- 1Crop Breeding Division, National Institute of Crop Science, Rural Development Administration, Wanju, Republic of Korea
- 2Department of Crop Science and Biotechnology, Jeonbuk National University, Jeonju, Republic of Korea
- 3Department of Southern Area Crop Science, National Institute of Crop Science, Rural Development Administration, Miryang, Republic of Korea
- 4Institute of Agricultural Science and Technology, Jeonbuk National University, Jeonju, Republic of Korea
Rice blast is a destructive fungal disease affecting rice plants at various growth stages, significantly threatening global yield stability. Development of resistant rice cultivars stands as a practical means of disease control. Generally, association mapping with a diversity panel powerfully identifies new alleles controlling trait of interest. On the other hand, utilization of a breeding panel has its advantage that can be directly applied in a breeding program. In this study, we conducted a genome-wide association study (GWAS) for blast resistance using 296 commercial rice cultivars with low population structure but large phenotypic diversity. We attempt to answer the genetic basis behind rice blast resistance among early maturing cultivars by subdividing the population based on its Heading date 1 (Hd1) functionality. Subpopulation-specific GWAS using the mixed linear model (MLM) based on blast nursery screening conducted in three years revealed a total of 26 significant signals, including three nucleotide-binding site leucine-rich repeat (NBS-LRR) genes (Os06g0286500, Os06g0286700, and Os06g0287500) located at Piz locus on chromosome 6, and one at the Pi-ta locus (Os12g0281300) on chromosome 12. Haplotype analysis revealed blast resistance associated with Piz locus was exclusively specific to Type 14 hd1 among japonica rice. Our findings provide valuable insights for breeding blast resistant rice and highlight the applicability of our elite cultivar panel to detect superior alleles associated with important agronomic traits.
1 Introduction
Rice blast, caused by the fungal pathogen Magnaporthe oryzae, annually results in a global reduction of 10−30% in crop yields, leading to significant economic losses in the agricultural sector (Skamnioti and Gurr, 2009). The most economical method of controlling blast disease is through the breeding of resistant varieties. However, many blast resistance (R) genes are specific to certain pathogen strains, making resistant varieties susceptible to rapid diversification of blast strains (Hittalmani et al., 2000; Oliveira-Garcia and Valent, 2015). Therefore, numerous breeding programs are actively searching for diverse genetic resources to enhance resistance and are simultaneously involved in developing new rice varieties by introducing novel R genes.
To date, over 100 blast R genes have been identified, with 25 of these genes characterized at the molecular level (Kalia and Rathour, 2019). Identification of genes underlying quantitative trait loci (QTL) associated with blast resistance is usually conducted using a classic biparental map-based cloning strategy. This strategy is laborious and time consuming, and has limitations on fully elucidating the genomic potential, often resulting in only two alleles representing the entire population (Zhao et al., 2011). Genome-wide association study (GWAS) has been used to detect significant genetic variants associated with trait of interest (Varshney et al., 2009; Huang et al., 2012; McCouch et al., 2016). Compared to biparental mapping approach, the genetic diversity of the population in GWAS is considerably broader, enabling the effective mapping of alleles associated with rice blast resistance. For example, a GWAS analysis conducted with 366 diverse indica accessions examined under 16 rice blast strains identified 30 loci associated with blast resistance (Wang et al., 2014). Similarly, a GWAS with rice diversity panel 1 (RDP1) comprising diverse rice cultivars collected from 82 countries, has identified a total of 97 loci, of which 15 are co-localized with previously reported loci associated with blast resistance (Kang et al., 2016). Using the same panel, a GWAS was conducted to identify loci associated with field blast resistance in three major rice production areas of China (Zhu et al., 2016), and evaluation on blast resistance on diverse Chinese isolates led to the identification of a new Pik allele (Li et al., 2019). The GWAS with a diversity panel has indeed demonstrated its advantage in detecting several natural allelic variations controlling blast resistance. However, the validation of discovered QTL in the genetic background of local breeding population is necessary for their utilization in cultivar development (Begum et al., 2015; Verdeprado et al., 2018).
Here, we conducted GWAS on blast resistance in 296 Korean commercial rice cultivars with low population structure, yet exhibiting significant phenotypic variations across various agronomic traits (Lee et al., 2023). Our previous comparative observations based on maturity group have demonstrated that early maturing cultivars exhibit significant blast resistance compared to medium and medium-late maturing cultivars in Korea. Furthermore, association analysis among molecular markers linked to rice blast resistance has revealed that multiple R gene families at the Piz locus on chromosome 6 are strongly associated with blast resistance phenotype among Korean rice cultivars (Baek et al., 2021; Lee et al., 2022). Therefore, we implemented subpopulation-specific GWAS on blast resistance to discern the significant loci that contribute to the blast resistance of cultivars belonging to different maturity groups. This was implemented based on the functionality of Heading date 1 (Hd1), which serves as a key determinant of heading date diversity among Korean rice cultivars (Mo et al., 2021). GWAS and haplotype analyses revealed significant blast resistance loci associated with specific Hd1 allele types. Additionally, we discussed the geographic distribution of potential R gene donors and their direct application in breeding blast resistant cultivars in Korea.
2 Materials and methods
2.1 Plant materials and phenotypic evaluation
A total of 296 Korean commercial rice cultivars, comprising 264 japonica and 32 Tongil-type, were used in this study (Supplementary Table 1). To evaluate blast resistance, nursery tests were conducted at the experimental field station of National Institute of Crop Science (NICS) located in Wanju (35°84′ N, 127°05′ E) for three years (2018, 2019, and 2023). We established a 12-bed blast nursery, each bed measuring 45 m in length and 1.2 m in width. We directly sowed 2 g of seeds from each cultivar in 30 cm-long rows, with each adjacent row spaced 10 cm apart. The susceptible variety, Hopyung (spreader), was sown along the inner and outer bordering rows of each bed to facilitate an even spread of the blast disease. Blast infection occurred naturally, and fertilizers were applied at a ratio of N-P2O5-K2O = 21-17-17 kg/10a, with application of N at 80% as a basal dose, supplemented by 10% each at 12 and 20 days after sowing to induce an environment conducive to blast incidence. Additionally, we installed mesh shades over the nursery beds to reduce direct sunlight while maintaining adequate airflow, simulating natural field conditions favorable for blast infection. Disease scoring began 30 days after sowing when susceptible check (Hopyung) exhibited blast score of 7 based on standard evaluation system (IRRI, 2002). Within this system, scores of 1−3 indicate resistance, 4−6 as moderate resistance, and 7−9 as susceptibility. Monthly average air temperature, relative humidity, and rainfall data were obtained from the Korea Meteorological Administration (https://data.kma.go.kr) (Supplementary Table 2). Heading dates were measured in the paddy fields located at the same experimental station during the regular planting seasons of 2018 and 2023. The rice cultivars were sown on 09 May 2018 and 02 May 2023, and transplanted on 01 June 2018 and 26 May 2023, respectively. In each cultivar, 26 plants were transplanted into 4.5 m rows, with individual plants spaced 15 cm apart, and 30 cm spacing between adjacent rows. Days to heading (DTH) was determined by counting the number of days from sowing to heading when 40% of the plants within a plot exhibit emerging panicles.
2.2 DNA extraction and sequencing
Leaf tissues of 21-day-old seedlings from each rice cultivar were sampled, and genomic DNA was extracted using the modified cetyltrimethylammonium bromide (CTAB) method (Murray and Thompson, 1980). The quality and quantity of the extracted DNA were checked using ND-1000 spectrophotometer (Thermo Fisher Scientific, USA) and Quant-iT™ dsDNA assay kit (Thermo Fisher Scientific, USA). High-throughput SNP genotyping of 296 cultivars was performed using the KNU Affymetric Axiom Oryza 580K Genotyping array (Thermo Fisher Scientific) at DNA Link (Seoul, Korea).
2.3 Population structure and PCA
A set of 201,624 (minor allele frequency > 0.05, call rate = 95%) polymorphic SNPs were generated using TASSEL v5.2.80 (Bradbury et al., 2007). The phylogenic tree was constructed using TASSEL v5.2.80 (Bradbury et al., 2007) with default parameters, and was visualized by iTOL v6.8.1 (Letunic and Bork, 2021). The input file conversion from VCF to STRUCTURE was conducted using PGDSpider v2.1.1.5 (Lischer and Excoffier, 2012). The population structure of 296 cultivars was identified using STRUCTURE v2.3.4 (Pritchard et al., 2000). Run length was given as 10,000 burning period length followed by 10,000 Markov Chain Monte Carlo (MCMC) replications. Each ancestry kinship (K) value was run for 5 replications with K value varying from 1 to 10. The optimum K value was predicted by calculating ΔK according to the Evanno method (Evanno et al., 2005) using Structure Harvester (Earl and VonHoldt, 2012). Principal component analysis (PCA) was conducted using TASSEL v5.2.80 (Bradbury et al., 2007). The PCA plots of the first two components featured groups delineated based on the predicted K values.
2.4 GWAS analysis
High-quality SNPs were mapped on the Os-Nipponbare-Reference-IRGSP-1.0 pseudomolecule assembly (Kawahara et al., 2013) and annotated using SnpEff (Cingolani et al., 2012). We filtered 46,877 SNPs in the coding sequence (CDS) and used for GWAS. We implemented the mixed linear model (MLM) (Yu et al., 2006) and the fixed and random model circulating probability unification (FarmCPU) (Liu et al., 2016) within the ‘rMVP’ package (Yin et al., 2021) in R. Manhattan and quantile–quantile (Q–Q) plots were produced using the ‘CMplot’ package. NCBI gene bank database (https://www.ncbi.nlm.nih.gov/) and the Rice Annotation Project Database (RAP-DB) were used to validate the physical positions of the significant SNPs and retrieve corresponding gene annotation information (Sakai et al., 2013). Based on Bonferroni correction with effective number of independent SNPs at a 5% level of significance, we set the p threshold qualification as 0.05/number of independent SNP. The calculated threshold value was set as −log10 (P) = 5.9.
2.5 Nucleotide diversity, Tajima’s D, haplotype analysis, and geographical distribution
To measure the degree of polymorphism and selective pressure at SNP sites, we calculated nucleotide diversity (π) and Tajima’s D using VCFtools (Danecek et al., 2011). Nucleotide diversity and Tajima’s D indices were calculated for both candidate gene regions and whole genome, with sliding windows of 10 kb and 100 kb, respectively. Haplotype analysis was conducted using a dataset comprising 201,624 SNPs, wherein heterozygotes and missing alleles were excluded. SNPs located within the genomic regions of candidate genes annotated as NBS-LRR genes were extracted from the variant call format (VCF) using site filtering option of VCFtools (Danecek et al., 2011). Comparative phenotypic analysis of the haplotypes was performed using Scheffe’s post hoc test (P ≤ 0.05) using the ‘agricolae’ package (De Mendiburu and Simon, 2015). Hd1 allele types of 296 cultivars were retrieved from our previous study (Mo et al., 2021). Extended haplotype network analysis was conducted using RiceVarMap v2.0 (Zhao et al., 2015) by inputting significant SNP IDs and setting the option to classification 3.
2.6 Statistical analysis
All statistical analyses and data visualization were performed using R version 4.2.1 (R Core Team, 2013). The best linear unbiased prediction (BLUP) value for each accession was obtained by fitting multi-season phenotype data into a linear mixed model using the ‘lme4’ package (Bates et al., 2014). Simple t-tests were conducted using the ‘stats’ package.
3 Results
3.1 SNP distribution and population structure of 296 rice cultivars
In this study, we performed high-throughput SNP genotyping on 296 commercial rice cultivars developed between 1979 and 2017 using Axiom genotyping platform (Supplementary Table 1). After data processing and SNP filtering, a total of 201,624 SNPs were physically mapped to the reference genome (IRGSP-1.0) across 12 chromosomes, with an average variant rate of 1.9 kb per chromosome (Supplementary Figure 1; Supplementary Table 3). The highest numbers of SNPs were mapped to chromosome 1 (27,378 SNPs), while the lowest number was observed on chromosome 9 (11,646 SNPs). The genetic properties of markers are summarized in Supplementary Table 4. The ratio of transition to transversion (ti/tv) SNPs was calculated as 2.5, with G/A, C/T, T/C, and A/G transitions collectively accounting for 17.5–18.3%, thus contributing to 71.5% of the total point mutation types.
The phylogenic tree and population structure analysis, conducted to determine the optimal K, indicated that the 296 commercial rice cultivars clustered into two subpopulations (K = 2), primarily between japonica (264) and Tongil-type (32) (Figures 1A, B). When K = 3, the japonica population was further subdivided into two subpopulations, while Tongil-type remained unchanged (Figure 1C). Similarly, the principal component analysis (PCA) showed separation into two subpopulations, elucidating a total of 64.1% genetic variation across the first two principal components (Figure 1D).
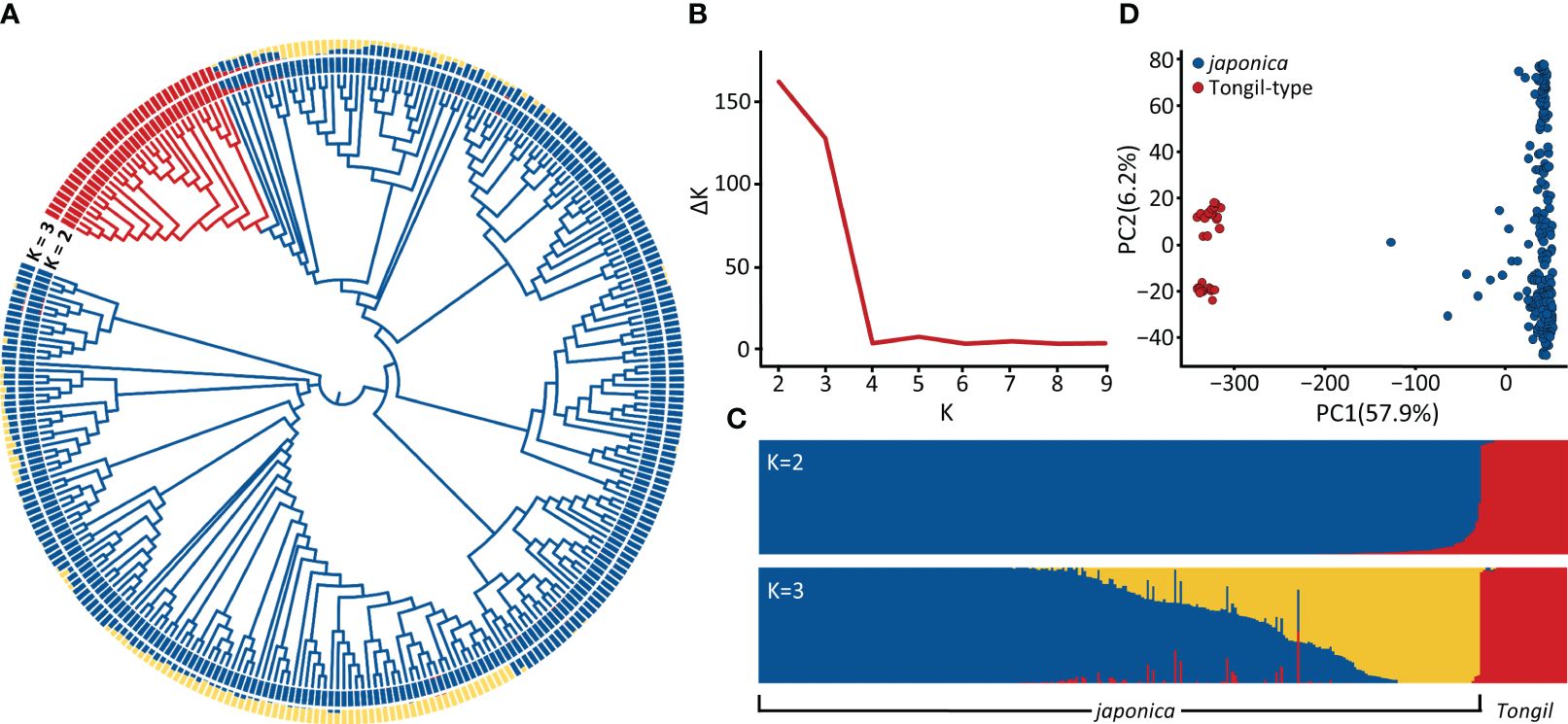
Figure 1 Population structure of 296 Korean rice cultivars. (A) Phylogenic tree constructed using high-quality 201,624 SNPs. (B) Plot of ΔK values ranging from 1 to 10, calculated according to the Evanno method (Evanno et al., 2005). (C) Genetic structure of 296 cultivars revealed by STRUCTURE with the ancestry of kinships set to 2 and 3; the proportion of genetic background of each ancestral population is indicated by vertical bar, representing japonica (blue), japonica when K=3 (yellow), and Tongil-type (red). (D) Principal component analysis (PCA) of the 296 cultivars.
3.2 Phenotypic variations of 296 cultivars
The three-year nursery evaluation of blast resistance for 296 cultivars is presented in Figure 2A and Supplementary Table 1. The mean blast scores among three years ranged from 3.4 to 4.9. Significant correlations were observed among yearly replications, with Pearson’s correlation coefficients ranging from 0.77 to 0.79 (Supplementary Table 5). To enhance the precision of phenotypic predictions, we applied BLUP estimation, minimizing the sum of squared residuals and estimating random effects to bring our phenotypic data closer to a normal distribution (Figure 2B). Consistent with our previous observations (Mo et al., 2021; Lee et al., 2022), early maturing cultivars carrying non-functional hd1 alleles exhibited significantly stronger blast resistance compared to mid-late maturing cultivars carrying functional Hd1 alleles (Figures 2C, D).
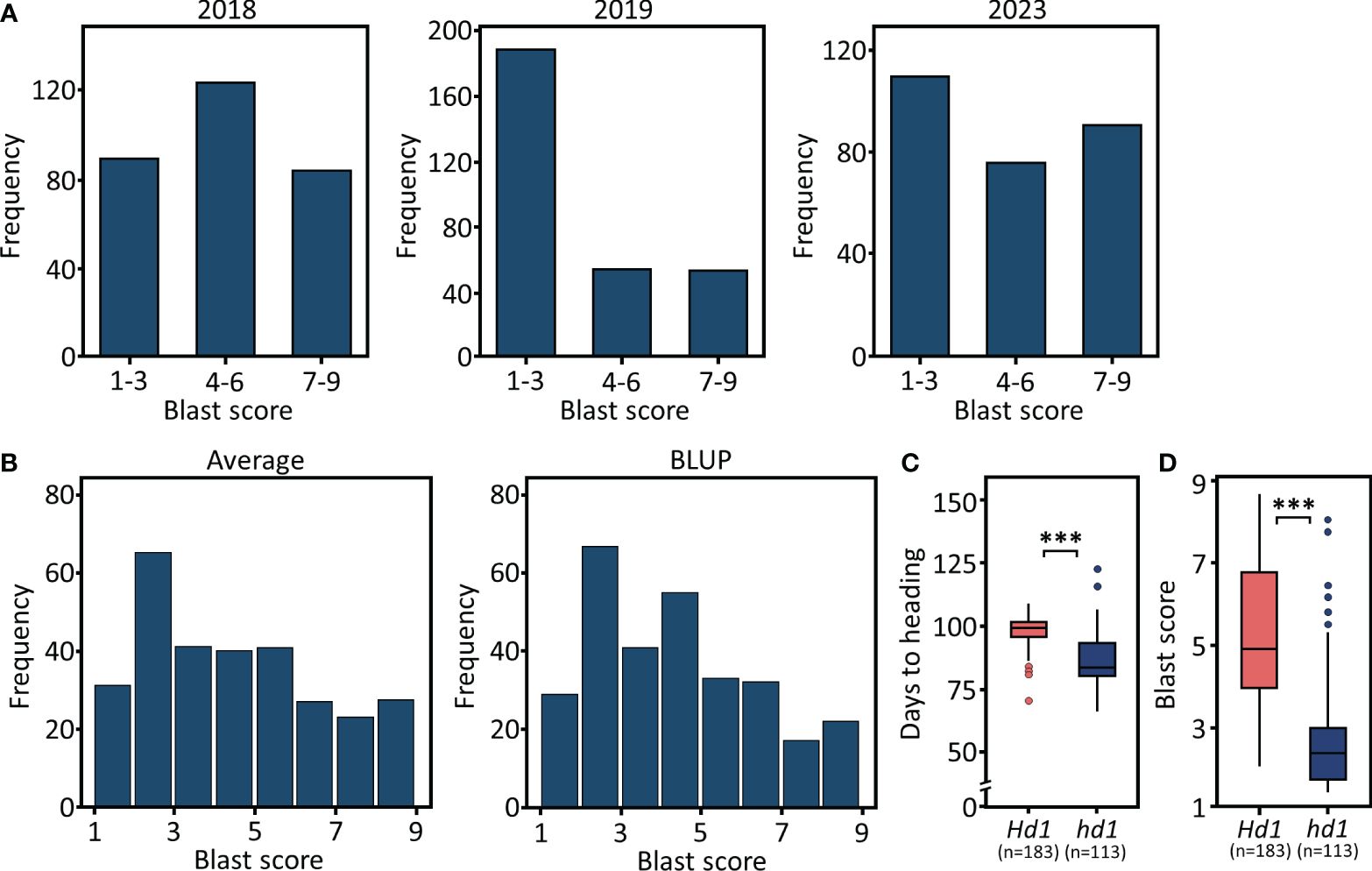
Figure 2 Phenotypic distribution of 296 Korean rice cultivars. (A) The frequency distribution of blast resistance scores across three-year trials (2019, 2020, and 2023). (B) Distribution of average and BLUP values for blast resistance scores. (C) Heading date variation based on functionality of Hd1. (D) Blast resistance score variation based on functionality of Hd1. ***P < 0.001.
3.3 Genome-wide association study
For the GWAS on blast resistance, we employed 46,877 high-quality SNPs located in the coding sequences (CDS) and utilized a mixed linear model (MLM) and fixed and random model circulating probability unification (FarmCPU). To increase accuracy in predictions, we utilized BLUP values as phenotypic data for blast resistance. Based on the genome-wide significance threshold at −log10 (P) = 5.9, we identified a total of 26 and 14 signals for blast resistance across the whole (n=296), Hd1 (n=183), and hd1 (n=113) populations using MLM and FarmCPU, respectively (Figure 3; Supplementary Figure 2). Specifically, 12, 2, and 12 signals were detected for the whole, Hd1, and hd1 populations using MLM, respectively (Table 1). Notably, the significant signals detected on chromosome 6 and 12 are localized within the previously reported Piz and Pi-ta loci, corresponding to nucleotide-binding site−leucine-rich repeat (NBS-LRR) proteins. The lead SNPs identified within the NBS-LRR genes were observed in both the whole and hd1 populations, specifically located at Chr06:10379493, Chr06:10389270, and Chr06:10436055 in the Piz locus (Figure 3A). Additionally, the SNP located at Chr12:10607554 within the Pi-ta locus was detected only in the Hd1 population (Figure 3B). These results suggest that blast resistance among early maturing cultivars is potentially associated with the Piz locus, while that among mid-late maturing cultivars appear to be linked with the Pi-ta locus.
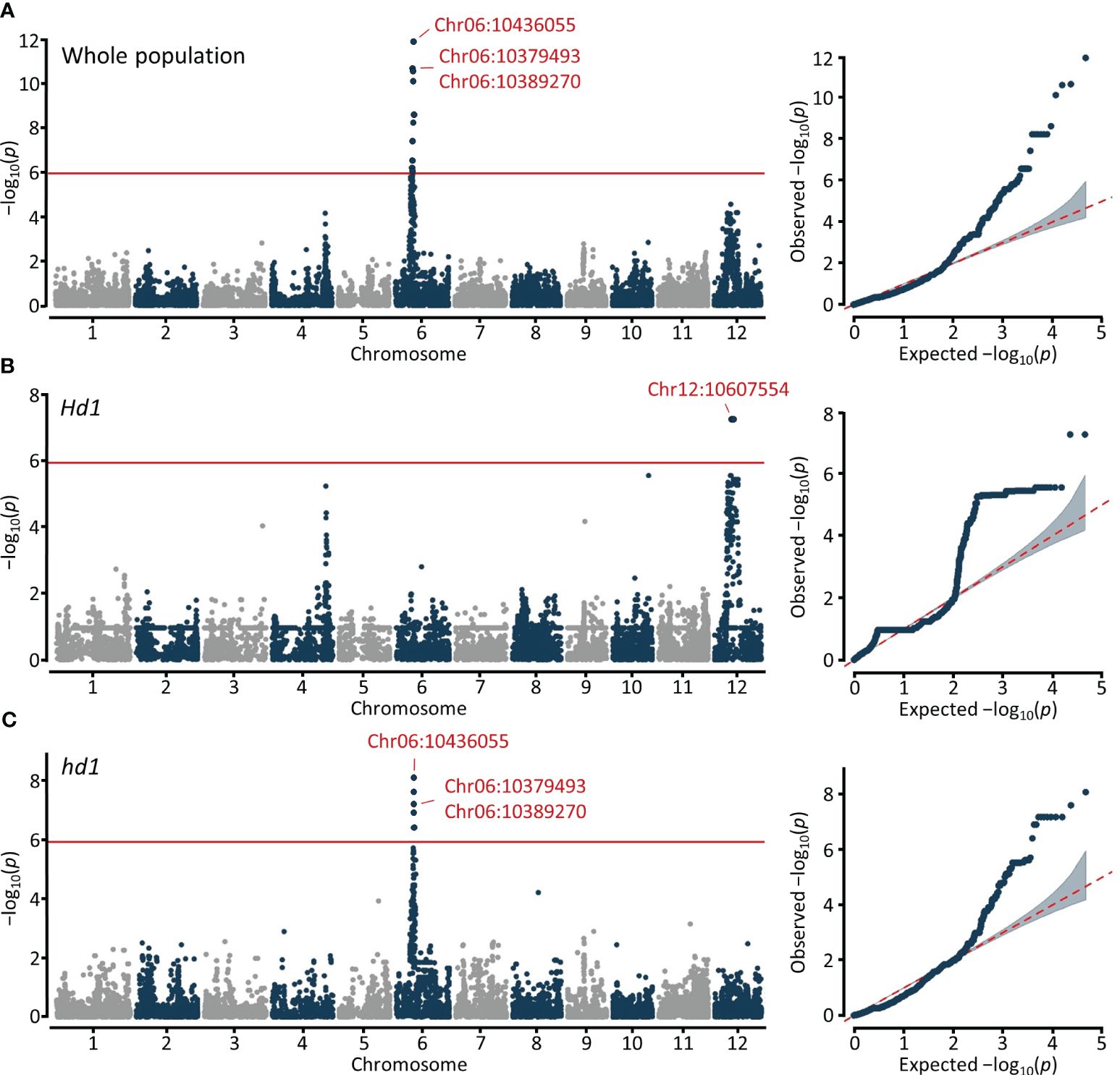
Figure 3 Manhattan and quantile-quantile (Q-Q) plots resulting from the GWAS for blast resistance in Korean rice cultivars using MLM. GWAS for blast resistance in (A) Whole population (n=296). (B) Functional Hd1 group (n=183). (C) Nonfunctional hd1 group (n=113). The x- and y-axis represent SNPs along each chromosome and −log10 (P) for the association, respectively. SNP positions in red indicate significant signals annotated as NBS-LRR.
3.4 Nucleotide diversity, selection, and haplotype analysis
Gene annotation revealed that three SNPs detected in the nonfunctional hd1 population were associated with NBS-LRR domain proteins at Piz locus: Os06g0286500, Os06g0286700, and Os06g0287500 (Figure 4A; Table 1). Since the physical distance between Hd1 and Piz locus was estimated to be approximately 1 Mb according to the Nipponbare reference genome, we evaluated the degree of polymorphism and the presence of artificial selection between the functionality of Hd1 and its relatedness with Piz. The nucleotide diversity of Hd1 population was lower than that of hd1 population within Hd1 gene region but showed no difference in Piz locus (Figure 4B). The Tajima’s D values for both populations were positive, with the Hd1 population exhibiting lower values than hd1 (Figure 4C). These results suggest that the lower nucleotide diversity observed in the Hd1 group may possibly be attributed to inbreeding depression and could have undergone domestication through balancing selection compared to the hd1 group (Tajima, 1989).
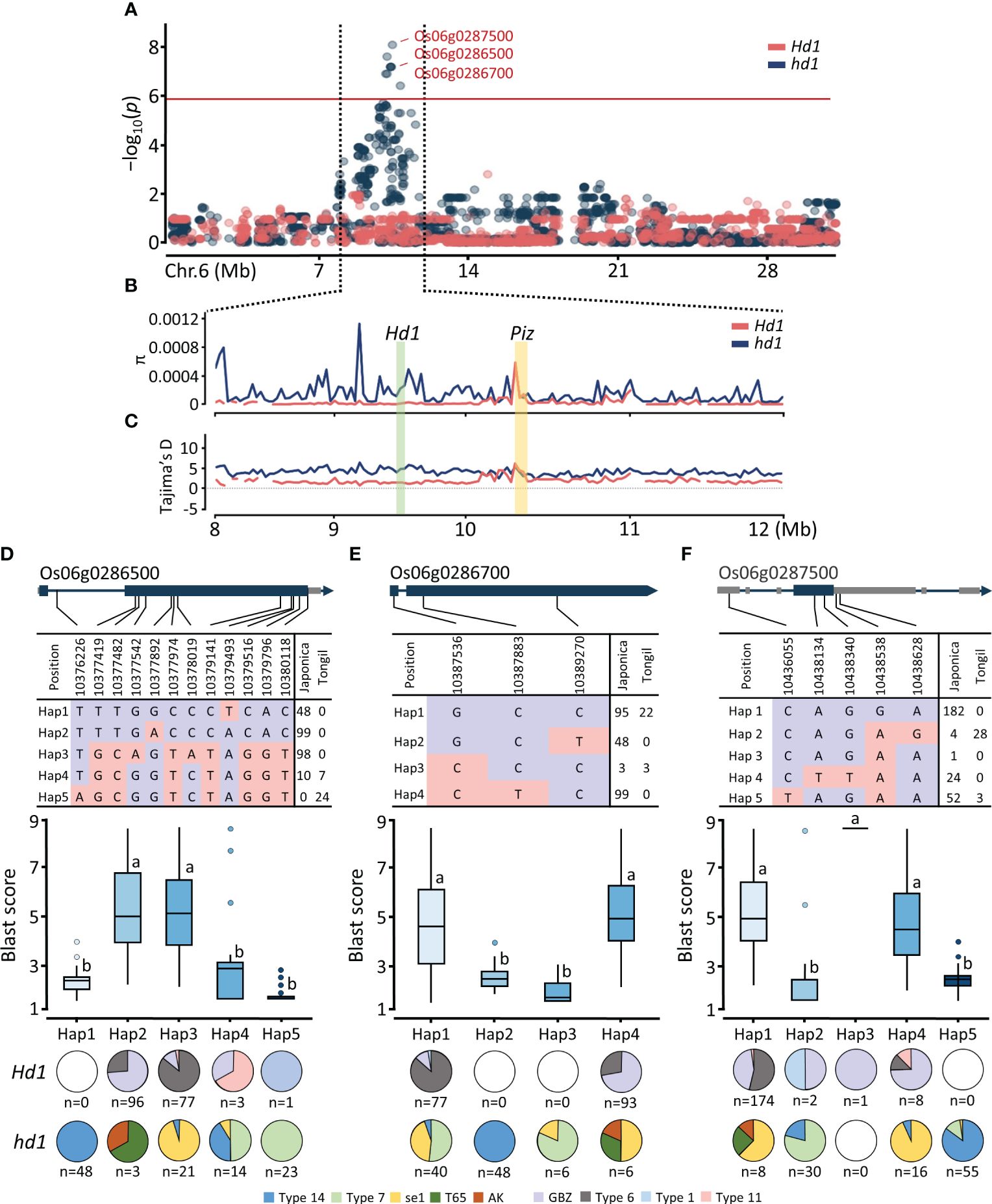
Figure 4 Regional Manhattan plot and haplotype analysis of Piz locus. (A) Regional Manhattan plot for the GWAS signals on chromosome 6. (B) Nucleotide diversity of Hd1 and Piz region with 10 kb sliding window. (C) Tajima’s D of Hd1 and Piz region with 10 kb sliding window. SNP variation, haplotype analysis, and distribution of Hd1 allele types of (D) Os06g0286500, (E) Os06g0286700, and (F) Os06g0287500. Different lowercase letters above the box plots indicate significant differences according to Scheffe’s method for post hoc comparison at P ≤ 0.05.
To investigate relationship between SNP variations and distribution of Hd1 allele types, we conducted haplotype analysis for the three NBS-LRR genes identified from the significant signals in Piz locus as determined by the MLM model (Supplementary Table 6). The allele types of Hd1 – four functional alleles (GBZ, Type 1, Type 6, and Type 11) and five nonfunctional alleles (se1, T65, Type 7, Type 14, and AK) – in 296 Korean cultivars are based on our previous report (Mo et al., 2021). Using 12 SNPs within the gene region of Os06g0286500, we identified five haplotypes. Among them, the synonymous A/T SNP (Chr06:10379493) was associated with blast resistance in the japonica subpopulation (Hap1). Additionally, the T/A SNP (Chr06:10376226) represented a specific SNP associated with blast resistance for the Tongil-type (Hap5) (Figure 4D). Notably, Os06g0286700_Hap2 exhibited japonica-specific blast resistance attributed to the C/T SNP previously reported as the causal variant (Wei et al., 2021), resulting in a nonsynonymous proline-to-leucine amino acid substitution (Figure 4E). Furthermore, both Os06g0286500_Hap1 and Os06g0286700_Hap2 were identified only in the cultivars possessing the nonfunctional Type 14 hd1. As of Os06g0287500, we identified five major haplotypes using five SNPs in the genic region. The majority of japonica cultivars (Hd1 = 174, hd1 = 8) were categorized as Hap 1. The significant C/T SNP (Chr06:10436055), annotated as a 5’ UTR premature start codon gain variant, was associated with blast resistance only in the cultivars possessing nonfunctional hd1 (Figure 4F). These findings revealed that Piz blast resistance is tightly linked to specific nonfunctional hd1 allele, with the association being specific to the Type 14 hd1 for japonica rice.
3.5 Haplotype analysis for Pi-ta
Based on the significant signals detected in the Pi-ta locus through GWAS analysis using a functional Hd1 population, we investigated the haplotype variation of Os12g0281300, which encodes Pi-ta protein belonging to NBS-LRR protein (Figure 5A). Based on 12 SNPs in the genic region, we identified five haplotypes of Os12g0281300 (Figure 5B). Hap1 was predominant in the japonica population (n=190), comprising 143 and 50 cultivars belonging to the Hd1 and hd1 groups, respectively. Hap4, prevalent among Tongil-type cultivars, exhibited significant blast resistance and can be determined by a A/G SNP at the Chr12:10607224 position (Figure 5C). Hap5 showed significant blast resistance, consisting of both Hd1 (n=36) and hd1 (n=32) group, attributed to the lead T/G SNP (Chr12:10607554). This SNP resulted in a nonsynonymous serine-to-alanine amino acid substitution, causing missense variant in the coding region. These results elucidate that blast resistance associated with the Pi-ta locus is widely distributed among early and mid-late maturing cultivars.
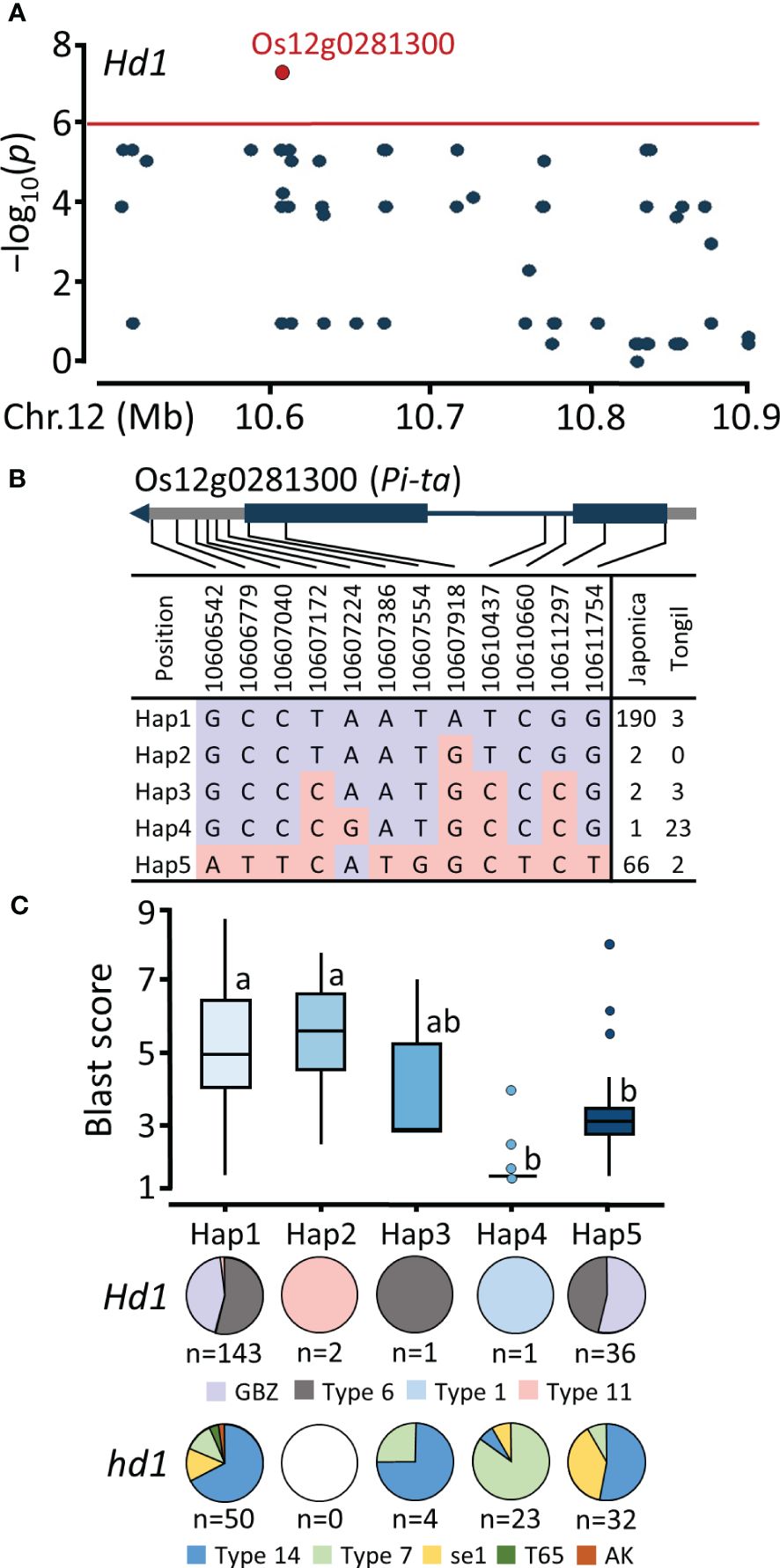
Figure 5 Regional Manhattan plot and haplotype analysis of Pi-ta locus. (A) Regional Manhattan plot for the candidate region on chromosome 12. (B) SNP variation of the Os12g0281300 gene region. (C) Haplotype analysis and distribution of Hd1 allele types. Different lowercase letters above the box plots indicate significant differences according to Scheffe’s method for post hoc comparison at P ≤ 0.05.
3.6 Geographical distribution of extended haplotype variation
Using RiceVarMap v2.0 (Zhao et al., 2015), we analyzed the extended haplotype frequency of the SNPs significantly associated with blast resistance along with the sequence variations responsible for Type 14 hd1 (japonica) and Type 7 hd1 (Tongil-type). The extended haplotype (EH) containing Type 14 hd1 along with significant SNPs associated with blast resistance at Piz locus showed no clear indications of geographical distribution (Figure 6A). In contrast, accessions carrying Type 7 hd1 with blast resistance were observed to be widely distributed in East and Southeast Asia, where SNPs associated with blast resistance might have originated. The 2-bp deletion in exon 2 (Yano et al., 2000), demonstrating Type 14 polymorphism (EH1–EH5) is widely distributed among tropical japonica and aus accessions. However, haplotype with tentative blast resistance is found in only a total of 29 accessions (Figure 6B). The 4-bp deletion in exon 2 serves as a distinguishing polymorphism of the Type 7 hd1 allele (Mo et al., 2021). The SNPs associated with blast resistance within accessions harboring Type 7 hd1, were predominantly distributed among indica accessions (Figure 6C). This observation was consistent with the findings reported by (Wu et al., 2020), where the mentioned mutation was frequently identified among indica accessions.
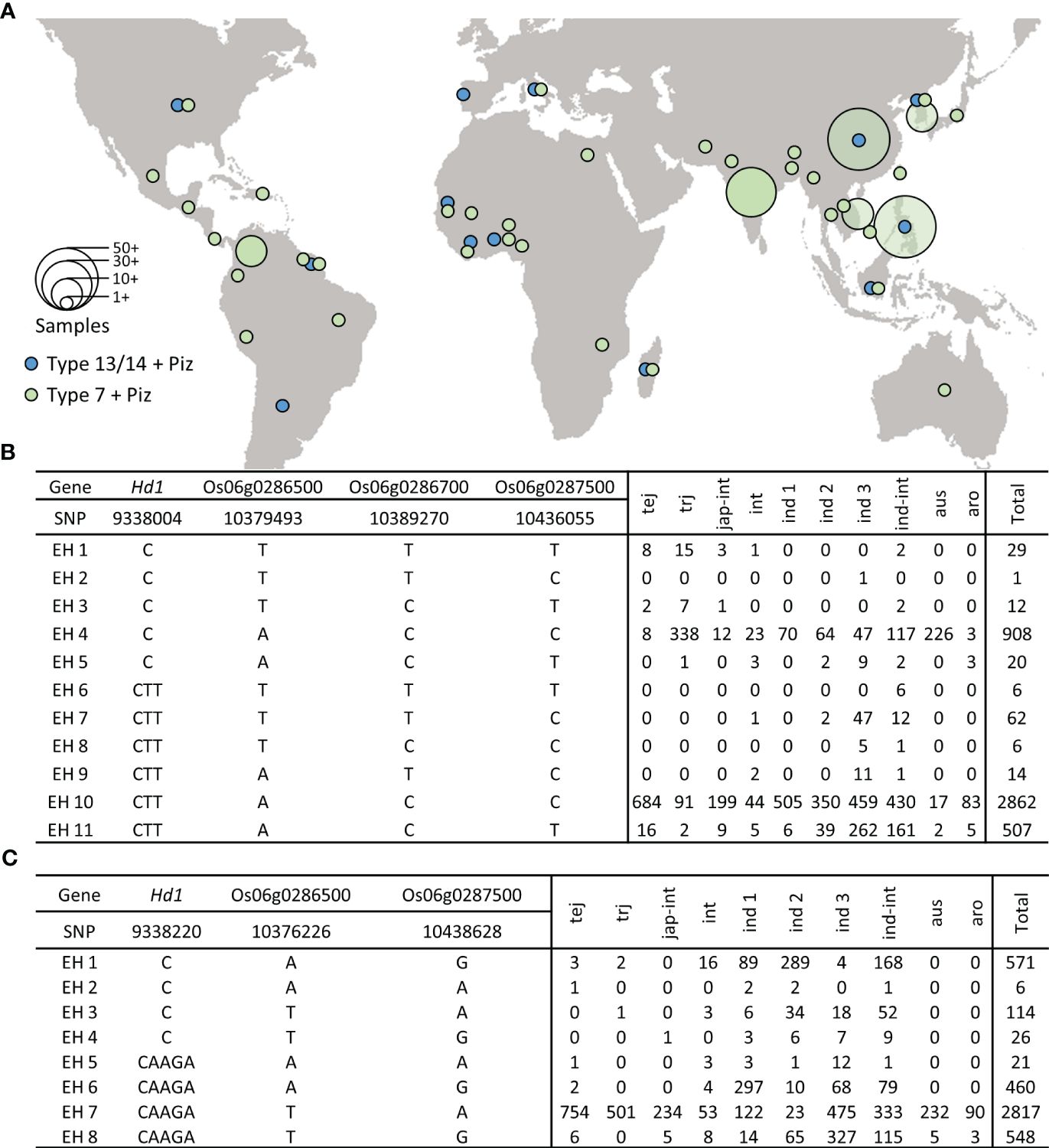
Figure 6 Geographical distribution and extended haplotype analysis of Piz. (A) Geographical distribution of accessions with japonica- and Tongil-specific Piz blast resistance. (B) Extended haplotype analysis of the SNPs significantly associated with blast resistance along with the sequence variations responsible for Type 14 hd1. (C) Extended haplotype analysis of the SNPs significantly associated with blast resistance along with the sequence variations responsible for Type 7 hd1. tej, Temperate japonica; trj, Tropical japonica; jap-int, japonica-intermediate; int, Intermediate; ind 1, indica I; ind 2, indica II; ind 3, indica III; ind-int, indica-intermediate; aus, Aus; aro, Aromatic.
4 Discussion
4.1 Utilizing elite cultivar panel as optimized breeding strategy
Crop domestication involves selective breeding of wild species, ultimately leading to the development of elite cultivars. This process is accompanied by a substantial reduction in genetic diversity, resulting in a low level of genetic variation and an increased susceptibility to genetic vulnerabilities (Meyer and Purugganan, 2013; Shi and Lai, 2015; He and Li, 2020). The narrow genetic diversity observed in the 296 cultivars examined in this study results from pre-breeding strategy, alleviating the performance gap between genetic donors and elite cultivars, aiming to facilitate the transfer of desirable trait into elite germplasm without compromising the phenotypic performance of the cultivar. For example, Saeilmi and Saeilpum are near-isogenic lines of Ilmibyeo and Ilpumbyeo, respectively. To enhance panicle blast resistance, Ilmibyeo was crossed with Hwayeongbyeo, possessing Pb1 gene, originating from indica cv. Modan (Lee et al., 2015). Similarly, Saeilpum possesses Xa3 and Pi20, enhancing bacterial leaf blight and leaf blast resistance derived from indica cv. IR24 (unpublished). Additionally, Anmi was developed by firstly transferring an introgression line derived from wild rice, O. australiensis (Bph18 donor), to the japonica background of Jinbubyeo, then crossing with Junam, an elite japonica cultivar with good grain quality (Suh et al., 2014). Therefore, despite the narrow genetic diversity, a wide range of phenotypic variation has been observed among various agronomic traits of Korean rice cultivars (Mo et al., 2020; Lee et al., 2023). Although association mapping with elite cultivars may not provide the diversity required to discover novel genes, it offers practical advantages because of its direct implementation in breeding programs designed for specific local environments (Begum et al., 2015; Fujino et al., 2015; Shinada et al., 2015; Verdeprado et al., 2018; Gudi et al., 2023, Gudi et al., 2024).
4.2 Pi9 associated with Type 14 hd1 confers blast resistance
The allelic variation of Hd1 is considered central to the heading date diversity in cultivated rice, with loss-of-function hd1 alleles being widely utilized to develop early maturing cultivars in Korea (Takahashi et al., 2009; Mo et al., 2021). Moreover, the loss-of-function hd1 alleles significantly contributes to the enhancement of rice adaptability in regions characterized by extended day length in high latitude areas (Fujino et al., 2019). The majority of cultivars harboring Pi40, an allelic variant of Piz (Jeung et al., 2007), exhibited early maturity and blast resistance (Cho et al., 2007; Lee et al., 2022), possibly attributed to the tight linkage between the Hd1 and Piz locus on chromosome 6 (Yokoo and Fujimaki, 1971; Yokoo and Kikuchi, 1977; Fukuta et al., 2009; Telebanco-Yanoria et al., 2010; Zhou et al., 2018). In this study, we discovered the causative C/T SNP at the gene region of Os06g0286700, annotated as Pi9 (Qu et al., 2006), which conferred strong blast resistance exclusively to cultivars with Type 14 hd1 (Figure 4E). Consistent with our findings, studies on quantitative trait nucleotide (QTN) library revealed Pi9 resistance allele at the same QTN site (Wei et al., 2021). In addition, the ‘T’ allele at the mentioned QTN site of Pi9, conferring broad spectrum resistance (Qu et al., 2006), exists in the indica line 75-1-127 (Liu et al., 2002; Deng et al., 2022), which was introgressed from tetraploid wild rice O. minuta (Amante-Bordeos et al., 1992). However, the elucidation of how the mentioned QTN site was introduced into Korean cultivars remains unclear. Type 14 hd1 was initially reported in the indica rice Kasalath (Yano et al., 2000), wherein a 2-bp deletion on exon 2 was found to be abundant among tropical japonica and aus worldwide accessions, which rarely possess the Pi9 resistance allele (Figure 6B). The primary donors for the Piz locus in Korean varieties are Japanese japonica varieties (Choi et al., 1989), with Jinbubyeo and Sambaegbyeo identified as secondary donors (Cho et al., 2007). Pedigree analysis of these donors suggest that Pi9 of Korean rice varieties did not originate from O. minuta (Choi et al., 1989). Using Pi9-specific markers, Korean japonica varieties tested negative, whereas Pi9 monogenic line IRBL9-W (Tsunematsu et al., 2000) exhibited positive, suggesting that the Pi9 present in Korean japonica varieties may potentially belong to multi R gene family (Cho et al., 2007). The frequency of potential resistance donors of the Pi9 allele comprises approximately 2.5% of worldwide germplasm, indicating its presence within natural variation. This suggests its probable application in enriching the genetic pool of blast resistance genes, thereby facilitating effective resistance breeding in rice.
4.3 Future prospects in selective breeding for blast resistant cultivars
Our prior investigation of molecular markers associated with the rice blast resistance revealed that among the multiple alleles at the Piz locus (Piz, Pi9(t), Pigm, Pi40, Piz-t/Piz-5, Piz-t, Pi2/Piz-5, and Pi9/Pi2), Pi40 exhibited the most significant association, accounting for 22.1% of the variation observed among early maturing cultivars in Korea (Lee et al., 2022). However, our results elucidated that Type 14 hd1-specific Pi9 displayed a higher association, representing 55.8% of blast resistance among early maturing cultivars. Moderately resistant cultivars were with Pi-ta resistance, while susceptible cultivars lacked both R genes. The se1 allele type hd1 has been widely utilized in rice breeding (Gómez-Ariza et al., 2015; Fujino et al., 2019; Leng et al., 2020), particularly in the development of early heading rice cultivars in Korea (Mo et al., 2021). However, cultivars possessing se1 type hd1 have shown a wide range of blast scores, ranging from moderate resistance to susceptibility, prompting rice breeders to utilize early maturing cultivars with blast resistance as source for breeding. Cultivars such as Odaebyeo, Unbongbyeo, and Jinbubyeo possess Type 14 hd1 and exhibit blast resistance. As mentioned above, they serve as primary and secondary donors of Piz locus and have been extensively utilized as sources for selective breeding to improve blast resistance among early maturing cultivars in Korea. Type 7 hd1 of Tongil-type rice, an indica-japonica hybrid, exhibit relatively stronger blast resistance compared to japonica rice. This may be attributed to the pyramiding effect of blast-resistant genes derived from indica rice. Previous studies have shown that the majority of Tongil-type rice tested positive for molecular markers specific to the blast-resistant genes Pib and Pigm, which originated from indica rice varieties, Engkatek (Wang et al., 1999) and Gumei 4 (Deng et al., 2017), respectively.
The main objective of modern crop breeding is to achieve both high yield and quality, often resulting in a reduction of genetic diversity within the population. We observed lower nucleotide diversity across the genome in the Hd1 population (5.3×10-5) compared to the hd1 population (2.1×10-4). This discrepancy led to distinct patterns in the Tajima’s D values across the genome: while the Hd1 population (-0.7) indicated directional selection, the hd1 (3.5) population showed signs of balancing selection (Supplementary Figures 3A, B). Cultivars possessing functional Hd1 are predominantly utilized as mid-late maturing varieties in Korea. Their prolonged vegetative stage facilitates sufficient biomass accumulation before flowering, resulting in higher yield and grain quality (Ye and Niu, 2018; Leng et al., 2020; Lee et al., 2024). This preference has driven intensified artificial selection over the past four decades, prioritizing high-yield and quality traits. The resistance gene Pi9, located at the Piz locus, has not been introduced into mid-late maturing cultivars in Korea (Supplementary Figure 3C). Thus, the introgression of multiple R gene hotspots from the Piz locus into mid-late maturing cultivars, by breaking the linkage with Hd1, could significantly enhance broad-spectrum blast resistance. This strategy would allow multiple R genes to recognize various effectors (Mukhtar et al., 2011; Zhang et al., 2015), providing valuable insights for the development of breeding blast resistant rice cultivars.
5 Conclusion
We conducted a genome-wide association study to elucidate the genetics underlying blast resistance associated with heading date among commercial rice cultivars in Korea. Through this analysis, we identified candidate genes associated with blast resistance, which were found to be specific to cultivars carrying certain allele types of Hd1. SNPs identified in our study provide useful resources for further gene validation and marker-assisted selection. Our study also demonstrates future insights on utilizing low-structured local commercial populations for detecting superior alleles regulating agronomic traits that can be directly adopted in breeding programs.
Data availability statement
The datasets presented in this study can be found in online repositories. The names of the repository/repositories and accession number(s) can be found in the article/Supplementary Material.
Author contributions
SYL: Conceptualization, Formal analysis, Investigation, Software, Validation, Visualization, Writing – original draft, Writing – review & editing. GL: Investigation, Writing – original draft. JH: Investigation, Writing – original draft. S-KH: Investigation, Writing – original draft. C-ML: Investigation, Writing – original draft. KK: Investigation, Writing – original draft. MJ: Investigation, Writing – original draft. J-PS: Writing – review & editing. J-UJ: Writing – review & editing. YM: Writing – review & editing. H-SL: Conceptualization, Investigation, Writing – original draft, Writing – review & editing.
Funding
The author(s) declare financial support was received for the research, authorship, and/or publication of this article. This work was supported by the “Development of maintaining seed viability and rice blast resistance breeding materials (Project No. PJ017269032024)” of the Rural Development Administration, Republic of Korea.
Acknowledgments
The authors express sincere appreciation to Dr. Su Jang from Seoul National University for offering valuable insights on data analysis.
Conflict of interest
The authors declare that the research was conducted in the absence of any commercial or financial relationships that could be construed as a potential conflict of interest.
Publisher’s note
All claims expressed in this article are solely those of the authors and do not necessarily represent those of their affiliated organizations, or those of the publisher, the editors and the reviewers. Any product that may be evaluated in this article, or claim that may be made by its manufacturer, is not guaranteed or endorsed by the publisher.
Supplementary material
The Supplementary Material for this article can be found online at: https://www.frontiersin.org/articles/10.3389/fpls.2024.1412614/full#supplementary-material
References
Amante-Bordeos, A., Sitch, L., Nelson, R., Dalmacio, R., Oliva, N., Aswidinnoor, H., et al. (1992). Transfer of bacterial blight and blast resistance from the tetraploid wild rice Oryza minuta to cultivated rice, Oryza sativa. Theor. Appl. Genet. 84, 345–354. doi: 10.1007/BF00229493
Baek, M.-K., Park, H.-S., Lee, C.-M., Lee, H.-J., Jeong, J.-M., Ahn, E.-K., et al. (2021). Identification of stable resistance genes based on resistance evaluation to blast for monogenic lines and leading japonica varieties in rice. Korean Soc. Breed. Sci. 53, 217–229. doi: 10.9787/KJBS.2021.53.3.217
Bates, D., Mächler, M., Bolker, B., Walker, S. (2014). Fitting linear mixed-effects models using lme4. arXiv preprint arXiv:1406.5823. 67 (1), 1–48. doi: 10.18637/jss.v067.i01
Begum, H., Spindel, J. E., Lalusin, A., Borromeo, T., Gregorio, G., Hernandez, J., et al. (2015). Genome-wide association mapping for yield and other agronomic traits in an elite breeding population of tropical rice (Oryza sativa). PloS One 10, e0119873. doi: 10.1371/journal.pone.0119873
Bradbury, P. J., Zhang, Z., Kroon, D. E., Casstevens, T. M., Ramdoss, Y., Buckler, E. S. (2007). TASSEL: software for association mapping of complex traits in diverse samples. Bioinformatics 23, 2633–2635. doi: 10.1093/bioinformatics/btm308
Cho, Y.-C., Kwon, S.-W., Choi, I.-S., Lee, S.-K., Jeon, J.-S., Oh, M.-K., et al. (2007). Identification of major blast resistance genes in Korean rice varieties (Oryza sativa L.) using molecular markers. J. Crop Sci. Biotech. 10, 265–276.
Choi, J. E., Park, J. S., Park, N. K. (1989). Resisitance of varieties to rice blast in korea I. Japonica type of rice varieties. Korean J. Agric. Sci. 16, 1–18.
Cingolani, P., Platts, A., Wang, L. L., Coon, M., Nguyen, T., Wang, L., et al. (2012). A program for annotating and predicting the effects of single nucleotide polymorphisms, SnpEff: SNPs in the genome of Drosophila melanogaster strain w1118; iso-2; iso-3. Fly 6, 80–92. doi: 10.4161/fly.19695
Danecek, P., Auton, A., Abecasis, G., Albers, C. A., Banks, E., DePristo, M. A., et al. (2011). The variant call format and VCFtools. Bioinformatics 27, 2156–2158. doi: 10.1093/bioinformatics/btr330
De Mendiburu, F., Simon, R. (2015). Agricolae-Ten years of an open source statistical tool for experiments in breeding, agriculture and biology. PeerJ. doi: 10.7287/peerj.preprints.1404v1
Deng, Y., Zhai, K., Xie, Z., Yang, D., Zhu, X., Liu, J., et al. (2017). Epigenetic regulation of antagonistic receptors confers rice blast resistance with yield balance. Science 355, 962–965. doi: 10.1126/science.aai8898
Deng, Z., Jiang, N., Fu, C.-J., Yan, T.-Z., Fu, X.-X., Hu, X.-C., et al. (2022). Analysis of blast resistance genes in Longliangyou and Jingliangyou hybrid rice varieties. Acta Agronomica Sin. 48 (5), 1071–1080. doi: 10.3724/SP.J.1006.2022.12002
Earl, D. A., VonHoldt, B. M. (2012). STRUCTURE HARVESTER: a website and program for visualizing STRUCTURE output and implementing the Evanno method. Conserv. Genet. Resour. 4, 359–361. doi: 10.1007/s12686-011-9548-7
Evanno, G., Regnaut, S., Goudet, J. (2005). Detecting the number of clusters of individuals using the software STRUCTURE: a simulation study. Mol. Ecol. 14, 2611–2620. doi: 10.1111/j.1365-294X.2005.02553.x
Fujino, K., Obara, M., Ikegaya, T. (2019). Establishment of adaptability to the northern-limit of rice production. Mol. Genet. Genomics 294, 729–737. doi: 10.1007/s00438-019-01542-2
Fujino, K., Obara, M., Shimizu, T., Koyanagi, K. O., Ikegaya, T. (2015). Genome-wide association mapping focusing on a rice population derived from rice breeding programs in a region. Breed. Sci. 65, 403–410. doi: 10.1270/jsbbs.65.403
Fukuta, Y., Xu, D., Yanoria, M. J. T., Hairmansis, A., Hayashi, N., Kobayashi, N. (2009). Genetic Characterization of Universal Differential Variety Sets Developed Under the IRRI-Japan Collaborative Research Project. In: Wang, GL., Valent, B. (eds) Advances in Genetics, Genomics and Control of Rice Blast Disease. (Dordrecht: Springer). doi: 10.1007/978-1-4020-9500-9_32
Gómez-Ariza, J., Galbiati, F., Goretti, D., Brambilla, V., Shrestha, R., Pappolla, A., et al. (2015). Loss of floral repressor function adapts rice to higher latitudes in Europe. J. Exp. Bot. 66, 2027–2039. doi: 10.1093/jxb/erv004
Gudi, S., Halladakeri, P., Singh, G., Kumar, P., Singh, S., Alwutayd, K. M., et al. (2024). Deciphering the genetic landscape of seedling drought stress tolerance in wheat (Triticum aestivum L.) through genome-wide association studies. Front. Plant Sci. 15. doi: 10.3389/fpls.2024.1351075
Gudi, S., Saini, D. K., Halladakeri, P., Singh, G., Singh, S., Kaur, S., et al. (2023). Genome-wide association study unravels genomic regions associated with chlorophyll fluorescence parameters in wheat (Triticum aestivum L.) under different sowing conditions. Plant Cell Rep. 42, 1453–1472. doi: 10.1007/s00299-023-03041-6
He, T., Li, C. (2020). Harness the power of genomic selection and the potential of germplasm in crop breeding for global food security in the era with rapid climate change. Crop J. 8, 688–700. doi: 10.1016/j.cj.2020.04.005
Hittalmani, S., Parco, A., Mew, T., Zeigler, R., Huang, N. (2000). Fine mapping and DNA marker-assisted pyramiding of the three major genes for blast resistance in rice. Theor. Appl. Genet. 100, 1121–1128. doi: 10.1007/s001220051395
Huang, X., Zhao, Y., Wei, X., Li, C., Wang, A., Zhao, Q., et al. (2012). Genome-wide association study of flowering time and grain yield traits in a worldwide collection of rice germplasm. Nat. Genet. 44, 32–39. doi: 10.1038/ng.1018
IRRI, I. (2002). Standard evaluation system for rice (SES) (Philippines: International Rice Research Institute).
Jeung, J., Kim, B., Cho, Y., Han, S., Moon, H., Lee, Y., et al. (2007). A novel gene, Pi40 (t), linked to the DNA markers derived from NBS-LRR motifs confers broad spectrum of blast resistance in rice. Theor. Appl. Genet. 115, 1163–1177. doi: 10.1007/s00122-007-0642-x
Kalia, S., Rathour, R. (2019). Current status on mapping of genes for resistance to leaf-and neck-blast disease in rice. 3 Biotech. 9, 1–14. doi: 10.1007/s13205-019-1738-0
Kang, H., Wang, Y., Peng, S., Zhang, Y., Xiao, Y., Wang, D., et al. (2016). Dissection of the genetic architecture of rice resistance to the blast fungus Magnaporthe oryzae. Mol. Plant Pathol. 17, 959–972. doi: 10.1111/mpp.12340
Kawahara, Y., de la Bastide, M., Hamilton, J. P., Kanamori, H., McCombie, W. R., Ouyang, S., et al. (2013). Improvement of the Oryza sativa Nipponbare reference genome using next generation sequence and optical map data. Rice 6, 1–10. doi: 10.1186/1939-8433-6-4
Lee, H.-S., Ha, S.-K., Lee, C.-M., Park, H.-S., Jeung, J.-U. (2022). Evaluation of molecular markers linked to rice blast resistance gene in Korean rice varieties. Korean J. Breed. Science. 54 (4), 375–384. doi: 10.9787/KJBS.2022.54.4.375
Lee, S. Y., Jeung, J.-U., Mo, Y. (2024). Allelic combinations of Hd1, Hd16, and Ghd7 exhibit pleiotropic effects on agronomic traits in rice. G3 Genes|Genomes|Genetics 4. doi: 10.1093/g3journal/jkad300
Lee, S. Y., Lee, H.-S., Lee, C.-M., Ha, S.-K., Park, H.-M., Lee, S.-M., et al. (2023). Multi-environment trials and stability analysis for yield-related traits of commercial rice cultivars. Agriculture 13, 256. doi: 10.3390/agriculture13020256
Lee, J.-H., Lee, J.-Y., Yoon, Y.-N., Kim, S.-Y., Hur, Y.-J., Yeo, U.-S., et al. (2015). Enhancement of panicle blast resistance in Korean rice cultivar ‘Saeilmi’by marker assisted backcross breeding. Plant Breed. Biotechnol. 3, 1–10. doi: 10.9787/PBB.2015.3.1.001
Leng, Y., Gao, Y., Chen, L., Yang, Y., Huang, L., Dai, L., et al. (2020). Using Heading date 1 preponderant alleles from indica cultivars to breed high-yield, high-quality japonica rice varieties for cultivation in south China. Plant Biotechnol. J. 18, 119–128. doi: 10.1111/pbi.13177
Letunic, I., Bork, P. (2021). Interactive Tree Of Life (iTOL) v5: an online tool for phylogenetic tree display and annotation. Nucleic Acids Res. 49, W293–W296. doi: 10.1093/nar/gkab301
Li, C., Wang, D., Peng, S., Chen, Y., Su, P., Chen, J., et al. (2019). Genome-wide association mapping of resistance against rice blast strains in South China and identification of a new Pik allele. Rice 12, 1–9. doi: 10.1186/s12284-019-0309-7
Lischer, H. E., Excoffier, L. (2012). PGDSpider: an automated data conversion tool for connecting population genetics and genomics programs. Bioinformatics 28, 298–299. doi: 10.1093/bioinformatics/btr642
Liu, X., Huang, M., Fan, B., Buckler, E. S., Zhang, Z. (2016). Iterative usage of fixed and random effect models for powerful and efficient genome-wide association studies. PloS Genet. 12, e1005767. doi: 10.1371/journal.pgen.1005767
Liu, G., Lu, G., Zeng, L., Wang, G.-L. (2002). Two broad-spectrum blast resistance genes, Pi9 (t) and Pi2 (t), are physically linked on rice chromosome 6. Mol. Genet. Genomics 267, 472–480. doi: 10.1007/s00438-002-0677-2
McCouch, S. R., Wright, M. H., Tung, C.-W., Maron, L. G., McNally, K. L., Fitzgerald, M., et al. (2016). Open access resources for genome-wide association mapping in rice. Nat. Commun. 7, 10532. doi: 10.1038/ncomms10532
Meyer, R. S., Purugganan, M. D. (2013). Evolution of crop species: genetics of domestication and diversification. Nat. Rev. Genet. 14, 840–852. doi: 10.1038/nrg3605
Mo, Y., Jeong, J.-M., Kim, B.-K., Kwon, S.-W., Jeung, J.-U. (2020). Utilization of elite Korean japonica rice varieties for association mapping of heading time, culm length, and amylose and protein content. Korean J. Crop Sci. 65, 1–21. doi: 10.7740/kjcs.2020.65.1.001
Mo, Y., Lee, C.-M., Park, H.-M., Ha, S.-K., Kim, M.-J., Kwak, J., et al. (2021). Hd1 allele types and their associations with major agronomic traits in Korean rice cultivars. Plants 10, 2408. doi: 10.3390/plants10112408
Mukhtar, M. S., Carvunis, A.-R., Dreze, M., Epple, P., Steinbrenner, J., Moore, J., et al. (2011). Independently evolved virulence effectors converge onto hubs in a plant immune system network. Science 333, 596–601. doi: 10.1126/science.1203659
Murray, M., Thompson, W. (1980). Rapid isolation of high molecular weight plant DNA. Nucleic Acids Res. 8, 4321–4326. doi: 10.1093/nar/8.19.4321
Oliveira-Garcia, E., Valent, B. (2015). How eukaryotic filamentous pathogens evade plant recognition. Curr. Opin. Microbiol. 26, 92–101. doi: 10.1016/j.mib.2015.06.012
Pritchard, J. K., Stephens, M., Donnelly, P. (2000). Inference of population structure using multilocus genotype data. Genetics 155, 945–959. doi: 10.1093/genetics/155.2.945
Qu, S., Liu, G., Zhou, B., Bellizzi, M., Zeng, L., Dai, L., et al. (2006). The broad-spectrum blast resistance gene Pi9 encodes a nucleotide-binding site–leucine-rich repeat protein and is a member of a multigene family in rice. Genetics 172, 1901–1914. doi: 10.1534/genetics.105.044891
R Core Team. (2013). R: A language and environment for statistical computing. (Vienna, Austria: R Foundation for Statistical Computing). Available at: https://www.R-project.org/.
Sakai, H., Lee, S. S., Tanaka, T., Numa, H., Kim, J., Kawahara, Y., et al. (2013). Rice Annotation Project Database (RAP-DB): an integrative and interactive database for rice genomics. Plant Cell Physiol. 54, e6. doi: 10.1093/pcp/pcs183
Shi, J., Lai, J. (2015). Patterns of genomic changes with crop domestication and breeding. Curr. Opin. Plant Biol. 24, 47–53. doi: 10.1016/j.pbi.2015.01.008
Shinada, H., Yamamoto, T., Sato, H., Yamamoto, E., Hori, K., Yonemaru, J., et al. (2015). Quantitative trait loci for rice blast resistance detected in a local rice breeding population by genome-wide association mapping. Breed. Sci. 65, 388–395. doi: 10.1270/jsbbs.65.388
Skamnioti, P., Gurr, S. J. (2009). Against the grain: safeguarding rice from rice blast disease. Trends Biotechnol. 27, 141–150. doi: 10.1016/j.tibtech.2008.12.002
Suh, J., Jeung, J., Kim, Y., Jena, K. K., Cho, Y., Lee, J., et al. (2014). A brown planthopper resistant and high grain quality rice variety'Anmi'developed by molecular breeding method. Korean J. Breed. Sci. 46, 152–159. doi: 10.9787/KJBS.2014.46.2.152
Tajima, F. (1989). Statistical method for testing the neutral mutation hypothesis by DNA polymorphism. Genetics 123, 585–595. doi: 10.1093/genetics/123.3.585
Takahashi, Y., Teshima, K. M., Yokoi, S., Innan, H., Shimamoto, K. (2009). Variations in Hd1 proteins, Hd3a promoters, and Ehd1 expression levels contribute to diversity of flowering time in cultivated rice. Proc. Natl. Acad. Sci. 106, 4555–4560. doi: 10.1073/pnas.0812092106
Telebanco-Yanoria, M. J., Koide, Y., Fukuta, Y., Imbe, T., Kato, H., Tsunematsu, H., et al. (2010). Development of near-isogenic lines of Japonica-type rice variety Lijiangxintuanheigu as differentials for blast resistance. Breed. Sci. 60, 629–638. doi: 10.1270/jsbbs.60.629
Tsunematsu, H., Yanoria, M. J. T., Ebron, L. A., Hayashi, N., Ando, I., Kato, H., et al. (2000). Development of monogenic lines of rice for blast resistance. Breed. Sci. 50, 229–234. doi: 10.1270/jsbbs.50.229
Varshney, R. K., Nayak, S. N., May, G. D., Jackson, S. A. (2009). Next-generation sequencing technologies and their implications for crop genetics and breeding. Trends Biotechnol. 27, 522–530. doi: 10.1016/j.tibtech.2009.05.006
Verdeprado, H., Kretzschmar, T., Begum, H., Raghavan, C., Joyce, P., Lakshmanan, P., et al. (2018). Association mapping in rice: basic concepts and perspectives for molecular breeding. Plant Production Sci. 21, 159–176. doi: 10.1080/1343943X.2018.1483205
Wang, C., Yang, Y., Yuan, X., Xu, Q., Feng, Y., Yu, H., et al. (2014). Genome-wide association study of blast resistance in indica rice. BMC Plant Biol. 14, 1–11. doi: 10.1186/s12870-014-0311-6
Wang, Z. X., Yano, M., Yamanouchi, U., Iwamoto, M., Monna, L., Hayasaka, H., et al. (1999). The Pib gene for rice blast resistance belongs to the nucleotide binding and leucine-rich repeat class of plant disease resistance genes. Plant J. 19, 55–64. doi: 10.1046/j.1365-313X.1999.00498.x
Wei, X., Qiu, J., Yong, K., Fan, J., Zhang, Q., Hua, H., et al. (2021). A quantitative genomics map of rice provides genetic insights and guides breeding. Nat. Genet. 53, 243–253. doi: 10.1038/s41588-020-00769-9
Wu, C.-C., Wei, F.-J., Chiou, W.-Y., Tsai, Y.-C., Wu, H.-P., Gotarkar, D., et al. (2020). Studies of rice Hd1 haplotypes worldwide reveal adaptation of flowering time to different environments. PloS One 15, e0239028. doi: 10.1371/journal.pone.0239028
Yano, M., Katayose, Y., Ashikari, M., Yamanouchi, U., Monna, L., Fuse, T., et al. (2000). Hd1, a major photoperiod sensitivity quantitative trait locus in rice, is closely related to the Arabidopsis flowering time gene CONSTANS. Plant Cell 12, 2473–2483. doi: 10.1105/tpc.12.12.2473
Ye, J., Niu, X. (2018). Divergent Hd1, Ghd7, and DTH7 alleles control heading date and yield potential of japonica rice in Northeast China. Front. Plant Sci. 9. doi: 10.3389/fpls.2018.00035
Yin, L., Zhang, H., Tang, Z., Xu, J., Yin, D., Zhang, Z., et al. (2021). rMVP: a memory-efficient, visualization-enhanced, and parallel-accelerated tool for genome-wide association study. Genomics Proteomics Bioinf. 19, 619–628. doi: 10.1016/j.gpb.2020.10.007
Yokoo, M., Fujimaki, H. (1971). Tight linkage of blast-resistance with late maturity observed in different indica varieties of rice. Japanese J. Breed. 21, 35–39. doi: 10.1270/jsbbs1951.21.35
Yokoo, M., Kikuchi, F. (1977). Multiple allelism of the locus controlling heading time of rice, detected using the close linkage with blast-resistance. Japanese J. Breed. 27, 123–130. doi: 10.1270/jsbbs1951.27.123
Yu, J., Pressoir, G., Briggs, W. H., Vroh Bi, I., Yamasaki, M., Doebley, J. F., et al. (2006). A unified mixed-model method for association mapping that accounts for multiple levels of relatedness. Nat. Genet. 38, 203–208. doi: 10.1038/ng1702
Zhang, X., Yang, S., Wang, J., Jia, Y., Huang, J., Tan, S., et al. (2015). A genome-wide survey reveals abundant rice blast R genes in resistant cultivars. Plant J. 84, 20–28. doi: 10.1111/tpj.12955
Zhao, K., Tung, C.-W., Eizenga, G. C., Wright, M. H., Ali, M. L., Price, A. H., et al. (2011). Genome-wide association mapping reveals a rich genetic architecture of complex traits in Oryza sativa. Nat. Commun. 2, 467. doi: 10.1038/ncomms1467
Zhao, H., Yao, W., Ouyang, Y., Yang, W., Wang, G., Lian, X., et al. (2015). RiceVarMap: a comprehensive database of rice genomic variations. Nucleic Acids Res. 43, D1018–D1022. doi: 10.1093/nar/gku894
Zhou, X., Jiang, G., Yang, L., Qiu, L., He, P., Nong, C., et al. (2018). Gene diagnosis and targeted breeding for blast-resistant Kongyu 131 without changing regional adaptability. J. Genet. Genomics 45, 539–547. doi: 10.1016/j.jgg.2018.08.003
Keywords: GWAS, rice blast, heading date, haplotype analysis, rice breeding
Citation: Lee SY, Lee G, Han J, Ha S-K, Lee C-M, Kang K, Jin M, Suh J-P, Jeung J-U, Mo Y and Lee H-S (2024) GWAS analysis reveals the genetic basis of blast resistance associated with heading date in rice. Front. Plant Sci. 15:1412614. doi: 10.3389/fpls.2024.1412614
Received: 05 April 2024; Accepted: 06 May 2024;
Published: 21 May 2024.
Edited by:
Dayun Tao, Yunnan Academy of Agricultural Sciences, ChinaReviewed by:
Gurjeet Singh, Texas A and M University, United StatesPartha Biswas, Bangladesh Rice Research Institute, Bangladesh
Copyright © 2024 Lee, Lee, Han, Ha, Lee, Kang, Jin, Suh, Jeung, Mo and Lee. This is an open-access article distributed under the terms of the Creative Commons Attribution License (CC BY). The use, distribution or reproduction in other forums is permitted, provided the original author(s) and the copyright owner(s) are credited and that the original publication in this journal is cited, in accordance with accepted academic practice. No use, distribution or reproduction is permitted which does not comply with these terms.
*Correspondence: Hyun-Sook Lee, bGVlaHMwMTA3QGdtYWlsLmNvbQ==; Youngjun Mo, eWptb0BqYm51LmVkdQ==