- 1Department of Agricultural, Food and Environmental Sciences, University of Perugia, Perugia, Italy
- 2TeamDev – Software, GIS and Web Engineering, Perugia, Italy
- 3O.P.O.O., Perugia, Italy
- 4Center of Plant Sciences, Scuola Superiore Sant’Anna, Pisa, Italy
Agroclimatic variables may affect insect and plant phenology, with unpredictable effects on pest populations and crop losses. Bactrocera oleae Rossi (Diptera: Tephritidae) is a specific pest of Olea europaea plants that can cause annual economic losses of more than one billion US dollars in the Mediterranean region. In this study, we aimed at understanding the effect of olive tree phenology and other agroclimatic variables on B. oleae infestation dynamics in the Umbria region (Central Italy). Analyses were carried out on B. oleae infestation data collected in 79 olive groves during a 7-year period (from 2015 to 2021). In July–August, B. oleae infestation (1% attack) was negatively affected by altitude and spring mean daily temperatures and positively by higher winter mean daily temperatures and olive tree cumulative degree days. In September–October, infestation was negatively affected by a positive soil water balance and high spring temperatures. High altitude and cumulative plant degree days were related to delayed attacks. In contrast, high winter and spring temperatures accelerated them. Our results could be helpful for the development of predictive models and for increasing the reliability of decision support systems currently used in olive orchards.
Introduction
Olea europaea L. is one of the oldest and most abundant tree crops in the Mediterranean regions, where it is of essential socioeconomic and ecological importance (Michalopoulos et al., 2020; Caselli and Petacchi, 2021). Approximately 95% of the global demand for olive oil is satisfied by southern European countries, with Spain, Italy, and Greece being the main olive oil producers (Fraga et al., 2021). Given the importance of olive cultivation, it is imperative to effectively manage pests that can cause significant production losses. More than 255 species, including insect pests, mites, nematodes, and pathogenic microorganisms, are potentially harmful to O. europaea (Caselli and Petacchi, 2021). Most of the yield loss is caused by the key pest Bactrocera oleae (Rossi) (Diptera: Tephritidae). In addition, moths [e.g., Prays oleae (Bernard)] contribute locally or occasionally to yield decline (Caselli and Petacchi, 2021). Insects can also be pathogen vectors, such as Philaenus spumarius L., the primary vector of Xylella fastidiosa subsp. pauca, which is responsible for the olive quick decline syndrome (Elbeaino et al., 2014; Sevarika et al., 2022). In a scenario of climate change, the ability to predict the evolution of the cycle of pests and plants, assessing the risks associated with their interaction, represents a critical challenge for the implementation of a proper control strategy.
Herbivorous insects, especially those with low thermal thresholds, are highly sensitive to changes in climate (Deutsch et al., 2008). Climate change may alter plant–pest phenological events, such as flowering and leaf unfolding, insect overwintering, and migration (Gordo and Sanz, 2005). Depending on the insect species, higher mean daily temperatures and extreme climate events, no longer sporadic, could cause the extension of suitable geographical ranges for herbivores but also the disruption of the synchronization of biological cycles among herbivores and their natural enemies (Forrest, 2016; Bonsignore et al., 2020; Skendžić et al., 2021), with an expected substantial increase in crop losses (Deutsch et al., 2018). As a result, monitoring methods and pest management programs must be reviewed and, if necessary, adapted to the new climatic changes that are occurring. In recent decades, pest control relied mainly on the use of broad-spectrum insecticides, with negative effects related to the decline of natural enemies, the occurrence of insecticide resistance in the target population, environmental pollution, and human health (Damos et al., 2015). Integrated pest management (IPM) is now commonly adopted, foreseeing the combined use of synthetic insecticides with more sustainable methods (Stetter and Lieb, 2000). The use of successful prediction models can play a pivotal role within IPM, also in combination with machine learning algorithms, used to manage complex datasets (McQueen et al., 1995; Damos, 2015; Raza et al., 2015; Benos et al., 2021; Caselli and Petacchi, 2021; Skendžić et al., 2021). Degree day models are a conventional instrument for predicting insect phenology (AliNiazee, 1979; Jones et al., 1991; Song et al., 2003; Rebaudo and Rabhi, 2018; Barker et al., 2020). However, physiologically based population modeling merges information on insect development and crop phenology to achieve more accurate predictions (Gutierrez et al., 2009; Rossini et al., 2022). Since a precise indication of pest outbreaks is necessary in an IPM context, machine learning has been applied in decision support systems (Capalbo et al., 2017; Ip et al., 2018; Rossini et al., 2022). A decision support system is a set of computer programs, mathematical models, and heuristic information that operate synergistically to improve decision-making (Nestel et al., 2019).
The olive fruit fly, B. oleae is an important pest of Olea spp. in Europe, Asia, Africa, and North America (Varikou, 2022). Bactrocera oleae is expected to expand due to global warming, thus colonizing areas at higher latitudes and altitudes (Petacchi et al., 2015; Marchi et al., 2016). Furthermore, an increase in average temperatures can affect the adult phenology in spring or can halt egg development in summer, but, on the other side, it can prolong the oviposition period in autumn, possibly resulting in increased yield losses (Caselli and Petacchi, 2021). The economic damage of B. oleae is estimated at more than one billion US dollars per year, only in the Mediterranean region (Van Asch et al., 2015).
The larval stage is responsible for both qualitative and quantitative damage due to its feeding activity within the olive mesocarp, leading to a strong decline in oil quality and premature fruit drop (Gömez-Caravaca et al., 2008). IPM programs against B. oleae are primarily based on monitoring of adults, sampling of olives to evaluate active infestation, and eventually pesticide treatments. In recent years, understanding the population dynamics of B. oleae has become a major focus of research on this pest. Since dimethoate use has been banned due to its toxic effects (Commission Implementing Regulation (EU), 2019/1090), alternative prevention-based control strategies are now recommended. Olive orchard monitoring is required due to the numerous factors that affect B. oleae infestations such as temperature, weather conditions, geographical location, olive tree variety, and management practices (Wang et al., 2009, 2013; Johnson et al., 2011; Rizzo et al., 2012; Petacchi et al., 2015; Volpi et al., 2020). Moreover, the olive variety can determine the fruit susceptibility to B. oleae attacks. Preferences are based on factors such as the size and shape of the fruits and their concentration of phenolic compounds (Varikou et al., 2022; González-Fernández et al., 2023). Furthermore, the mineral element content of the fruit may also influence female choice, making fruits that contain higher amounts of K and Fe more susceptible to attacks (Garantonakis et al., 2016). Concerning the use of digital tools, so far, some predictive models, machine learning algorithms, and decision support systems have been used for B. oleae monitoring and control (Ordano et al., 2015; Petacchi et al., 2015; Marchi et al., 2016; Miranda et al., 2019; Benhadi-Marín et al., 2020; Volpi et al., 2020; González-Fernández et al., 2023). However, proper calibration of these tools for the Umbria region (Central Italy) is lacking. To fill the knowledge gap about the effect of climate and environment on B. oleae in the Umbria region, we analyzed the dynamics of B. oleae infestation over 7 years (from 2015 to 2021) in 79 olive groves in total.
Material and methods
Bactrocera oleae infestation data
Analyses were conducted on B. oleae infestation data with the collaboration of the Umbrian Olive Oil Producer Association (O.P.O.O.) operating in the Umbria region (Central Italy). The dataset accessed contains monitoring data collected from 2015 to 2021 by expert field technicians. Surveys included a total of 79 olive orchards. Fruits were sampled weekly from the second half of July (pit hardening) until harvest. Each sample consisted of 100 olives randomly collected from different plants (one fruit per plant) (Quaglia et al., 1982). The olives were visually inspected in the laboratory with a stereomicroscope. Healthy olives were separated from those with oviposition punctures (Daher et al., 2022). Fruits exhibiting oviposition punctures were dissected with a scalpel and observed under a stereomicroscope to assess the presence of B. oleae. Alive eggs and larvae were considered for calculating the active infestation index (calculated as in Delrio and Prota, 1977 and Tsolakis et al., 2011).
Variables associated with Bactrocera oleae infestation
Several environmental, morphometric, and weather variables have been correlated, over time, to the olive fruit fly infestations, such as precipitations, altitude, elevation, and distance from the water (Torres-Villa et al., 2006; Volpi et al., 2020). Based on these studies, we identified a set of candidate explanatory variables related to weather (average daily air temperature, daily precipitation), morphometric (altitude, slope, exposition), and environmental (distance from lakes) parameters. Weather data used were obtained from the regional monitoring network—Regional Hydrographic Service (https://annali.regione.umbria.it/#). The monitoring network consists of sensors that send data in real time to the central station through radio links distributed throughout the territory, which manages the peripherals and stores the data. Daily observed data on air temperature and precipitation from 116 meteorological stations for the period 2014–2021 have been accessed and processed. Data processing included mapping of weather stations, time series charting and analysis, evaluation and removal of records with missing values, and evaluation and removal of records with outlier values. Weather data were georeferenced on the respective sensors’ positions. The point data were then used to interpolate and create weather surfaces. To create a surface of predicted temperature values for the region using the sample data, the Geostatistical Wizard in ArcGIS Pro was used (Apaydin et al., 2004; Kim et al., 2010; Merbitz et al., 2012; Antal et al., 2021; Katipoğlu, 2022). Geostatistical techniques quantify the spatial autocorrelation among measured points and account for the spatial configuration of the sample points around the prediction location. The inverse distance weighted (IDW) method was used to create the daily interpolated surfaces for the weather parameters over the 7-year period. The meteorological surfaces produced by the model were used to calculate the daily maximum, minimum, and average air temperature (°C) and daily precipitation (mm) for each monitoring record at its specific position. Those parameters relevant to model selection are reported in Table 1.
Calculation of agrometeorological variables
Weather data and weather surfaces have been used to calculate a set of variables, aiming at the identification and description of climatic drivers influencing B. oleae infestation. The agrometeorological variables were selected according to the annual cycle of B. oleae (Koveos, 2001) and based on available methodology (Volpi et al., 2020). The bioclimatic variables identified (Table 1) refer to three periods: 1) winter period, calculated for each year in the period November (of the previous year)–February; 2) spring period, calculated for each year in the period March–May; and 3) summer period, calculated from the beginning of June to the day of the attack, or calculated in the 7 days prior to the day of the attack. Another variable was chosen to consider the soil water balance and, therefore, refers to the water status of the olive grove, calculated in the 30 days before the day of the attack. The soil water balance was calculated as proposed by Hargreaves and Samani (1985) and adopted in Volpi et al. (2020). The cumulative degree days were calculated using the package “TrenchR” in the R environment (R Core Team, 2022; Buckley et al., 2023). Olive tree phenology was considered by calculating the cumulative degree day from January, with a lower threshold of 5°C [CDD (PLANT)] (Volpi et al., 2020). Other variables were originally calculated and considered, the cumulative degree day with a lower threshold of 8.99°C and an upper threshold of 30°C [CDD (INSECT)] (according to thresholds in Crovetti et al., 1982; Gonçalves and Torres, 2011), or the cumulative precipitation (in mm) during summer (RAIN) (Volpi et al., 2020), but they were not included in the model selection because of high correlation (Spearman) with other variables [e.g., CDD (INSECT) and CDD (PLANT)].
Morphometric data processing
The Euclidean distance from lakes or rivers and morphometric parameters (e.g., altitude) were processed in ArcGIS Pro and then extracted from the raster file for each monitoring record by using an automation model built with Model Builder function (Bajjali, 2023). Digital elevation models (DEMs) were obtained by TINITALY/01 (https://tinitaly.pi.ingv.it/), which is currently considered the most accurate DEM covering the whole Italian territory (Tarquini et al., 2007). TINITALY/01 is a DEM in triangular irregular network format created for the entire Italian territory in the UTM 32 WGS 84 coordinate system (Tarquini et al., 2007). The whole TINITALY/01 DEM was converted in grid format (10-m cell size) according to a tiled structure composed of 193.50-km side square elements (Mascandola et al., 2021). DEM coordinates were assigned as WGS 1984 UTM Zone 32N (WKID 32632).
Statistical analysis
Bactrocera oleae infestation was evaluated as the occurrence of active infestation at 1% threshold and as the Julian day of occurrence of the first attacks. Each dependent variable was analyzed in two different periods, that is, July–August (i.e., early season) and September–October (i.e., late season) of each year from 2015 to 2021. The initial explanatory variables considered were DEM, LAKES, TEMP (MAR–MAY), TEMP (NOV–FEB), CDD (PLANT), TEMP (−7D), and SWB (Table 1). Considered orchards were monovarietal or mixed olive cultivars. To evaluate the effect of cultivars, we have tested the effect of a four-level categorical variable (cultivar, CV). Three levels were Leccino, Frantoio, and Moraiolo varieties. A fourth level grouped mostly olive plantations with mixed cultivars or with cultivars marginally represented in the dataset (e.g., “Nostrale di Rigali”). Agronomic management has a variable effect on B. oleae attacks (Gkisakis et al., 2018). A dummy variable (management, MAN) was included to compare the two management systems applied in olive orchards, i.e., IPM vs. organic. However, neither CV nor MAN variables revealed a significant effect on B. oleae occurrence of active infestation and Julian day of occurrence of first attacks; hence, they were not retained in the final models (see Supplementary Tables 1, 2 for statistical results). Similarly, the Euclidean distance from the rivers was initially evaluated, but its effect on B. oleae attacks was never significant. All variables were standardized (mean-centered with a unit standard deviation) prior to analysis. Attack probability was initially evaluated by means of generalized mixed-effects models (with logit link and binomial distribution) to account for dependent observations (multiple observations on the same orchard across different years) (Supplementary Tables 1, 2). The Julian day of occurrence of the first attacks was evaluated by means of linear mixed-effects models (Burnham and Anderson, 2002; Rondoni et al., 2012), excluding from the final analysis the data from 2017, due to the limited number of infestation outbreaks. For both types of models, the relevance of the fixed and random structure was evaluated by means of the likelihood ratio test (LRT) and Akaike information criteria (AIC) (Pinheiro and Bates, 2006; Jacobs et al., 2022; Tidau et al., 2023). The best-fitted models, i.e., retaining the minimum number of explanatory variables, were selected using LRT (Burnham and Anderson, 2002; Ferracini et al., 2023). The multicollinearity of variables was assessed through the calculation of the variance inflation factor and revealed low (Zuur et al., 2009). A residual plot was evaluated for each of the best-fitted models. Data were analyzed and visualized using “MASS” (Venables and Ripley, 2002), “nlme” (Pinheiro and Bates, 2006), “lme4” (Bates et al., 2015), “ciTools” (Haman and Avery, 2020), “ggplot2” (Wickham, 2016), and “ggeffects” (Lüdecke, 2018) packages in R (version 4.2.2) (R Core Team, 2022).
Results
The best model to explain the probability of attack in July–August retained five explanatory variables. Attack was negatively affected by DEM and TEMP (MAR–MAY), but positively affected by TEMP (NOV–FEB), CDD (PLANT), and SWB (the results of the best-fitted generalized mixed-effect model are reported in Table 2; Figure 1). Concerning the attacks in September–October, these were negatively affected by the increase of SWB and TEMP (MAR–MAY) but positively by TEMP (NOV–FEB) and TEMP (−7D) (Table 3; Figure 1). Concerning the day of the first attacks (Table 4), the increase in CDD (PLANT), DEM, and SWB delayed the occurrence of the attacks in the July–August period. On the contrary, an increase in LAKES, TEMP (NOV–FEB), TEMP (MAR–MAY), and TEMP (−7D) had a positive effect in anticipating the attacks. For the September–October period, higher values of DEM and CDD (PLANT) delayed the attacks, while an increase in TEMP (MAR–MAY) and TEMP (−7D) anticipated the attacks by B. oleae (Table 5).
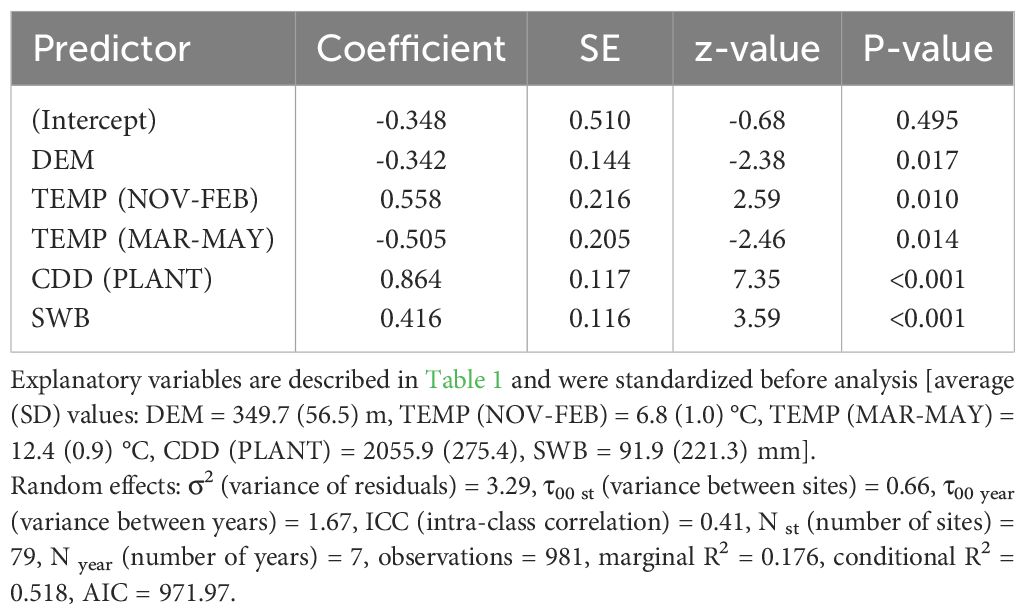
Table 2 Coefficients and significance level for each explanatory variable retained within the best fitted model (Generalized linear mixed-effects model, binomial distribution) for the probability of occurrence of the first active infestation (1% threshold) within the period July-August of all years from 2015 to 2021.
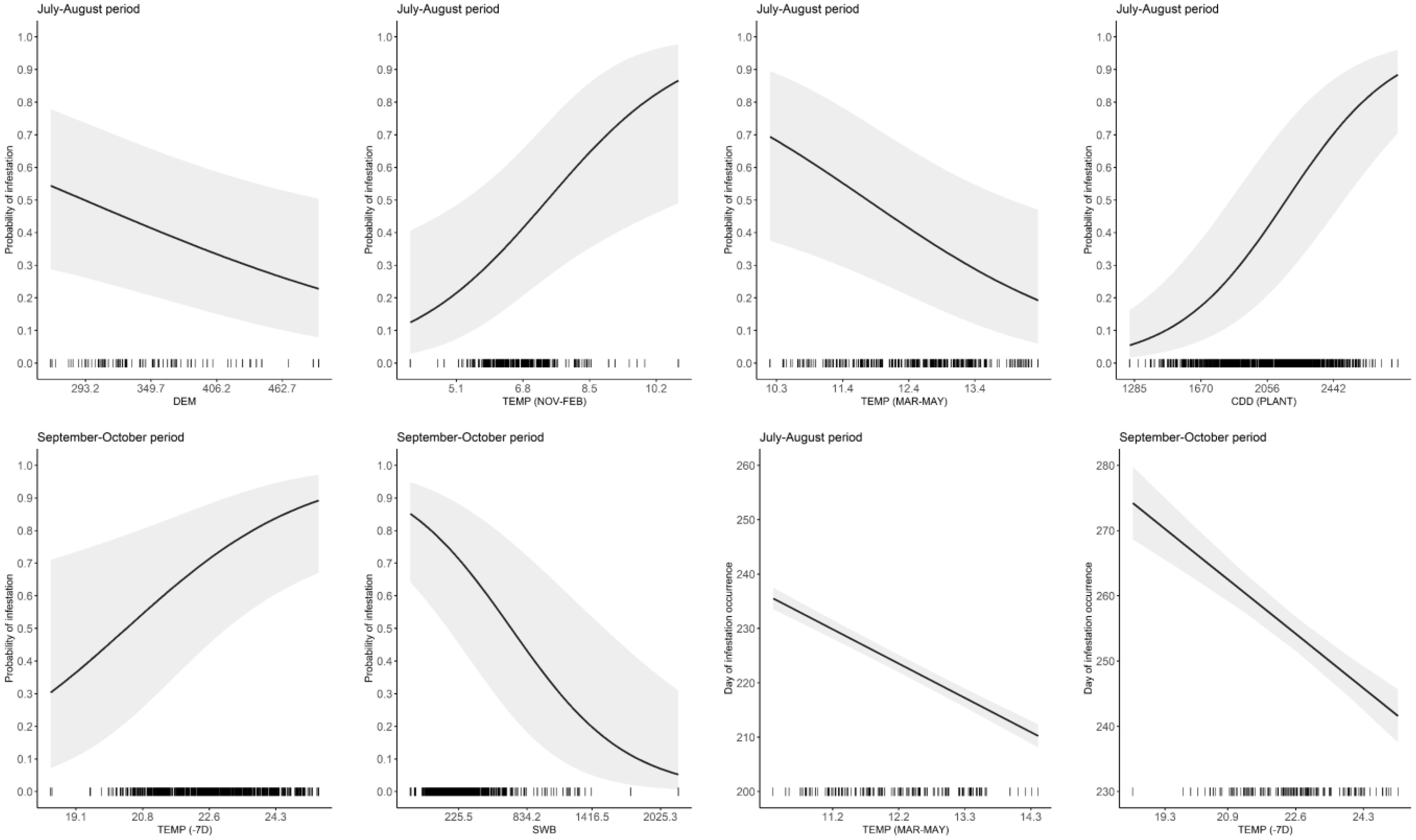
Figure 1 Relationships between infestation probability or Julian day of occurrence of Bactrocera oleae and some of the variables retained in the best-fitted models of Tables 2–5. Curves represent the model estimate (solid line) and 95% confidence intervals (shaded area). For each plot, the variables not represented were set to their average value on the original scale (reported in the caption of Tables 2–5). Tick symbols represent the distribution of the original data. Explanatory variables are described in Table 1.
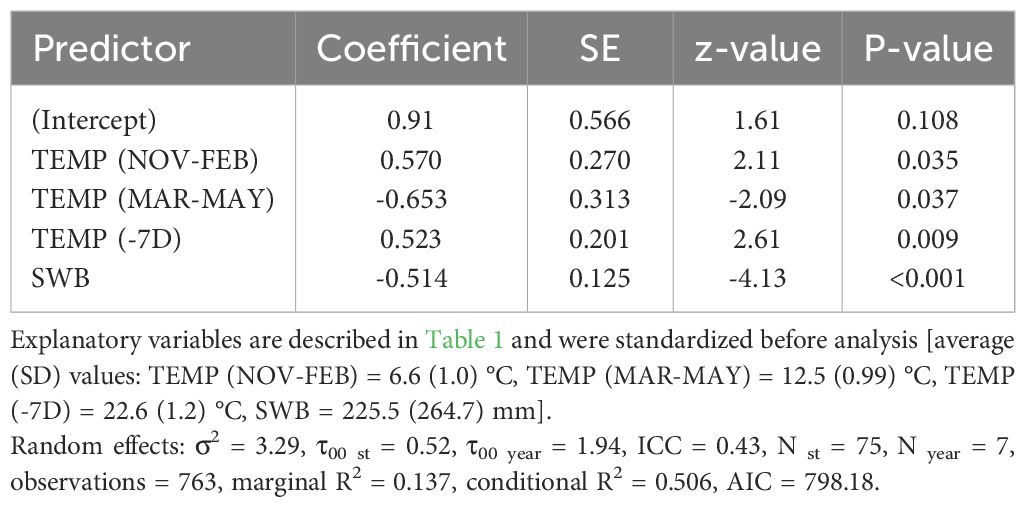
Table 3 Coefficients and significance level for each explanatory variable retained within the best fitted model (Generalized linear mixed-effects model, binomial distribution) for the probability of occurrence of the first active infestation (1% threshold) within the period September-October of all years from 2015 to 2021.
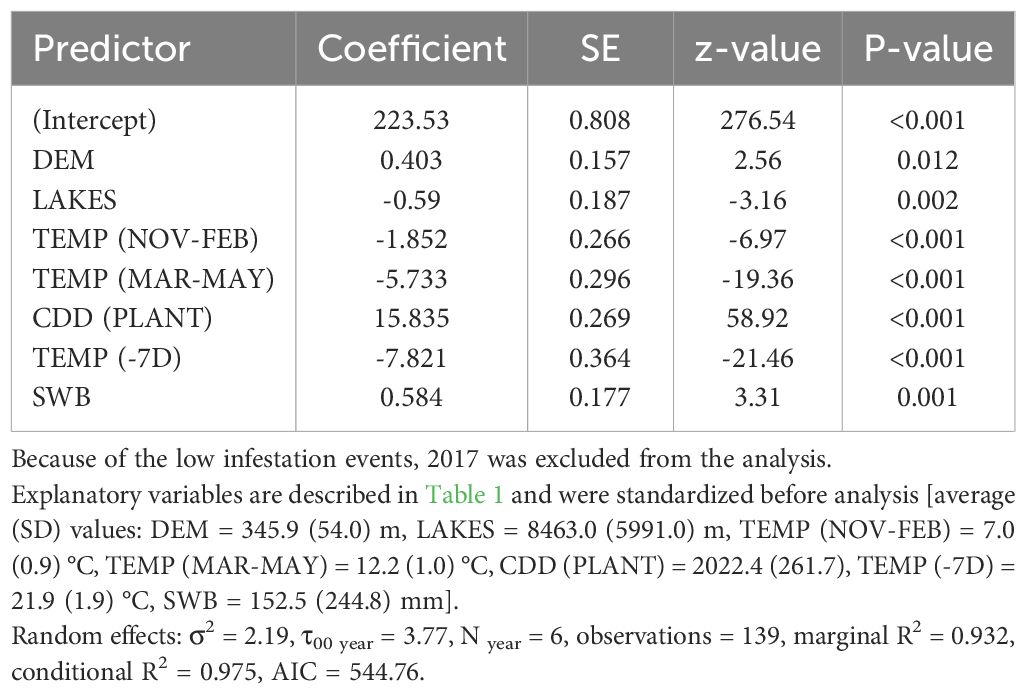
Table 4 Coefficients and significance level for each explanatory variable retained within the best fitted model (Linear mixed-effects model) for Julian day of the occurrence of the first active infestation (1% threshold) within the period July-August in the period 2015 to 2021.
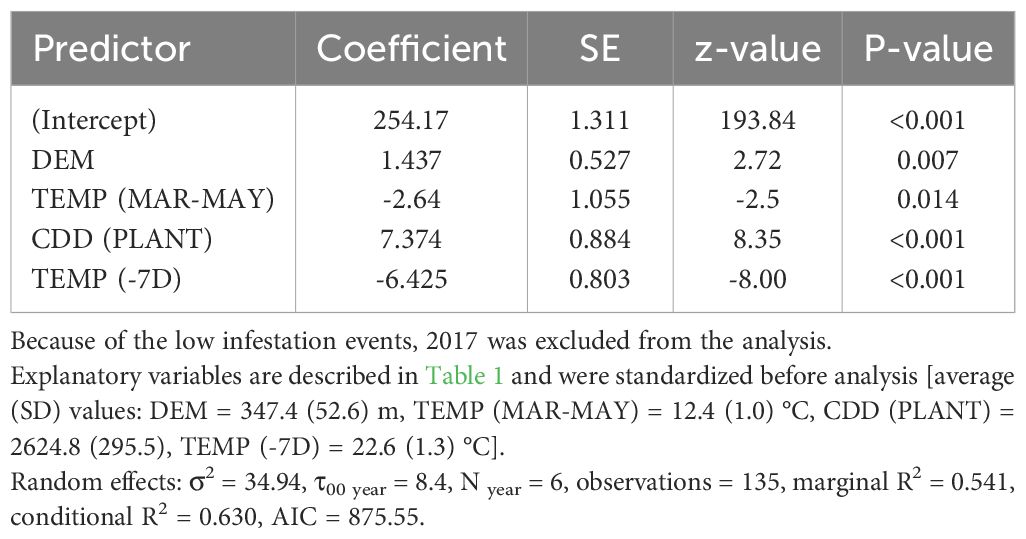
Table 5 Coefficients and significance level for each explanatory variable retained within the best fitted model (Linear mixed-effects model) for Julian day of the occurrence of the first active infestation (1% threshold) within the period September-October in the period 2015 to 2021.
Discussion
This study represents the first attempt to understand B. oleae population dynamics in Umbria (Central Italy) using a combination of landscape and agroclimatic variables, which could be further leveraged in studies related to larger territories with similar geographical characteristics. The assessment of B. oleae infestation was carried out over two crucial periods of the year, that is, in July–August, during the fruit growth period, and in September–October, during the preharvest period.
Our analysis reveals that olive orchards located at higher altitude expected lower attacks during summer (July–August period). In these sites, attacks are also delayed during the year (July–August and September–October periods). Similarly, Helvaci et al. (2018) reported that the infestation rate was inversely related to both altitude and relative humidity in olive orchards of Northern Cyprus. Furthermore, temperatures in winter and spring have a strong effect on determining attacks and timing of infestation. Average daily temperatures were approximately 7°C in November–February, i.e., when B. oleae is mostly overwintering, and determined an attack probability of approximately 50% (averaged across years). Temperature increase in this period has a positive effect in enhancing future attacks throughout the year, e.g., with an increase in the early season of ca. 35% probability of attacks at 8.5°C average temperatures. Our analysis suggests that temperatures also anticipate the timing of attacks during the early season period of approximately 2 d per degree. Insects that overwinter as pupa in soil have increased survival when temperature increases above 1°C (Bale and Hayward, 2010). Winter temperatures above 0°C gradually reduce the mortality of B. oleae, which can successfully overwinter with large populations (Hatherly et al., 2005; Wang et al., 2013; Petacchi et al., 2015; Marchi et al., 2016). Similarly, Marchi et al. (2016) registered an anticipation of B. oleae appearance in spring and higher infestation rates of juvenile forms (i.e., eggs, first and second instar larvae alive and dead) during the early season period (July to August) in years characterized by mild winters. Helvaci et al. (2018) detected a higher infestation rate as a consequence of higher winter air temperatures.
The increase of the average temperatures in the March–May period negatively affects attack probability but remarkably anticipates the occurrence of first attacks. Given the massive influence of temperature on insects and the fact that higher temperatures often lead to shorter life spans, the combination of a mild winter and a hot spring could potentially be responsible for the premature decline of overwintering adults (Preu et al., 2020), possibly resulting in a lower attack probability. In non-irrigated orchards, such as those investigated here, warmer spring temperatures may also negatively affect pupal survival, whereas irrigation may prevent pupal desiccation (reviewed by Yokoyama, 2015). In addition, during spring, most of the newly emerged B. oleae adults disperse from olive orchards, where fruits are not available, to seek flowers and nectar for survival (Paredes et al., 2023). During these migratory flights, newly hatched adults can also encounter abandoned olive groves, where they can find fruits from the previous year to oviposit. In this scenario, the reduced infestation found in the surveyed olive groves might be due to the higher spring temperatures followed by an increased frequency and distance of migratory flights (Economopoulos et al., 1978; Mazomenos et al., 2002; Ragaglini et al., 2007; Skouras et al., 2007; Marchini et al., 2017; Ortega et al., 2022). The part of the population that did not migrate, on the other hand, may have been responsible for the early attacks recorded.
More hypotheses could be drawn for the observed negative effect of increased spring temperatures on attacks and could, for example, consider the modification of the chemical and physical factors involved in the susceptibility of olives to B. oleae (Tognetti et al., 2006; Malheiro et al., 2015).
The accumulation of degree days relevant to the olive phenology positively determined B. oleae attacks but only for the July–August period, confirming the results of previous studies conducted in other Italian regions (Marchi et al., 2016; Volpi et al., 2020).
Notably, higher temperatures in the 7-day period before the monitoring day increase the attack probability only in the late season and anticipate the occurrence of attacks in both early and late seasons. Other variables rather than temperature may affect the interactions between olive fruit fly and its host, such as soil water content and irrigation type. For example, we detected that an increase in the soil water content increases the attack probability by B. oleae. A low water level can lead to loss of plant turgor and premature fruit drop. Conversely, adequate water supply improves fruit turgidity, which elicits higher B. oleae attacks on fruits (Marchi et al., 2016). Irrigation practice was not considered within the model selection because in Central Italy productive olive plantations are not irrigated. Vicinity to lakes is known to increase the attack probability by B. oleae because of the peculiar microclimate caused by lakes (Gutierrez et al., 2009). However, in our analysis, proximity to lakes was of marginal importance and never affected the probability of attacks. An explanation is that a possible variability in the attacks in orchards gradually distant from lakes could have been captured by other variables considered (e.g., winter and spring temperatures).
Collectively, our results support a potential application of IPM control strategies leveraging predictive models in the future implementation of decision support systems. The main bottleneck of this application is the evaluation of false negatives that can underestimate the infestation rates in olive orchards (Volpi et al., 2020). For these reasons, different interpolation methods must be tested before choosing the best-fit model for a specific geographic and climatic area (Petacchi et al., 2015). In the case of B. oleae, adequate monitoring networks are needed to implement a proper program, as insect distribution is affected by environmental and landscape factors (Thies et al., 2003; Krasnov et al., 2019). Despite the difficulties of precision agriculture strategy for pest control, there are previous studies confirming the successful implementation of digital tools (Miranda et al., 2019). Sciarretta et al. (2019) reported a substantial reduction in the number and volume of pesticide applications, for the control of the Mediterranean fruit fly (medfly), Ceratitis capitata (Wiedermann), in areas managed with a dedicated decision support system and electronic monitoring traps. Given the close relationship between insect development and temperature, further studies on the impact of temperature variations on the spatial and temporal distribution of B. oleae populations are urgently needed. In addition to air temperature, the emergence of B. oleae can be affected by soil temperature and moisture. For example, low soil temperature and high soil moisture due to rain can increase B. oleae pupal mortality (Neuenschwander et al., 1981; Wang et al., 2013). Conversely, low soil humidity may be responsible for pupal desiccation, drastically reducing adult emergence in the spring (Wang et al., 2013; Yokoyama, 2015). The use of specific sensors to measure soil parameters would be necessary to further elucidate the effect of soil temperature and moisture on the overwintering population of B. oleae.
Moreover, traditional control methods (i.e., cover and bait sprays) based on conventional insecticides could become inefficient if the olive fruit fly continues to increase its resistance (Skouras et al., 2007; Pereira-Castro et al., 2015; Marchi et al., 2016). Alternative approaches, by combining powder dust or kaolin with propolis, exhibited an interesting and concrete possibility to reduce fruit infestations by B. oleae (Daher et al., 2022). An integration of different olive fruit fly management methods is recommended, including the use of chemical, biotechnical, and biological control (Lantero et al., 2023). The complexity of the landscape surrounding olive groves has been shown to reduce the abundance of B. oleae and other insect pests (Ortega et al., 2018; Villa et al., 2020). In addition, a more complex environment has been found to enhance the effectiveness of biocontrol agents (Boccaccio and Petacchi, 2009; Ortega et al., 2018). Therefore, agricultural spatial planning should consider the impact of landscape complexity on insect development. Our analysis allowed the identification of key environmental variables influencing the probability and timing of B. oleae attacks that could be used in distribution modeling at the regional scale, or even in more complex modeling methods, based on machine learning algorithms. Further analyses should also evaluate the effect of landscape complexity and composition on olive fruit fly infestations occurring in Central Italy.
Data availability statement
Data will be provided upon request directed to GR (Z2FicmllbGUucm9uZG9uaUB1bmlwZy5pdA==).
Ethics statement
The manuscript presents research on animals that do not require ethical approval for their study.
Author contributions
GR: Conceptualization, Formal analysis, Methodology, Writing – original draft, Writing – review & editing, Supervision. EM: Methodology, Writing – original draft, Writing – review & editing, Conceptualization, Formal analysis. VAG: Methodology, Writing – original draft, Writing – review & editing. ElC: Methodology, Writing – review & editing, Writing – original draft. AB: Conceptualization, Investigation, Methodology, Writing – review & editing, Supervision. GN: Writing – review & editing, Conceptualization, Investigation, Methodology. RP: Writing – review & editing. FF: Conceptualization, Supervision, Writing – review & editing. AN: Conceptualization, Methodology, Supervision, Writing – review & editing. ErC: Conceptualization, Methodology, Supervision, Writing – review & editing.
Funding
The author(s) declare financial support was received for the research, authorship, and/or publication of this article. This research was partially funded by PRIMA “Innovative farm strategies that integrate sustainable N fertilization, water management and pest control to reduce water and soil pollution and salinization in the Mediterranean-Safe-H2O-Farm” (PRIMA22_00023 and J63C23000090005 to ECo and FF). GR was funded by H2020-MSCA-GF– PESTNET, Grant agreement ID: 101026399.
Acknowledgments
The authors wish to thank Virna Cairoli, Alessandro Mazzetti, and Lucia Mazzetti for their help with data collection and Dr. Andrea Pascucci from the Umbria Regional Hydrographic Service for providing meteorological data.
Conflict of interest
Authors EM and AN were employed by the company TeamDev – Software, GIS and Web Engineering. Authors AB and GN were employed by the company O.P.O.O.
The remaining authors declare that the research was conducted in the absence of any commercial or financial relationships that could be construed as a potential conflict of interest.
The author(s) declared that they were an editorial board member of Frontiers, at the time of submission. This had no impact on the peer review process and the final decision.
Publisher’s note
All claims expressed in this article are solely those of the authors and do not necessarily represent those of their affiliated organizations, or those of the publisher, the editors and the reviewers. Any product that may be evaluated in this article, or claim that may be made by its manufacturer, is not guaranteed or endorsed by the publisher.
Supplementary material
The Supplementary Material for this article can be found online at: https://www.frontiersin.org/articles/10.3389/fpls.2024.1401669/full#supplementary-material
References
AliNiazee, M. T. (1979). A computerized phenology model for predicting biological events of Rhagoletis indifferens (Diptera: Tephritidae). Can. Ent. 111, 1101–1109. doi: 10.4039/Ent1111101-10
Antal, A., Guerreiro, P. M. P., Cheval, S. (2021). Comparison of spatial interpolation methods for estimating the precipitation distribution in Portugal. Theor. Appl. Climatol. 145, 1193–1206. doi: 10.1007/s00704-021-03675-0
Apaydin, H., Kemal Sonmez, F., Yildirim, Y. E. (2004). Spatial interpolation techniques for climate data in the GAP region in Turkey. Clim. Res. 28, 31–40. doi: 10.3354/cr028031
Bajjali, W. (2023). “Working with arcGIS pro,” in ArcGIS Pro and ArcGIS Online: Applications in Water and Environmental Sciences (Springer International Publishing, Cham), 15–33. doi: 10.1007/978–3-031–42227-0_2
Bale, J. S., Hayward, S. A. L. (2010). Insect overwintering in a changing climate. J. Exp. Biol. 213, 980–994. doi: 10.1242/jeb.037911
Barker, B. S., Coop, L., Wepprich, T., Grevstad, F., Cook, G. (2020). DDRP: real-time phenology and climatic suitability modeling of invasive insects. PloS One 15, e0244005. doi: 10.1371/journal.pone.0244005
Bates, D., Mächler, M., Bolker, B., Walker, S. (2015). Fitting linear mixed-effects models using lme4. J. Stat. Softw. 67, 1–48. doi: 10.18637/jss.v067.i01
Benhadi-Marín, J., Santos, S. A. P., Baptista, P., Pereira, J. A. (2020). Distribution of Bactrocera oleae (Ross) throughout the iberian peninsula based on a maximum entropy modelling approach. Ann. Appl. Biol. 177, 112–120. doi: 10.1111/aab.12584
Benos, L., Tagarakis, A. C., Dolias, G., Berruto, R., Kateris, D., Bochtis, D. (2021). Machine learning in agriculture: A comprehensive updated review. Sensors. 21, 3758. doi: 10.3390/s21113758
Boccaccio, L., Petacchi, R. (2009). Landscape effects on the complex of Bactrocera oleae parasitoids and implications for conservation biological control. BioControl. 54, 607–616. doi: 10.1007/s10526-009-9214-0
Bonsignore, C. P., Vizzari, G., Vono, G., Bernardo, U. (2020). Short-term cold stress affects parasitism on the Asian chestnut gall wasp Dryocosmus kuriphilus. Insects. 11, 841. doi: 10.3390/insects11120841
Buckley, L. B., Briones Ortiz, B. A., Caruso, I., John, A., Levy, O., Meyer, A. V., et al. (2023). TrenchR: An R package for modular and accessible microclimate and biophysical ecology. PloS Clim. 2, e0000139. doi: 10.1371/journal.pclm.0000139
Burnham, K. P., Anderson, D. R. (2002). Model selection and multimodel inference: a practical information-theoretic approach. 2nd Edn (New York: Springer).
Capalbo, S. M., Antle, J. M., Seavert, C. (2017). Next generation data systems and knowledge products to support agricultural producers and science-based policy decision making. Agric. Syst. 155, 191–199. doi: 10.1016/j.agsy.2016.10.009
Caselli, A., Petacchi, R. (2021). Climate change and major pests of mediterranean olive orchards: Are we ready to face the global heating? Insects. 12, 802. doi: 10.3390/insects12090802
Crovetti, A., Quaglia, F., Loi, G., Rossi, E., Malfatti, P., Chesi, F., et al. (1982). Influenza di temperatura e umidità sullo sviluppo degli stadi preimaginali di Dacus oleae (Gmelin). Frust. Entom. V, 133–166.
Daher, E., Cinosi, N., Chierici, E., Rondoni, G., Famiani, F., Conti, E. (2022). Field and laboratory efficacy of low-impact commercial products in preventing olive fruit fly, Bactrocera oleae, infestation. Insects. 13, 213. doi: 10.3390/insects13020213
Damos, P. (2015). Modular structure of web-based decision support systems for integrated pest management. A review. Agron. Sustain. Dev. 35, 1347–1372. doi: 10.1007/s13593-015-0319-9
Damos, P., Colomar, L. A. E., Ioriatti, C. (2015). Integrated fruit production and pest management in Europe: The apple case study and how far we are from the original concept? Insects. 6, 626–657. doi: 10.3390/insects6030626
Delrio, G., Prota, R. (1977). Osservazioni eco etologiche sul Dacus oleae Gmelin nella Sardegna nord occidentale. Bollettino di zoologia agraria e bachicoltura. 13, 49–118.
Deutsch, C. A., Tewksbury, J. J., Huey, R. B., Sheldon, K. S., Ghalambor, C. K., Haak, D. C., et al. (2008). Impacts of climate warming on terrestrial ectotherms across latitude. PNAS. 105, 6668–6672. doi: 10.1073/pnas.0709472105
Deutsch, C. A., Tewksbury, J. J., Tigchelaar, M., Battisti, D. S., Merrill, S. C., Huey, R. B., et al. (2018). Increase in crop losses to insect pests in a warming climate. Science. 361, 916–919. doi: 10.1126/science.aat3466
Economopoulos, A. P., Haniotakis, G. E., Mathioudis, J., Missis, N., Kinigakis, P. (1978). Long-distance flight of wild and artificially-reared Dacus oleae (Gmelin) (Diptera, Tephritidae). Z. Fur Angew. Entomol. 87, 101–108. doi: 10.1111/j.1439-0418.1978.tb02430.x
Elbeaino, T., Yaseen, T., Valentini, F., Ben Moussa, I. E., Mazzoni, V., D’Onghia, A. M. (2014). Identification of three potential insect vectors of Xylella fastidiosa in southern Italy. Phytopathol. Mediterr. 53, 328–332. doi: 10.14601/Phytopathol_Mediterr-14113
Ferracini, C., Saitta, V., Rondoni, G., Rollet, I. (2023). Variables affecting the pine processionary moth flight: A survey in the North-Western Italian Alps. Forests. 14, 31. doi: 10.3390/f14010031
Forrest, J. R. (2016). Complex responses of insect phenology to climate change. Curr. Opin. Insect Sci. 17, 49–54. doi: 10.1016/j.cois.2016.07.002
Fraga, H., Moriondo, M., Leolini, L., Santos, J. A. (2021). Mediterranean olive orchards under climate change: A review of future impacts and adaptation strategies. Agronomy. 11, 56. doi: 10.3390/agronomy11010056
Garantonakis, N., Varikou, K., Markakis, E., Birouraki, A., Sergentani, C., Psarras, G., et al. (2016). Interaction between Bactrocera oleae (Diptera: Tephritidae) infestation and fruit mineral element content in Olea europaea (Lamiales: Oleaceae) cultivars of global interest. Appl. Entomol. Zool. 51, 257–265. doi: 10.1007/s13355-016-0397-4
Gkisakis, V. D., Bàrberi, P., Kabourakis, E. M. (2018). Olive canopy arthropods under organic, integrated, and conventional management. The effect of farming practices, climate and landscape. Agroecol. Sustain. Food Syst. 42, 843–858. doi: 10.1080/21683565.2018.1469066
Gömez-Caravaca, A. M., Cerretani, L., Bendini, A., Segura-Carretero, A., Fernández-Gutiérrez, A., Del Carlo, M., et al. (2008). Effects of fly attack (Bactrocera oleae) on the phenolic profile and selected chemical parameters of olive oil. J. Agric. Food Chem. 56, 4577–4583. doi: 10.1021/jf800118t
Gonçalves, M. F., Torres, L. M. (2011). The use of the cumulative degree-days to predict olive fly, Bactrocera oleae (Rossi), activity in traditional olive groves from the northeast of Portugal. J. Pest Sci. 84, 187–197. doi: 10.1007/s10340-010-0340-3
González-Fernández, A., Rallo, P., Peres, A. M., Pereira, J. A., Morales-Sillero, A. (2023). Developing predictive models under controlled conditions for the selection of new genotypes that are less susceptible to Bactrocera oleae (Rossi) in table olive (Olea europaea L.) breeding programs. Agronomy. 13, 3050. doi: 10.3390/agronomy13123050
Gordo, O., Sanz, J. J. (2005). Phenology and climate change: A long-term study in a Mediterranean locality. Oecologia. 146, 484–495. doi: 10.1007/s00442-005-0240-z
Gutierrez, A. P., Ponti, L., Cossu, Q. A. (2009). Effects of climate warming on olive and olive fly (Bactrocera oleae (Gmelin)) in California and Italy. Clim. Change 95, 195–217. doi: 10.1007/s10584-008-9528-4
Haman, J., Avery, M. (2020). ciTools: Confidence or prediction intervals, quantiles, and probabilities for statistical models. R Package version 0.6 1.
Hargreaves, G. H., Samani, Z. A. (1985). Reference crop evapotranspiration from temperature. Appl. Eng. Agric. 1, 96–99. doi: 10.13031/2013.26773
Hatherly, I. S., Hart, A. J., Tullett, A. G., Bale, J. S. (2005). Use of thermal data as a screen for the establishment potential of non-native biological control agents in the UK. BioControl. 50, 687–698. doi: 10.1007/s10526-005-6758-5
Helvaci, M., Aktaş, M., Özden, Ö. (2018). Occurrence, damage, and population dynamics of the olive fruit fly (Bactrocera oleae Gmelin) in the Turkish Republic of Northern Cyprus. Turk. J. Agric. For. 42, 453–458. doi: 10.3906/tar-1802-101
Ip, R. H. L., Ang, L. M., Seng, K. P., Broster, J. C., Pratley, J. E. (2018). Big data and machine learning for crop protection. Comput. Electron. Agric. 151, 376–383. doi: 10.1016/j.compag.2018.06.008
Jacobs, K., Jonard, M., Muys, B., Ponette, Q. (2022). Shifts in dominance and complementarity between sessile oak and beech along ecological gradients. J. Ecol. 110, 2404–2417. doi: 10.1111/1365-2745.13958
Johnson, M. W., Wang, X. G., Nadel, H., Opp, S. B., Lynn-Patterson, K., Stewart-Leslie, J., et al. (2011). High temperature affects olive fruit fly populations in California’s Central Valley. Calif. Agric. (Berkeley). 65, 29–33. doi: 10.3733/ca.v065n01p29
Jones, V. P., Alston, D., Brunner, J. F., Davis, D. W., Shelton, M. D. (1991). Phenology of western cherry fruit fly (Diptera: Tephritidae) in utah and washington. Ann. Entomol. Soc Am. 84, 488–492. doi: 10.1002/ps.1913
Katipoğlu, O. M. (2022). Analysis of spatial variation of temperature trends in the semiarid Euphrates basin using statistical approaches. Acta Geophys. 70, 1899–1921. doi: 10.1007/s11600-022-00819-2
Kim, S. N., Lee, W. K., Shin, K. I., Kafatos, M., Seo, D. J., Kwak, H. (2010). Comparison of spatial interpolation techniques for predicting climate factors in Korea. For. Sci. Technol. 6, 97–109. doi: 10.1080/21580103.2010.9671977
Koveos, D. S. (2001). Rapid cold hardening in the olive fruit fly Bactrocera oleae under laboratory and field conditions. Entomol. Exp. Et. Appl. 101, 257–263. doi: 10.1046/j.1570-7458.2001.00910.x
Krasnov, H., Cohen, Y., Goldshtein, E., Mendelsohn, O., Silberstein, M., Gazit, Y., et al. (2019). The effect of local and landscape variables on Mediterranean fruit fly dynamics in citrus orchards utilizing the ecoinformatics approach. J. Pest Sci. 92, 453–463. doi: 10.1007/s10340–018-1023–8
Lantero, E., Matallanas, B., Callejas, C. (2023). Current status of the main olive pests: Useful Integrated Pest Management strategies and genetic tools. Appl. Sci. 13, 12078. doi: 10.3390/app132112078
Lüdecke, D. (2018). ggeffects: Tidy data frames of marginal effects from regression models. J. Open Source Softw. 3, 772. doi: 10.21105/joss.00772
Malheiro, R., Casal, S., Baptista, P., Pereira, J. A. (2015). A review of Bactrocera oleae (Rossi) impact in olive products: From the tree to the table. Trends Food Sci. Technol. 44, 226–242. doi: 10.1016/j.tifs.2015.04.009
Marchi, S., Guidotti, D., Ricciolini, M., Petacchi, R. (2016). Towards understanding temporal and spatial dynamics of Bactrocera oleae (Rossi) infestations using decade-long agrometeorological time series. Int. J. Biometeorol. 60, 1681–1694. doi: 10.1007/s00484-016-1159-2
Marchini, D., Petacchi, R., Marchi, S. (2017). Bactrocera oleae reproductive biology: new evidence on wintering wild populations in olive groves of Tuscany (Italy). Bull. Insectol. 70, 121–128.
Mascandola, C., Luzi, L., Felicetta, C., Pacor, F. (2021). A GIS procedure for the topographic classification of Italy, according to the seismic code provisions. Soil Dyn. Earthq. Eng. 148, 106848. doi: 10.1016/j.soildyn.2021.106848
Mazomenos, B. E., Pantazi-Mazomenou, A., Stefanou, D. (2002). Attract and kill of the olive fruit fly Bactrocera oleae in Greece as a part of an integrated control system. IOBC WPRS Bull. 25, 137–146.
McQueen, R. J., Garner, S. R., Nevill-Manning, C. G., Witten, I. H. (1995). Computers and electronics in agriculture applying machine learning to agricultural data. Comput. Electron. Agric. 12, 275–293. doi: 10.1016/0168-1699(95)98601-9
Merbitz, H., Buttstädt, M., Michael, S., Dott, W., Schneider, C. (2012). GIS-based identification of spatial variables enhancing heat and poor air quality in urban areas. Appl. Geogr. 33, 94–106. doi: 10.1016/j.apgeog.2011.06.008
Michalopoulos, G., Kasapi, K. A., Koubouris, G., Psarras, G., Arampatzis, G., Hatzigiannakis, E., et al. (2020). Adaptation of Mediterranean olive groves to climate change through sustainable cultivation practices. Climate. 8, 54. doi: 10.3390/cli8040054
Miranda, M.Á., Barceló, C., Valdés, F., Feliu, J. F., Nestel, D., Papadopoulos, N., et al. (2019). Developing and implementation of decision support system (DSS) for the control of olive fruit fly, Bactrocera oleae, in mediterranean olive orchards. Agronomy. 9, 620. doi: 10.3390/agronomy9100620
Nestel, D., Cohen, Y., Shaked, B., Alchanatis, V., Nemny-Lavy, E., Miranda, M. A., et al. (2019). An integrated decision support system for environmentally-friendly management of the ethiopian fruit fly in greenhouse crops. Agronomy. 9, 459. doi: 10.3390/agronomy9080459
Neuenschwander, P., Michelakis, S., Bigler, F. (1981). Abiotic factors affecting mortality of Dacus oleae larvae and pupae in the soil. Entomol. Exp. Appl. 30, 1–9. doi: 10.1111/j.1570-7458.1981.tb03577.x
Ordano, M., Engelhard, I., Rempoulakis, P., Nemny-Lavy, E., Blum, M., Yasin, S., et al. (2015). Olive fruit fly (Bactrocera oleae) population dynamics in the Eastern Mediterranean: Influence of exogenous uncertainty on a monophagous frugivorous insect. PloS One 10, e0127798. doi: 10.1371/journal.pone.0127798
Ortega, M., Moreno, N., Fernández, C. E., Pascual, S. (2022). Olive landscape affects Bactrocera oleae abundance, movement and infestation. Agronomy. 12, 4. doi: 10.3390/agronomy12010004
Ortega, M., Sánchez-Ramos, I., González-Núñez, M., Pascual, S. (2018). Time course study of Bactrocera oleae (Diptera: Tephritidae) pupae predation in soil: The effect of landscape structure and soil condition. Agric. For. Entomol. 20, 201–207. doi: 10.1111/afe.12245
Paredes, D., Alves, J. F., Mendes, S., Costa, J. M., Alves, J., da Silva, A. A., et al. (2023). Landscape simplification increases Bactrocera oleae abundance in olive groves: adult population dynamics in different land uses. J. Pest Sci. 96, 71–79. doi: 10.1007/s10340-022-01489-1
Pereira-Castro, I., Van Asch, B., Rei, F. T., Da Costa, L. T. (2015). Bactrocera oleae (Diptera: Tephritidae) organophosphate resistance alleles in Iberia: recent expansion and variable frequencies. Eur. J. Entomol. 112, 20–26. doi: 10.14411/eje.2015.019
Petacchi, R., Marchi, S., Federici, S., Ragaglini, G. (2015). Large-scale simulation of temperature-dependent phenology in wintering populations of Bactrocera oleae (Rossi). J. Appl. Ent. 139, 496–509. doi: 10.1111/jen.12189
Pinheiro, J., Bates, D. (2006). Mixed-effects models in S and S-PLUS (New York, NY: Springer). doi: 10.1007/b98882
Preu, M., Frieß, J. L., Breckling, B., Schröder, W. (2020). “Case study 1: olive fruit fly (Bactrocera oleae),” in Gene Drives at Tipping Points: Precautionary Technology Assessment and Governance of New Approaches to Genetically Modify Animal and Plant Populations. Eds. von Gleich, A., Schröder, W. (Springer, Cham, Switzerland), 79–101. doi: 10.1007/978–3-030–38934-5
Quaglia, F., Malfatti, P., Conti, B. (1981). Modalitá diverse per la valutazione dell'infestazione dacica. Esame preliminare dei risultati ottenuti nella sperimentazione condotta nel 1980 in Toscana. Frustula Entomol. 17, 267–276.
Ragaglini, G., Tomassone, D., Petacchi, R. (2007). Can spring-preventive adulticide treatments be assumed to improve Bactrocera oleae (Rossi) management? IOBC WPRS Bull. 30, 309–314.
Raza, M. M., Khan, M. A., Arshad, M., Sagheer, M., Sattar, Z., Shafi, J., et al. (2015). Impact of global warming on insects. Arch (84–94: Phytopathol. Plant Protection48). doi: 10.1080/03235408.2014.882132
R Core Team. (2022). R: A Language and environment for Statistical Computing. Available online at: https://www.R-project.org/.
Rebaudo, F., Rabhi, V. B. (2018). Modeling temperature-dependent development rate and phenology in insects: Review of major developments, challenges, and future directions. Entomol. Exp. Appl. 166, 607–617. doi: 10.1111/eea.12693
Rizzo, R., Caleca, V., Lombardo, A. (2012). Relation of fruit color, elongation, hardness, and volume to the infestation of olive cultivars by the olive fruit fly, Bactrocera oleae. Entomol. Exp. Et Appl. 145, 15–22. doi: 10.1111/j.1570-7458.2012.01311.x
Rondoni, G., Onofri, A., Ricci, C. (2012). Differential susceptibility in a specialized aphidophagous ladybird, Platynaspis luteorubra (Coleoptera: Coccinellidae), facing intraguild predation by exotic and native generalist predators. Biocontrol Sci. Technol. 22, 1334–1350. doi: 10.1080/09583157.2012.726607
Rossini, L., Bruzzone, O. A., Contarini, M., Bufacchi, L., Speranza, S. (2022). A physiologically based ODE model for an old pest: Modeling life cycle and population dynamics of Bactrocera oleae (Rossi). Agronomy. 12, 2298. doi: 10.3390/agronomy12102298
Sciarretta, A., Tabilio, M. R., Amore, A., Colacci, M., Miranda, M., Nestel, D., et al. (2019). Defining and evaluating a Decision Support System (DSS) for the precise pest management of the Mediterranean fruit fly, Ceratitis capitata, at the Farm Level. Agronomy 9, 608. doi: 10.3390/agronomy9100608
Sevarika, M., Rondoni, G., Ganassi, S., Pistillo, O. M., Germinara, G. S., De Cristofaro, A., et al. (2022). Behavioural and electrophysiological responses of Philaenus spumarius to odours from conspecifics. Sci. Rep. 12, 8402. doi: 10.1038/s41598–022-11885–3
Skendžić, S., Zovko, M., Živković, I. P., Lešić, V., Lemić, D. (2021). Effect of climate change on introduced and native agricultural invasive insect pests in Europe. Insects 12, 985. doi: 10.3390/insects12110985
Skouras, P. J., Margaritopoulos, J. T., Seraphides, N. A., Ioannides, I. M., Kakani, E. G., Mathiopoulos, K. D., et al. (2007). Organophosphate resistance in olive fruit fly, Bactrocera oleae, populations in Greece and Cyprus. Pest. Manage. Sci. 63, 42–48. doi: 10.1002/ps.1306
Song, Y. H., Coop, L. B., Omeg, M., Rield, H. (2003). Development of a phenology model for predicting western cherry fruit fly, rhagoletis indifferens curran (Diptera: Tephritidae), emergence in the mid columbia area of the western united states. J. Asia. Pac. Entomol. 6, 187–192. doi: 10.1016/S1226-8615(08)60185-5
Stetter, J., Lieb, F. (2000). Innovation in crop protection: trends in research. Angew. Chem. Int. Ed. Engl. 39, 1724–1744. doi: 10.1002/(SICI)1521–3773(20000515)39:10<1724::AID-ANIE1724>3.0.CO;2–5
Tarquini, S., Isola, I., Favalli, M., Mazzarini, F., Bisson, M., Pareschi, M. T., et al. (2007). TINITALY/01: a new triangular irregular network of Italy. Ann. Geophys. 50, 407–425. doi: 10.1034/j.1600–0706.2003.12567.x
Thies, C., Steffan-Dewenter, I., Tscharntke, T. (2003). Effects of landscape context on herbivory and parasitism at different spatial scales. Oikos. 101, 18–25. doi: 10.1034/j.1600-0706.2003.12567.x
Tidau, S., Brough, F. T., Gimenez, L., Jenkins, S. R., Davies, T. W. (2023). Impacts of artificial light at night on the early life history of two ecosystem engineers. Philos. Trans. R. Soc Lond. Ser. B Biol. Sci. 378, 1892. doi: 10.1098/rstb.2022.0363
Tognetti, R., d’Andria, R., Lavini, A., Morelli, G. (2006). The effect of deficit irrigation on crop yield and vegetative development of Olea europaea L. (cvs. Frantoio and Leccino). Eur. J. Agron. 25, 356–364. doi: 10.1016/j.eja.2006.07.003
Torres-Villa, L. M., Sanchez Gonzalez, A., Ponce Escudero, F., Delgado Valiente, E., Aza Barrero, M. C., Barrena Galan, F., et al. (2006). Dinámica poblacional de” Bactrocera oleae” Gmelin en Extremadura: fluctuación estacional en el estado reproductivo y en el tamaño imaginal. Bol. Sanid. Veg. Plagas 32, 57–70.
Tsolakis, H., Ragusa, E., Tarantino, P. (2011). Control of Bactrocera oleae by low environmental impact methods: NPC methodology to evaluate the efficacy of lure-and-kill method and copper hydroxide treatments. Bull. Insectol. 64, 1–8.
Van Asch, B., Pereira-Castro, I., Rei, F. T., Da Costa, L. T. (2015). Marked genetic differentiation between Western Iberian and Italic populations of the olive fly: Southern France as an intermediate area. PloS One 10, e0126702. doi: 10.1371/journal.pone.0126702
Varikou, K. (2022). Bactrocera oleae (olive fruit fly). CABI Compendium. doi: 10.1079/cabicompendium.17689
Varikou, K., Garantonakis, N., Denaxa, N. K., Tsafouros, A., Ntanos, E., Economou, L., et al. (2022). Olive cultivar differences in fruit phenolic compounds and size define host preference of Bactrocera oleae (Diptera: Tephritidae). Int. J. Pest Manage., 1–12. doi: 10.1080/09670874.2021.2015009
Venables, W. N., Ripley, B. D. (2002). Modern applied statistics with S. Fourth Edition (New York, NY: Springer). doi: 10.1007/978–0-387–21706-2
Villa, M., Santos, S. A., Sousa, J. P., Ferreira, A., da Silva, P. M., Patanita, I., et al. (2020). Landscape composition and configuration affect the abundance of the olive moth (Prays oleae, Bernard) in olive groves. Agric. Ecosyst. Environ. 294, 106854. doi: 10.1016/j.agee.2020.106854
Volpi, I., Guidotti, D., Mammini, M., Petacchi, R., Marchi, S. (2020). Managing complex datasets to predict Bactrocera oleae infestation at the regional scale. Comput. Electron. Agric. 179, 105867. doi: 10.1016/j.compag.2020.105867
Wang, X. G., Johnson, M. W., Daane, K. M., Nadel, H. (2009). High summer temperatures affect the survival and reproduction of olive fruit fly (Diptera: Tephritidae). Environ. Entomol. 38, 1496–1504. doi: 10.1603/022.038.0518
Wang, X. G., Levy, K., Nadel, H., Johnson, M. W., Blanchet, A., Argov, Y., et al. (2013). Overwintering survival of olive fruit fly (Diptera: Tephritidae) and two introduced parasitoids in California. Environ. Entomol. 42, 467–476. doi: 10.1603/EN12299
Wickham, H. (2016). ggplot2: Elegant graphics for data analysis (New York: Springer-Verlag). doi: 10.1007/978–3-319–24277-4
Yokoyama, V. Y. (2015). Olive Fruit Fly (Diptera: Tephritidae) in California table olives, USA: Invasion, distribution, and management implications. J. Integr. Pest Manage. 6, 14. doi: 10.1093/jipm/pmv014
Keywords: Bactrocera oleae, Diptera, insect monitoring, Oleaceae, oviposition, pest management, Tephritidae
Citation: Rondoni G, Mattioli E, Giannuzzi VA, Chierici E, Betti A, Natale G, Petacchi R, Famiani F, Natale A and Conti E (2024) Evaluation of the effect of agroclimatic variables on the probability and timing of olive fruit fly attack. Front. Plant Sci. 15:1401669. doi: 10.3389/fpls.2024.1401669
Received: 15 March 2024; Accepted: 10 June 2024;
Published: 15 July 2024.
Edited by:
Gaetano Distefano, University of Catania, ItalyReviewed by:
Clive Kaiser, Lincoln University, New ZealandUmberto Bernardo, National Research Council (CNR), Italy
Copyright © 2024 Rondoni, Mattioli, Giannuzzi, Chierici, Betti, Natale, Petacchi, Famiani, Natale and Conti. This is an open-access article distributed under the terms of the Creative Commons Attribution License (CC BY). The use, distribution or reproduction in other forums is permitted, provided the original author(s) and the copyright owner(s) are credited and that the original publication in this journal is cited, in accordance with accepted academic practice. No use, distribution or reproduction is permitted which does not comply with these terms.
*Correspondence: Gabriele Rondoni, Z2FicmllbGUucm9uZG9uaUB1bmlwZy5pdA==
†These authors share first authorship