- Aula Dei Experimental Station, EEAD, CSIC, Zaragoza, Spain
The process of allocating resources to different plant organs in the early stage of development can affect their adaptation to drought conditions, by influencing water uptake, transpiration, photosynthesis, and carbon storage. Early barley development can affect the response to drought conditions and mitigate yield losses. A distinct behavior of biomass partitioning between two Spanish barley landraces (SBCC073 and SBCC146) was observed in a previous rhizotron experiment. An RIL population of approximately 200 lines, derived from the cross of those lines, was advanced using speed breeding. We devised an experiment to test if seedling biomass partitioning was under genetic control, growing the seedlings in pots filled with silica sand, in a growth chamber under controlled conditions. After 1 week, the shoot and root were separated, oven dried, and weighted. There were genotypic differences for shoot dry weight, root dry weight, and root-to-shoot ratio. The population was genotyped with a commercial 15k SNP chip, and a genetic map was constructed with 1,353 SNP markers. A QTL analysis revealed no QTL for shoot or root dry weight. However, a clear single QTL for biomass partitioning (RatioRS) was found, in the long arm of chromosome 5H. By exploring the high-confidence genes in the region surrounding the QTL peak, five genes with missense mutations between SBCC146 and SBCC073, and differential expression in roots compared to other organs, were identified. We provide evidence of five promising candidate genes with a role in biomass partitioning that deserve further research.
1 Introduction
Photosynthate allocation and partitioning encompass the regulatory processes governing the distribution of fixed carbon within plants. These regulatory mechanisms dictate the allocation of carbon toward storage, intracellular metabolism, or immediate transport to sink tissues. In sink tissues, sugars are allocated toward growth processes of different plant organs (Taiz et al., 2015). On the other hand, partitioning refers to the selective distribution of photosynthates throughout the entire plant. Partitioning mechanisms dictate the amounts of fixed carbon directed toward specific sink tissues. Processes, such as phloem loading and unloading, alongside photosynthate allocation and partitioning, are subjects of significant research interest due to their pivotal roles in enhancing crop productivity (Slafer et al., 2023).
Over the life cycle of the plants, the dynamics of photosynthate allocation result in allometric growth of plant tissues, with an effect on the relative size of the organs and, hence, on the ability to capture the resources from the surrounding environment. Plants devoting more growth to roots invest more in foraging for water and nutrients, whereas plants investing more on shoots and leaves maximize radiation and CO2 capture (Siddiqui et al., 2021). Then, to have reproductive and agronomic success, plants must be able to derive an important part of resources toward the reproductive organs, to produce healthy seeds in the largest number possible.
To identify bottlenecks limiting growth and yield, plant physiologists classically take a source-sink perspective. This is a view of growth at the whole-plant scale incorporating mechanistic interactions between physiology, resource allocation, and plant development (White et al., 2016). Sink strength can be defined as the ability of a sink tissue to mobilize photosynthate by itself. It depends on two components: the sink size (total weight of sink tissue) and the sink activity (rate of uptake photosynthates per unit weight of sink tissue). Tissues can be simultaneously source and sink (roots are a source for nitrogen and a sink for carbon). The pull strength of sinks determines the allometric growth. Biomass partitioning between roots and shoots has been identified as an adaptive factor in tree species (Aranda et al., 2010; Voltas et al., 2024), even at seedling stage (Corcuera et al., 2012), with intraspecific variation. In general, populations coming from arid environments show a larger investment in roots than populations coming from humid environments (Lombardi et al., 2021). This feature confers a higher capacity to scavenge for water, thus becoming advantageous for the plants in water-limited environments. Several studies have already looked into biomass partitioning between root and shoot in barley (Bertholdsson and Kolodinska Brantestam, 2009), in some cases finding phenotypic diversity (Voss-Fels et al., 2018; Wang et al., 2021). Some studies looked for QTL in different populations involving a cross of wild and cultivated barley (Arifuzzaman et al., 2014), a wide panel of wild and cultivated barley (Reinert et al., 2016), spring cultivars (Abdel-Ghani et al., 2019), or spring landraces (Khodaeiaminjan et al., 2023). In all these cases, a few QTL for root-to-shoot ratio (calculated in different manners) were found. There are no studies, however, focusing on winter landraces, which make the most abundant barley landrace groups in Spain (Yahiaoui et al., 2008).
Three lines of the Spanish Barley Core Collection (SBCC042, SBCC073, and SBCC146) and three cultivars (Cierzo, Orria, and Scarlett) were tested previously under control and drought conditions for root and shoot growth (Boudiar et al., 2020). This experiment was carried out for 4 weeks using the rhizotrons of the GrowScreen-Rhizo phenotyping platform (Nagel et al., 2012) from the Plant Sciences, Forschungszentrum Jülich GmbH, Germany. It was found that SBCC073 devoted relatively more resources to roots, whereas SBCC146 devoted relatively more resources to shoots. These two lines also showed good field performance, particularly SBCC073, which was the best line of a large set of landraces and modern cultivars tested in a field network in Spain (Yahiaoui et al., 2014).
Our research question is to find out whether genetic variation for biomass partitioning between roots and shoots exists in winter barley landraces from Spain, and to discover QTL that may underlie its genetic control. We will use a biparental barley RIL population that, based on earlier evidence, has the potential to show differences in early allometric growth.
2 Materials and methods
2.1 Plant material development
The population was developed from an F1 cross between inbred lines SBCC073 and SBCC146. These lines were developed by single seed descent from two Spanish six-rowed landraces, belonging to the two main germplasm groups detected in the Spanish Barley Core Collection (SBCC), using molecular markers (Yahiaoui et al., 2008). SBCC073 comes from the southern part of the country, with warm temperatures and high evapotranspiration, and SBCC146 from cooler areas in the central plateau region. The original cross was performed in 2016. The F1 was multiplied in the field the following year, and the F2 was sown in a growth chamber in the 2018–2019 season. A total of 232 spikes from individual plants were harvested in the F3 plot, to start producing a recombinant inbred line population by single seed descent (SSD). The seed was advanced from F3 to F5 by two generations of speed breeding in a growth chamber following the protocols of Ghosh et al. (2018). The F5 plants were then multiplied in the field in the season 2019–2020. At the end of this process, 195 families were left, which were field multiplied and/or tested in the ensuing seasons. The remaining F6 lines were sown in the field in the 2020–2021 season, for seed multiplication, in plots of two rows, 1-m long, without replication, at the experimental fields of EEAD-CSIC.
2.2 Phenotyping test
One hundred and ninety-three genotypes derived from the cross of SBCC073 and SBCC146, and the parents, were tested for root dry weight (RDW), shoot dry weight (SDW), total dry weight (TDW), and root/shoot ratio (RatioRS). Five varieties were used as fillers. We devised a bespoke phenotyping test, for which several sand substrates were evaluated in several tests, until an appropriate type was found. The sand chosen produced barley plants of normal aspect and development and allowed easy washing of the roots. The particularity of this phenotyping test lies in the choice of substrate; since one of the main drawbacks of using commercial substrates is the impossibility of eliminating all traces of soil adhered to the root.
Seeds were pre-germinated in Petri dishes. After 72 h, five similar size germinated seeds of each line were transferred to pots (18-cm high, 15-cm outer diameter) filled with 2 L of silica sand (0.4–0.8 mm) and irrigated with 100 ml of Hoagland nutrient solution 0.2× (Figure 1A). For 1 week, plants developed in a growth chamber under 16-h light/8-h night photoperiod, at 22°C/18°C, 70% humidity, and 250 µmol m−2 s−1. Pots were irrigated with 50 ml of Hoagland 0.2× twice during the experiment. After 1 week, plants were extracted from pots, and roots were cleaned using low-pressure tap water, carefully (Figure 1B). Roots and shoots were air dried during a few hours (Figure 1C) and then transferred to paper envelopes. All plants from each pot were kept together in the same envelope (five plants per pot were the experimental unit). Seedlings with abnormal root or shoot development were discarded. Abnormal plants were discarded visually after extracting and cleaning the roots. Extremely short shoot and/or root compared to the other plants of the same genotype were discarded. Roots and shoots were oven dried for 72 h at 70°C (Figure 1D) and weighted in a precision scale (Ohaus PR223/E).
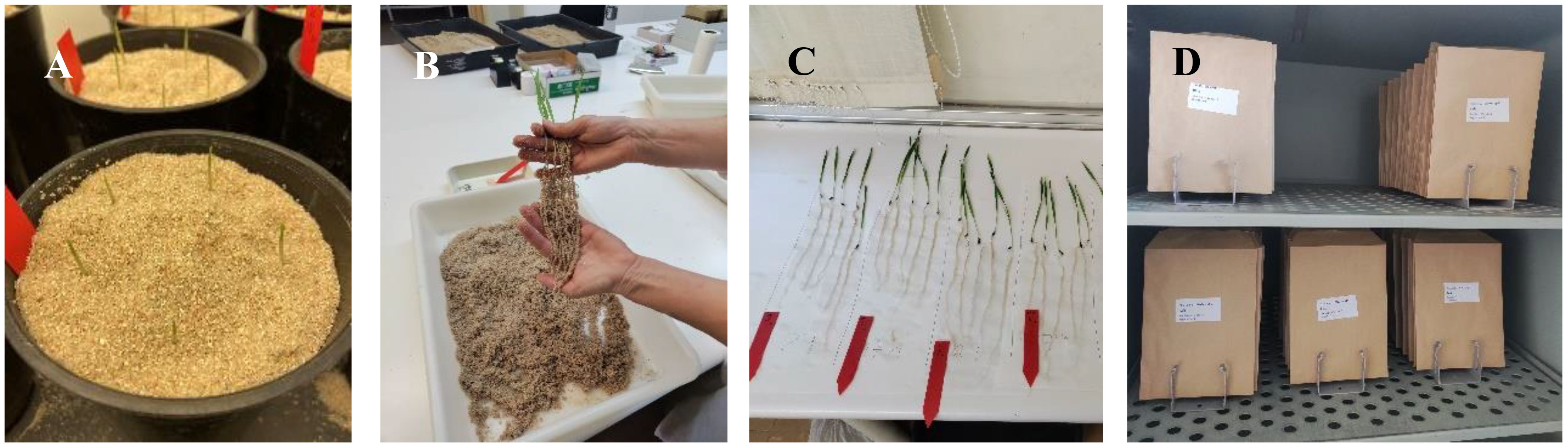
Figure 1. Development of the different experimental phases. (A) Pre-germinated seedlings just transplanted to the pot. (B) Root extraction. (C) Roots and shoot air dried. (D) Shoots and roots oven dried.
The experiment comprised four replications of 200 genotypes each. Four rounds, being 50 genotypes studied in each round, formed each replication. Each round (50 pots, in five trays) was planted and transferred to the growth chamber in a single day. Two or three sowing dates were performed per week. Each genotype was replicated four times (four pots), so a total of 800 pots were used in the experiment. The experimental unit for each genotype was the aggregated value of five seedlings planted in a single pot. Recombinant inbred lines (RIL), checks, and parents (200 genotypes in total) were randomized in five trays, with 10 pots on each tray. Four rounds of five trays conformed one repetition of the whole experiment. Trays were numbered, and their positions inside the growth chamber were consistent across the experiment. When abnormally developed plants were discarded, the data taken from the remaining four plants were multiplied by the appropriate factor to equalize them to the other observations.
2.3 Statistical analysis
Statistical analyses were performed, considering growth chamber position, round, repetition, and round nested within repetition as random effects. The variance components for the population and parents were obtained using dummy variables for RILS vs. parents, parents, and RILs as in Piepho et al. (2006). Best linear unbiased estimations (BLUEs) for each RIL were obtained considering genotype as fixed effect. To control for the possible influence of seed size on the traits measured, additional analyses were run with thousand kernel weight (TKW) as covariate. All models were run using ASReml-R (Butler et al., 2017). Means and confidence intervals were obtained using the R package “predictmeans” (Luo et al., 2018).
2.4 QTL analysis
DNA was extracted from 7-day-old individual seedlings using the EchoLUTION Plant DNA kit (BioEcho, GmbH). Genotyping was performed with a proprietary 15k Barley Infinium SNP array by SGS Institute Fresenius GmbH, TraitGenetics Section. A genetic map was then constructed for 193 F5 RIL lines with JoinMap 4, assigning 3,566 SNP markers to seven linkage groups. After filtering co-segregating markers, 1,353 SNPs with unique map position were used for QTL analysis (Supplementary Table S1), with the software Genstat Release 22.1 (VSN International, 2022). For each trait, QTL analysis was carried out using composite interval mapping, calculating genetic predictors every 3 cM, setting the minimum distance for QTL peaks to 20 cM, and the minimum distance between cofactors to 30 cM. The procedure was run iteratively until the number of QTLs detected stabilized. The Li and Ji (2005) method was used to estimate a 5% genome-wide significance threshold for the −log10 p-values. For each marker, physical position in the Morex V3 reference genome (Mascher et al., 2021) was retrieved from Barleymap (Cantalapiedra et al., 2015).
2.5 Searching for candidate genes
Gene models in the reference genome Morex V3 (Mascher et al., 2021), within the QTL confidence interval, were retrieved using Barleymap (Cantalapiedra et al., 2015). Then, genes were classified into high- (HC) or low-confidence classes, and the HC genes with design of exome capture targets were identified in BARLEX (Colmsee et al., 2015). The subset of exome-captured HC genes was further reduced to those genes showing SNP polymorphisms between exome capture data of the parents, which was available in-house (Casas et al., 2018). Exome sequencing was performed previously according to the methods described by Mascher et al. (2013). DNA sequencing, made at CNAG (Centro Nacional de Análisis Genómico, Barcelona), and data analysis were performed as described by Cantalapiedra et al. (2016). Expression data from a selected set of genes was retrieved from the Barley Expression Database (Li et al., 2023). The selected genes were clustered according to their expression in informative experiments and tissues from the expression database using R function heatmap.2 in package gplots (Warnes et al., 2016). Presence of the identified polymorphisms was validated by comparison to the SBCC073 transcriptome (Cantalapiedra et al., 2017) and the barley pangenome (Jayakodi et al., 2020). SIFT scores of non-synonymous protein mutations were retrieved from Ensembl Plants using recipe 8 from Contreras-Moreira et al. (2022). Collinear pangene clusters containing gene models of interest from MorexV3 and the other accessions in the pangenome, built with version 11012024 of GET_PANGENES (Contreras-Moreira et al., 2023), were retrieved from https://eead-csic-compbio.github.io/barley_pangenes. Alignment of amino acid sequences of candidate genes were plotted with NCBI MSA Viewer 1.25.0. We used the Frequency-Based Differences method, which assigns scores to bases based on their representation in the column’s frequency profile.
3 Results
3.1 Genotypic differences in seedling growth
The two parents showed quite divergent values for all traits, and the distributions of the variables for the population showed a smooth quantitative variation, when lines were ordered from lowest to highest values, for all four traits considered (Figure 2, Supplementary Table S2).
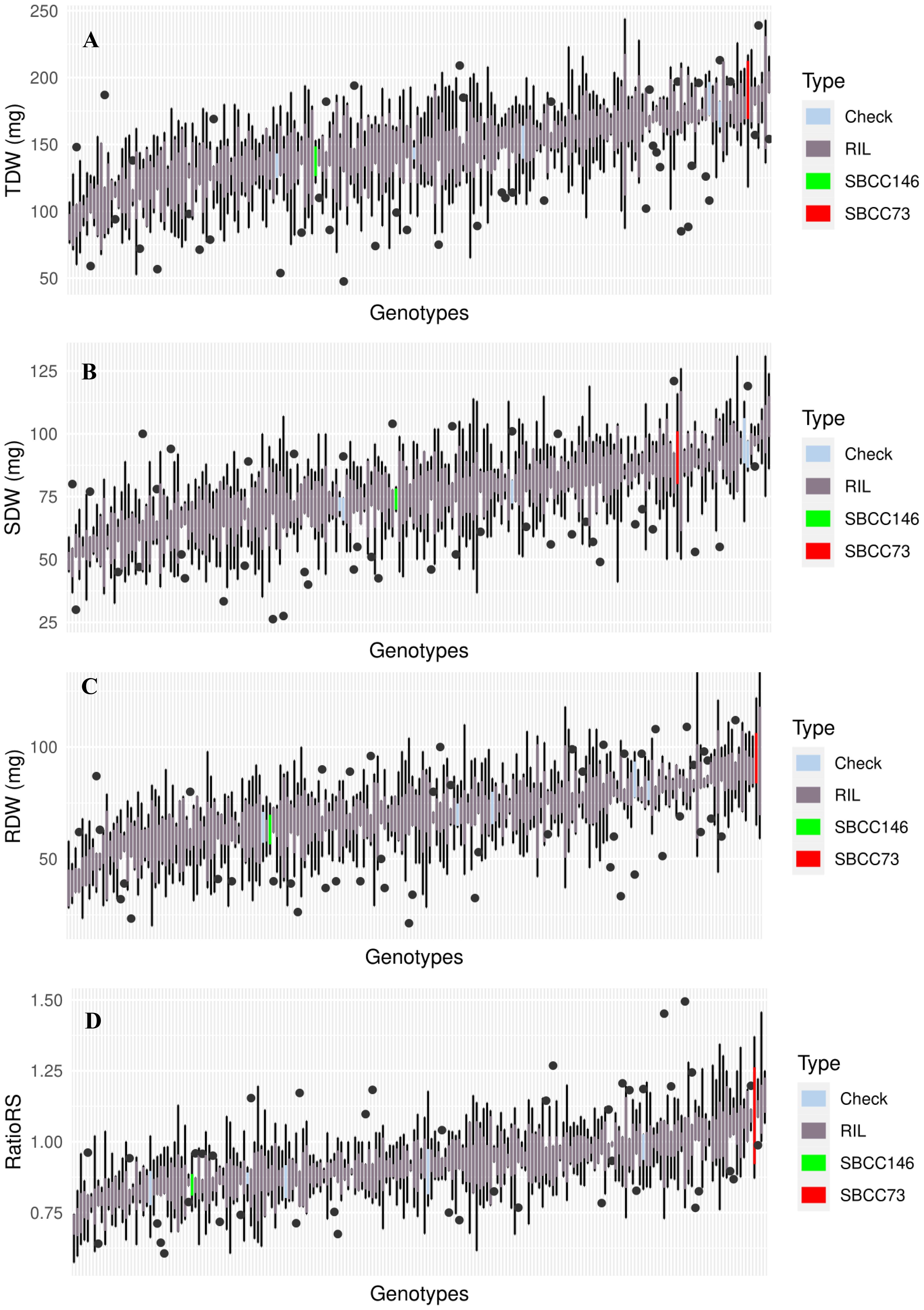
Figure 2. Boxplot representing median, minimum, and maximum of (A) TDW, (B) SDW, (C) RDW, and (D) RatioRS of the whole set of genotypes tested in the experiment. In red, parent SBCC073; in green, parent SBCC0146; in dark gray, a set of RIL; and in light blue, five genotypes as checks.
The analyses of variance revealed significant genotypic differences for all four traits considered, for the population lines, and for parents (Table 1). Parent SBCC073 showed higher RDW and SDW than SBCC146, although the difference was not significant. SBCC073 turned out to present the highest RDW mean value of the whole experiment. The parents of the population only showed differences in RatioRS, even when TKW was used as a covariate (Supplementary Figure S1), confirming the results of Boudiar et al. (2020). Considering these traits, SBCC073 exhibits more RDW, SDW, TDW, and RatioRS; therefore, it appears to be a genotype that invests more photosynthetic assimilates in both parts when compared to the other parent of the population, SBCC146.
3.2 QTL analysis
A single QTL was detected for the RatioRS trait, located on the long arm of chromosome 5H at 66.6 cM (400,664,453 bp) (Figure 3), explaining 15.2% of the phenotypic variance. The allele of SBCC073 led to increasing the ratio by 0.062. This QTL was independent of seed size, as it was also detected when TKW was used as covariate. Interestingly, no other QTL was detected for any other traits, although there were genotypic differences for all of them (Table 1) suggesting that the partitioning QTL detected was instrumental in the phenotypic differences found for seedling architecture.
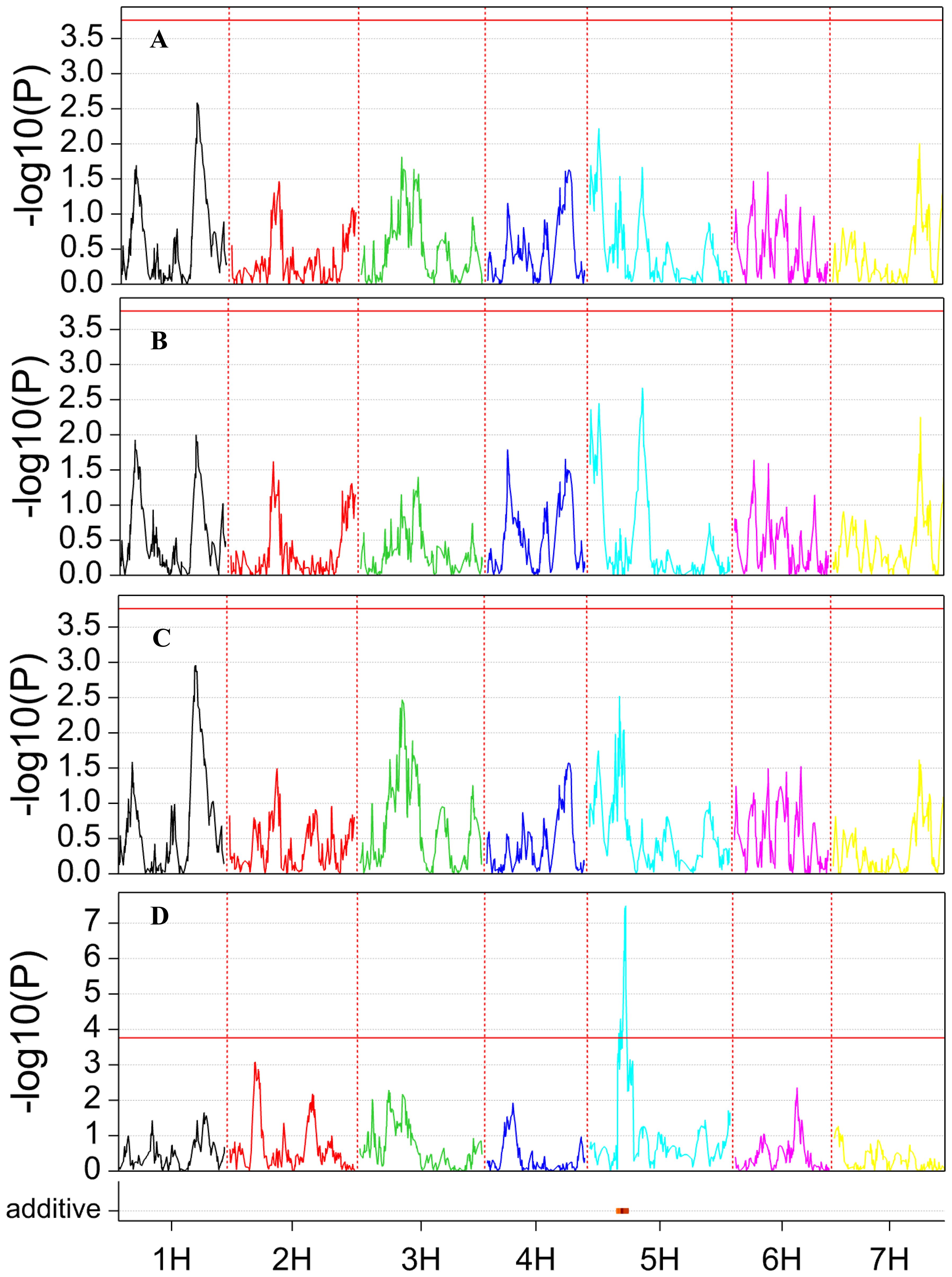
Figure 3. QTL scan for the traits recorded in this study. (A) TDW, (B) SDW, (C) RDW, and (D) RatioRS. At the bottom of the graphs, a red dash indicates the presence of a QTL for which SBCC073 contributes the allele with the larger value.
3.3 Candidate genes
The peak marker for the RatioRS QTL was BOPA2_12_10725 located within gene HORVU.MOREX.r3.5HG0475170. The QTL confidence interval ranged from 62.6 to 66.9 cM, or 350,508,439 to 403,211,990 bp in the reference genome. The region contains 589 gene models, with 270 HC genes, including 189 with exome capture data. For each of those genes, inspection of putative variants between SBCC146 and SBCC073 recognized 102 genes without variants, 43 genes with polymorphisms outside the coding region, 22 genes with synonymous SNPs, and 22 genes with non-synonymous SNPs. We examined the annotated gene descriptions and constructed a heat map, based on expression data, for 24 genes, including those with non-synonymous SNPs (Supplementary Tables S3 and S4; Supplementary Figure S2). SBCC073 displayed missense mutations in four genes that showed higher expression in roots than in other tissues making them good candidate genes (Supplementary Table S5). Alignment of amino acid sequences for the candidate genes in the barley pangenome revealed natural variation for all of them (Supplementary Figure S3). Thus, five polymorphisms were found in gene HORVU.MOREX.r3.5HG0473970, Glycosyltransferase (D344V, R362Q, R413H, K430E, and V469A). The variants identified in SBCC073 were also found in pangenome genotypes, such as HOR13942 or RGT Planet, so they are likely true variants. Also, seven polymorphisms were found in model HORVU.MOREX.r3.5HG0474460, Protein kinase, putative (G14A, W147R, H149S, S159L, V184A, F281L, and G757S). The same variants were identified in the pangenome genotype HOR13942. Nine polymorphisms were identified in gene HORVU.MOREX.r3.5HG0474880, Leguminosin group485 secreted peptide (Y25H, G26C, I46L, V63A, V64M, A68T, V90L, R138P, and E153A), all of them in common with pangenome genotype HOR13942. It is worth mentioning another gene (HORVU.MOREX.r3.5HG0471560, Receptor kinase), in which SBCC073 showed a missense polymorphism (F7L), although in this case, our results do not cover the complete sequence of the gene. The other parent of the population, SBCC146, had missense polymorphisms in another gene, HORVU.MOREX.r3.5HG0474810, Receptor-like protein kinase, which showed lower expression in roots than in other tissues. SBCC146 and SBCC073 differed in three polymorphisms (P204T, P277L, and A422V), and both genotypes carried the alternative allele in two other cases (S324P and N599T). Those five variants, identified in SBCC146, were all present in pangenome genotype Igri. Therefore, this gene is also a good candidate yielding in total five candidates.
4 Discussion
The results found in this experiment indicate a difference in assimilate partitioning in young seedlings of the RIL population between two Spanish landraces belonging to different genetic groups, which prevailed in areas with contrasting agro-climatic conditions. The parents follow a similar root growth trend as reported by Boudiar et al. (2020), with more vigorous root growth for SBCC073, but not for shoot growth, which was similar for the two genotypes. The differences in experimental set up and duration of the experiment are likely causing this discrepancy. Both experiments support that SBCC073 allocates more biomass to root tissue.
Is it more beneficial for a plant to invest more carbon resources to root tissue or to an aerial part at the first stage of development? During the first stages of development, crops need to be well established acquiring enough water and nutrients, and the root system is one of the main organs that requires carbon investment. On the other hand, plants need to produce energy through photosynthesis, so increasing shoots will derive into more photosynthetic tissue and a more vigorous canopy development. The difference found between these landraces actually bodes well with their geographic origin. SBCC073 is representative of a genetic group coming from dryer and warmer areas than those where the group of SBCC146 was established (Contreras-Moreira et al., 2019). We hypothesize that the presence of an allele favoring biomass allocation to roots in a barley from dry areas can be an adaptive trait. This hypothesis should be tested in more landrace materials of known origins. Studies comparing root system architecture at seedling and adult stages suggested that the root system architecture does not correlate well at both developmental phases (Maccaferri et al., 2016; Williams et al., 2022). This means that the root architecture traits observed at seedling stage are not expected to carry over at adult stage and that it is necessary to confirm the biomass partitioning trait detected in this population at adult stage.
Moreover, it will be interesting to test how this population modulates biomass allocation under drought stress, at seedling and adult stages, since plants are able to modify its phenotype in response to biotic and abiotic environmental signals (Poorter et al., 2012). A previous study (Boudiar et al., 2020) suggested that SBCC073 decreased RDW by 43% and SBCC146 by only 16%, under drought, in a 4-week experiment in rhizotrons.
A QTL for the root/shoot ratio was identified on chromosome 5H, with SBCC073 contributing the favorable allele. The QTL peak marker was at 66.6 cM (400.66 Mb), with a confidence interval ranging from 350.50 to 403.21 Mbp in the reference genome. This region is proximal to the one (between 68.78 and 320.04 Mbp on chromosome 5H) identified by Wonneberger et al. (2023) in modern European spring two-rowed barleys. The authors suggested that this haplotype probably originated from Northern Africa. Indeed, the six-rowed Spanish landrace SBCC073 mostly shared the RGT Planet haplotype in that region (132 identical SNPs out of 137, from 67.6 to 319.9 Mbp), but was different at the QTL region. Different genome-wide association studies have searched for QTL for root-to-shoot ratio in barley using plastic pots (Reinert et al., 2016), filter rolls (Abdel-Ghani et al., 2019), or germination pouches (Khodaeiaminjan et al., 2023). Those studies looked at biomass partitioning in plants older than ours, and identified QTL for this trait on chromosome 5H but distal to our QTL region (475–495 Mbp), pointing to a different region in the genome.
We searched for putative candidate genes in the region, focusing on those with differential expression between roots and shoots in other transcriptomic studies, and containing SNP polymorphisms in the coding region. Two of them, a UDP-glycosyltransferase (HORVU.MOREX.r3.5HG0473970), and a gene annotated as Leguminosin group485 secreted peptide (HORVU.MOREX.r3.5HG0474880) showed higher expression in root samples, and both of them accumulated several missense variants between the parents of the population. SBCC073 shared those variants with the pangenome genotype “HOR13942,” a landrace originating from Baeza, Southern Spain, close to the collection site of SBCC073. UDP-glycosyltransferases glycosylate small molecules such as phytohormones, secondary metabolites, and xenobiotics (Gharabli et al., 2023). A rice UDP-glycosyltransferase was identified as QTL regulating grain size by modulating cell proliferation and expansion indirectly affecting auxin levels (Dong et al., 2020). Cytokinin glucosyltransferases have also been identified as key regulators of cytokinin homeostasis, with potential value for wheat breeding (Chen et al., 2021). A gene annotated as Leguminosin group458 secreted protein was the most upregulated gene in barley leaves during the recovery phase after a drought treatment (Paul et al., 2023). Nevertheless, that gene is located in a different position on chromosome 5H (539.41 Mbp). We did not identify orthologs in Arabidopsis or rice, but a BLASTp of the transcript corresponding to the gene identified in this study had its best match [100% identity, expected value (E-value) of 3e−93] to a protein PELPK1-like from barley (XP_044947259.1). An Arabidopsis thaliana PELPK1 is a cell wall protein required for normal germination and growth (Rashid and Deyholos, 2011). Other gene annotated as Protein kinase, putative (HORVU.MOREX.r3.5HG0474460), an LRR receptor-like protein kinase, was highly expressed in root samples of different transcriptomic studies. Li et al. (2020), investigating the dynamics of C/N-nutrient-related phosphorylation signals in A. thaliana, identified related proteins. A Brassinosteroid-like receptor kinase (BRL1-like, HORVU.MOREX.r3.5HG0471560) was also included in the confidence interval of the QTL, although we only identified a tolerated missense variant in that gene. Therefore, we have less evidence in this case to be declared as a candidate gene, although it is still worth mentioning due to the relevance of the gene family. It has been shown that brassinosteroids affect barley root growth (Kartal et al., 2009), and a missense mutation in a BR receptor protein is associated with the semi-dwarf “uzu” mutation in barley (Chono et al., 2003). In rice, Nakamura et al. (2006) observed a high expression of the OsBRL1 and OsBRL3 genes in roots and suggested that they may be involved in BR perception in the roots. SBCC146 showed two missense variants in another gene, annotated as Receptor-like protein kinase (HORVU.MOREX.r3.5HG0474810), highly expressed in shoots. Receptor-like kinases are signaling proteins implicated in the regulation of development and stress responses (Morillo and Tax, 2006; Vij et al., 2008; Gish and Clark, 2011). Nevertheless, we cannot rule out that other genes in the interval are responsible for the trait. The results should be confirmed performing the experiment for a longer period validating the polymorphisms identified and testing gene expression of the candidate genes in this population.
We have identified genetic diversity for biomass partitioning between shoots and roots at the seedling stage in barley landraces coming from distinct agro-ecological regions, with potential adaptive meaning. The genetic control of partitioning seems to be a single gene, and we provide genomic evidence for five possible candidate genes that deserve further research, evaluating the performance of contrasting genotypes at seedling stage extending to the growth period, and at adult stage in the field.
Data availability statement
The exome capture dataset utilized in this study is available at the European Nucleotide Archive, accession PRJEB73755.
Author contributions
AC: Conceptualization, Data curation, Formal analysis, Investigation, Methodology, Visualization, Writing – original draft, Writing – review & editing. AMC: Conceptualization, Formal analysis, Funding acquisition, Supervision, Writing – original draft, Writing – review & editing. BL: Investigation, Methodology, Writing – review & editing, Writing – original draft. MC: Investigation, Methodology, Writing – review & editing, Writing – original draft. VM: Investigation, Methodology, Writing – review & editing, Writing – original draft. BC-M: Formal analysis, Visualization, Writing – review & editing, Writing – original draft. EI: Conceptualization, Formal analysis, Funding acquisition, Supervision, Writing – original draft, Writing – review & editing.
Funding
The author(s) declare financial support was received for the research, authorship, and/or publication of this article. Funding of this research was provided by project AGL2016-80967-R (MINECO/AEI) 10.13039/501100011033 and by “ERDF A way of making Europe,” project PID2019-111621RB-I00 (MCIN/AEI) 10.13039/501100011033, project PID2022-142116OB-I00 (MCIN/AEI) 10.13039/501100011033, and by “ERDF A way of making Europe,” grant A08_23R (Government of Aragón). Computing hardware was funded by grant FAS2022_052 from CSIC. AC was supported by the FPI grant BES-2017-082746 (MCIN/AEI) 10.13039/501100011033, and by “ESF investing in your future.”
Acknowledgments
The authors acknowledge the support of Francesc Montardit for bioinformatics analysis and of Antonio Pérez-Torres for support in the experimental set-up.
Conflict of interest
The authors declare that the research was conducted in the absence of any commercial or financial relationships that could be construed as a potential conflict of interest.
Publisher’s note
All claims expressed in this article are solely those of the authors and do not necessarily represent those of their affiliated organizations, or those of the publisher, the editors and the reviewers. Any product that may be evaluated in this article, or claim that may be made by its manufacturer, is not guaranteed or endorsed by the publisher.
Supplementary material
The Supplementary Material for this article can be found online at: https://www.frontiersin.org/articles/10.3389/fpls.2024.1408043/full#supplementary-material
References
Abdel-Ghani, A. H., Sharma, R., Wabila, C., Dhanagond, S., Owais, S. J., Duwayri, M. A., et al. (2019). Genome-wide association mapping in a diverse spring barley collection reveals the presence of QTL hotspots and candidate genes for root and shoot architecture traits at seedling stage. BMC Plant Biol. 19, 216. doi: 10.1186/s12870-019-1828-5
Aranda, I., Alía, R., Ortega, U., Dantas, ÂK., Majada, J. (2010). Intra-specific variability in biomass partitioning and carbon isotopic discrimination under moderate drought stress in seedlings from four Pinus pinaster populations. Tree Genet.Genomes. 6, 169–178. doi: 10.1007/s11295-009-0238-5
Arifuzzaman, M., Sayed, M. A., Muzammil, S., Pillen, K., Schumann, H., Naz, A. A., et al. (2014). Detection and validation of novel QTL for shoot and root traits in barley (Hordeum vulgare L.). Mol. Breed. 34, 1373–1387. doi: 10.1007/s11032-014-0122-3
Bertholdsson, N., Kolodinska Brantestam, A. (2009). A century of Nordic barley breeding—Effects on early vigour root and shoot growth, straw length, harvest index and grain weight. Eur. J. Agron. 30, 266–274. doi: 10.1016/j.eja.2008.12.003
Boudiar, R., Casas, A. M., Gioia, T., Fiorani, F., Nagel, K. A., Igartua, E. (2020). Effects of low water availability on root placement and shoot development in landraces and modern barley cultivars. Agronomy-Basel. 10, 134. doi: 10.3390/agronomy10010134
Butler, D. G., Cullis, B. R., Gilmour, A. R., Gogel, B. J., Thompson, R. (2017). ASReml-R reference manual version 4 (Hemel Hempstead, HP1 1ES, UK: VSN International Ltd).
Cantalapiedra, C. P., Boudiar, R., Casas, A. M., Igartua, E., Contreras-Moreira, B. (2015). BARLEYMAP: physical and genetic mapping of nucleotide sequences and annotation of surrounding loci in barley. Mol. Breed. 35, 13. doi: 10.1007/s11032-015-0253-1
Cantalapiedra, C. P., Contreras-Moreira, B., Silvar, C., Perovic, D., Ordon, F., Gracia, M. P., et al. (2016). A cluster of nucleotide-binding site–leucine-rich repeat genes resides in a barley powdery mildew resistance quantitative trait loci on 7HL. Plant Genome. 9, 1–14. doi: 10.3835/plantgenome2015.10.0101
Cantalapiedra, C. P., García-Pereira, M. J., Gracia, M. P., Igartua, E., Casas, A. M., Contreras-Moreira, B. (2017). Large differences in gene expression responses to drought and heat stress between elite barley cultivar scarlett and a spanish landrace. Front. Plant Sci. 8. doi: 10.3389/fpls.2017.00647
Casas, A. M., Contreras-Moreira, B., Cantalapiedra, C. P., Sakuma, S., Gracia, M. P., Moralejo, M., et al. (2018). Resequencing the Vrs1 gene in Spanish barley landraces revealed reversion of six-rowed to two-rowed spike. Mol. Breed 38, 51. doi: 10.1007/s11032-018-0816-z
Chen, L., Zhao, J., Song, J., Jameson, P. E. (2021). Cytokinin glucosyl transferases, key regulators of cytokinin homeostasis, have potential value for wheat improvement. Plant Biotechnol. J. 19, 878–896. doi: 10.1111/pbi.13595
Chono, M., Honda, I., Zeniya, H., Yoneyama, K., Saisho, D., Takeda, K., et al. (2003). A semidwarf phenotype of barley uzu results from a nucleotide substitution in the gene encoding a putative brassinosteroid receptor. Plant Physiol. 133, 1209–1219. doi: 10.1104/103.026195
Colmsee, C., Beier, S., Himmelbach, A., Schmutzer, T., Stein, N., Scholz, U., et al. (2015). BARLEX- the barley draft genome explorer. Mol. Plant 8, 964–966. doi: 10.1016/j.molp.2015.03.009
Contreras-Moreira, B., Naamati, G., Rosello, M., Allen, J. E., Hunt, S. E., Muffato, M., et al. (2022). Scripting analyses of genomes in ensembl plants. Methods Mol.Biol., 2443, 27–55. doi: 10.1007/978-1-0716-2067-0_2
Contreras-Moreira, B., Saraf, S., Naamati, G., Casas, A. M., Amberkar, S. S., Flicek, P., et al. (2023). GET_PANGENES: calling pangenes from plant genome alignments confirms presence-absence variation. Genome Biol. 24, 223. doi: 10.1186/s13059-023-03071-z
Contreras-Moreira, B., Serrano-Notivoli, R., Mohammed, N. E., Cantalapiedra, C. P., Beguería, S., Casas, A. M., et al. (2019). Genetic association with high-resolution climate data reveals selection footprints in the genomes of barley landraces across the Iberian Peninsula. Mol. Ecol. 28, 1994–2012. doi: 10.1111/mec.15009
Corcuera, L., Gil-Pelegrín, E., Notivol, E. (2012). Differences in hydraulic architecture between mesic and xeric Pinus pinaster populations at the seedling stage. Tree Physiol. 32, 1442–1457. doi: 10.1093/treephys/tps103
Dong, N., Sun, Y., Guo, T., Shi, C., Zhang, Y., Kan, Y., et al. (2020). UDP-glucosyltransferase regulates grain size and abiotic stress tolerance associated with metabolic flux redirection in rice. Nat. Commun. 11, 2629. doi: 10.1038/s41467-020-16403-5
Gharabli, H., Della Gala, V., Welner, D. H. (2023). The function of UDP-glycosyltransferases in plants and their possible use in crop protection. Biotechnol. Adv. 67, 108182. doi: 10.1016/j.bioteChadv.2023.108182
Ghosh, S., Watson, A., Gonzalez-Navarro, O. E., Ramirez-Gonzalez, R. H., Yanes, L., Mendoza-Suárez, M., et al. (2018). Speed breeding in growth chambers and glasshouses for crop breeding and model plant research. Nat. Protoc. 13, 2944–2963. doi: 10.1038/s41596-018-0072-z
Gish, L. A., Clark, S. E. (2011). The RLK/Pelle family of kinases. Plant J. 66, 117–127. doi: 10.1111/j.1365-313X.2011.04518.x
Jayakodi, M., Padmarasu, S., Haberer, G., Bonthala, V. S., Gundlach, H., Monat, C., et al. (2020). The barley pan-genome reveals the hidden legacy of mutation breeding. Nature. 588, 284–289. doi: 10.1038/S41586-020-2947-8
Kartal, G., Temel, A., Arican, E., Gozukirmizi, N. (2009). Effects of brassinosteroids on barley root growth, antioxidant system and cell division. Plant Growth Regul. 58, 261–267. doi: 10.1007/s10725-009-9374-z
Khodaeiaminjan, M., Knoch, D., Ndella Thiaw, M. R., Marchetti, C. F., Kořínková, N., Techer, A., et al. (2023). Genome-wide association study in two-row spring barley landraces identifies QTL associated with plantlets root system architecture traits in well-watered and osmotic stress conditions. Front. Plant Sci. 4. doi: 10.3389/fpls.2023.1125672
Li, J., Ji, L. (2005). Adjusting multiple testing in multilocus analyses using the eigenvalues of a correlation matrix. Heredity 95, 221–227. doi: 10.1038/sj.hdy.6800717
Li, T., Li, Y., Shangguan, H., Bian, J., Luo, R., Tian, Y., et al. (2023). BarleyExpDB: an integrative gene expression database for barley. BMC Plant Biol. 23, 170. doi: 10.1186/s12870-023-04193-z
Li, X., Sanagi, M., Lu, Y., Nomura, Y., Stolze, S. C., Yasuda, S., et al. (2020). Protein phosphorylation dynamics under carbon/nitrogen-nutrient stress and identification of a cell death-related receptor-like kinase in arabidopsis. Front.Plant Sci. 11. doi: 10.3389/fpls.2020.00377
Lombardi, E., Ferrio, J. P., Rodríguez-Robles, U., Resco de Dios, V., Voltas, J. (2021). Ground-Penetrating Radar as phenotyping tool for characterizing intraspecific variability in root traits of a widespread conifer. Plant Soil 468, 319–336. doi: 10.1007/s11104-021-05135-0
Luo, D., Ganesh, S., Koolaard, J., Luo, M. D. (2018). Package predictmeans (Comprehensive R Archive Network). Available online at: https://CRAN.R-project.org/package=predictmeans.
Maccaferri, M., El-Feki., W., Nazemi., G., Salvi., S., Cane., M. A., Colalongo, M. C., et al. (2016). Prioritizing quantitative trait loci for root system architecture in tetraploid wheat. J. Exp. Bot. 67, 1161–1178. doi: 10.1093/jxb/erw039
Mascher, M., Richmond, T. A., Gerhardt, D. J., Himmelbach, A., Clissold, L., Sampath, D., et al. (2013). Barley whole exome capture: a tool for genomic research in the genus Hordeum and beyond. Plant J. 76, 494–505. doi: 10.1111/tpj.12294
Mascher, M., Wicker, T., Jenkins, J., Plott, C., Lux, T., Koh, C. S., et al. (2021). Long-read sequence assembly: a technical evaluation in barley. Plant Cell. 33, 1888–1906. doi: 10.1093/plcell/koab077
Morillo, S. A., Tax, F. E. (2006). Functional analysis of receptor-like kinases in monocots and dicots. Curr. Opin. Plant Biol. 9, 460–469. doi: 10.1016/j.pbi.2006.07.009
Nagel, K. A., Putz, A., Gilmer, F., Heinz, K., Fischbach, A., Pfeifer, J., et al. (2012). GROWSCREEN-Rhizo is a novel phenotyping robot enabling simultaneous measurements of root and shoot growth for plants grown in soil-filled rhizotrons. Funct. Plant Biol. 39, 891–904. doi: 10.1071/FP12023
Nakamura, A., Fujioka, S., Sunohara, H., Kamiya, N., Hong, Z., Inukai, Y., et al. (2006). The role of osBRI1 and its homologous genes, osBRL1 and osBRL3, in rice. Plant Physiol. 140, 580–590. doi: 10.1104/105.072330
Paul, M., Tanskanen, J., Jääskeläinen, M., Chang, W., Dalal, A., Moshelion, M., et al. (2023). Drought and recovery in barley: key gene networks and retrotransposon response. Front.Plant Sci. 14. doi: 10.3389/fpls.2023.1193284
Piepho, H. P., Williams, E. R., Fleck, M. (2006). A note on the analysis of designed experiments with complex treatment structure. Hortscience. 41, 446–452. doi: 10.21273/HORTSCI.41.2.446
Poorter, H., Niklas, K. J., Reich, P. B., Oleksyn, J., Poot, P., Mommer, L. (2012). Biomass allocation to leaves, stems and roots: meta-analyses of interspecific variation and environmental control. New Phytol. 193, 30–50. doi: 10.1111/j.1469-8137.2011.03952.x
Rashid, A., Deyholos, M. K. (2011). PELPK1 (At5g09530) contains a unique pentapeptide repeat and is a positive regulator of germination in Arabidopsis thaliana. Plant Cell Rep. 30, 1735–1745. doi: 10.1007/s00299-011-1081-3
Reinert, S., Kortz, A., Léon, J., Naz, A. A. (2016). Genome-wide association mapping in the global diversity set reveals new QTL controlling root system and related shoot variation in barley. Front. Plant Sci. 7. doi: 10.3389/fpls.2016.01061
Siddiqui, M. N., Léon, J., Naz, A. A., Ballvora, A. (2021). Genetics and genomics of root system variation in adaptation to drought stress in cereal crops. J. Exp. Bot. 72, 1007–1019. doi: 10.1093/jxb/eraa487
Slafer, G. A., Foulkes, M. J., Reynolds, M. P., Murchie, E. H., Carmo-Silva, E., Flavell, R., et al. (2023). A ‘wiring diagram’ for sink strength traits impacting wheat yield potential. J. Exp. Bot. 74, 40–71. doi: 10.1093/jxb/erac410
Taiz, L., Zeiger, E., Møller, I. M., Murphy, A. (2015). Plant physiology and development Vol. 6 (Sunderland (USA: Sinauer Associates Incorporated), 761.
Vij, S., Giri, J., Dansana, P. K., Kapoor, S., Tyagi, A. K. (2008). The receptor-like cytoplasmic kinase (OsRLCK) gene family in rice: organization, phylogenetic relationship, and expression during development and stress. Mol.Plant 1, 732–750. doi: 10.1093/mp/ssn047
Voltas, J., Amigó, R., Shestakova, T. A., Matteo, G., Díaz, R., Zas, R. (2024). Phylogeography and climate shape the quantitative genetic landscape and range-wide plasticity of a prevalent conifer. Ecol. Monogr. 94, e1596. doi: 10.1002/ecm.1596
Voss-Fels, K. P., Robinson, H., Mudge, S. R., Richard, C., Newman, S., Wittkop, B., et al. (2018). VERNALIZATION1 modulates root system architecture in wheat and barley. Mol. Plant 11, 226–229. doi: 10.1016/j.molp.2017.10.005
Wang, J., Chen, Y., Zhang, Y., Zhang, Y., Ai, Y., Feng, Y., et al. (2021). Phenotyping and validation of root morphological traits in barley (Hordeum vulgare L.). Agronomy-Basel. 11, 1583. doi: 10.3390/agronomy11081583
Warnes, M. G. R., Bolker, B., Bonebakker, L., Gentleman, R., Huber, W., Liaw, A. (2016). Package gplots. Available online at: https://CRAN.R-project.org/package=gplots.
White, A. C., Rogers, A., Rees, M., Osborne, C. P. (2016). How can we make plants grow faster? A source–sink perspective on growth rate. J. Exp. Bot. 67, 31–45. doi: 10.1093/jxb/erv447
Williams, J. L., Sherman, J. D., Lamb, P., Cook, J., Lachowiec, J. A., Bourgault, M. (2022). Relationships between roots, the stay-green phenotype, and agronomic performance in barley and wheat grown in semi-arid conditions. Plant Phenome J. 5, e220050. doi: 10.1002/ppj2.20050
Wonneberger, R., Schreiber, M., Haaning, A., Muehlbauer, G. J., Waugh, R., Stein, N. (2023). Major chromosome 5H haplotype switch structures the European two-rowed spring barley germplasm of the past 190 years. Theor. Appl. Genet. 136, 174. doi: 10.1007/s00122-023-04418-7
Yahiaoui, S., Cuesta-Marcos, A., Gracia, M. P., Medina, B., Lasa, J. M., Casas, A. M., et al. (2014). Spanish barley landraces outperform modern cultivars at low-productivity sites. Plant Breed. 133, 218–226. doi: 10.1111/pbr.12148
Keywords: barley, seedling, biomass partitioning, carbon allocation, plant architecture
Citation: Cabeza A, Casas AM, Larruy B, Costar MA, Martínez V, Contreras-Moreira B and Igartua E (2024) Genetic control of root/shoot biomass partitioning in barley seedlings. Front. Plant Sci. 15:1408043. doi: 10.3389/fpls.2024.1408043
Received: 27 March 2024; Accepted: 13 November 2024;
Published: 02 December 2024.
Edited by:
Iker Aranjuelo, Institute of Agrobiotechnology, Spanish National Research Council (CSIC), SpainReviewed by:
Jordi Voltas, Universitat de Lleida, SpainSumeet Mankar, Donald Danforth Plant Science Center, United States
Copyright © 2024 Cabeza, Casas, Larruy, Costar, Martínez, Contreras-Moreira and Igartua. This is an open-access article distributed under the terms of the Creative Commons Attribution License (CC BY). The use, distribution or reproduction in other forums is permitted, provided the original author(s) and the copyright owner(s) are credited and that the original publication in this journal is cited, in accordance with accepted academic practice. No use, distribution or reproduction is permitted which does not comply with these terms.
*Correspondence: Ana M. Casas, YWNhc2FzQGVlYWQuY3NpYy5lcw==