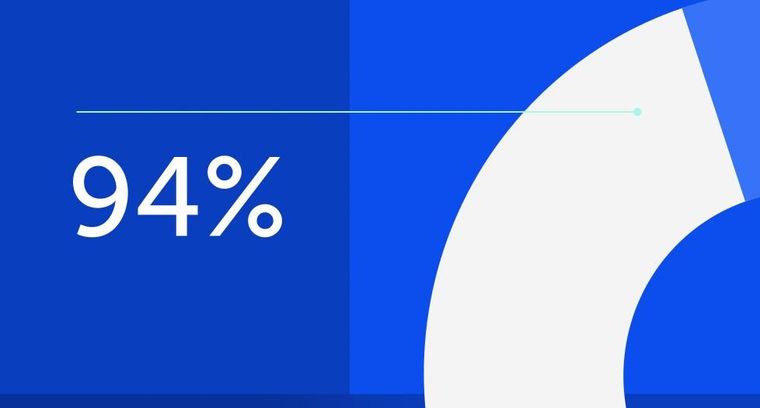
94% of researchers rate our articles as excellent or good
Learn more about the work of our research integrity team to safeguard the quality of each article we publish.
Find out more
ORIGINAL RESEARCH article
Front. Plant Sci., 23 May 2024
Sec. Plant Pathogen Interactions
Volume 15 - 2024 | https://doi.org/10.3389/fpls.2024.1416253
This study presents a comprehensive genomic analysis of Burkholderia plantarii, a rice pathogen that causes blight and grain rot in seedlings. The entire genome of B. plantarii KACC 18964 was sequenced, followed by a comparative genomic analysis with other available genomes to gain insights into its virulence, fitness, and interactions with rice. Multiple secondary metabolite gene clusters were identified. Among these, 12 demonstrated varying similarity levels to known clusters linked to bioactive compounds, whereas eight exhibited no similarity, indicating B. plantarii as a source of potentially novel secondary metabolites. Notably, the genes responsible for tropolone and quorum sensing were conserved across the examined genomes. Additionally, B. plantarii was observed to possess three complete CRISPR systems and a range of secretion systems, exhibiting minor variations among the analyzed genomes. Genomic islands were analyzed across the four genomes, and a detailed study of the B. plantarii KACC 18964 genome revealed 59 unique islands. These islands were thoroughly investigated for their gene contents and potential roles in virulence. Particular attention has been devoted to the Type III secretion system (T3SS), a crucial virulence factor. An in silico analysis of potential T3SS effectors identified a conserved gene, aroA. Further mutational studies, in planta and in vitro analyses validated the association between aroA and virulence in rice. Overall, this study enriches our understanding of the genomic basis of B. plantarii pathogenicity and emphasizes the potential role of aroA in virulence. This understanding may guide the development of effective disease management strategies.
The Burkholderia genus, a member of the β-proteobacteria subphylum, encompasses gram-negative bacterial species that are distinguished by their genetic versatility and adaptability to various ecological niches (O’Sullivan and Mahenthiralingam, 2005). These microorganisms are known for their high genetic plasticity and large multi-replicon genomes, which are rich in insertion sequences and genomic islands. These genomes contain a high proportion of coding regions, providing these bacteria with a substantial metabolic capacity to produce various metabolites capable of degrading environmental substances. This metabolic capacity enables their survival and fitness across a multitude of habitats (Compant et al., 2008; Seo et al., 2015; Mannaa et al., 2018).
Within the Burkholderia genus, three plant pathogenic species have been identified that cause diseases in rice plants: B. glumae, B. gladioli, and B. plantarii. B. glumae and B. gladioli have been extensively studied, but B. plantarii has received relatively little attention. B. plantarii was identified in Japan in 1985 and has subsequently spread to China and Korea (Azegami et al., 1987; Ra et al., 2016; Wang et al., 2016). This pathogen, once believed to affect only specific stages of rice growth, has recently been implicated in causing more severe rice diseases and has exhibited the ability to spread to other geographic locations. In addition to seedling blight, this pathogen causes grain rot, demonstrating its unique and damaging characteristics (Ra et al., 2016). This pathogen inflicts various symptoms in rice seedlings, including chlorosis, stunting, and inhibition of root growth, as well as grain rot symptoms such as grain abortion and discoloration of the hull and grain at the flowering and maturity stages (Azegami et al., 1988; Ra et al., 2016).
B. plantarii virulence is primarily attributed to its production of tropolone, a potent phytotoxin. Tropolone virulence in rice seedlings is facilitated by a powerful cationic metal chelating effect (Wang et al., 2013). However, the pathogenicity of B. plantarii might not rely solely on tropolone. Certain plant species remain symptom-free when exposed to pure tropolone, whereas inoculation with tropolone-producing B. plantarii triggers disease development (Iiyama et al., 1998). This indicates that other, less understood virulence factors may also play significant roles in the disease process.
One essential element among the various virulence factors implicated in bacterial infections in eukaryotic hosts is the type III secretion system (T3SS). This complex and specialized macromolecular structure, embedded in the membranes of gram-negative bacteria, serves as a protein delivery machine for translocation into eukaryotic cells (Reinhold-Hurek and Hurek, 2011; Angus et al., 2014). T3SS can be used to inject protein effectors into the host, a feature exploited by many bacterial pathogens, emphasizing the potential association between the presence of T3SS and virulence in B. plantarii. The T3SS is common among pathogenic bacterial strains, but it is relatively rare among plant-beneficial strains and endophytes. Consequently, T3SS is frequently considered a pathogenic marker (Angus et al., 2014; Wallner et al., 2021).
The significance of the T3SS in pathogenicity has been observed in B. plantarii related species. For instance, in B. glumae, the T3SS has been found to efficiently translocate effectors to plant cells, contributing to its virulence (Sharma et al., 2013). The T3SS of B. gladioli has not yet been thoroughly investigated for its role in rice pathogenesis; however, it has been implicated in mycophagy, which is promoted by the injection of a prophage-tail-like effector via the T3SS of B. gladioli (Swain et al., 2017). Given these observations, investigating T3SS and understanding its role and potential effectors in B. plantarii is critical for unraveling the mechanisms of virulence and identifying avenues for effective disease management.
The advent of high-throughput sequencing technologies has revolutionized the field of bacterial genomics and paved the way for advanced studies on pathogen biology (Goodwin et al., 2016). Comparative genomics has emerged as a potent approach to investigate the virulence, adaptation, and evolutionary dynamics of bacterial pathogens, providing vital insights into their interactions with host organisms (Land et al., 2015). Application of these techniques to B. plantarii has revealed unique genetic traits associated with pathogenicity and fitness, enhancing our understanding of the biology of the pathogen and its interactions with the rice host (Seo et al., 2015).
Despite advancements in our understanding, significant gaps remain regarding the genomic characteristics of B. plantarii, particularly those contributing to its fitness and virulence. Thus, this study aimed to conduct a thorough genomic analysis of B. plantarii utilizing the complete and nearly complete genomes for elements potentially linked to bacterial virulence and fitness. Our focus extended to features such as secondary metabolite production, quorum sensing (QS), tropolone biosynthesis, CRISPR systems, genomic islands, and a variety of secretion systems. A particular focus was placed on identifying potential T3SS effectors and examining the role of a specific effector gene in the virulence of B. plantarii in rice, along with its possible contributions to other physiological functions. Through this detailed genomic exploration, we aim to enhance our understanding of the pathogenic mechanisms employed by B. plantarii and establish a solid foundation for subsequent research into a critical virulence effector, which may have a close association with the T3SS.
Complete and nearly complete genomes of B. plantarii strains were downloaded from the National Center for Biotechnology Information (NCBI) database. In addition, the complete genome of B. plantarii KACC 18964, originally isolated from rice panicles showing blight symptoms in South Korea, has been sequenced (Ra et al., 2016). To sequence its whole genome, a pure culture of the bacterium was obtained by streaking it on Luria-Bertani (LB) agar, followed by incubation at 28°C. A single colony from the plate was transferred to fresh LB broth and incubated in a shaking incubator at 200 rpm and 28°C. Genomic DNA was extracted from this culture using a Wizard Genomic DNA Purification Kit (Promega, Madison, WI, USA) according to the manufacturer’s protocol. The quantity and purity of the DNA samples were assessed using a NanoDrop 2000 spectrophotometer (Thermo Fisher Scientific, Waltham, MA, USA).
The whole genome sequence library of genomic DNA of B. plantarii KACC 18964 was prepared using the SMRTbell template prep kit (Pacific Biosciences, Menlo Park, CA, USA). Single-molecule real-time (SMRT) sequencing was performed using the PacBio RS II platform (Pacific Biosciences) at Macrogen (Seoul, South Korea). To improve contig accuracy, HiSeq3000 (Illumina, San Diego, CA, USA) paired-end reads were used for sequence compensation. The hierarchical genome assembly process (HGAP) version 3 in the PacBio SMRT Analysis algorithm version 2.3.0 package was implemented for pre- and de novo assemblies of long reads (Chin et al., 2013). Quality control of the long reads was performed using the PreAssembler filter version 1 protocol from HGAP, and the sequences were subsequently polished with Quiver to enhance quality (Chin et al., 2013). Pilon version 1.21 was used to map the HiSeq reads to the PacBio assembly twice, improving the accuracy of the genome assembly (Chin et al., 2013). Initially, genomic features were predicted and annotated using Prokka version 1.13 (Seemann, 2014), and re-annotation was performed using the NCBI prokaryotic genome annotation pipeline based on the best-placed reference protein set and GeneMarkS+ (Besemer et al., 2001). Genomic resources of B. plantarii KACC 18964 have been deposited in the NCBI GenBank (GCA_030644525.1).
The genome sequence of the KACC 18964 strain was further annotated using the Rapid Annotation Subsystem Technology toolkit (Brettin et al., 2015). Subsystem categories were assigned, and the number of genes was determined using the Bacterial and Viral Bioinformatics Resource Center (https://www.bv-brc.org/). To visualize genomic features, a circular feature map was generated using the CGView server (Stothard et al., 2019). For comparative analysis, the genomic sequences of all B. plantarii strains were downloaded from the FTP site of the RefSeq database at NCBI (ftp://ftp.ncbi.nih.gov/genomes).
The whole genome sequence of B. plantarii KACC 18964 was compared to that of three other B. plantarii strains (ATCC 43733, PG1, and ZJ171) as well as the congeneric outgroup species B. glumae BGR1. Average nucleotide identity (ANI) values were computed using the OrthoANIu algorithm in EzBioCloud to assess the similarity between the complete genome sequences of B. plantarii KACC 18964 and other strains (Yoon et al., 2017).
Whole-genome alignment and digital DNA-DNA hybridization (dDDH) values were calculated using the genome-to-genome distance calculator web server with Formula 2 (http://ggdc.dsmz.de/distcalc2.php). Additionally, the KACC 18964 genome was compared with other related genomes of Burkholderia type strains using the Type (Strain) Genome Server (TYGS) (Meier-Kolthoff et al., 2013). Phylogenomic pairwise comparisons were performed among a set of related Burkholderia genomes using the genome BLAST distance phylogeny (GBDP) approach. Accurate intergenomic distances were inferred using the ‘trimming’ algorithm and the distance formula d5 with 100 distance replicates (Meier-Kolthoff et al., 2013; Meier-Kolthoff and Göker, 2019).
Based on these intergenomic distances, a balanced minimum evolution tree was constructed using FASTME 2.1.6.1. Branch support was inferred from 100 pseudo-bootstrap replicates (Lefort et al., 2015). To cluster genomes into species, type-based species clustering was performed using a 70% dDDH radius around each type strain (Meier-Kolthoff and Göker, 2019). The resulting tree was visualized using iTOL v5 (Letunic and Bork, 2021).
Pan-genome analysis was performed using Roary version 3.11.2 (Page et al., 2015). To generate input files for Roary, the gff format files were derived from the gbk format files of each strain, which were downloaded from the NCBI RefSeq database using a custom Python script. The CD-HIT algorithm was used in Roary (Fu et al., 2012) with default parameters, including a minimum percentage identity of 95% for blastp and a core gene threshold of 99% occurrence across the strains, to cluster orthologous gene families and calculate the pan-genome of the four B. plantarii strains and B. glumae BGR1. The Roary pipeline incorporates the PRANK aligner (Löytynoja, 2014) to create a concatenated multiple sequence alignment of the core gene families. To construct a maximum-likelihood tree from the core gene alignment, RAxML version 8 was used (Stamatakis, 2014). The best-scoring tree was generated using the GTRGAMMA model, supported by 1000 rapid bootstrap replicates. The resulting tree was visualized using iTOL v5 (Letunic and Bork, 2021). To graphically represent the distribution of the pan-genome across the strains, we utilized the roary_plots Python scripts (Galardini, 2017). These scripts used the core genome tree constructed from the RAxML tool, along with the gene presence/absence matrix obtained from Roary analysis.
Whole-genome mining was employed to identify potential functional elements. Particularly, we investigated the QS I/R system (plaI, plaM, and plaR) of B. plantarii, as well as the tropolone biosynthesis genes across multiple B. plantarii strains (ATCC 43733, KCCM 18964, PG1, and ZJ171).
To assess the potential for secondary metabolite production in B. plantarii KACC 18964, we used the antiSMASH tool server, which predicts secondary metabolite biosynthesis gene clusters (Blin et al., 2021). The outcomes were then visualized by mapping the predicted gene clusters onto a circular representation of the genome, and the similarity levels to known gene clusters were determined.
The CRISPR regions and CRISPR-associated Cas proteins were predicted in all B. plantarii and B. glumae BGR1 genomes using CRISPRCasFinder (Couvin et al., 2018). For this analysis, only CRISPR arrays with an evidence level > 3 were considered to avoid potential false-positive results, such as short arrays with one to three spacers. The arrays identified from CRISPRCasFinder were cross-verified with the results from CRISPRDetect version 2.4 (Biswas et al., 2016) and the corresponding NCBI genome annotations.
To characterize the consensus sequences, CRISPR repeat sequences were aligned using T-COFFEE (Notredame et al., 2000) with default parameters and presented using ESPript programs (Robert and Gouet, 2014). Each strain’s CRISPR array was grouped based on its conserved sequences, and a number was assigned to each group (e.g., CRISPR 1, CRISPR 2, and CRISPR 3). The sequence logo of the T-COFFEE-aligned CRISPR DR was generated using Weblogo (Crooks et al., 2004).
To assess the inter-strain and intra-species divergence of the spacers, CRISPRStudio was used with default parameters (Dion et al., 2018). This allowed us to concatenate, compare, and visualize the spacers predicted by CRISPRCasFinder and CRISPRDetect using HEX color codes.
Genomic island prediction for B. plantarii was performed using two distinct tools. Initial comparative analysis utilized IslandCompare v1.0 (Bertelli et al., 2022), for cross-genome GI content exploration within the four studied B. plantarii to identify GIs, and antimicrobial gene determinants enabling comparative analysis in tested genomes.
Subsequent in-depth prediction and analysis of GIs within the B. plantarii KACC18964 genome were conducted using IslandViewer 4 (Bertelli et al., 2017), an integrated tool for the computational identification and visualization of GIs and antimicrobial resistance genes, combining IslandPath-DIMOB, and SIGI-HMM prediction algorithms to provide a comprehensive overview of potential GIs. Gene content within predicted GIs was assessed for virulence potential, focusing on toxins, effectors, transport, and secretion systems-related genes.
The detection and visualization of different secretion systems in all B. plantarii genomes were performed using MacSyFinder software (Abby et al., 2014) and associated models (Abby et al., 2016), which employ hidden Markov model profiles for efficient genomic detection of bacterial secretion systems with default parameters. The secretion systems detected in the four B. plantarii strains were compared based on the structure of the gene clusters.
After confirming the presence of the type III secretion system, associated effector proteins were predicted from both B. plantarii and B. glumae using EffectiveDB (Eichinger et al., 2016), a comprehensive tool that integrates various algorithms to recognize T3SS signals (EffectiveT3), conserved binding sites of type III chaperones (EffectiveCCBD), eukaryotic-like domains (EffectiveELD), and subcellular targeting signals in the host (Predotar). The default parameters were used for the tools as follows: Effective T3, minimal score = 0.9999 (2.0.1 model); EffectiveELD, minimal score = 4; and Predotar, plant model. Only high-confidence predictions were included in the results. The predicted specific effector that was identified by all algorithms was considered for further analysis to explore the potential mechanisms of interaction with the plant host cells and its involvement in virulence in a rice in planta assay, and in vitro characterization of its involvement with various physiological functions in B. plantarii.
After investigating the potential T3SS effectors, the aroA gene (locus tag: GIY62_15125, 1302 bp) was identified as a potential effector by all four algorithms used in the examined strains, suggesting its potential involvement in the virulence of B. plantarii in rice plants. To examine the potential involvement of aroA in B. plantarii KACC 18964 virulence, deletion mutant (ΔaroA) and complemented (CaroA) strains were generated as previously described (Lee et al., 2019). Briefly, standard protocols (Sambrook et al., 1989) were used for DNA amplification, recombinant DNA construction, and mutant strain generation. To create ΔaroA strains, the upstream (L fragment) and downstream (R fragment) regions were amplified from B. plantarii KACC18964 gDNA using Solgent Pfu-X DNA polymerase and primers with the appropriate restriction sites (Supplementary Table S2). These fragments were ligated into the pK18mobsacB suicide vector after digestion with specific restriction enzymes (Schäfer et al., 1994). The recombinant plasmids were introduced into E. coli DH5α λpir cells, transferred to E. coli S17–1 λpir (donor strain), and transformed into B. plantarii KACC18964 (recipient strain) through conjugation (Simon et al., 1983). Deletion mutants were selected using appropriate antibiotics, and a second homologous recombination was induced by subculturing them in LB containing 30% sucrose. To generate CaroA strains, the complete open reading frame of aroA was amplified and inserted into the pBBR1MCS-2 expression vector (Kovach et al., 1995). The recombinant vectors were introduced into each mutant strain via conjugation. Polymerase chain reaction (PCR) was performed to confirm the mutant and complemented strains. The strains and plasmids used are listed in Supplementary Table S3.
The generated ΔaroA, CaroA, and wild-type strains were evaluated in rice using in planta assays. Pure cultures of the wild type and mutant strains were grown on LB agar plates at 28°C for 48 h. Single colonies were then transferred into 4 mL of LB broth and grown overnight at 28°C with shaking. Subsequently, 100 µL of the cultures were transferred into 50 mL of LB broth and grown for an additional 48 h at 28°C with shaking. Bacterial cells were harvested by centrifugation at 4000 g for 20 min. The cells were washed twice and resuspended in sterilized 10 mM MgSO4. The optical density of the bacterial suspensions was adjusted to OD600 nm = 0.8 (equivalent to approximately 109 cells/mL).
Rice plants used in this experiment were cultivated under greenhouse conditions with an average temperature of 30°C during the day and 25°C at night. At the flowering stage, the rice panicles were inoculated by dipping them in 30 mL of the bacterial suspension in a glass tube for 1 min. Ten days post-inoculation (dpi), the disease severity in the rice panicles was assessed and photographed. Sterilized 10 mM MgSO4 buffer was used as a negative control. Disease severity evaluation was performed using a scale of disease severity ranking from 0 to 5: 0 for panicles with asymptomatic healthy grains, 1 for panicles with 0–20% symptomatic grains, 2 for panicles with 21–40% symptomatic grains, 3 for panicles with 41–60% symptomatic grains, 4 for panicles with 61–80% symptomatic grains, and 5 for panicles with 81–100% symptomatic grains. Disease severity was calculated using the following formula:
For the seedling assay, rice seeds underwent surface sterilization with 70% ethanol and 1% NaOCl for 5 min, followed by three rinses in sterile distilled water (SDW) and drying on sterile paper. The seeds were then immersed in the bacterial suspensions with the control group treated with 10 mM MgSO4. After a 3-day dark incubation at 28°C, germinated seeds were transferred to plant culture containers with sterilized Kimtech tissue and 25 mL of SDW. The growth chamber maintained a 28°C average temperature and a 12-hour day/night light cycle. Seedlings were subsequently assessed for shoot and root length and photographed to evaluate disease severity 7 days after incubation in the growth chamber.
To evaluate the impact of aroA on the bacterial ability to colonize rice plants, another set of seedling assays was performed under aseptic conditions. After incubation, the entire seedling was homogenized in 10 mM MgSO4 and serially diluted. Subsequently, 200 µL of each dilution were spread on LB agar plates containing kanamycin (50 μg/mL) to count colony-forming units (CFUs). This procedure was conducted to assess the bacterial survivability and colonization capacity in rice plant tissues between the WT and the generated mutants.
To assess the effect of aroA deletion on the bacterial growth, oxidative stress tolerance, extracellular protease activity, and motility, several in vitro assays have been conducted. The growth rate of the generated mutant ΔaroA and the complemented strain CaroA was compared to that of the wild-type. Briefly, overnight cultures of each bacterial strain were diluted to a standardized OD600 of 0.05 in fresh LB medium, incubated at 28°C with shaking at 200 rpm, and growth rates were evaluated by measuring the OD600 every 6 h.
For the motility assay, strains were grown each in LB broth to an OD600 of 0.8 to standardize the cell density before inoculation. For the assessment of swarming and swimming motility, LB agar plates with 0.5% and 0.3% agar concentrations were prepared, respectively. From each bacterial culture, 3 µl were transferred to the center of the respective agar plates. Plates were incubated at 28°C for 48 h to allow for bacterial motility. The surface area covered by bacterial growth was measured using ImageJ software. The analysis quantified the area to determine the extent of swarming and swimming behaviors. Comparisons of motility across the three strains were made using LSD test to determine significance, with P < 0.05 considered significant difference.
Tolerance to oxidative stress was assessed using disk diffusion assays (Lee et al., 2019). LB agar plates were uniformly seeded with each bacterial strain. Sterile paper disks, saturated with specific concentrations of H2O2 (7%, and 28%), were placed at the center of the agar plates. The plates were then incubated for 24 h at 28°C, after which zones of inhibition were observed to evaluate the bacterial tolerance to oxidative stress.
Extracellular protease activity was determined using LB agar plates enhanced with 2% skim milk (Mannaa et al., 2023). Wells were formed in the agar, into which the bacterial strains were inoculated. After incubation, the presence of clear zones surrounding the wells indicated protease activity, serving as a measure of the ability to degrade extracellular proteins.
Bioinformatic analyses were conducted as follows unless stated otherwise. The gene loci were graphically represented with colored arrows using EasyFig version 2.1 (Sullivan et al., 2011). Phylogenetic trees were constructed using the neighbor-joining method with the Maximum Composite Likelihood model and 1000 bootstrap replicates unless otherwise indicated. Heatmaps were visualized using the heatmap.2 function of the ggplots package in R (https://CRAN.R-project.org/package=gplots). Circular maps were generated using CGview (Stothard et al., 2019). Data analysis for the plant, and in vitro assays was conducted using Statistical Analysis Systems software (SAS Institute, Cary, NC, USA). The General Linear Model procedure in SAS was used to perform an analysis of variance. Differences between the means were evaluated using the least significant difference test, and the level of statistical significance was set at P < 0.05.
The complete genome of B. plantarii KACC 18964 was successfully assembled into two chromosomes and one plasmid, totaling 8,124,970 bp with a G+C content of 68.66%. Table 1 provides an overview of the essential genomic features, and Figure 1A presents a circular view of both chromosomes and the plasmid, highlighting the coding DNA sequences (CDS), transfer RNAs (tRNAs), ribosomal RNAs (rRNAs), GC content, and positive/negative GC skew.
Figure 1 (A) A Circular representation of the complete genome of Burkholderia plantarii strain KACC 18964. The key in the bottom-right corner illustrates the individual circles in a top-down outermost to innermost direction. (B) The subsystem categories of the B. plantarii KACC 18964 genome showing the distribution of the numbers of detected subsystems and genes with assigned functions.
Through RAST analysis of the B. plantarii KACC 18964 genome, 7,709 CDSs were identified. Among these, 5,998 CDSs (77%) were not assigned to any specific subsystem, whereas 1,711 CDSs (23%) were assigned to various subsystems. The majority of the genes were classified under metabolism (45% of assigned genes), membrane transport (13% of assigned genes), energy (11% of assigned genes), protein processing (10% of assigned genes), and stress response, defense, and virulence (7% of assigned genes). A detailed breakdown of the identified subsystem categories and the distribution of the assigned genes within these subsystems is shown in Figure 1B.
The complete genome sequence of B. plantarii KACC18964 was compared with that of other B. plantarii strains (ATCC 43733, KCCM 18964, PG1, and ZJ171) and B. glumae BGR1 obtained from the NCBI database. Table 2 provides detailed information on the genomes used for comparison, including accession numbers and basic features.
In silico analyses were performed to determine the relatedness of the sequenced KACC 18964 genome to the other B. plantarii and B. glumae genomes in terms of ANI and dDDH. The results demonstrated that the ANI and dDDH values fell within species boundaries, consistent with the species identification (Figures 2A, B). To further explore the phylogenetic relationships, a phylogenomic tree was constructed using GBDP based on the whole-genome sequences of B. plantarii strains and other related species within the Burkholderia genus. The resulting tree confirmed the taxonomic positions and grouped KACC 18964 with other strains of B. plantarii (Figure 2C).
Figure 2 Comparative genomic analysis of B. plantarii KACC 18964 with other B. plantarii strains (ATCC 43733, PG1, and ZJ171) and B. glumae BGR1 as a congeneric control for differentiation. (A) Pairwise comparison of average nucleotide identity (ANI) among KACC 18964, the other B. plantarii strains (ATCC 43733, PG1, and ZJ171), and B. glumae BGR1. (B) Whole genome alignment illustrating the differences in digital DNA-DNA hybridization (dDDH) among KACC 18964, the other B. plantarii strains (ATCC 43733, PG1, and ZJ171), and B. glumae BGR1. The ANI and dDDH% values were found to be consistent with the proposed and widely accepted species boundary thresholds of 95–96% and 70%, respectively. (C) Phylogenomic tree constructed using TYGS (https://tygs.dsmz.de/), inferred with FastME 2.1.6.1, based on genome GBDP distances calculated from the whole genome sequences of B. plantarii-related species. The branch lengths are scaled using the GBDP formula d5, with an average branch support of 99.8%. The tree is rooted at the midpoint. (D) Matrix of presence/absence genes generated using Roary software, accompanied by the maximum-likelihood phylogenetic tree of B. plantarii strains (KCCM 18964, ATCC 43733, PG1, and ZJ171) and B. glumae BGR1. Blue bars indicate the presence of specific genes across the panel, with genes shared among all B. plantarii strains outlined in red (core genome).
A schematic representation of the pan-genome analysis findings of the four B. plantarii strains and B. glumae BGR1 revealed the presence or absence of genes in the core and accessory genomes (Figure 2D). Furthermore, the maximum likelihood phylogenetic tree constructed using the core and accessory genomes of the examined strains revealed the formation of a distinct cluster for B. plantarii strains, separate from the congeneric B. glumae BGR1. Specifically, strain KACC 18964 clustered with strain ATCC 43733, whereas strain PG1 clustered with strain ZJ171 (Figure 2D).
The genome of B. plantarii KACC 18964 sequenced in this study served as a representative for investigating putative secondary metabolite gene clusters using antiSMASH. The analysis revealed 20 different gene clusters associated with putative secondary metabolites. Seven gene clusters were identified on chromosome I: (1) type I polyketide synthase (PKS) and non-ribosomal peptide synthetase (NRPS)-like fragment, (2) terpene, (3) NRPS, (4) NRPS-like fragment, (5) type I PKS, (6) homoserine lactone, and (7) NRPS. On chromosome II, 13 gene clusters were detected, including (1) phosphonate, (2) NRPS, (3) type I PKS, (4) NRPS-like fragment and β-lactone containing protease inhibitor, (5) non-ribosomal peptide metallophores and NRPS, (6) NRPS, (7) β-lactam, (8) terpene, (9) redox-cofactors such as PQQ, (10) terpene, (11) NRPS, β-lactone containing protease inhibitor, and type I PKS, (12) β-lactone containing protease inhibitor and terpene, and (13) NRPS and homoserine lactone. Figure 3A demonstrates the positions of the secondary metabolite gene clusters detected in the B. plantarii KACC 18964 genome. Figure 3B shows the representations of the gene clusters and highlights the main functions of the genes involved.
Figure 3 Predicted secondary metabolite gene clusters in B. plantarii KACC Identified by antiSMASH (version 7). (A) A circular representation of the whole genome indicating the locations of the detected secondary metabolite gene clusters. (B) Organization of gene clusters and the predicted classes of secondary metabolites. The secondary metabolite types are abbreviated as follows: T1PKS, Type I PKS (Polyketide synthase); NRPS-like, Non-ribosomal peptide synthetase-like fragment; NRPS, Non-ribosomal peptide synthetase; hserlactone, Homoserine lactone; beta lactone, Beta-lactone containing protease inhibitor; NRP-metallophore, Non-ribosomal peptide metallophores; lactam, β-lactam; redox-cofactor, Redox-cofactors such as PQQ (NC_021985:1458906–1494876).
Among the identified gene clusters, 12 displayed varying degrees of similarity to known secondary metabolite clusters, whereas eight did not exhibit any similarity to known clusters (Supplementary Table S1). Notably, clusters 11 and 12 showed 100% similarity to Plantaribactin clusters found in other B. plantarii strains (Hermenau et al., 2019), and Rhizomide A/Rhizomide B/Rhizomide C clusters from Paraburkholderia rhizoxinica (Wang et al., 2018), respectively. Cluster 10 exhibited 62% similarity with fragin gene clusters in Burkholderia cenocepacia (Jenul et al., 2018). Furthermore, cluster 9 showed 41% similarity to Yersiniabactin from Pseudomonas syringae pv. tomato (Jones et al., 2007). Additional similarities were observed, with cluster 18 sharing 33% similarity to Barbamide from Lyngbya majuscule (Chang et al., 2002), cluster 5 exhibiting 25% similarity to capsular polysaccharide from Mannheimia haemolytica (Lo et al., 2001), cluster 17 displaying 20% similarity to Bacillomycin D from Bacillus velezensis (Koumoutsi et al., 2004), and cluster 13 showing 16% similarity to 1-carbapen-2-em-3-carboxylic acid from Pectobacterium carotovorum (McGowan et al., 1997), cluster 15 sharing 13% similarity to Lankacidin C from Streptomyces rochei (Suwa et al., 2000), cluster 19 exhibiting 9% similarity to Bactobolin from Burkholderia thailandensis (Carr et al., 2011), cluster 8 showing 6% similarity to phosphinothricin tripeptide from Streptomyces viridochromogenes (Schwartz et al., 2004), and cluster 4 displaying 3% similarity to Pyoverdine DC3000 from P. syringae pv. tomato (Ravel and Cornelis, 2003).
The genomes of B. plantarii strains (ATCC 43733, KCCM 18964, PG1, and ZJ171) were examined to identify genes associated with the QS system. All four strains were found to have the plaI, plaM, and plaR genes involved in QS. Comparison of the organization of these genes revealed that strains ATCC 43733 and KCCM 18964 clustered together in the phylogenetic analysis, indicating their similarity, whereas strains PG1 and ZJ171 formed a separate cluster (Figure 4A).
Figure 4 Comparison of gene cluster organization and phylogenetic tree based on sequence relatedness of (A) quorum sensing system and (B) tropolone biosynthesis genes among Burkholderia plantarii strains (ATCC 43733, KCCM 18964, PG1, and ZJ171).
Furthermore, the regulatory genes associated with tropolone production, namely troR1, troR2, and troK, were identified in all four B. plantarii strains, exhibiting a high level of similarity. In the phylogenetic analysis, strains ATCC 43733 and KCCM 18964 displayed greater similarity and clustered together, whereas strains PG1 and ZJ171 were similar and formed a distinct cluster (Figure 4B).
These findings imply the common presence of the QS system and tropolone biosynthesis genes among the studied B. plantarii strains. The clustering patterns observed in the phylogenetic analysis highlighted genetic similarities and potential evolutionary relationships between the strains.
The classification of CRISPR systems primarily relies on the sequences of Cas genes, direct repeats within CRISPR arrays, and the organization of Cas operons. Within the genomes of the four B. plantarii strains (ATCC 43733, KCCM 18964, PG1, and ZJ171), six Cas genes were identified. Phylogenetic analysis based on the sequence relatedness of these Cas genes within the CRISPR system revealed distinct clustering patterns (Figure 5A). The organization of Cas genes and phylogenetic analysis of concatenated Cas genes in B. plantarii strains are presented in Figure 5B.
Figure 5 Comparative analysis of Cas genes and CRISPR spacers within the genomes of B. plantarii strains (ATCC 43733, KCCM 18964, PG1, and ZJ171). (A) Phylogenetic analysis based on sequence relatedness of the six detected Cas genes within the CRISPR systems. (B) Organization of Cas genes and phylogenetic analysis of the concatenated Cas genes in B. plantarii strains. (C) CRISPRStudio alignment of the arrays for three CRISPR systems, illustrating the schematic representation of spacer genes within the genomes of B. plantarii strains and B. glumae BGR1. The analysis reveals the absence of shared spacers between B. glumae BGR1 and B. plantarii strains, while all the spacers of CRISPR system 1 are common among the B. plantarii strains.
Figure 5C illustrates the CRISPRStudio alignment of arrays for the three CRISPR systems, depicting a schematic representation of spacer genes within the genomes of the B. plantarii strains and B. glumae BGR1. All four B. plantarii strains have CRISPR 1 and 2 spacers, with PG1 and ZJ171 also having CRISPR 3 spacers. The analysis further indicated the absence of shared spacers between the B. glumae BGR1 and B. plantarii strains. Notably, all spacers of CRISPR system 1 were common among the B. plantarii strains.
To explore sequence variations and conservation, multiple alignments of CRISPR repeats 1–3 were performed in B. plantarii strains (ATCC 43733, KCCM 18964, PG1, and ZJ171) and B. glumae BGR1 (Figure 6). Multiple CRISPR systems were identified in the B. plantarii strains, with most strains possessing two systems, whereas strains PG1 and ZJ171 had three systems. In B. glumae BGR1, CRISPR 1 system repeats were detected, showing high similarity to CRISPR 2 in B. plantarii. Notably, a discrepancy was observed at the 12th position, where T was present instead of C. Sequence variations and conservation among the tested strains were further analyzed using Weblogo (Figure 6).
Figure 6 Multiple alignment of CRISPR 1–3 repeats in B. plantarii strains (ATCC 43733, KCCM 18964, PG1, and ZJ171) and B. glumae BGR1, and Weblogo analysis highlighting sequence variations and conservation among the examined strains. Multiple CRISPR systems were identified in B. plantarii strains, with most strains having two systems. However, strains PG1 and ZJ171 exhibited three CRISPR systems. In B. glumae BGR1, CRISPR 1 system repeats were detected, and their sequence showed a high similarity to CRISPR 2 in B. plantarii. Notably, a discrepancy was observed at the 12th sequence position, where T was present instead of C.
The comparative genomic analysis using IslandCompare v1.0 revealed a complex network of GIs across the four genomes of B. plantarii. The phylogenetic tree elucidates the evolutionary relationships among the strains, while the linear genome maps display the GIs as color-coded blocks. Homologous regions across the genomes indicate the shared evolutionary history and potential for horizontal gene transfer (Figure 7A).
Figure 7 (A) Comparative visualization of the four Burkholderia plantarii genomes, generated using IslandCompare, highlights the genomic islands (GIs) and antimicrobial gene determinants. A phylogenetic tree, positioned on the upper left, elucidates the evolutionary relationships among the strains. Genomic islands (GIs) are represented as color-coded blocks aligned along the linear genome maps, (B). Distribution of predicted GIs in the B. plantarii KACC18964 genome, as analyzed by IslandViewer 4. The circular map marks the GIs with a color-coding scheme that reflects various predictive criteria. The figure captures the genomic complexity and identifies potential hotspots for gene exchange that could influence the bacterial pathogenicity and environmental adaptation. Regions associated with potential features relevant to bacterial virulence and fitness are notably highlighted, including those containing genes related to transport, toxins, and secretion. Labels indicating the start and end points of the GIs facilitate an understanding of their genomic context.
IslandViewer 4 analysis revealed 59 unique GIs within the genome of B. plantarii KACC18964 (Figure 7B). These islands are marked with a color-coding scheme reflecting different predictive criteria, providing insights into the genomic complexity and identifying potential hotspots for gene exchange. The GIs were evaluated for their gene content, revealing clusters of genes potentially involved in virulence, including toxins, effectors, transport, and secretion systems-related genes, indicative of bacterial environmental adaptability and pathogenicity. All the gene contents of the predicted genomic islands, specifying their start and end points, and gene annotations are listed (Supplementary Table S4).
In addition to the annotated GIs, the genomic landscape was predominantly characterized by genes encoding “hypothetical proteins” (247 instances), underscoring the vast unexplored genetic content within these GIs.
A comparative analysis of the predicted secretion systems among the four strains of B. plantarii (ATCC 43733, KCCM 18964, PG1, and ZJ171) revealed similarities in the types of secretion systems with minor variations. Strain KACC 18964 lacked the Type I secretion system (T1SS), which was present in the other three strains. The number of genes in the Type II secretion system (T2SS) was higher in ZJ171 than in the other strains. The Type IV secretion system, type F (T4SS_typeF) was present in KACC 18964 and ATCC 43733 but not in ZJ171 and PG1. T4SS_typeT was absent in PG1 but present in the other three strains. All strains shared the presence of Tad, T3SS, Type Vb secretion system (T5SSb), and Type VI secretion system (T6SSi). A comparison of the number of genes in each strain and phylogenetic analysis based on gene count is shown in Figure 8A.
Figure 8 Comparison of secretion system gene clusters within the genomes of B. plantarii strains (ATCC 43733, KCCM 18964, PG1, and ZJ171). (A) A heatmap showing the number of genes identified within the detected secretion systems. (B) Comparative analysis of gene clusters from different detected secretion systems among B. plantarii strains. The type I secretion system was present in all four strains. Strain ZJ171 exhibited an additional Type II secretion system cluster. The type III secretion system showed slight variations between ATCC 43733 and ZJ171, as well as KCCM 18964 and PG1. The type IV secretion system differed among the strains, and PG1 lacked this gene cluster. The type VI secretion system exhibited the same structure and configuration across all four tested strains.
The structures of the secretion system gene clusters in the four B. plantarii strains were compared and demonstrated in a schematic representation of the gene clusters in Figure 8B. The T1SS was identical in strains ATCC 43733, PG1, and ZJ171 but absent in KACC 18964. The T2SS was conserved in ATCC 43733, PG1, and KACC 18964, while in ZJ171, slight differences were observed in the identification of two clusters. Tad was conserved in the same structure in all strains. T3SS was identified in all strains, and although it was identical in ATCC 43733 and ZJ171, minor variations were observed compared with the T3SS in PG1 and KACC 18964. The flagellum gene cluster was highly conserved in ATCC 43733, PG1, and KACC 18964, with slight variation from the gene cluster in ZJ171. T5SS and T6SSi were identical in all the strains (Figure 8B).
Potential effector genes were predicted using four different algorithms, namely EffectiveELD, Predator, EffectiveCCBD, and EffectiveT3, in B. plantarii strains (ATCC 43733, KCCM 18964, PG1, and ZJ171) and B. glumae BGR1. The inclusion of B. glumae BGR1 in the analysis was justified by its possession of a T3SS. The prediction results were visualized using a Venn diagram presenting the number of potential effectors identified (Figure 9A). Notably, a single gene was predicted using all four algorithms in all B. plantarii strains, and the same gene was detected in the B. glumae strain using the EffectiveCCBD algorithm. Consequently, this gene, which encodes the potential effector protein aroA, is suspected to function as a T3SS effector.
Figure 9 Analysis of effectors from the type III secretion system in B. plantarii strains (ATCC 43733, KCCM 18964, PG1, and ZJ171). and B. glumae BGR1. (A) Venn diagram demonstrating the analysis of effector genes using four different system criteria (EffectiveELD, Predator, EffectiveCCBD, and EffectiveT3). The number of detected effector genes is shown, with only one gene (highlighted as a red star) found in all analyses. This gene was also detected in B. glumae using the EffectiveCCBD criteria. (B) Phylogenetic tree generated using the maximum likelihood method with 1000 bootstrap replications, highlighting the evolutionary relationship of the aroA from Burkholderia plantarii (highlighted in yellow) against a backdrop of homologous sequences. Sequences were retrieved following a BLASTp search against the clustered non-redundant (nr) protein database of the NCBI, where each sequence is clustered at 90% identity and coverage. The representative sequence for each cluster is indicated, providing a compact result with increased taxonomic depth. The bootstrap values are represented by the size of the nodes, with larger nodes indicating higher support for the clade. The tree scale indicates the number of substitutions per site.
To provide a broader evolutionary context and underscore the ubiquity of the aroA gene, a phylogenetic analysis was performed. The sequences for this analysis were retrieved from a BLASTp search against the NCBI’s clustered non-redundant (nr) protein database, where sequences are clustered at 90% identity and coverage. The resulting phylogenetic tree, as depicted in Figure 9B, employs the maximum likelihood method with 1000 bootstrap replications. It distinctly positions the aroA gene of B. plantarii among a backdrop of homologous sequences from related organisms, highlighting its evolutionary conservation. This analysis does not directly confirm the effector functionality of aroA but provides essential insights into its conservation and potential roles within these bacterial species.
The results of the in planta assays confirmed the involvement of the aroA gene in B. plantarii KACC 18964 virulence in rice. As observed in the photographs in Figure 10A, blight symptoms on the rice panicles treated with the mutant ΔaroA showed a noticeable reduction compared to those treated with wild-type B. plantarii KACC 18964 and the complemented strain CaroA.
Figure 10 In planta assays for evaluation of the disease severity between the wild-type B. plantarii KACC18964, the mutant (ΔaroA), and the complemented strain (CaroA) (A) Disease severity in rice plants 10 d post-inoculation. Photographs depicting the variation in blight symptoms between the treatments. The bar graph on the right side illustrating the disease severity results in inoculated rice plants. Different letters on the error bars (Standard error, n = 5) indicate significant differences between the treatments, according to the least significant difference test at P < 0.05. A sterilized 1 mM MgSO4 solution was used as the negative control. (B) Rice seedling growth and disease severity post-inoculation. Photographs displaying the seedlings post-treatment with respective bacterial strains or control. The bar graph on the right side are showing the quantified shoot and root lengths. Seedlings treated with the ΔaroA deletion mutant exhibit less disease severity and greater shoot and root growth compared to those inoculated with the wild type, whereas complementation of the aroA mutant restores virulence to levels comparable to the wild type. Control seedlings treated with sterilized 1 mM MgSO4 showed no disease symptoms nor growth impairment, indicating healthy development. Different uppercase and lowercase letters on the error bars (standard error; n=30), indicate significant differences according to the LSD test at P < 0.05 for the shoot and root length, respectively.
Disease severity was assessed in accordance with this observation. A significant (P < 0.05) reduction in the evaluated disease severity was observed in rice treated with the mutant ΔaroA, compared to treatment with the wild-type KACC 18964 and the complemented strain CaroA. The disease severity in rice treated with the complemented strain, CaroA, was not significantly different from that in wild-type-treated rice (Figure 10A), indicating the recovery of virulence by complementation with aroA. These results confirm the involvement of aroA deletion in the partial impairment of B. plantarii virulence in rice.
The outcomes of the seedling development assay were consistent with those of the rice plant assay. The ΔaroA mutant of B. plantarii showed a reduction in pathogenicity. This was evidenced by enhanced seedling growth and decreased disease severity in comparison to the wild-type strain. Control seedlings maintained healthy development and exhibited no signs of disease, as depicted in the photographs (Figure 10B). Quantitative analysis revealed a significant increase (P < 0.05) in both shoot and root lengths in seedlings treated with the ΔaroA mutant. In contrast, the complemented strain displayed a level of pathogenicity comparable to the wild-type strain, as indicated in (Figure 10B).
In regards to the colonization of rice plant tissues, the bacterial population derived from seedlings treated with the wild-type strain was equivalent to 2.86 ± 0.95 log CFU/g; n=3, which was slightly higher than that of both the ΔaroA mutant (2.85 ± 0.88 log CFU/g) and the complemented strain, CaroA (2.73 ± 0.88 log CFU/g). However, there were no statistically significant differences between the wild type and either the mutant or the complemented strains.
The growth curves revealed distinct differences between the WT, ΔaroA mutant, and CaroA complemented strains. While the WT and CaroA strains exhibited similar growth patterns, the ΔaroA mutant showed a notable reduction in optical density, particularly at the start of the logarithmic phase. Despite this reduction, the ΔaroA mutant reached a final optical density comparable to that of the WT and CaroA strains, suggesting a temporary growth impairment (Figure 11A).
Figure 11 Comparative analysis of growth, oxidative stress tolerance, and protease activity in Burkholderia plantarii wild-type KACC18964, the mutant (ΔaroA), and the complemented strain (CaroA). (A) Growth curves for the WT, ΔaroA, and CaroA strains, indicating the optical density (OD600) measurements over 6-h intervals. Different letters above the error bars (Standard error; n=3) indicate statistically significant differences (P < 0.05) between strains at the corresponding time points. (B) Disk diffusion assay for H2O2 sensitivity showing zones of inhibition around disks soaked in various H2O2 concentrations. (C) Extracellular protease activity assays with clear proteolysis zones on LB agar plates containing 2% skim milk, indicating protease secretion. Observed clear zones were comparable for the oxidative stress tolerance, and protease activity suggesting no difference between strains.
For the oxidative stress and protease activity, the aroA deletion mutant demonstrated an unchanged tolerance to H2O2 when compared to the wild-type and complemented strains, as observed by similar inhibition zones around the H2O2 disks. Additionally, protease activity assays showed no significant differences among the strains, suggesting that aroA deletion does not impair the strain’s ability to produce extracellular proteases (Figures 11B, C).
For bacterial motility, after incubation, images captured from both swarming (Figure 12A) and swimming (Figure 12B) assays showed that the bacterial spread on the agar surfaces was comparable across all three strains. Quantitative analysis using ImageJ software confirmed that there were no statistically significant differences in the surface area of growth spread among the wild-type, aroA deletion mutant, and aroA-complemented strains (Figure 12).
Figure 12 Comparative analysis of Burkholderia plantarii KACC 19864 wild-type, aroA deletion mutant, and aroA-complemented strains motility assays. (A) Swimming motility was assessed on 0.3% agar LB plates, and (B) swarming motility assay on 0.5% agar LB plates. After incubation, plates were imaged and the spread of bacterial growth was measured using ImageJ software. The bar graphs on the right side demonstrate the surface area of motility spread, with bars representing the mean from 3 replicates. Error bars represent standard deviation, illustrating no significant difference in motility among the strains.
This study aimed to perform genome mining analysis of B. plantarii using all available complete and nearly complete genomes. In particular, the genome of B. plantarii strain KACC 18964 was sequenced in this study and compared with other available sequences. Whole-genome comparison using B. glumae BGR1 as a congeneric control indicated the taxonomic position of KACC 18964 as a B. plantarii strain. The genome of B. plantarii is relatively large, ranging from 7.90 to 8.08 Mb, and comprises two chromosomes and one plasmid. Notably, there was little variation in the genome size among the studied B. plantarii genomes, in contrast to the significant variation observed in the sizes of B. glumae genomes that have been previously reported. For example, B. glumae strain AU6208 exhibited the smallest genome size (approximately 4.9 Mbp), whereas the BGR1 strain had the largest genome size (approximately 7.2 Mbp). The relatively small genome size of B. glumae AU6208 may be attributed to genome rearrangements or deletions resulting from its adaptation to a different host.
In a previous comparative genomic study of phytopathogenic Burkholderia species, Seo et al. (2015) analyzed the genome sequences of different strains of B. glumae, B. gladioli, and B. plantarii and identified variations in genome size between species as well as among different strains of the same species. The large genome size of B. plantarii with multiple replicons indicates a versatile lifestyle that allows it to thrive in soil and infect plants. Larger genomes are thought to require more complex regulation of gene expression and an increased number of genes encoding regulatory proteins, and genome size and content are largely influenced by environmental pressures (Bentley and Parkhill, 2004; Mannaa et al., 2018).
The large B. plantarii genome harbors a diverse array of secondary metabolite gene clusters, as evidenced by the detection of 20 different secondary metabolite gene clusters using anti-SMASH analysis of the KACC 18964 genome. These metabolites likely confer various ecological benefits to B. plantarii, enabling their survival, interactions, and competition with the surrounding organisms. Among the detected clusters, 12 showed varying degrees of similarity to known gene clusters for secondary metabolites.
Notably, a cluster encoding non-ribosomal peptide metallophores and a non-ribosomal peptide synthetase displayed 100% similarity to a gene cluster encoding plantaribactin, a compound previously isolated and characterized from B. plantarii (DSM9505 = ATCC43733). This cluster has also been identified in other plant-associated Burkholderia and Paraburkholderia species (Hermenau et al., 2019). Plantaribactin is an iron-chelating and nitric oxide-donating diazeniumdiolate siderophore that, like other siderophores, is used by many bacterial pathogens to overcome iron limitations in the host environment. Therefore, plantaribactin may play a key role in conferring fitness benefits to microbes (Lamont et al., 2002; Johnson et al., 2013; Palmer and Skaar, 2016).
Additionally, another cluster with 100% similarity to a secondary metabolite gene cluster encoding a non-ribosomal peptide synthetase was detected, which was similar to the rhizomides found in Paraburkholderia rhizoxinica. Previous studies have demonstrated that rhizomes possess weak antibacterial properties, implying potential benefits for bacterial fitness (Wang et al., 2018). Furthermore, a gene cluster encoding a non-ribosomal peptide synthetase-like fragment was detected that exhibited 62% similarity to a cluster associated with fragin in B. cenocepacia (Jenul et al., 2018). While fragin is known for its antifungal properties and potential metal chelation activity, the similarity noted does not confirm the production of fragin or its related compounds in B. plantarii.
A cluster encoding a non-ribosomal peptide synthetase and type I polyketide synthase demonstrated 41% similarity to the siderophore yersiniabactin from P. syringae pv. Tomato (Jones et al., 2007). Another cluster encoding a non-ribosomal peptide synthetase, beta-lactone-containing protease inhibitor, and type I polyketide synthase showed 20% similarity to bacillomycin D from Bacillus velezensis (Koumoutsi et al., 2004). Bacillomycin D, which is produced by several Bacillus strains, has been shown to confer antagonistic activity against various microorganisms, including Candida spp., Aspergillus flavus, and Fusarium graminearum (Moyne et al., 2001; Olfa et al., 2015; Gu et al., 2017). Notably, several other secondary metabolite biosynthetic gene clusters showed low levels of similarity or no similarity to clusters of known metabolites, indicating that B. plantarii KACC 18964 could be a valuable source for studying novel bioactive secondary metabolites.
The secondary metabolite gene clusters identified in B. plantarii KACC 18964 genome not only demonstrate the bacterial potential for producing a wide spectrum of bioactive compounds but also reflect the intricate biological processes underlying its interaction with the environment. Particularly, the presence of diverse biosynthetic pathways, such as those for non-ribosomal peptides, polyketides, and terpenes, underscores the organism’s adaptability and underscores its potential to influence and thrive in various ecological niches (Raaijmakers and Mazzola, 2012). Future studies focusing on the expression, regulation, and functional analysis of these pathways can provide deeper insights into their roles in the organism’s life cycle and interactions, as well as their potential utility in various applications.
Another critical feature of B. plantarii is its ability to produce tropolone, a phytotoxin that represents the most significant virulence factor causing disease in rice (Azegami et al., 1987). Tropolone is a non-benzenoid aromatic compound that exhibits properties similar to those of phenols and acids. This compound possesses both antimicrobial and phytotoxic properties (Trust, 1975; Azegami et al., 1987). As a biotoxin, tropolone acts as a virulence factor for plant-pathogenic bacteria and displays varying degrees of toxicity towards several organisms. Consequently, its presence poses potential risks to agricultural production, plant-soil-water ecosystems, and human health (Liu et al., 2018).
Our results demonstrated the conservation of the operon consisting of three essential genes (troR1, troK, and troR2) responsible for regulation of tropolone biosynthesis across all examined B. plantarii genomes. Early experiments suggested that tropolone exposure led to disease manifestations in certain plants, including rice; however, others, such as Gladiolus gandavensis, remained symptom-free when treated solely with the phytotoxin. Notably, these gladiolus plants exhibited disease symptoms when inoculated with the toxin-producing bacterium B. plantarii. These findings imply that factors other than tropolone play significant roles in the virulence of B. plantarii (Iiyama et al., 1998).
The QS system, a critical cell density-dependent regulatory mechanism, is prevalent in various bacterial genera, including Burkholderia, and influences myriad bacterial biological processes and virulence characteristics (Mannaa et al., 2018). In B. glumae, the principal virulence factor, toxoflavin, is governed by the LysR family regulator, ToxR, which functions as a coinducer. Another regulatory element, ToxJ, plays a significant role in the regulation of the toxoflavin operon, and its expression is mediated by the TofI or TofR components of the QS system (Kim et al., 2004; Seo et al., 2015). The QS system regulates the transcription of specific genes at quorum concentrations in response to the accumulation of signal molecules in the surrounding medium. In gram-negative bacteria, N-acyl homoserine lactone (AHL) signaling molecules generated by an AHL synthase enzyme of the LuxI protein family are primarily used. AHL molecules bind to the transcriptional regulators of the LuxR family at quorum concentrations, thereby influencing the transcriptional regulation of target genes (Fuqua et al., 1994). This mechanism provides significant advantages to bacterial communities, particularly those that produce extracellular enzymes and virulence factors.
In B. plantarii, the QS system operon—comprising plaI, plaM, and plaR genes—closely resembles the corresponding system in B. glumae, as demonstrated by the analysis of four B. plantarii strains in which it was conserved. The AHL-QS mechanism in B. plantarii plays a pivotal role in the progression of rice seedling blight. Studies involving the generation of plaR knockout mutants highlight the significance of the AHL-QS regulatory system in the pathogenicity of B. plantarii, because disabling the PlaIR function delayed the onset of rice seedling blight significantly (Solis et al., 2006). Subsequent studies revealed that the application of AHL-QS inhibitors resulted in reduced tropolone production due to inhibited plaI expression (Wang et al., 2013). This discovery draws parallels between the regulation of toxoflavin in B. glumae and that of tropolone in B. plantarii, highlighting the importance of QS-related genes. However, the exact role of the QS system in B. plantarii requires further investigations.
Previously, the role of the CRISPR system was considered to be limited to safeguarding bacteria against invasion by bacteriophages or foreign plasmid DNA (Jansen et al., 2002). However, recent perspectives have expanded to include its broader ecological implications, such as its potential influence on virulence, biofilm formation in pathogenic bacteria, and as a barrier to horizontal gene transfer. Furthermore, research has revealed that environmental stimuli can modulate the expression of the CRISPR system and its role as a regulator of gene expression (Hatoum-Aslan and Marraffini, 2014; Louwen et al., 2014; Gao et al., 2015). Our findings indicated that the B. plantarii genome harbors near-complete CRISPR-Cas systems, unlike other rice-associated Burkholderia species. These systems encompassed a series of Cas genes that were conserved across the four examined B. plantarii genomes. We also observed high conservation levels within the direct repeats of the CRISPR systems across these genomes. These findings imply that the CRISPR systems in B. plantarii could serve distinct roles that subtly differentiate them from other closely related rice-pathogenic bacteria (i.e., B. glumae and B. gladioli) despite their similarities (Seo et al., 2015).
In strains ATCC 43733 and KCCM 18964, we identified two CRISPR systems, whereas PG1 and ZJ171 had three. The sequence of CRISPR 3 was consistent between PG1 and ZJ171. A previous study on B. plantarii PG1 detected three CRISPR arrays, indicating their potential importance in plant interactions (Gao et al., 2015), and reported the QS-dependent regulation of CRISPR/Cas genes, which may affect the bacterial immune system in B. plantarii PG1. The detection of nearly complete CRISPR systems in B. plantarii genomes emphasizes the necessity for further exploration of their possible functional roles.
The analysis of genomic islands across the examined genomes uncovered a variety of consistent GIs among different strains. Detailed study of B. plantarii KACC 18964 as representative genome revealed the prediction of 59 unique genomic islands, rich in genes of uncharacterized function and mobile genetic elements, indicates the complex genetic architecture that may fortify the ecological fitness and pathogenic potential of the bacterium (Juhas et al., 2009; Langille and Brinkman, 2010). These GIs likely function as reservoirs for virulence-related genes, underscoring the bacterial ability to adapt and interact with plant hosts.
The identification of specific virulence and fitness-related genes within these islands, such as toxin family proteins and type VI secretion system effector proteins, aligns with previous studies underscoring the role of such genes in bacterial competitiveness and host interaction (Russell et al., 2011; Hood et al., 2010). These genetic elements may contribute to the bacterial ability to affect rice plant health directly through pathogenic mechanisms or indirectly through competitive suppression of beneficial microbiota.
The presence of mobile genetic elements and toxin-antitoxin systems suggests a genetic toolkit for rapid adaptation and survival under selective pressures, such as plant immune responses or microbial competition (Hacker and Carniel, 2001).
Further research should investigate the functional roles of these GIs, particularly their gene expression in response to environmental stimuli and their influence on the host-pathogen interface.
The ability of gram-negative bacteria, including Burkholderia species, to regulate the translocation of powerful effectors from their synthesis sites to the cellular exterior or even to neighboring organisms is a crucial survival and communication mechanism (Green and Mecsas, 2016). Burkholderia spp., as demonstrated by genomic studies, exhibit diverse secretion systems, each potentially playing a key role in virulence, communication, and competitive interaction with other microbes (Seo et al., 2015). The present study indicates that the four B. plantarii genomes have various types of secretion systems, including T1SS, T2SS, and T3SS; several clusters of T4SS, T5SS, and T6SS; and associated genes. Different types of secretion systems can serve unique functions, but different clusters of a particular secretion system may also play distinctive roles. Kim et al. (2020) reported such functional distinctions among T6SS clusters in the related species B. glumae; one cluster was associated with virulence in rice plants, whereas the other was connected to bacterial competition within the surrounding microbial community. These traits are essential for B. glumae to compete effectively, establish a population, and induce disease in rice.
Among the various secretion systems in gram-negative bacteria, the T3SS serves as an effective molecular syringe, enabling gram-negative bacteria to penetrate, grow, and survive in eukaryotic hosts. Numerous animal and plant pathogens utilize T3SS to inject a suite of proteins, known as bacterial ‘effector proteins,’ into the cytosol of host cells. These proteins can modulate eukaryotic regulatory or signaling pathways during bacterial infection, causing a range of symptoms (Puhar and Sansonetti, 2014; Radics et al., 2014). Therefore, our study aimed to examine the potential involvement of the T3SS in B. plantarii virulence in rice by investigating the potential effector proteins. T3SS was conserved across the genomes of the four B. plantarii strains examined. In silico analysis using different algorithms for potential effectors yielded a large number of potential effectors. To prioritize the most promising candidates, we focused on the aroA gene, a potential effector that was detected by all algorithms in the four studied B. plantarii genomes.
The aroA gene, which is ubiquitous in many bacterial species, encodes the enzyme 3-phosphoshikimate 1-carboxyvinyltransferase, which is a crucial participant in the shikimate pathway. This pathway is responsible for aromatic amino acid synthesis (Duncan et al., 1984). Notably, the shikimate pathway is present in plants and various microorganisms but is absent in mammals, making it an ideal target for herbicidal strategies. For instance, glyphosate (commercially known as Roundup), a common herbicide, functions by inhibiting the synthase enzyme, disrupting the production of essential amino acids in plants and consequently leading to their demise (Funke et al., 2006).
Our study revealed that an aroA deletion mutant of B. plantarii KACC 18964 significantly reduced bacterial virulence in rice plants. This finding is consistent with previous research, where deletion of the aroA gene in B. glumae similarly impaired its virulence in rice (Karki and Ham, 2014). However, it is essential to clarify the exact role of aroA, identified here as a potential effector of T3SS. We are currently investigating whether the reduced virulence in aroA deletion mutant, results from changes in the bacterial ability to produce certain metabolites or whether it can be attributed to the role of aroA as a T3SS-mediated effector. The unchanged oxidative stress response, protease activity and motility in the ΔaroA mutant alongside the temporary growth delay suggest that aroA plays a non-essential role in these specific traits. The ability to withstand oxidative stress is a vital trait for pathogens, allowing them to survive the burst of reactive oxygen species produced as a part of the plant’s innate immune response (O’Brien et al., 2012). Similarly, extracellular proteases play a critical role in phytopathogen virulence by degrading plant structural proteins, facilitating bacterial invasion, and disabling plant defense proteins (Figaj et al., 2019). The absence of significant changes in protease activity and H2O2 tolerance suggests that aroA role in virulence may not directly be linked to these mechanisms but could instead be related to pathogenic interactions within the plant host. However, the observed growth pattern disruption indicates its potential involvement in bacterial metabolism. This supports the hypothesis that aroA could be serving dual functions: as an enzyme within the shikimate pathway and, potentially, as a T3SS effector that interferes with plant metabolism through molecular mimicry. This dual functionality hypothesis could be supported by the understanding that T3SS effectors often mimic eukaryotic proteins to facilitate pathogenicity, coupled with the fact that the shikimate pathway, crucial for the synthesis of aromatic amino acids, is present in both plants and bacteria. The conservation of this pathway across different biological kingdoms provides a molecular basis for aroA multifunctionality, enabling it to participate in bacterial metabolism and, when introduced into plant cells, to potentially interfere with analogous metabolic processes through molecular mimicry (Coburn et al., 2007; Dean, 2011; Shende et al., 2024). The aroA product may have evolved to acquire an entirely new function as a virulence factor when secreted into host cells. These fundamental questions about aroA function in B. plantarii pathogenesis remain to be explored. Further investigation is crucial to substantiate these hypotheses and to elucidate the precise roles of aroA, particularly in validating its bifunctional nature—operating both within the bacterium and within the host plant as a T3SS effector.
In conclusion, this comprehensive study offers novel insights into the genomic features, virulence, and ecological adaptability of the rice pathogenic B. plantarii. Through meticulous genomic analysis, including comparison among B. plantarii genomes and identification of unique secondary metabolite gene clusters, our research elucidates the complex genetic make-up that potentially underlies B. plantarii pathogenicity and environmental interactions. The detailed investigation of CRISPR-Cas systems and genomic islands further accentuates the genetic diversity and evolutionary potential of B. plantarii, highlighting its adaptability and the dynamic nature of its interactions within diverse environments. Our findings not only contribute to the understanding of B. plantarii genomic complexity and pathogenic mechanisms but also open avenues for future research focused on exploring the functional roles of identified gene clusters and aroA, as a potential T3SS effector.
The original contributions presented in the study are included in the article/Supplementary Material. Further inquiries can be directed to the corresponding author.
MM: Conceptualization, Data curation, Formal analysis, Investigation, Writing – original draft, Writing – review & editing. DL: Conceptualization, Data curation, Methodology, Writing – original draft. H-HL: Conceptualization, Data curation, Methodology, Software, Writing – original draft. GH: Data curation, Formal analysis, Methodology, Writing – review & editing. MK: Data curation, Formal analysis, Methodology, Writing – review & editing. T-JK: Visualization, Writing – review & editing. JP: Formal analysis, Writing – review & editing. Y-SS: Conceptualization, Funding acquisition, Investigation, Project administration, Resources, Supervision, Validation, Visualization, Writing – review & editing.
The author(s) declare financial support was received for the research, authorship, and/or publication of this article. This research was supported by the Basic Science Research Program of the National Research Foundation (NRF), funded by the Ministry of Education (2022R1A2C1003190 and 2022R1A4A5031503), Republic of Korea.
We thank Prof. Hokeun Son for helping with the comparative genome analyses.
The authors declare that the research was conducted in the absence of any commercial or financial relationships that could be construed as a potential conflict of interest.
The author(s) declared that they were an editorial board member of Frontiers, at the time of submission. This had no impact on the peer review process and the final decision.
All claims expressed in this article are solely those of the authors and do not necessarily represent those of their affiliated organizations, or those of the publisher, the editors and the reviewers. Any product that may be evaluated in this article, or claim that may be made by its manufacturer, is not guaranteed or endorsed by the publisher.
The Supplementary Material for this article can be found online at: https://www.frontiersin.org/articles/10.3389/fpls.2024.1416253/full#supplementary-material
Abby, S. S., Cury, J., Guglielmini, J., Néron, B., Touchon, M., Rocha, E. P. (2016). Identification of protein secretion systems in bacterial genomes. Sci. Rep. 6, 23080. doi: 10.1038/srep23080
Abby, S. S., Néron, B., Ménager, H., Touchon, M., Rocha, E. P. (2014). MacSyFinder: a program to mine genomes for molecular systems with an application to CRISPR-Cas systems. PloS One 9, e110726. doi: 10.1371/journal.pone.0110726
Angus, A. A., Agapakis, C. M., Fong, S., Yerrapragada, S., Estrada-de Los Santos, P., Yang, P., et al. (2014). Plant-associated symbiotic Burkholderia species lack hallmark strategies required in mammalian pathogenesis. PloS One 9, e83779. doi: 10.1371/journal.pone.0083779
Azegami, K., Nishiyama, K., Kato, H. (1988). Effect of iron limitation on “Pseudomonas plantarii” growth and tropolone and protein production. Appl. Environ. Microbiol. 54, 844–847. doi: 10.1128/aem.54.3.844-847.1988
Azegami, K., Nishiyama, K., Watanabe, Y., Kadota, I., Ohuchi, A., Fukazawa, C. (1987). Pseudomonas plantarii sp. nov., the causal agent of rice seedling blight. Int. J. Syst. Evol. Microbiol. 37, 144–152. doi: 10.1099/00207713-37-2-144
Bentley, S. D., Parkhill, J. (2004). Comparative genomic structure of prokaryotes. Annu. Rev. Genet. 38, 771–791. doi: 10.1146/annurev.genet.38.072902.094318
Bertelli, C., Gray, K. L., Woods, N., Lim, A. C., Tilley, K. E., Winsor, G. L., et al. (2022). Enabling genomic island prediction and comparison in multiple genomes to investigate bacterial evolution and outbreaks. Microb. Genom. 8, 000818. doi: 10.1099/mgen.0.000818
Bertelli, C., Laird, M. R., Williams, K. P., Simon Fraser University Research Computing Group, Lau, B. Y., Hoad, G., et al. (2017). IslandViewer 4: expanded prediction of genomic islands for larger-scale datasets. Nucleic Acids Res. 45, W30–W35. doi: 10.1093/nar/gkx343
Besemer, J., Lomsadze, A., Borodovsky, M. (2001). GeneMarkS: A self-training method for the prediction of gene starts in microbial genomes. Implications for finding sequence motifs in regulatory regions. Nucleic Acids Res. 29, 2607–2618. doi: 10.1093/nar/29.12.2607
Biswas, A., Staals, R. H., Morales, S. E., Fineran, P. C., Brown, C. M. (2016). CRISPRDetect: a flexible algorithm to define CRISPR arrays. BMC Genom. 17, 1–14. doi: 10.1186/s12864-016-2627-0
Blin, K., Shaw, S., Kloosterman, A. M., Charlop-Powers, Z., Van Wezel, G. P., Medema, M. H., et al. (2021). antiSMASH 6.0: improving cluster detection and comparison capabilities. Nucleic Acids Res. 49, W29–W35. doi: 10.1093/nar/gkab335
Brettin, T., Davis, J. J., Disz, T., Edwards, R. A., Gerdes, S., Olsen, G. J., et al. (2015). RASTtk: a modular and extensible implementation of the RAST algorithm for building custom annotation pipelines and annotating batches of genomes. Sci. Rep. 5, 8365. doi: 10.1038/srep08365
Carr, G., Seyedsayamdost, M. R., Chandler, J. R., Greenberg, E. P., Clardy, J. (2011). Sources of diversity in bactobolin biosynthesis by Burkholderia Thailandensis E264. 13, 3048–3051. doi: 10.1021/ol200922s
Chang, Z., Flatt, P., Gerwick, W. H., Nguyen, V. A., Willis, C. L., Sherman, D. H. (2002). The barbamide biosynthetic gene cluster: a novel marine cyanobacterial system of mixed polyketide synthase (PKS)-non-ribosomal peptide synthetase (NRPS) origin involving an unusual trichloroleucyl starter unit. Gene 296, 235–247. doi: 10.1016/S0378-1119(02)00860-0
Chin, C. S., Alexander, D. H., Marks, P., Klammer, A. A., Drake, J., Heiner, C., et al. (2013). Nonhybrid, finished microbial genome assemblies from long-read SMRT sequencing data. Nat. Methods 10, 563–569. doi: 10.1038/nmeth.2474
Coburn, B., Sekirov, I., Finlay, B. B. (2007). Type III secretion systems and disease. Clin. Microbiol. Rev. 20, 535–549. doi: 10.1128/CMR.00013-07
Compant, S., Nowak, J., Coenye, T., Clément, C., Ait Barka, E. (2008). Diversity and occurrence of Burkholderia spp. in the natural environment. FEMS Microbiol. Rev. 32, 607–626. doi: 10.1111/j.1574-6976.2008.00113.x
Couvin, D., Bernheim, A., Toffano-Nioche, C., Touchon, M., Michalik, J., Néron, B., et al. (2018). CRISPRCasFinder, an update of CRISRFinder, includes a portable version, enhanced performance and integrates search for Cas proteins. Nucleic Acids Res. 46, W246–W251. doi: 10.1093/nar/gky425
Crooks, G. E., Hon, G., Chandonia, J. M., Brenner, S. E. (2004). WebLogo: a sequence logo generator. Genome Res. 14, 1188–1190. doi: 10.1101/gr.849004
Dean, P. (2011). Functional domains and motifs of bacterial type III effector proteins and their roles in infection. FEMS Microbiol. Rev. 35, 1100–1125. doi: 10.1111/j.1574-6976.2011.00271.x
Dion, M. B., Labrie, S. J., Shah, S. A., Moineau, S. (2018). CRISPRStudio: a user-friendly software for rapid CRISPR array visualization. Viruses 10, 602. doi: 10.3390/v10110602
Duncan, K., Lewendon, A., Coggins, J. R. (1984). The purification of 5-enolpyruvylshikimate 3-phosphate synthase from an overproducing strain of Escherichia coli. FEBS Lett. 165, 121–127. doi: 10.1016/0014-5793(84)80027-7
Eichinger, V., Nussbaumer, T., Platzer, A., Jehl, M. A., Arnold, R., Rattei, T. (2016). EffectiveDB—updates and novel features for a better annotation of bacterial secreted proteins and Type III, IV, VI secretion systems. Nucleic Acids Res. 44, D669–D674. doi: 10.1093/nar/gkv1269
Figaj, D., Ambroziak, P., Przepiora, T., Skorko-Glonek, J. (2019). The role of proteases in the virulence of plant pathogenic bacteria. Int. J. Mol. Sci. 20, 672. doi: 10.3390/ijms20030672
Fu, L., Niu, B., Zhu, Z., Wu, S., Li, W. (2012). CD-HIT: accelerated for clustering the next-generation sequencing data. Bioinformatics 28, 3150–3152. doi: 10.1093/bioinformatics/bts565
Funke, T., Han, H., Healy-Fried, M. L., Fischer, M., Schönbrunn, E. (2006). Molecular basis for the herbicide resistance of Roundup Ready crops. Proc. Natl. Acad. Sci. 103, 13010–13015. doi: 10.1073/pnas.0603638103
Fuqua, W. C., Winans, S. C., Greenberg, E. P. (1994). Quorum sensing in bacteria: the LuxR-LuxI family of cell density-responsive transcriptional regulators. J. Bacteriol. 176, 269–275. doi: 10.1128/jb.176.2.269-275.1994
Galardini, M. (2017). roary_plots.py, an ipython script to visualize pangenome results. Pathogen Inf. WSI.
Gao, R., Krysciak, D., Petersen, K., Utpatel, C., Knapp, A., Schmeisser, C., et al. (2015). Genome-wide RNA sequencing analysis of quorum sensing-controlled regulons in the plant-associated Burkholderia glumae PG1 strain. Appl. Environ. Microbiol. 81, 7993–8007. doi: 10.1128/AEM.01043-15
Goodwin, S., McPherson, J. D., McCombie, W. R. (2016). Coming of age: ten years of next-generation sequencing technologies. Nat. Rev. Genet. 17, 333–351. doi: 10.1038/nrg.2016.49
Green, E. R., Mecsas, J. (2016). Bacterial secretion systems: an overview. Microbiol. Spect. 4, VMBF-0012–2015. doi: 10.1128/9781555819286.ch8
Gu, Q., Yang, Y., Yuan, Q., Shi, G., Wu, L., Lou, Z., et al. (2017). Bacillomycin D produced by Bacillus amyloliquefaciens is involved in the antagonistic interaction with the plant-pathogenic fungus. Fusarium graminearum. Appl. Environ. Microbiol. 83, e01075–e01017. doi: 10.1128/AEM.01075-17
Hacker, J., Carniel, E. (2001). Ecological fitness, genomic islands and bacterial pathogenicity. EMBO Rep. 2, 376–381. doi: 10.1093/embo-reports/kve097
Hatoum-Aslan, A., Marraffini, L. A. (2014). Impact of CRISPR immunity on the emergence and virulence of bacterial pathogens. Curr. Opin. Microbiol. 17, 82–90. doi: 10.1016/j.mib.2013.12.001
Hermenau, R., Mehl, J. L., Ishida, K., Dose, B., Pidot, S. J., Stinear, T. P., et al. (2019). Genomics-driven discovery of NO-donating diazeniumdiolate siderophores in diverse plant-associated bacteria. Angew. Chem. 131, 13158–13163. doi: 10.1002/ange.201906326
Hood, R. D., Singh, P., Hsu, F., Güvener, T., Carl, M. A., Trinidad, R. R., et al. (2010). A type VI secretion system of Pseudomonas aeruginosa targets a toxin to bacteria. Cell Host Microbe 7, 25–37. doi: 10.1016/j.chom.2009.12.007
Iiyama, K., Furuya, N., Ura, H., Matsuyama, N. (1998). Role of phytotoxins in the pathogenesis of Burkholderia species. J. Fac. Agr. Kyushu Univ. 42, 289–293. doi: 10.5109/24215
Jansen, R., Embden, J. D. V., Gaastra, W., Schouls, L. M. (2002). Identification of genes that are associated with DNA repeats in prokaryotes. Mol. Microbiol. 43, 1565–1575. doi: 10.1046/j.1365-2958.2002.02839.x
Jenul, C., Sieber, S., Daeppen, C., Mathew, A., Lardi, M., Pessi, G., et al. (2018). Biosynthesis of fragin is controlled by a novel quorum sensing signal. Nat. Commun. 9, 1297. doi: 10.1038/s41467-018-03690-2
Johnson, L. J., Koulman, A., Christensen, M., Lane, G. A., Fraser, K., Forester, N., et al. (2013). An extracellular siderophore is required to maintain the mutualistic interaction of Epichloë festucae with Lolium perenne. PloS Pathog. 9, e1003332. doi: 10.1371/journal.ppat.1003332
Jones, A. M., Lindow, S. E., Wildermuth, M. C. (2007). Salicylic acid, yersiniabactin, and pyoverdin production by the model phytopathogen Pseudomonas syringae pv. tomato DC3000: synthesis, regulation, and impact on tomato and Arabidopsis host plants. J. Bacteriol. 189, 6773–6786. doi: 10.1128/JB.00827-07
Juhas, M., van der Meer, J. R., Gaillard, M., Harding, R. M., Hood, D. W., Crook, D. W. (2009). Genomic islands: tools of bacterial horizontal gene transfer and evolution. FEMS Microbiol. Rev. 33, 376–393. doi: 10.1111/j.1574-6976.2008.00136.x
Karki, H. S., Ham, J. H. (2014). The roles of the shikimate pathway genes, aroA and aroB, in virulence, growth and UV tolerance of Burkholderia glumae strain 411gr-6. Mol. Plant Pathol. 15, 940–947. doi: 10.1111/mpp.12147
Kim, J., Kim, J. G., Kang, Y., Jang, J. Y., Jog, G. J., Lim, J. Y., et al. (2004). Quorum sensing and the LysR-type transcriptional activator ToxR regulate toxoflavin biosynthesis and transport in Burkholderia glumae. Mol. Microbiol. 54, 921–934. doi: 10.1111/j.1365-2958.2004.04338.x
Kim, N., Kim, J. J., Kim, I., Mannaa, M., Park, J., Kim, J., et al. (2020). Type VI secretion systems of plant-pathogenic Burkholderia glumae BGR1 play a functionally distinct role in interspecies interactions and virulence. Mol. Plant Pathol. 21, 1055 1069.
Koumoutsi, A., Chen, X. H., Henne, A., Liesegang, H., Hitzeroth, G., Franke, P., et al. (2004). Structural and functional characterization of gene clusters directing nonribosomal synthesis of bioactive cyclic lipopeptides in Bacillus amyloliquefaciens strain FZB42. J. Bacteriol. 186, 1084–1096. doi: 10.1128/JB.186.4.1084-1096.2004
Kovach, M. E., Elzer, P. H., Hill, D. S., Robertson, G. T., Farris, M. A., Roop, R. M., II, et al. (1995). Four new derivatives of the broad-host-range cloning vector pBBR1MCS, carrying different antibiotic-resistance cassettes. Gene 166, 175–176. doi: 10.1016/0378-1119(95)00584-1
Lamont, I. L., Beare, P. A., Ochsner, U., Vasil, A. I., Vasil, M. L. (2002). Siderophore-mediated signaling regulates virulence factor production in Pseudomonas aeruginosa. Proc. Natl. Acad. Sci. 99, 7072–7077. doi: 10.1073/pnas.092016999
Land, M., Hauser, L., Jun, S. R., Nookaew, I., Leuze, M. R., Ahn, T. H., et al. (2015). Insights from 20 years of bacterial genome sequencing. Funct. Integr. Genom. 15, 141–161. doi: 10.1007/s10142-015-0433-4
Langille, M. G. I., Brinkman, F. S. L. (2010). IslandViewer: an integrated interface for computational identification and visualization of genomic islands. Bioinformatics 26, 1766–1767. doi: 10.1093/bioinformatics/btp030
Lee, C., Mannaa, M., Kim, N., Kim, J., Choi, Y., Kim, S. H., et al. (2019). Stress tolerance and virulence-related roles of lipopolysaccharide in Burkholderia glumae. Plant Pathol. J. 35, 445. doi: 10.5423/PPJ.OA.04.2019.0124
Lefort, V., Desper, R., Gascuel, O. (2015). FastME 2.0: a comprehensive, accurate, and fast distance-based phylogeny inference program. Mol. Biol. Evol. 32, 2798–2800. doi: 10.1093/molbev/msv150
Letunic, I., Bork, P. (2021). Interactive Tree Of Life (iTOL) v5: an online tool for phylogenetic tree display and annotation. Nucleic Acids Res. 49, W293–W296. doi: 10.1093/nar/gkab301
Liu, X., Fan, X., Matsumoto, H., Nie, Y., Sha, Z., Yi, K., et al. (2018). Biotoxin tropolone contamination associated with nationwide occurrence of pathogen Burkholderia plantarii in agricultural environments in China. Environ. Sci. Technol. 52, 5105–5114. doi: 10.1021/acs.est.7b05915
Lo, R. Y., McKerral, L. J., Hills, T. L., Kostrzynska, M. (2001). Analysis of the capsule biosynthetic locus of Mannheimia (Pasteurella) haemolytica A1 and proposal of a nomenclature system. Infect. Immun. 69, 4458–4464. doi: 10.1128/IAI.69.7.4458-4464.2001
Louwen, R., Staals, R. H., Endtz, H. P., van Baarlen, P., van der Oost, J. (2014). The role of CRISPR-Cas systems in virulence of pathogenic bacteria. Microbiol. Mol. Biol. Rev. 78, 74–88. doi: 10.1128/MMBR.00039-13
Mannaa, M., Han, G., Jeong, T., Kang, M., Lee, D., Jung, H., et al. (2023). Taxonomy-guided selection of Paraburkholderia busanensis sp. nov.: a versatile biocontrol agent with mycophagy against Colletotrichum scovillei causing pepper anthracnose. Microbiol. Spectr. 11, e02426–e02423. doi: 10.1128/spectrum.02426-23
Mannaa, M., Park, I., Seo, Y. S. (2018). Genomic features and insights into the taxonomy, virulence, and benevolence of plant-associated Burkholderia species. Int. J. Mol. Sci. 20, 121. doi: 10.3390/ijms20010121
McGowan, S. J., Sebaihia, M., O'leary, S., Hardie, K. R., Williams, P., Stewart, G. S. A. B., et al. (1997). Analysis of the carbapenem gene cluster of Erwinia carotovora: definition of the antibiotic biosynthetic genes and evidence for a novel β-lactam resistance mechanism. Mol. Microbiol. 26, 545–556. doi: 10.1046/j.1365-2958.1997.6001974.x
Meier-Kolthoff, J. P., Auch, A. F., Klenk, H. P., Göker, M. (2013). Genome sequence-based species delimitation with confidence intervals and improved distance functions. BMC Bioinformatic. 14, 1–14. doi: 10.1186/1471-2105-14-60
Meier-Kolthoff, J. P., Göker, M. (2019). TYGS is an automated high-throughput platform for state-of-the-art genome-based taxonomy. Nat. Commun. 10, 2182. doi: 10.1038/s41467-019-10210-3
Moyne, A. L., Shelby, R., Cleveland, T. E., Tuzun, S. (2001). Bacillomycin D: an iturin with antifungal activity against Aspergillus flavus. J. Appl. Microbiol. 90, 622–629. doi: 10.1046/j.1365-2672.2001.01290.x
Notredame, C., Higgins, D. G., Heringa, J. (2000). T-Coffee: A novel method for fast and accurate multiple sequence alignment. J. Mol. Biol. 302, 205–217. doi: 10.1006/jmbi.2000.4042
O’Brien, J. A., Daudi, A., Butt, V. S., Bolwell, G. P. (2012). Reactive oxygen species and their role in plant defence and cell wall metabolism. Planta 236, 765–779. doi: 10.1007/s00425-012-1696-9
Olfa, T., Antonio, D. G., Sana, A., Imen, B. S., Salem, E., Mohamed Najib, A., et al. (2015). Synergistic fungicidal activity of the lipopeptide bacillomycin D with amphotericin B against pathogenic Candida species. FEMS Yeast Res. 15, fov022. doi: 10.1093/femsyr/fov022
O’Sullivan, L. A., Mahenthiralingam, E. (2005). Biotechnological potential within the genus Burkholderia. Lett. Appl. Microbiol. 41, 8–11. doi: 10.1111/j.1472-765X.2005.01758.x
Page, A. J., Cummins, C. A., Hunt, M., Wong, V. K., Reuter, S., Holden, M. T., et al. (2015). Roary: rapid large-scale prokaryote pan genome analysis. Bioinformatics 31, 3691–3693. doi: 10.1093/bioinformatics/btv421
Palmer, L. D., Skaar, E. P. (2016). Transition metals and virulence in bacteria. Annu. Rev. Genet. 50, 67–91. doi: 10.1146/annurev-genet-120215-035146
Puhar, A., Sansonetti, P. J. (2014). Type III secretion system. Curr. Biol. 24, R784–R791. doi: 10.1016/j.cub.2014.07.016
Ra, J. E., Kang, M. H., Seo, S. J., Lee, B. C., Choi, N. J., Chung, I. M., et al. (2016). First report of bacterial grain rot caused by Burkholderia plantarii in Republic of Korea. J. Plant Pathol. 98, 3.
Raaijmakers, J. M., Mazzola, M. (2012). Diversity and natural functions of antibiotics produced by beneficial and plant pathogenic bacteria. Ann. Rev. Phytopathol. 50, 403–424. doi: 10.1146/annurev-phyto-081211-172908
Radics, J., Königsmaier, L., Marlovits, T. C. (2014). Structure of a pathogenic type 3 secretion system in action. Nat. Struct. Mol. Biol. 21, 82–87. doi: 10.1038/nsmb.2722
Ravel, J., Cornelis, P. (2003). Genomics of pyoverdine-mediated iron uptake in pseudomonads. Trends Microbiol. 11, 195–200. doi: 10.1016/S0966-842X(03)00076-3
Reinhold-Hurek, B., Hurek, T. (2011). Living inside plants: bacterial endophytes. Curr. Opin. Plant Biol. 14, 435–443. doi: 10.1016/j.pbi.2011.04.004
Robert, X., Gouet, P. (2014). Deciphering key features in protein structures with the new ENDscript server. Nucleic Acids Res. 42, W320–W324. doi: 10.1093/nar/gku316
Russell, A. B., Hood, R. D., Bui, N. K., LeRoux, M., Vollmer, W., Mougous, J. D. (2011). Type VI secretion delivers bacteriolytic effectors to target cells. Nature 475, 343–347. doi: 10.1038/nature10244
Sambrook, J., Fritsch, E. F., Maniatis, T. (1989). Molecular cloning: a laboratory manual. 2nd ed (Cold Spring Harbor, NY, USA: Cold Spring Harbor Laboratory Press).
Schäfer, A., Tauch, A., Jäger, W., Kalinowski, J., Thierbach, G., Pühler, A. (1994). Small mobilizable multi-purpose cloning vectors derived from the Escherichia coli plasmids pK18 and pK19: selection of defined deletions in the chromosome of Corynebacterium glutamicum. Gene 145, 69–73. doi: 10.1016/0378-1119(94)90324-7
Schwartz, D., Berger, S., Heinzelmann, E., Muschko, K., Welzel, K., Wohlleben, W. (2004). Biosynthetic gene cluster of the herbicide phosphinothricin tripeptide from Streptomyces viridochromogenes Tu494. Appl. Environ. Microbiol. 70, 7093–7102. doi: 10.1128/AEM.70.12.7093-7102.2004
Seemann, T. (2014). Prokka: rapid prokaryotic genome annotation. Bioinformatics 30, 2068–2069. doi: 10.1093/bioinformatics/btu153
Seo, Y. S., Lim, J. Y., Park, J., Kim, S., Lee, H. H., Cheong, H., et al. (2015). Comparative genome analysis of rice-pathogenic Burkholderia provides insight into capacity to adapt to different environments and hosts. BMC Genom. 16, 1–11. doi: 10.1186/s12864-015-1558-5
Sharma, S., Sharma, S., Hirabuchi, A., Yoshida, K., Fujisaki, K., Ito, A., et al. (2013). Deployment of the Burkholderia glumae type III secretion system as an efficient tool for translocating pathogen effectors to monocot cells. Plant J. 74, 701–712. doi: 10.1111/tpj.12148
Shende, V. V., Bauman, K. D., Moore, B. S. (2024). The shikimate pathway: gateway to metabolic diversity. Nat. Prod. Rep. 38, 130–239. doi: 10.1039/D3NP00037K
Simon, R., Priefer, U., Pühler, A. (1983). A broad host range mobilization system for in vivo genetic engineering: transposon mutagenesis in gram-negative bacteria. Nat. Biotechnol. 1, 784–791. doi: 10.1038/nbt1183-784
Solis, R., Bertani, I., Degrassi, G., Devescovi, G., Venturi, V. (2006). Involvement of quorum sensing and RpoS in rice seedling blight caused by Burkholderia plantarii. FEMS Microbiol. Lett. 259, 106–112. doi: 10.1111/fml.2006.259.issue-1
Stamatakis, A. (2014). RAxML version 8: a tool for phylogenetic analysis and post-analysis of large phylogenies. Bioinformatics 30, 1312–1313. doi: 10.1093/bioinformatics/btu033
Stothard, P., Grant, J. R., Van Domselaar, G. (2019). Visualizing and comparing circular genomes using the CGView family of tools. Brief. Bioinform. 20, 1576–1582. doi: 10.1093/bib/bbx081
Sullivan, M. J., Petty, N. K., Beatson, S. A. (2011). Easyfig: a genome comparison visualizer. Bioinformatics 27, 1009–1010. doi: 10.1093/bioinformatics/btr039
Suwa, M., Sugino, H., Sasaoka, A., Mori, E., Fujii, S., Shinkawa, H., et al. (2000). Identification of two polyketide synthase gene clusters on the linear plasmid pSLA2-L in Streptomyces rochei. Gene 246, 123–131. doi: 10.1016/S0378-1119(00)00060-3
Swain, D. M., Yadav, S. K., Tyagi, I., Kumar, R., Kumar, R., Ghosh, S., et al. (2017). A prophage tail-like protein is deployed by Burkholderia bacteria to feed on fungi. Nat. Commun. 8, 404. doi: 10.1038/s41467-017-00529-0
Trust, T. J. (1975). Antibacterial activity of tropolone. Antimicrob. Agents Chemother. 7, 500–506. doi: 10.1128/AAC.7.5.500
Wallner, A., Moulin, L., Busset, N., Rimbault, I., Béna, G. (2021). Genetic diversity of type 3 secretion system in Burkholderia sl and links with plant host adaptation. Front. Microbiol. 12, 761215. doi: 10.3389/fmicb.2021.761215
Wang, M., Hashimoto, M., Hashidoko, Y. (2013). Repression of tropolone production and induction of a Burkholderia plantarii pseudo-biofilm by carot-4-en-9, 10-diol, a cell-to-cell signaling disrupter produced by Trichoderma virens. PloS One 8, e78024. doi: 10.1371/journal.pone.0078024
Wang, M., Wei, P., Cao, M., Zhu, L., Lu, Y. (2016). First report of rice seedling blight caused by Burkholderia plantarii in North and Southeast China. Plant Dis. 100, 645. doi: 10.1094/PDIS-07-15-0765-PDN
Wang, X., Zhou, H., Chen, H., Jing, X., Zheng, W., Li, R., et al. (2018). Discovery of recombinases enables genome mining of cryptic biosynthetic gene clusters in Burkholderiales species. Proc. Natl. Acad. Sci. 115, E4255–E4263. doi: 10.1073/pnas.1720941115
Keywords: type III secretion system, secondary metabolites, genome analysis, rice pathogenic bacteria, virulence
Citation: Mannaa M, Lee D, Lee H-H, Han G, Kang M, Kim T-J, Park J and Seo Y-S (2024) Exploring the comparative genome of rice pathogen Burkholderia plantarii: unveiling virulence, fitness traits, and a potential type III secretion system effector. Front. Plant Sci. 15:1416253. doi: 10.3389/fpls.2024.1416253
Received: 12 April 2024; Accepted: 10 May 2024;
Published: 23 May 2024.
Edited by:
Jesús Murillo, Public University of Navarre, SpainReviewed by:
Clemencia Rojas, University of Arkansas, United StatesCopyright © 2024 Mannaa, Lee, Lee, Han, Kang, Kim, Park and Seo. This is an open-access article distributed under the terms of the Creative Commons Attribution License (CC BY). The use, distribution or reproduction in other forums is permitted, provided the original author(s) and the copyright owner(s) are credited and that the original publication in this journal is cited, in accordance with accepted academic practice. No use, distribution or reproduction is permitted which does not comply with these terms.
*Correspondence: Young-Su Seo, eXNlbzIwMTFAcHVzYW4uYWMua3I=
†These authors have contributed equally to this work
Disclaimer: All claims expressed in this article are solely those of the authors and do not necessarily represent those of their affiliated organizations, or those of the publisher, the editors and the reviewers. Any product that may be evaluated in this article or claim that may be made by its manufacturer is not guaranteed or endorsed by the publisher.
Research integrity at Frontiers
Learn more about the work of our research integrity team to safeguard the quality of each article we publish.