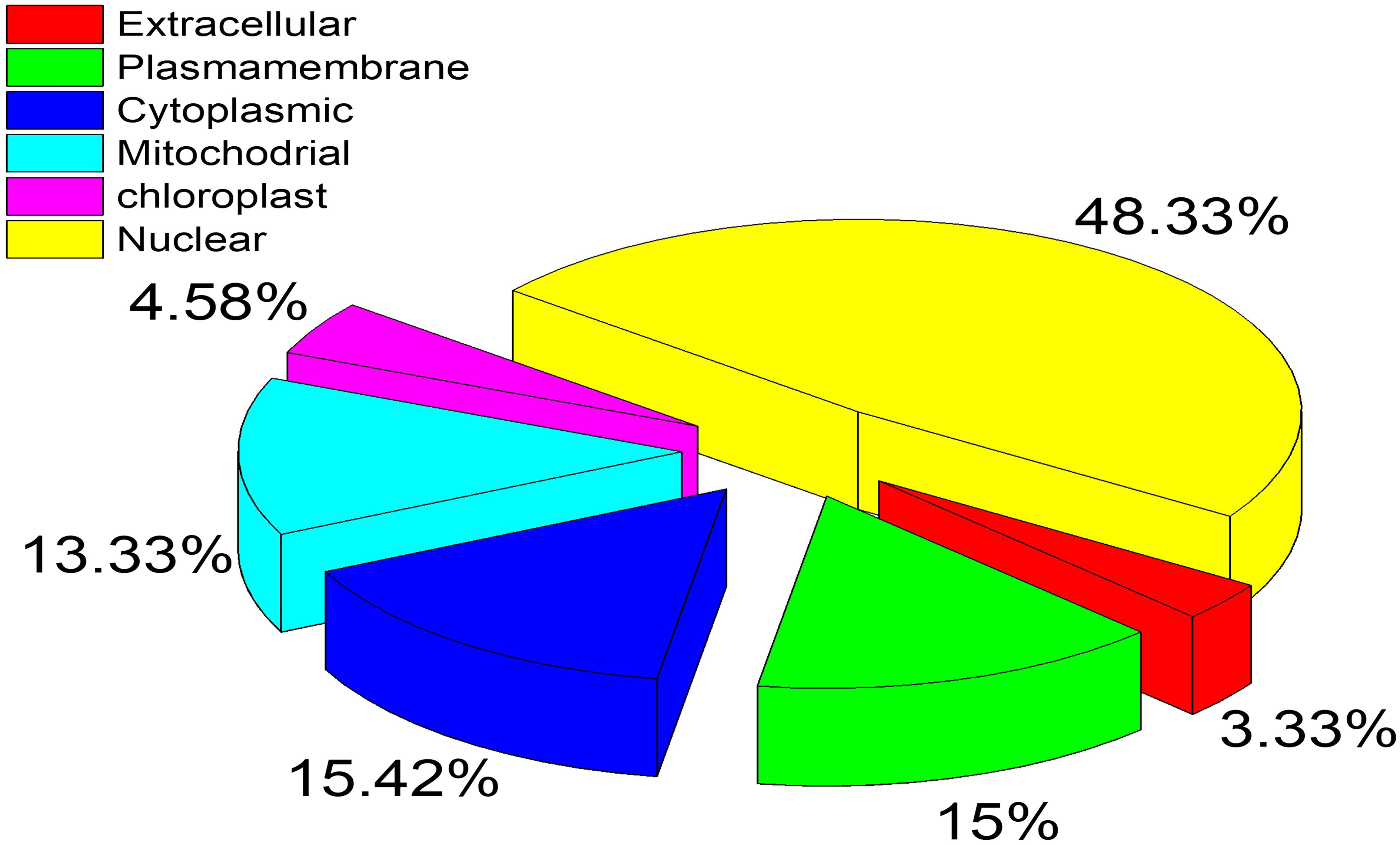
Figure 3. Subcellular location prediction of essential proteins by CELLO2GO server. The respective location is represented by a different color with the percentage of proteins.
3.6 Unique pathway analysis
The 13 uncharacterized proteins that are essential for the survival of T. deformans and located in the cytoplasm were subjected to pathway analysis using the KAAS tool available in the KEGG database. These proteins were classified into distinctive pathways according to their functions and significance in unique metabolic pathways. Not only do KAAS outputs provide convenient metabolic routes but they also have remarkable informational features, such as KO list projects and opportunity pathways of enzymes and Enzyme Commission (EC) numbers. The KO list of T. deformans uncharacterized proteins was compared to host metabolic pathways that revealed nine proteins having key roles in metabolic pathways unique to the pathogen. The unique metabolic pathways (UMPs) are shown in Table 1. The uncharacterized protein glutamate–cysteine ligase (Uniprot ID: R4XJV2) was identified as the most suitable fungicide target. It is involved in the cysteine and methionine metabolism, glutathione metabolism, and ferroptosis pathways. The target protein is involved in step one of the subpathway that synthesizes glutathione from L-cysteine and L-glutamate (Fahey and Sundquist, 1991).
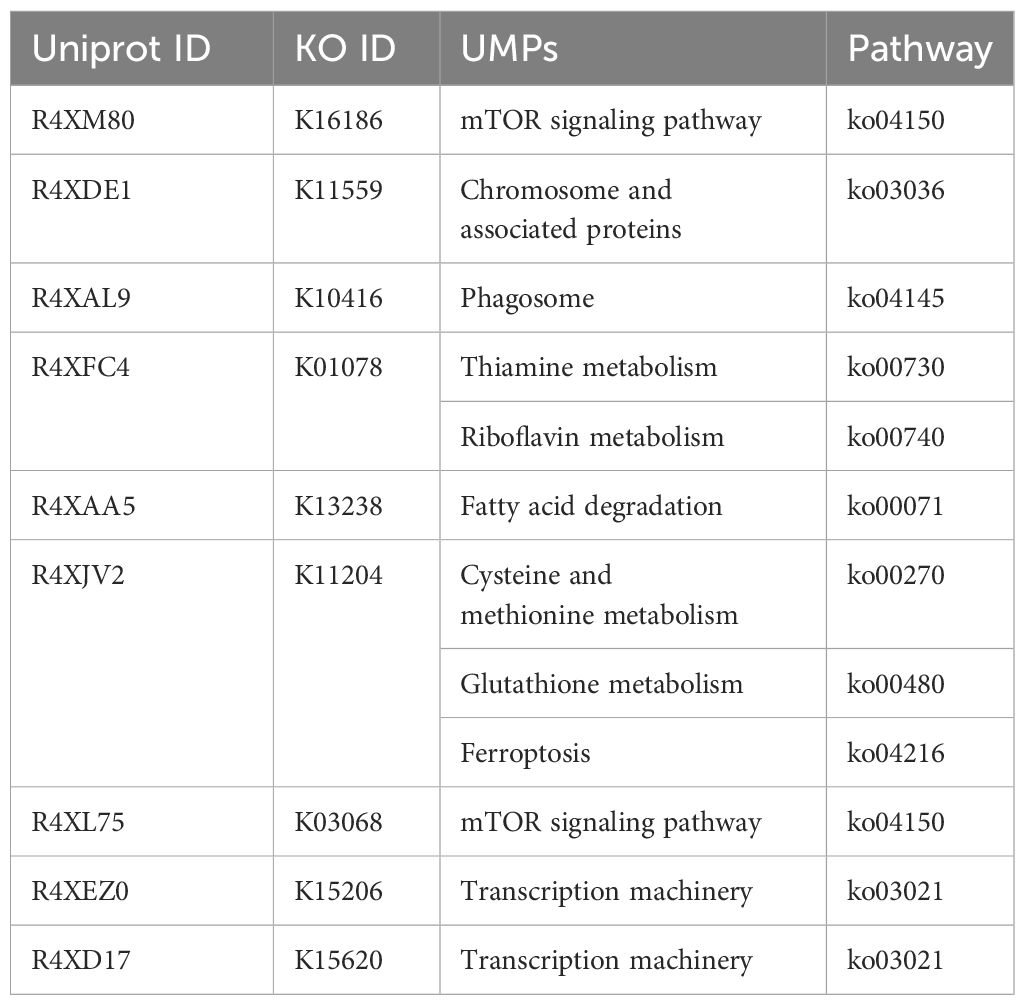
Table 1. Identified unique metabolic pathways (UMPs) in the T. deformans essential proteins located in the cytoplasm.
3.7 Tertiary structure prediction and validation of target protein
The primary amino acid sequence of glutamate–cysteine ligase was processed for 3D structural modeling using Phyre2 web modeling server. A 647-amino-acid-long sequence was modeled with 90% confidence by using multiple templates. The query sequence shares 97% sequence identity with glutamate–cysteine ligase from Saccharomyces cerevisiae and 21% sequence identity with glutamine synthetase of Salmonella Typhimurium and Escherichia coli. The visual analysis shows that the structure has proper folding with uniformly interspersed secondary structural elements. The structure possesses 44% alpha-helices, 10% beta-sheets, and 18% disordered and 8% trans-membrane helices. The protein’s active site was identified using COFACTOR server and revealed residues Glu50, Glu110, Tyr111, His195, Gln272, Ser427, Trp430, Arg434, and Arg451 as active sites. The modeled 3D structure and the binding site residues are shown in Figure 4A as a cartoon and surface representation. Evaluation of the 3D structure was performed using various algorithms, which revealed that most of the residues lie in the favored region while only five residues are in the disallowed region. The ProSA-web (interactive web service for the recognition of errors in the 3D structures of proteins) further confirmed that the Z-score of the modeled structure is -5.71, which reflects the best quality of the 3D structure. Overall, the structural evaluation shows that the structure could be used for further analysis—the evaluation results obtained from different servers as shown in Figures 4B, C.
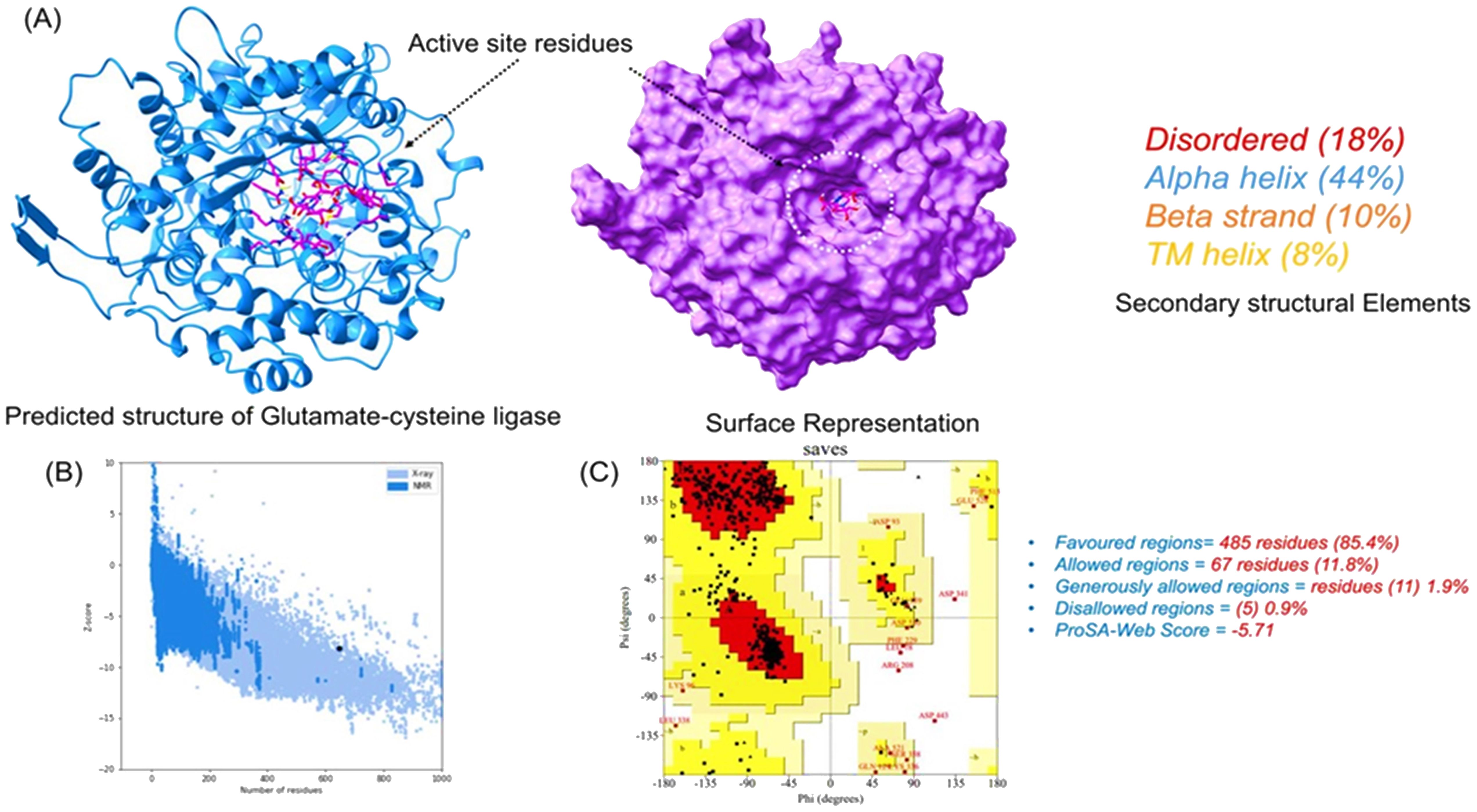
Figure 4. Structural modeling and evaluation of the predicted 3D structure. (A) 3D modeled structure with the active site residues. (B) ProSA-Web results (Z = -5.71), while the Ramachandran plot obtained from SAVES server is given in (C).
3.8 Identification of fungicides as control agents against T. deformans
A computational virtual screening algorithm was employed using PyRx to screen the 31 selected fungicides against the active site of glutamate–cysteine ligase. Residues Glu50, Glu110, Tyr111, His195, Gln272, Ser427, Trp430, Arg434, and Arg451 were confirmed as active site residues that can bind to fungicides. The initial screening results revealed the range of docking from -7.34 to -2.38 kcal/mol. Fungicides with this score greater than -6.0 were subjected to a second round of screening using the induced-fit docking approach, which owns the advantage of efficient conformational optimization for better binding. In the first screening, fungicides, i.e., polyoxin D zinc salt, with this score (-7.34 kcal/mol) were identified as the best fungicide. Among the other best hits, fluoxastrobin (docking score = -6.81 kcal/mol), trifloxystrobin (docking score = -6.49 kcal/mol), azoxystrobin (docking score = -6.37 kcal/mol), pyraclostrobin (docking score = -6.31 kcal/mol), vinclozolin (docking score = -6.18 kcal/mol), and propiconazole (docking score = -6.03 kcal/mol) were identified as the best hits which could potentially inhibit the peach fungus T. deformans by targeting the glutamate–cysteine ligase protein. The docking results along with the names, PubChem IDs, first screening, and induced fit docking scores of the top hits are given in Table 2.
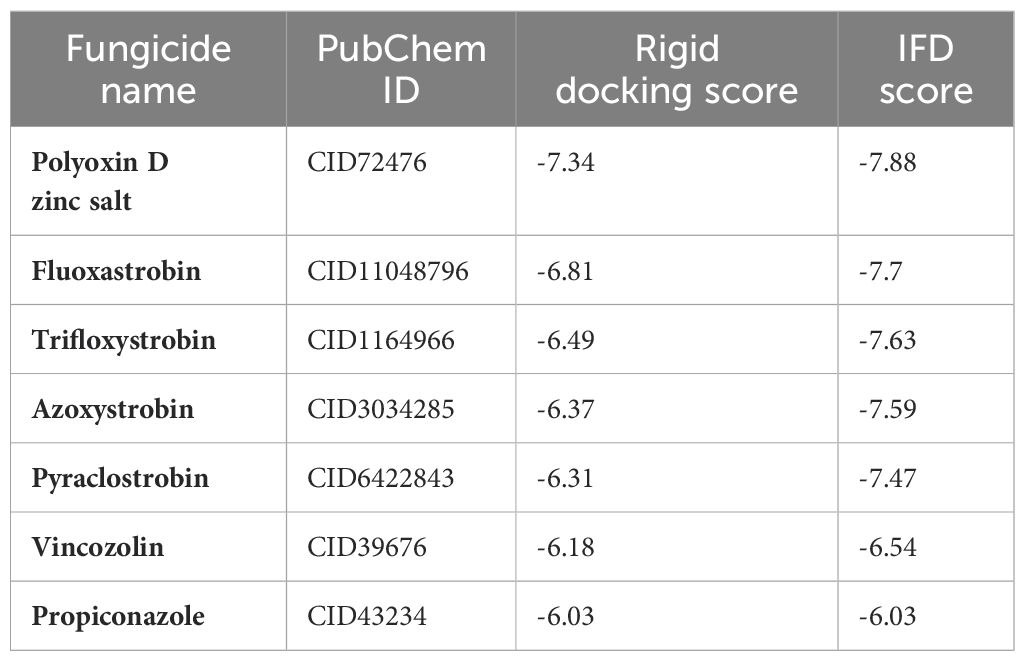
Table 2. Fungicide names, their PubChem ID, virtual screening docking scores, and the induced-fit docking (IFD) scores of top hits.
Next, the top hits were again subjected using the IFD protocol to select strong control agents for T. deformans infection. The docking score range for the IFD protocol was -7.88 to -6.08 kcal/mol (Table 2). Fungicides in the IFD protocol with docking scores greater than -7.50 kcal/mol were selected as the best hits for interaction evaluation and structural–dynamic feature estimation. Among the top hits, polyoxin D zinc salt (with docking score = -7.88 kcal/mol) formed 10 hydrogen bonds, and 23 hydrophobic interactions were reported. Only two hydrophobic interactions were formed by the two arginine active site residues at positions 434 and 451. In the case of the hydrogen bonds, Glu50, His195, Tyr111, His195, Gln272, Ser427, Trp430, Arg434, and Arg451 amino acids were involved (Figure 5A). Unlike the polyoxin D zinc salt, fluoxastrobin established only three hydrogen bonds and two hydrophobic bonds, and a single salt bridge was observed. The interaction pattern revealed that Tyr111, Trp430, and Arg451 are involved in hydrogen bonding interaction, His108 and Tyr350 formed a hydrophobic interaction, and Glu52 established the only salt bridge (Figure 5B).
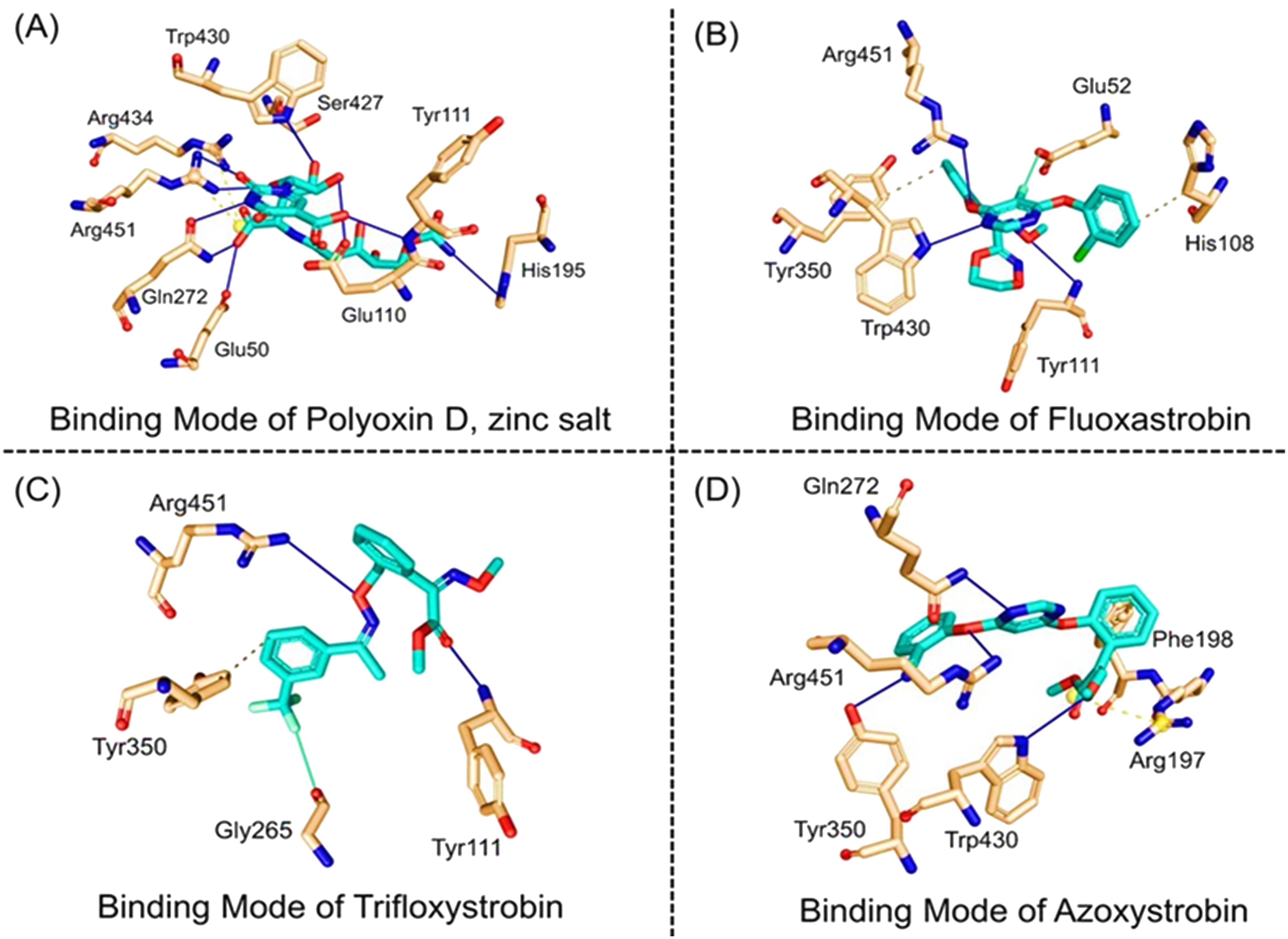
Figure 5. Docking representation of the top hits selected from induced-fit docking (IFD) protocol. (A) 3D interaction pattern of polyoxin D zinc salt, (B) 3D interaction pattern of fluoxastrobin, (C) 3D interaction pattern of trifloxystrobin, and (D) 3D interaction pattern of azoxystrobin.
Similarly, the only two hydrogen bonds in the trifloxystrobin complex (with docking score = -7.63 kcal/mol) were formed by Tyr111 and Arg451. Only a single hydrophobic interaction with Tyr350 and a salt bridge by Gly265 were observed (Figure 5C). The azoxystrobin complex formed four hydrogen interactions, and one hydrophobic interaction with Arg197 was detected (Figure 5D). In conclusion, the results show that the shortlisted fungicides robustly interact with the fungus target and could potentially inhibit the growth of T. deformans in the field. This pattern of the top hits is shown in Figure 5, and the 2D structures of fungicides, PubChem IDs, hydrogen bonding residues, salt bridges, and hydrophobic interactions are shown in Table 3.
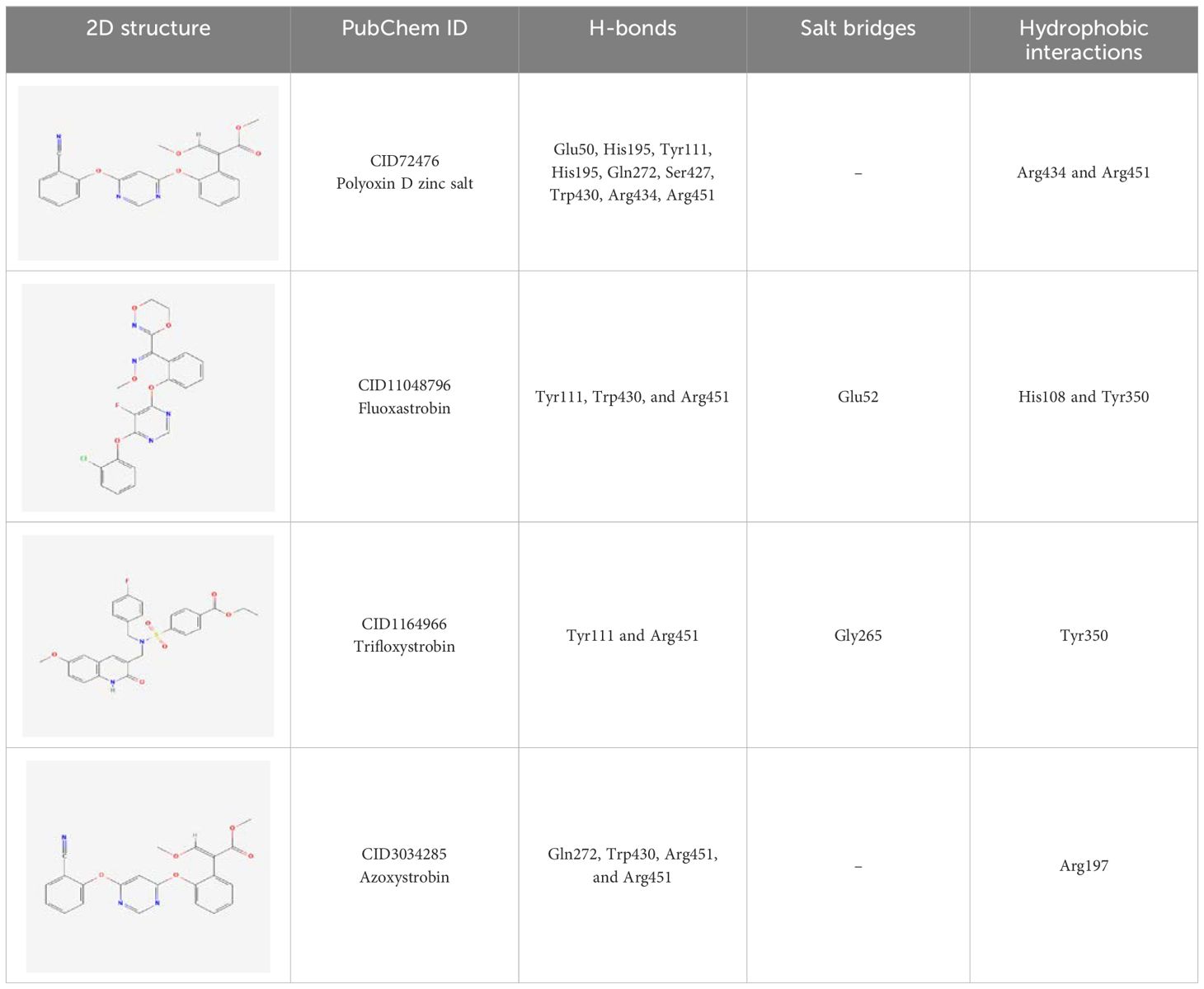
Table 3. 2D structure representation of fungicides, PubChem IDs, hydrogen bonds, salt bridges, and hydrophobic interactions.
3.9 Dynamic stability estimation of the top hits (fungicides)
Two-step fungicide screening approaches shortlisted fungicides, polyoxin D zinc salt, fluoxastrobin, trifloxystrobin, and azoxystrobin as the best hits. The complexes of these top hits were subjected to all-atom molecular dynamics simulation to estimate the thermodynamic stability, residual flexibility, structural compactness, hydrogen bond count, and computations of free energy. Every complex’s thermodynamic stability was determined using the root mean square deviation (RMSD) as a function of time. The RMSDs for each complex demonstrate that throughout the 50ns simulation period, every complex remained stable as shown in Figure 5. No significant structural perturbation was observed in any complex. In the polyoxin D zinc salt complex case, the RMSD reached equilibrium at 3 ns, while a slight deviation between 25 and 30 ns was observed. No deviation from the mean structure was observed during the simulation. The average RMSD for polyoxin D zinc salt was observed to be 2.0 Å. On the other hand, the fluoxastrobin complex was also observed to be very stable, but the average RMSD remained higher than the polyoxin D zinc salt complex. Comparatively, fluoxastrobin remained more stable and reached equilibrium at 2 ns (Figure 6). Similarly, the trifloxystrobin complex also remained stable, and no structural perturbation was observed, but the RMSD value gradually increased over the simulation time. The average RMSD was observed to be 3.0 Å. Furthermore, the RMSD pattern of azoxystrobin and trifloxystrobin, respectively, was observed to be alike. A gradual increase over the simulation time was recorded in both cases; however, a slightly higher average RMSF for azoxystrobin was observed. Consequently, these results show that all of the fungicides stably remained bound in the binding cavity of GCL and thus possess stronger inhibitory properties. The RMSD graphs of each complex are shown in Figure 6.
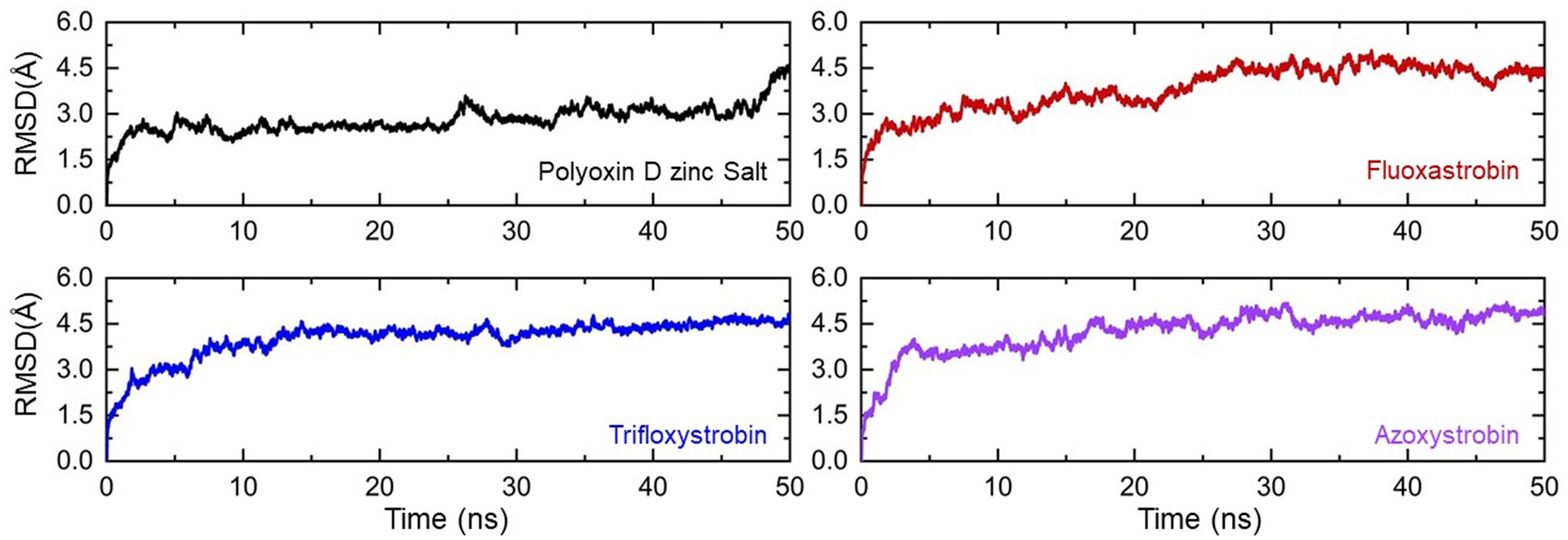
Figure 6. Thermodynamic stability of the top hits calculated as root mean square deviation. Each complex of polyoxin D zinc salt, fluoxastrobin, trifloxystrobin, and azoxystrobin is represented with a different color, respectively.
3.10 Structural compactness evaluation
The compactness of each fungicide-bound complex was then estimated as its radius of gyration (Rg), and this was assessed to identify any binding or unbinding events that occurred during the simulation. All of the fungicides and GCL protein complexes possess a dynamically compact topology, and no significant variations were observed except in the polyoxin D zinc salt complex (Figure 7). The average Rg value for polyoxin D zinc salt was observed to be 24.80 Å.
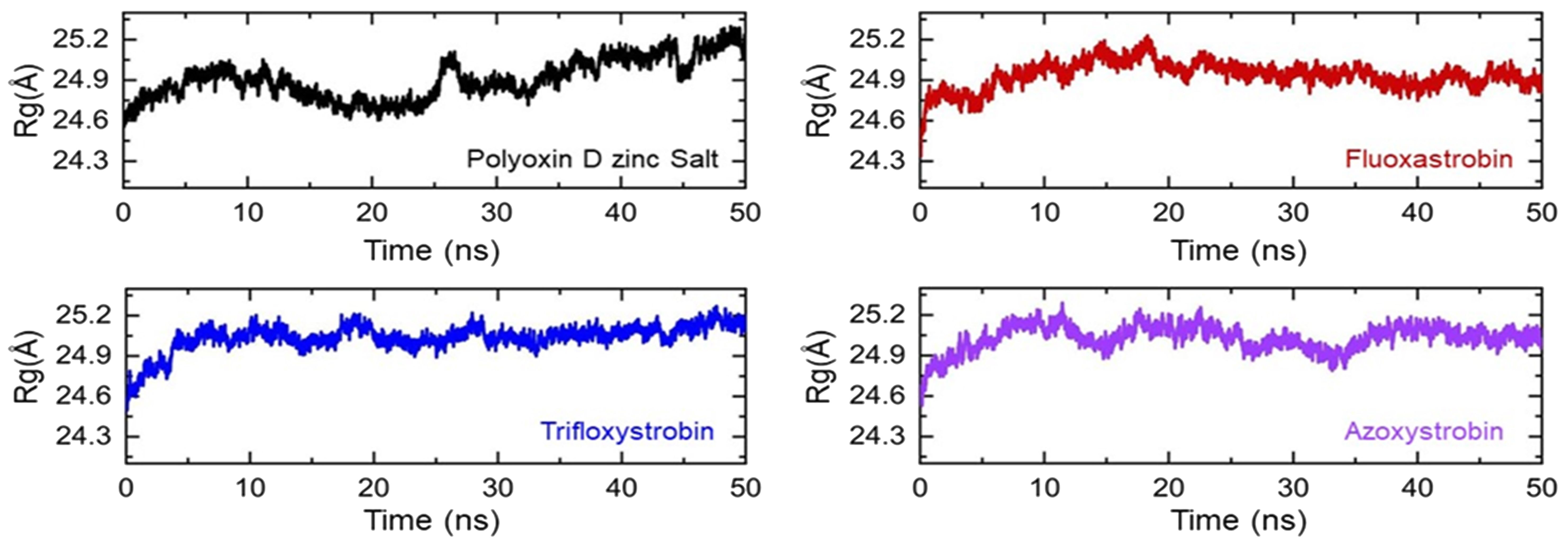
Figure 7. Structural compactness of the top hits calculated as Rg/Rog (radius of gyration). Each complex of polyoxin D zinc salt, fluoxastrobin, trifloxystrobin, and azoxystrobin is represented with a different color, respectively.
During the first 25 ns, no significant deviation was observed; then, the compactness decreased and reached approximately 25.10 Å during the last 25 ns. In the case of the other complexes, i.e., fluoxastrobin, trifloxystrobin, and azoxystrobin, the average Rg value was reported to be 24.90 Å for each complex. In conclusion, the Rg results show that all of the fungicides are stably bound inside the protein’s active site and possess stably binding dynamics. Figure 7 shows the graphical representation of each complex as a function of time.
3.11 Residual flexibility of the fungicide-bound complexes
Residual flexibility plays an imperative role in determining the inhibitory effects, catalysis, and proteins’ conformational switches. Herein the residual flexibility was calculated as root mean square fluctuation (RMSF). The graph given in Figure 8 shows that those regions 75–100, 225–250, 325–350, 500–540, and 555–575 are dynamically more flexible than the other regions. It can also be seen that the binding cavity regions, i.e., 101–175, 251–324, and 355–500, exhibit a lower dynamic fluctuation, which is reduced by the binding of each fungicide. This shows that the binding has affected the residual dynamics by inducing the inhibitory effects. The RMSF graph presenting each complex with different colors is shown in Figure 8.
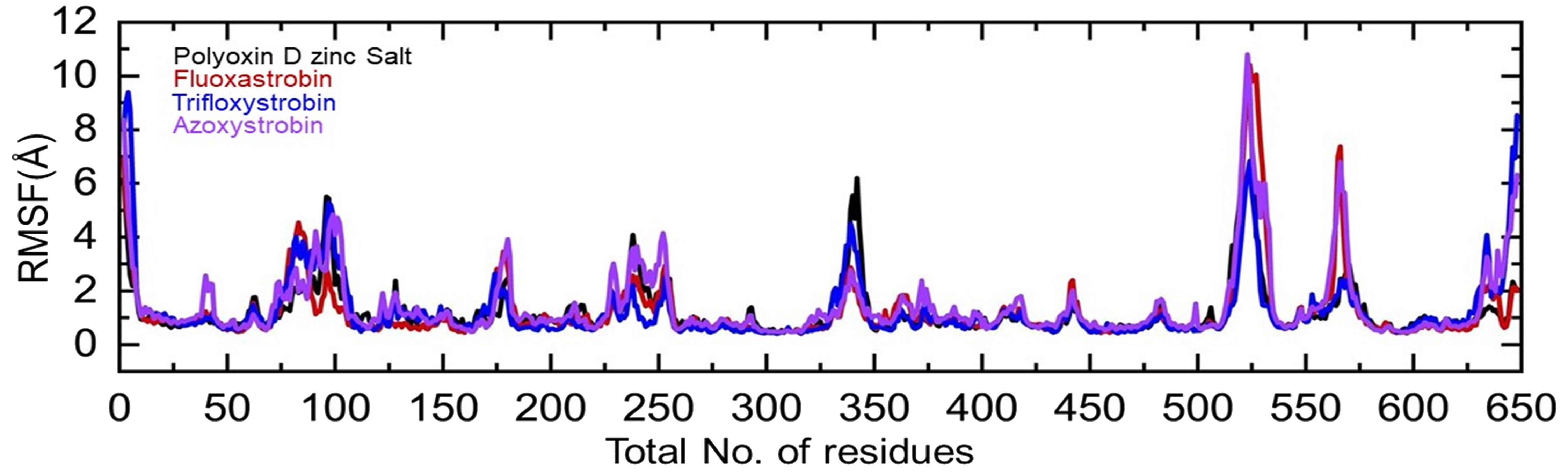
Figure 8. Residual flexibility of the top hits calculated as root mean square fluctuation. Each complex of polyoxin D zinc salt, fluoxastrobin, trifloxystrobin, and azoxystrobin is represented with a different color, respectively.
3.12 Hydrogen bond count
To evaluate the binding efficiency of each fungicide, the total number of hydrogen bonds is the best alternative estimate to reveal the binding robustness. The total number of hydrogen bonds during the simulation varies from frame to frame and provides a better view of the binding differences. Using the simulation time as a function of time, herein the total average number of bonds in the polyoxin D zinc salt complex was observed to be 320, while in fluoxastrobin during the first 10 ns the average number of bonds was more than that in polyoxin D zinc salt; however, the bonds are then reduced, and the average number of bonds was observed to be 310, which was similar in other complexes, i.e., trifloxystrobin and azoxystrobin. In azoxystrobin, the number of bonds gradually decreased after 45 ns and thus specified the unbinding events that occurred during the simulation. This shows that all of these fungicides exhibit a strong interaction cloud for the interactions and inhibition of glutamate–cysteine ligase protein. The hydrogen bond graphs are shown in Figure 9.
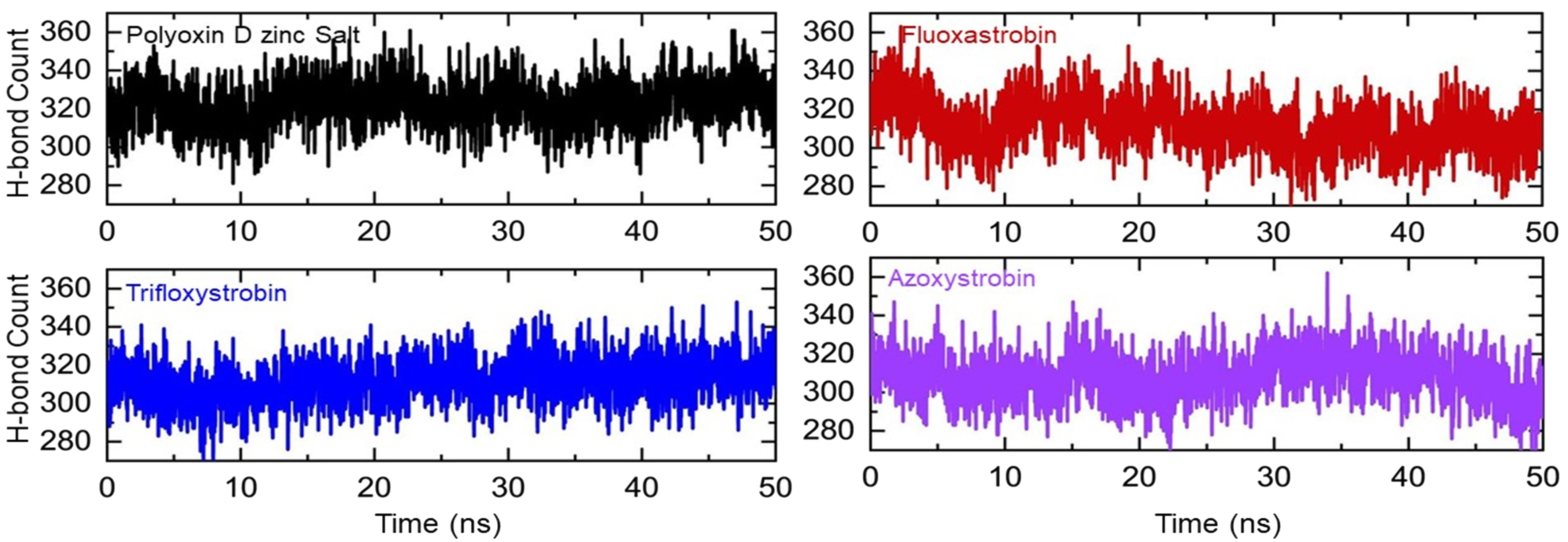
Figure 9. The total number of hydrogen bonds in each complex of polyoxin D zinc salt, fluoxastrobin, trifloxystrobin, and azoxystrobin is represented with a different color, respectively.
3.13 Binding free energy calculations
To connect the dynamic properties with the real-time binding free energy calculations and reveal the nearly experimental affinity of the top hits’ fungicides, molecular mechanics/generalized Born surface area (MM/GBSA) approach was employed. The total binding free energy for polyoxin D zinc salt was reported to be -41.76 kcal/mol; for fluoxastrobin, it was -39.06 kcal/mol; the TBE for trifloxystrobin was -33.77 kcal/mol, and for azoxystrobin, the total binding energy was -35.75 kcal/mol. This shows that these fungicides bind more robustly and block the GDP protein of Taphrina deformans. The other factors such as van der Waal energy (vdW) and electrostatic generalized Born (EGB) are also given in Table 4.
4 Discussion
Plant fungal pathogens are evolving and emerging new strategies to cause infections in plants around the globe (Zeng et al., 2018). This study addresses the growing need for novel antifungal targets due to the resistance developed by pathogens like Taphrina deformans against commonly used fungicides. The extensive use of these chemicals not only contributes to resistance but also poses environmental risks, underscoring the urgency for sustainable alternatives (Pecknold, 2015a). Due to the extensive and long-term use of fungicides, the pathogens developed resistance against widely used fungicides (Feng et al., 2020). To understand fungal pathogenesis and its control, it is important to study the genome and proteome of pathogens (Sammut et al., 2008). Our research utilized genomic and proteomic data to pinpoint essential genes in T. deformans that are promising drug targets because they show little homology with the host, Prunus persica (Luo et al., 2014; Sammut et al., 2008). Out of the whole genome, essential genes of pathogens that do not have a significant homology with the host can serve as potential drug targets (Luo et al., 2014). As the fungal infections’ incidence is increasing gradually, the demand for new antifungal drugs is also increasing. Hence, the key steps in developing a new drug are target identification and validation. To implement bioinformatics tools, it helps to identify, select, and prioritize potential drug targets (Hughes et al., 2011; Yang et al., 2012).
Oxidative stress responses have a crucial role in the virulence and survival of pathogens (Huynh et al., 2003; Mukherjee et al., 2009). Glutathione plays a key role in redox homeostasis and in cellular response to oxidative stress (Sipos et al., 2002). The critical enzyme involved in glutathione biosynthesis is glutamate–cysteine ligase (GCL). GCL is a cytoplasmic ligase considered as an essential protein for the survival and pathogenesis of T. deformans. In this study, we identified a protein, GCL of T. deformans, as a suitable antifungal target by subtractive proteomics approach. This finding is supported by our subtractive proteomics analysis, which also aligns with the methodologies used in previous studies to identify potential drug targets in pathogenic fungi(González-Fernandez et al., 2010; Bencurova et al., 2018; Jha et al., 2020; Shahid et al., 2020). The GCL 3D structure was modeled by comparative modeling that shows 90% confidence and 97% sequence identity with glutamate–cysteine ligase of Saccharomyces cerevisiae as shown in Figure 4. Comparative modeling is the most successful and accurate method to identify the structure of evolutionarily related proteins (Errami et al., 2003; Choong et al., 2011). However, in the previous literature, we could not find any data that shows GCL as an antifungal target. Furthermore, GCL was studied for active site identification, docking, molecular dynamic simulations, and free energy binding calculations against antifungal compounds.
The active sites of GCL were identified as Glu50, Glu110, Tyr111, His195, Gln272, Ser427, Trp430, Arg434, and Arg451 residues (Figure 4). In a structure-based drug discovery, the identification of active sites on a target protein has a great importance (Harigua-Souiai et al., 2015). The fungicides that interact by blocking the active sites have a significant effect on inhibiting the function of proteins (Aamir et al., 2018).
In this study, docking and simulation of GCL with fungicides were performed to find out the top hits by induced fit docking protocol, with those that scored greater than -7.50 kcal/mol selected as the best candidates (Table 2). The efficacy of fungicides like polyoxin D, fluoxastrobin, trifloxystrobin, and azoxystrobin was assessed through rigorous computational analyses, which showed a high binding affinity of these chemicals toward GCL, indicating their potential as effective fungicides. These fungicides were selected for their role in inhibiting vital functions within the pathogen, analogous to how azoxystrobin impedes mitochondrial respiration. Among the fungicide candidates, poloxin D’s score was the highest (-7.88 kcal/mol), and that of azoxystrobin was the lowest (-7.59 kcal/mol) as shown in Table 2. Polyoxin D is an antibiotic that is involved in cell wall synthesis inhibition as reported earlier (Endo et al., 1970). It has been studied that the use of polyoxin D against Botrytis cinerea, causal agent of gray mold on strawberry, has effective results against it (Dowling et al., 2016). Azoxystrobin inhibits mitochondrial respiration as it blocks the movement of electrons in the mitochondrial bc1 complex for mitochondrial electrons (Herms et al., 2002; Lu et al., 2019). The family of strobilurin fungicides is widely used around the world to combat white mold, rot, early and late leaf spot, rusts, and rice blast (Feng et al., 2020).
The MD simulations and free energy calculation of antifungal compounds polyoxin D zinc salt, fluoxastrobin, trifloxystrobin, and azoxystrobin with GCL showed that all of the complexes were stable at 50-ns simulation time (Figure 6). The average root mean square deviation for polyoxin D zinc salt was 2.0 Å, while for fluoxastrobin, trifloxystrobin, and azoxystrobin it was 3.0 Å. This shows that polyoxin D has a higher binding affinity to GCL than the other compounds. It has been reported in the previous study that the use of fungicides against Fusarium wilt, the two fungicides (oxathiapiprolin and famoxadone) with a potential energy of −113,166.16 and −112,628.96 kcal/mol, respectively, was revealed best via molecular docking and virtual screening. The stability of the protein–fungicide docked complexes was measured at 50-ns MD simulations. The average RMSD for the oxathiapiprolin was 2.49 and 2.42 Å, while for famoxadone it was 2.83 and 1.20 Å, respectively (Laskowski et al., 1993).
We evaluated the compactness as the radius of gyration (Rg) of each fungicide with GCL as given in Figure 7. For the structural compactness of fungicides with GCL active sites, the average Rg value for polyoxin D zinc salt was observed as 24.80 Å, while for fluoxastrobin, trifloxystrobin, and azoxystrobin it was 24.90 Å. Rg is considered as one of the essential parameters to calculate the binding stability of complexes inside the cavity (Khan et al., 2021a). This shows that all of the four fungicides can bind strongly to GCL. The strong binding affinity of fungicides with GCL can be confirmed by total hydrogen binding and total binding free energy calculations.
The total number of hydrogen bonds in the polyoxin D zinc salt complex was n = 320, while for fluoxastrobin, trifloxystrobin, and azoxystrobin this was n = 310 (Figure 8). Hydrogen bonding is a crucial stabilizing factor in the formation of biological complexes (Khan et al., 2021b). Polyoxin D has the highest number of hydrogen bonds than the other fungicides and can bind more firmly to GCL. The total binding free energy for all four fungicides was performed by MM/GBSA calculation (Errami et al., 2003). TBE for polyoxin D zinc salt was -41.76 kcal/mol; for fluoxastrobin, it was -39.06 kcal/mol; for trifloxystrobin, it was -33.77 kcal/mol; and for azoxystrobin, the TBE was -35.75 kcal/mol (Table 3). The analysis above revealed that polyoxin D shows more compactness and binding affinity to GCL. The results obtained for binding energy calculations of the protein–ligand interactions through MM/GBSA calculation were reported to be more effective (Genheden and Ryde, 2015). In previous studies, the MM/GBSA approach has proved to be the most accurate and reliable in protein–ligand docked complexes (Singh and Warshel, 2010; Sun et al., 2014). Binding free energy simulations are increasingly used in industries and academia for drug discovery (Feng et al., 2020).
From our study, it has been understood that GCL plays an important role in the metabolic processes of T. deformans. Its blocking and inhibition could control peach leaf curl disease. Furthermore, this protein has a significant potential for enhanced utilization in structure-based drug design and catalysis. The data from this study could drive future experimentation to design novel antifungal drugs by targeting the GCL of T. deformans. This approach not only offers a pathway to develop more effective fungicides but also contributes to the broader goal of achieving environmentally sustainable disease management practices. By focusing on specific targets like GCL, which are not present in the host organism, we can mitigate the impact on non-target species and reduce environmental contamination.
5 Conclusions
In conclusion, using subtractive proteomics approach, we identified the glutamate–cysteine ligase of T. deformans for the first time as a suitable antifungal target. The critical role of GCL in essential metabolic pathways, particularly in glutathione biosynthesis, positions it as an ideal candidate for novel fungicide development. Moreover, to further explore GCL’s potential as a fungicide, we performed two-step virtual screening and MD simulation analysis. The fungicides, such as, polyoxin D zinc salt, fluoxastrobin, trifloxystrobin, and azoxystrobin, showed strong binding affinity toward the active site of GCL, suggesting that GCL may be the best antifungal target in T. deformans. These findings have far-reaching ramifications. By targeting GCL’s crucial role in T. deformans survival and pathogenicity, we open new paths toward creating more effective fungicides capable of counter-resistance posed by the long-term use of existing ones. Furthermore, GCL could even be further utilized as part of drug design using its well-defined active sites for structure-based drug design; the computational tools used here—virtual screening and molecular dynamics—proved to be highly efficient at identifying potential drug targets for validation.
Future research should prioritize the synthesis and experimental validation of GCL fungicide analogs as potential next-generation treatments to effectively combat peach leaf curl disease while being environmentally safer to address concerns over chemical fungicide use. Furthermore, a rigorous examination into using GCL as a target against other fungal pathogens beyond T. deformans may extend these findings and contribute to global efforts in managing fungal diseases in agriculture.
This research presents an innovative and promising strategy to combat T. deformans by targeting glutamate–cysteine ligase. Our insights here could form the basis of future innovations in antifungal treatments that offer sustainable long-term solutions to manage peach leaf curl disease or other fungal infections.
Data availability statement
The original contributions presented in the study are included in the article/Supplementary Material. Further inquiries can be directed to the corresponding author.
Author contributions
WA: Investigation, Methodology, Software, Validation, Visualization, Writing – original draft, Writing – review & editing, Conceptualization, Data curation, Formal analysis. ZR: Conceptualization, Formal analysis, Funding acquisition, Investigation, Methodology, Project administration, Resources, Software, Supervision, Visualization, Writing – original draft, Writing – review & editing, Data curation, Validation. HK: Conceptualization, Data curation, Formal analysis, Investigation, Methodology, Project administration, Software, Supervision, Validation, Visualization, Writing – review & editing, Writing – original draft. JN: Formal analysis, Investigation, Methodology, Software, Validation, Visualization, Writing – review & editing, Writing – original draft. HR: Conceptualization, Data curation, Formal analysis, Investigation, Methodology, Project administration, Writing – review & editing, Writing – original draft. MS: Conceptualization, Formal analysis, Investigation, Methodology, Project administration, Software, Supervision, Validation, Writing – review & editing, Writing – original draft. WS: Conceptualization, Data curation, Formal analysis, Investigation, Supervision, Validation, Visualization, Writing – original draft, Writing – review & editing.
Funding
The author(s) declare that no financial support was received for the research, authorship, and/or publication of this article.
Conflict of interest
The authors declare that the research was conducted in the absence of any commercial or financial relationships that could be construed as a potential conflict of interest.
Publisher’s note
All claims expressed in this article are solely those of the authors and do not necessarily represent those of their affiliated organizations, or those of the publisher, the editors and the reviewers. Any product that may be evaluated in this article, or claim that may be made by its manufacturer, is not guaranteed or endorsed by the publisher.
Supplementary material
The Supplementary Material for this article can be found online at: https://www.frontiersin.org/articles/10.3389/fpls.2024.1429890/full#supplementary-material
References
Aamir, M., Singh, V. K., Dubey, M. K., Meena, M., Kashyap, S. P., Katari, S. K., et al. (2018). In silico prediction, characterization, molecular docking, and dynamic studies on fungal SDRs as novel targets for searching potential fungicides against Fusarium wilt in tomato. Front. Pharmacol. 9, 1038. doi: 10.3389/fphar.2018.01038
Ahmad, I., Ali, S. S., Zafar, B., Hashmi, H. F., Shah, I., Khan, S., et al. (2022). Development of multi-epitope subunit vaccine for protection against the norovirus’ infections based on computational vaccinology. J. Biomol Struct. Dyn 40, 3098–3109. doi: 10.1080/07391102.2020.1845799
Bassi, M., Conti, G. G., Barbieri, N. (1984). Cell wall degradation by Taphrina deformans in host leaf cells: Ultrastructural and cytochemical observations. Mycopathologia 88, 115–125. doi: 10.1007/BF00436441
Bencurova, E., Gupta, S. K., Sarukhanyan, E., Dandekar, T. (2018). Identification of antifungal targets based on computer modeling. J. Fungi 4, 81. doi: 10.3390/jof4030081
Case, D. A., Cheatham, T. E., III, Darden, T., Gohlke, H., Luo, R., Merz, K. M., Jr., et al. (2005). The Amber biomolecular simulation programs. J. Comput. Chem. 26, 1668–1688. doi: 10.1002/jcc.v26:16
Chen, F., Liu, H., Sun, H., Pan, P., Li, Y., Li, D., et al. (2016). Assessing the performance of the MM/PBSA and MM/GBSA methos. 6. Capability to predict protein–protein binding free energies and re-rank binding poses generated by protein–protein docking. Phys. Chem. Chem. Phys. 18, 22129–22139. doi: 10.1039/C6CP03670H
Chester, K. S. (1947). Nature and prevention of plant diseases, 2nd ed (Philadelphia, PA: The Blakiston Company).
Choong, Y. S., Lim, T. S., Chew, A. L., Aziah, I., Ismail, A. (2011). Structural and functional studies of a 50 kDa antigenic protein from Salmonella enterica serovar Typhi. J. Mol. Graph Model. 29, 834–842. doi: 10.1016/j.jmgm.2011.01.008
Cissé, O. H., Almeida, J. M., Fonseca, Á., Kumar, A. A., Salojärvi, J., Overmyer, K., et al. (2013). Genome sequencing of the plant pathogen Taphrina deformans, the causal agent of peach leaf curl. mBio 4, 10–1128. doi: 10.1128/mBio.99055-13
Consortium, U. (2019). UniProt: a worldwide hub of protein knowledge. Nucleic Acids Res. 47, D506–D515.
Dowling, M. E., Hu, M.-J., Schmitz, L. T., Wilson, J. R., Schnabel, G. (2016). Characterization of Botrytis cinerea isolates from strawberry with reduced sensitivity to polyoxin D zinc salt. Plant Dis. 100, 2057–2061. doi: 10.1094/PDIS-02-16-0166-RE
Dym, O., Eisenberg, D., Yeates, T. O. (2012). “ERRAT”, in International tables for crystallographyvolume chapter 21.3. pp. 678–679. doi: 10.110797809553602060000881
Endo, A., Kakiki, K., Misato, T. (1970). Mechanism of action of the antifugal agent polyoxin D. J. Bacteriol 104, 189–196. doi: 10.1128/jb.104.1.189-196.1970
Errami, M., Geourjon, C., Deleage, G. (2003). Detection of unrelated proteins in sequences multiple alignments by using predicted secondary structures. Bioinformatics 19, 506–512. doi: 10.1093/bioinformatics/btg016
Fahey, R. C., Sundquist, A. R. (1991). Evolution of glutathione metabolism. Adv. Enzymol. Relat. Areas Mol. Biol. 64, 53. doi: 10.1002/9780470123102.ch1
Feng, Y., Huang, Y., Zhan, H., Bhatt, P., Chen, S. (2020). An overview of strobilurin fungicide degradation: current status and future perspective. Front. Microbiol. 11, 389. doi: 10.3389/fmicb.2020.00389
Fitzpatrick, R. E. (1934). The life history and parasitism of Taphrina deformans. Sci. Agric. 14, 305–326.
Genheden, S., Ryde, U. (2015). The MM/PBSA and MM/GBSA methods to estimate ligand-binding affinities. Expert Opin. Drug Discovery 10, 449–461. doi: 10.1517/17460441.2015.1032936
Giordani, E., Padula, G., Radice, S. (2013). Compared Anatomy of Young Leaves of Prunus persica (L.) Batsch with Different Degrees of Susceptibility to Taphrina deformans (Berk.) T ul. J. Phytopathol. 161, 190–196. doi: 10.1111/jph.2013.161.issue-3
Giosuè, S., Spada, G., Rossi, V., Carli, G., Ponti, I. (2000). Forecasting infections of the leaf curl disease on peaches caused by Taphrina deformans. Eur. J. Plant Pathol. 106, 563–571. doi: 10.1023/A:1008778814623
Gogorcena Aoiz, Y., Sanchez, G., Moreno-Vázquez, S., Pérez, S., Ksouri, N. (2020). “Genomic-based breeding for climate-smart peach varieties,” in Genomic designing of climate-smart fruit crops (Springer International Publishing, Cham), 271–331.
González-Fernández, R., Prats, E., Jorrín-Novo, J. V. (2010). Proteomics of plant pathogenic fungi. BioMed. Res. Int. 2010, 932527. doi: 10.1155/2010/932527
Gul, H., Ali, S. S., Saleem, S., Khan, S., Khan, J., Wadood, A., et al. (2020). Subtractive proteomics and immunoinformatics approaches to explore Bartonella bacilliformis proteome (virulence factors) to design B and T cell multi-epitope subunit vaccine. Infection Genet. Evol. 85, 104551. doi: 10.1016/j.meegid.2020.104551
Harigua-Souiai, E., Cortes-Ciriano, I., Desdouits, N., Malliavin, T. E., Guizani, I., Nilges, M., et al. (2015). Identification of binding sites and favorable ligand binding moieties by virtual screening and self-organizing map analysis. BMC Bioinf. 16, 1–15. doi: 10.1186/s12859-015-0518-z
Herms, S., Seehaus, K., Koehle, H., Conrath, U. (2002). A strobilurin fungicide enhances the resistance of tobacco against tobacco mosaic virus and Pseudomonas syringae pv tabaci. Plant Physiol. 130, 120–127. doi: 10.1104/pp.004432
Hou, T., Li, N., Li, Y., Wang, W. (2012). Characterization of domain–peptide interaction interface: prediction of SH3 domain-mediated protein–protein interaction network in yeast by generic structure-based models. J. Proteome Res. 11, 2982–2995. doi: 10.1021/pr3000688
Hughes, J. P., Rees, S., Kalindjian, S. B., Philpott, K. L. (2011). Principles of early drug discovery. Br. J. Pharmacol. 162, 1239–1249. doi: 10.1111/j.1476-5381.2010.01127.x
Huynh, T. T., Huynh, V. T., Harmon, M. A., Phillips, M. A. (2003). Gene knockdown of γ-glutamylcysteine synthetase by RNAi in the parasitic protozoa Trypanosoma brucei demonstrates that it is an essential enzyme. J. Biol. Chem. 278, 39794–39800. doi: 10.1074/jbc.M306306200
Islam, M. S., Shahik, S. M., Sohel, M., Patwary, N. I. A., Hasan, M. A. (2015). In silico structural and functional annotation of hypothetical proteins of Vibrio cholerae O139. Genomics Inform 13, 53. doi: 10.5808/GI.2015.13.2.53
Jha, A., Kumar, A., Kumar, A. (2020). Subtractive proteome analysis of Candida albicans divulges promising antifungal targets. Int. J. Pept. Res. Ther. 26, 1559–1566. doi: 10.1007/s10989-019-09953-w
Kelley, L. A., Mezulis, S., Yates, C. M., Wass, M. N., Sternberg, M. J. E. (2015). The Phyre2 web portal for protein modeling, prediction and analysis. Nat. Protoc. 10, 845–858. doi: 10.1038/nprot.2015.053
Kern, H., Naef-Roth, S. (1975). Zur Bildung von Auxinen und Cytokininen durch Taphrina-Arten. Phytopathologische Z. 83. doi: 10.1111/j.1439-0434.1975.tb03532.x
Khan, A., Ahsan, O., Wei, D.-Q., Ansari, J. K., Najmi, M. H., Muhammad, K., et al. (2021a). Computational evaluation of abrogation of hbx-bcl-xl complex with high-affinity carbon nanotubes (Fullerene) to halt the hepatitis b virus replication. Molecules 26, 21. doi: 10.3390/molecules26216433
Khan, S., Ali, S. S., Zaheer, I., Saleem, S., Ziaullah, Zaman, N., et al. (2022). Proteome-wide mapping and reverse vaccinology-based B and T cell multi-epitope subunit vaccine designing for immune response reinforcement against Porphyromonas gingivalis. J. Biomol Struct. Dyn 40, 833–847. doi: 10.1080/07391102.2020.1819423
Khan, A., Gui, J., Ahmad, W., Haq, I., Shahid, M., Khan, A. A., et al. (2021b). The SARS-CoV-2 B. 1.618 variant slightly alters the spike RBD–ACE2 binding affinity and is an antibody escaping variant: a computational structural perspective. RSC Adv. 11, 30132–30147. doi: 10.1039/D1RA04694B
Khan, S., Jamal, M. S., Anjum, F., Rasool, M., Ansari, A., Islam, A., et al. (2016). Functional annotation of putative conserved proteins from Borrelia burgdorferi to find potential drug targets. Int. J. Comput. Biol. Drug Des. 9, 295–318. doi: 10.1504/IJCBDD.2016.080099
Khan, S., Khan, A., Rehman, A. U., Ahmad, I., Ullah, S., Khan, A. A., et al. (2019). Immunoinformatics and structural vaccinology driven prediction of multi-epitope vaccine against Mayaro virus and validation through in-silico expression. Infection Genet. Evol. 73, 390–400. doi: 10.1016/j.meegid.2019.06.006
Kolattukudy, P. E. (1985). Enzymatic penetration of the plant cuticle by fungal pathogens. Annu. Rev. Phytopathol. 23, 223–250. doi: 10.1146/annurev.py.23.090185.001255
Kurtzman, C. P., Fell, J. W., Boekhout, T. (2011). The yeasts, a taxonomic study. Available online at: https://pure.knaw.nl/portal/en/publications/the-yeasts-a-taxonomic-study-5th-ed (Accessed January 15, 2024).
Laskowski, R. A., MacArthur, M. W., Moss, D. S., Thornton, J. M. (1993). PROCHECK: a program to check the stereochemical quality of protein structures. J. Appl. Crystallogr. 26, 283–291. doi: 10.1107/S0021889892009944
La Torre, A., Iovino, V., Caradonia, F. (2018). Copper in plant protection: Current situation and prospects. Phytopathol. Mediterr. 57, 201–236. doi: 10.14601/Phytopathol_Mediterr-23407
Li, W., Godzik, A. (2006). Cd-hit: a fast program for clustering and comparing large sets of protein or nucleotide sequences. Bioinformatics 22, 1658–1659. doi: 10.1093/bioinformatics/btl158
Lu, T., Zhang, Q., Lavoie, M., Zhu, Y., Ye, Y., Yang, J., et al. (2019). The fungicide azoxystrobin promotes freshwater cyanobacterial dominance through altering competition. Microbiome 7, 1–13. doi: 10.1186/s40168-019-0744-0
Luo, H., Lin, Y., Gao, F., Zhang, C.-T., Zhang, R. (2014). DEG 10, an update of the database of essential genes that includes both protein-coding genes and noncoding genomic elements. Nucleic Acids Res. 42, D574–D580. doi: 10.1093/nar/gkt1131
Maniatis, E. I., Karamichali, I., Stefanidou, E., Boutsika, A., Tsitsigiannis, D. I., Paplomatas, E., et al. (2024). Insights into the transcriptional reprogramming of peach leaves inoculated with Taphrina deformans. Plants 13, 861. doi: 10.3390/plants13060861
Miller, B. R., III, McGee, T. D., Jr., Swails, J. M., Homeyer, N., Gohlke, H., Roitberg, A. E. (2012). MMPBSA. py: an efficient program for end-state free energy calculations. J. Chem. Theory Comput. 8, 3314–3321. doi: 10.1021/ct300418h
Moriya, Y., Itoh, M., Okuda, S., Yoshizawa, A. C., Kanehisa, M. (2007). KAAS: an automatic genome annotation and pathway reconstruction server. Nucleic Acids Res. 35, W182–W185. doi: 10.1093/nar/gkm321
Mukherjee, A., Roy, G., Guimond, C., Ouellette, M. (2009). The γ-glutamylcysteine synthetase gene of Leishmania is essential and involved in response to oxidants. Mol. Microbiol. 74, 914–927. doi: 10.1111/j.1365-2958.2009.06907.x
Naqvi, A. A. T., Rahman, S., Zeya, F., Kumar, K., Choudhary, H., Jamal, M. S., et al. (2017). Genome analysis of Chlamydia trachomatis for functional characterization of hypothetical proteins to discover novel drug targets. Int. J. Biol. Macromol 96, 234–240. doi: 10.1016/j.ijbiomac.2016.12.045
Pecknold, P. C. (2015a). “Peach leaf curl,” in Historical documents of the purdue cooperative extension service. Paper 1091. Available online at: https://docs.lib.purdue.edu/.
Pecknold, P. C. (2015b). Purdue e-Pubs Historical Documents of the Purdue Cooperative Extension Service. Available online at: https://docs.lib.purdue.edu/agext/1091 (Accesssed May 15, 2022).
Price, D. J., Brooks, C. L., III (2004). A modified TIP3P water potential for simulation with Ewald summation. J. Chem. Phys. 121, 10096–10103. doi: 10.1063/1.1808117
Ravindranath, P. A., Forli, S., Goodsell, D. S., Olson, A. J., Sanner, M. F. (2015). AutoDockFR: advances in protein-ligand docking with explicitly specified binding site flexibility. PloS Comput. Biol. 11, e1004586. doi: 10.1371/journal.pcbi.1004586
Roe, D. R., Cheatham, T. E., III (2013). PTRAJ and CPPTRAJ: software for processing and analysis of molecular dynamics trajectory data. J. Chem. Theory Comput. 9, 3084–3095. doi: 10.1021/ct400341p
Rossi, V., Bolognesi, M., Giosue, S. (2007). Influence of weather conditions on infection of peach fruit by Taphrina deformans. Phytopathology 97, 1625–1633. doi: 10.1094/PHYTO-97-12-1625
Sammut, S. J., Finn, R. D., Bateman, A. (2008). Pfam 10 years on: 10 000 families and still growing. Brief Bioinform. 9, 210–219. doi: 10.1093/bib/bbn010
Shahbaaz, M., ImtaiyazHassan, M. D., Ahmad, F. (2013). Functional annotation of conserved hypothetical proteins from Haemophilus influenzae Rd KW20. PloS One 8, e84263. doi: 10.1371/journal.pone.0084263
Shahid, F., Ashfaq, U. A., Saeed, S., Munir, S., Almatroudi, A., Khurshid, M. (2020). In silico subtractive proteomics approach for identification of potential drug targets in Staphylococcus saprophyticus. Int. J. Environ. Res. Public Health 17, 3644. doi: 10.3390/ijerph17103644
Shahid, A. A., Asif, M., Shahbaz, M., Ali, M. (2016). “Antifungal potential of Ganoderma lucidum extract against plant pathogenic fungi of Calendula officinalis L,” in 5th International Conference on Biological, Chemical and Environmental Sciences (BCES-2016), March, 24–25. (London UK). Available online at: http://www.icbe.org/2016/03/25/70.
Singh, N., Warshel, A. (2010). Absolute binding free energy calculations: on the accuracy of computational scoring of protein–ligand interactions. Proteins: Structure Function Bioinf. 78, 1705–1723. doi: 10.1002/prot.22687
Sipos, K., Lange, H., Fekete, Z., Ullmann, P., Lill, R., Kispal, G. (2002). Maturation of cytosolic iron-sulfur proteins requires glutathione. J. Biol. Chem. 277, 26944–26949. doi: 10.1074/jbc.M200677200
Sun, H., Li, Y., Tian, S., Xu, L., Hou, T. (2014). Assessing the performance of MM/PBSA and MM/GBSA methods. 4. Accuracies of MM/PBSA and MM/GBSA methodologies evaluated by various simulation protocols using PDBbind data set. Phys. Chem. Chem. Phys. 16, 16719–16729. doi: 10.1039/C4CP01388C
Svetaz, L. A., Bustamante, C. A., Goldy, C., Rivero, N., Müller, G. L., Valentini, G. H., et al. (2017). Unravelling early events in the Taphrina deformans–Prunus persica interaction: an insight into the differential responses in resistant and susceptible genotypes. Plant Cell Environ. 40, 1456–1473. doi: 10.1111/pce.12942
Thomidis, T., Zioziou, E., Koundouras, S., Navrozidis, I., Nikolaou, N. (2018). Effect of prohexadione-Ca on leaf chlorophyll content, gas exchange, berry size and composition, wine quality and disease susceptibility in Vitis vinifera L. cv Xinomavro. Scientia Horticulturae 238, 369–374. doi: 10.1016/j.scienta.2018.05.008
Tsai, I. J., Tanaka, E., Masuya, H., Tanaka, R., Hirooka, Y. (2014). Comparative genomics of Taphrina fungi causing varying degrees of tumorous deformity in plants. Genome Biol Evol. 6, 861–872. doi: 10.1093/gbe/evu067
van Vlijmen, H., Desjarlais, R. L., Mirzadegan, T. (2017). Computational chemistry at Janssen. J. Comput. Aided Mol. Des. 31, 267–273. doi: 10.1007/s10822-016-9998-9
Wang, Y., Khan, A., Kaushik, A. C., Junaid, M., Zhang, X., Wei, D.-Q. (2018). The systematic modeling studies and free energy calculations of the phenazine compounds as anti-tuberculosis agents. J. Biomol Struct. Dyn 37, 4051–4069. doi: 10.1080/07391102.2018.1537896
Wiederstein, M., Sippl, M. J. (2007). ProSA-web: interactive web service for the recognition of errors in three-dimensional structures of proteins. Nucleic Acids Res. 35, W407–W410. doi: 10.1093/nar/gkm290
Yang, Y., Adelstein, S. J., Kassis, A. I. (2012). Target discovery from data mining approaches. Drug Discovery Today 17, S16–S23. doi: 10.1016/j.drudis.2011.12.006
Yu, C., Chen, Y., Lu, C., Hwang, J. (2006). Prediction of protein subcellular localization. Proteins: Structure Function Bioinf. 64, 643–651. doi: 10.1002/prot.21018
Zeng, R., Gao, S., Xu, L., Liu, X., Dai, F. (2018). Prediction of pathogenesis-related secreted proteins from Stemphylium lycopersici. BMC Microbiol. 18, 1–10. doi: 10.1186/s12866-018-1329-y
Zhang, R., Ou, H., Zhang, C. (2004). DEG: a database of essential genes. Nucleic Acids Res. 32, D271–D272. doi: 10.1093/nar/gkh024
Keywords: T. deformans, Prunus persica, antifungal targets, fungicides, docking, simulation
Citation: Ahmad W, Rahman Z, Khan H, Nawab J, Rahman H, Siddiqui MF and Saeed W (2024) Computational proteomics analysis of Taphrina deformans for the identification of antifungal drug targets and validation with commercial fungicides. Front. Plant Sci. 15:1429890. doi: 10.3389/fpls.2024.1429890
Received: 08 May 2024; Accepted: 10 October 2024;
Published: 07 November 2024.
Edited by:
Prabu Gnanasekaran, Washington State University, United StatesReviewed by:
Deepjyoti Singh, North Carolina State University, United StatesKaren Barandoc Alviar, University of the Philippines Los Baños, Philippines
Jian-Wei Guo, Kunming University, China
Ved Prakash, Kansas State University, United States
Copyright © 2024 Ahmad, Rahman, Khan, Nawab, Rahman, Siddiqui and Saeed. This is an open-access article distributed under the terms of the Creative Commons Attribution License (CC BY). The use, distribution or reproduction in other forums is permitted, provided the original author(s) and the copyright owner(s) are credited and that the original publication in this journal is cited, in accordance with accepted academic practice. No use, distribution or reproduction is permitted which does not comply with these terms.
*Correspondence: Ziaur Rahman, enJhaG1hbkBhd2t1bS5lZHUucGs=
†ORCID: Waqar Ahmad, orcid.org/0009-0005-9464-3641
Zia ur Rahman, orcid.org/0000-0002-0795-200X
Haji Khan, orcid.org/0000-0002-3263-306X
Javed Nawab, orcid.org/0000-0001-5115-776X
Hazir Rahman, orcid.org/0000-0002-6572-8428
Muhammad Faisal Siddiqui, orcid.org/0000-0001-6547-9532
Wajeeha Saeed, orcid.org/0000-0002-4665-8479