1 Introduction
Alfalfa (Medicago sativa L.) is the most widely planted perennial leguminous forage in the world and it plays a vital role in the diet of dairy cows (Fink et al., 2022; Zhang et al., 2023). Feeding alfalfa can substantially improve animal’s growth, reproduction, meat and milk production (Laroche et al., 2022; Ma et al., 2022; Motsinger, 2022). Alfalfa is currently the most commonly cultivated forage crop in China (Wan et al., 2022). Ningxia is located in the northwest agricultural and pastoral ecotone in China. It is one of the major alfalfa production areas in Northeast China. By 2022, the alfalfa planting area in Ningxia has reached 330000 hectares (Wang B. et al., 2022). The country’s aggressive promotion of “grain-forage substitution”, has expanded the planting area of silage maize, alfalfa and other feed crops, increased the harvest and storage capacity, promoted the specialization of feed varieties, production scale, and commercialization of sales, and opened up new possibilities for the Ningxia alfalfa business (Hui et al., 2021). However, the expanding cultivation area of alfalfa in Ningxia is facing significant challenges due to the influence of regional climate, limited water resources, and soil conditions (Qian et al., 2020). This has resulted in a growing contradiction between the increasing demand for water resources and the expanding area dedicated to alfalfa cultivation. Consequently, there is a growing need to monitor alfalfa yields to address yield gaps, including those caused by water stress and fertility limitations (Baral et al., 2022).
Timely and appropriate application of irrigation and fertilizer are essential for improving the yield and quality of alfalfa (Liu et al., 2021; Wang et al., 2021). The leaf area index (LAI) is a valuable dynamic indicator that reflects the size of crop populations and serves as a critical basis for analyzing crop growth patterns and predicting yield (Feng et al., 2022). It plays a crucial role in determining the net primary productivity, water and nutrient utilization, and carbon balance in alfalfa (Moghaddam and Mofidian, 2022). Therefore, it is important to study the characteristics and spatiotemporal changes of leaf area (LA) and LAI in alfalfa, which can improve yield estimation and the efficiency of water and fertilizer utilization in arid and semi-arid regions.
Currently, extensive research has been undertaken by researchers on the variations in LAI (Leaf Area Index) across different crops like rice, wheat, and maize. (Wang E. et al., 2022). Some of these studies have employed deep learning technology to estimate the LAI and assess its impact on crop yield (Jin et al., 2020; Qi et al., 2020; Tian et al., 2021). For example, Adeluyi et al. (2021) implemented rice experiments in the central northern region of Nigeria. Three irrigation treatments and three nitrogen fertilizer application levels were designed and LAIs were measured in each plot using the LAI-2200 plant canopy analyzer from December 2017 to April 2018. Zhang et al. (2021) conducted winter wheat experiments in the Xindian area of Luoyang City. They designed four nitrogen fertilizer treatments, and 132 LAI data points were collected in 2018 during the different growth stage of winter wheat. There are also studies on maize LAI experiments. Castro-Valdecantos et al. (2022) implemented maize breeding trials in Seville, Spain from 2018 to 2019. A total of 32 maize plots were selected, and two representative plants were randomly selected from each plot on each sampling date. The LAI for each experimental plot was estimated using the allometric growth relationship. However, the aforementioned literature did not publish the related datasets. In recent years, extensive research has been conducted on the growth characteristics, water consumption patterns, and irrigation regimes of alfalfa, with a focus on the interaction between field management practices, crop morphological characteristics, and yield (Liu et al., 2022; Ojaghlou et al., 2023; Wang et al., 2023). However, there have been relatively few studies on alfalfa LAI. To the best of our knowledge, there is currently no publication on alfalfa leaf area (LA) and LAI datasets in Northwest China, despite the fact that China is the second-largest producer of alfalfa in the world (Lv et al., 2023).
To address this issue, we proposed a dataset that can be utilized for estimating LA and predicting LAI of alfalfa. The dataset included meteorological data, soil moisture measurements at multiple depths (0-10 cm, >10-20 cm, and >20-30 cm), and various parameters associated with alfalfa growth, including plant height, LAI, yield, and leaf dimensions (length, width, and area). The main objective of this research work is to provide a dataset of the characteristics and spatiotemporal changes of LA and LAI in alfalfa for estimating yield and optimizing field water and fertilizer management.
2 Value of the data
(1) The dataset provides growth data of alfalfa under different water and nitrogen treatments. A total of 5118 alfalfa samples were collected, and the length, width, and LA of 76688 leaves were measured. Moreover, the LAI of different experimental plots was calculated. This dataset filled the gap in publicly published alfalfa growth data in northwest China, helping to address the challenges of estimating LA and predicting LAI of alfalfa in different production environments. Furthermore, the dataset provides essential data support for optimizing water and nitrogen strategies in alfalfa field management.
(2) The dataset includes meteorological data throughout the entire growth period of alfalfa and soil moisture data at various depths. Integrating multiple features is beneficial for expanding the applicability of estimation and prediction models and improving their accuracy.
3 Materials and methods
3.1 Experimental design
In this study, the interest area is the Ningxia Irrigation Area of Yellow River (NIR), which is situated in the Ningxia Hui Autonomous Region in China. The experiment was implemented at the first grassland experiment site of Ningxia State Farm Maosheng Prataculture Co., Ltd., located on the eastern foothills of the Helan Mountains in the alluvial fan plain. The effects of different water and nitrogen application amounts on alfalfa LA and LAI vary, and the responses of different alfalfa varieties to LA and LAI also differ under rates of irrigation and nitrogen application. Based on the actual cultivation of alfalfa in the NIR, we designed two experiments to collect LA and LAI data for alfalfa (2017-2018 and 2022). During the period from 2019 to 2021, the experimental field was utilized for planting silage maize using a rotation method.
The first experiment, conducted from 2017 to 2018, involved collecting data on the same alfalfa variety, Juneng 7, under different water and nitrogen treatments. Juneng 7 is a widely planted alfalfa variety in Ningxia. The seeding rate was 22.5 kg ha-1, with a seeding depth of 2 cm and row spacing of 15 cm. According to the irrigation methods used in the NIR, we designed two irrigation methods: flood irrigation and subsurface drip irrigation. The experiment adopted a split-plot design, with irrigation amount as the main treatment and nitrogen application amount as the sub-treatment. 1) A total of 5 levels were designed for the irrigation amounts, including 1199 mm (flood irrigation plot, 12000 m3 ha-1), 525 mm (W1, 5250 m3 ha-1), 600 mm (W2, 6000 m3 ha-1), 675 mm (W3, 6750 m3 ha-1), and 750 mm (W4, 7500 m3 ha-1), respectively. 2) A total of 4 levels were designed for the nitrogen application rates, including N0 (0 kg ha-1), N1 (60 kg ha-1), N2 (120 kg ha-1), and N3 (180 kg ha-1), respectively. This experiment consisted of 18 treatments, each repeated 3 times. The irrigation schedule was implemented when the alfalfa was turning green. The specific irrigation schedule is shown in Table 1.
The second experiment was conducted in 2022, where data was measured on seven different alfalfa varieties: Juneng No.7, Zhongmu No.3, Gannong No.4, Zhonglan No.2, DF310, Miracle, and Algangjin. This experiment focused on measuring alfalfa growth data under different rate of irrigation while keeping the nitrogen application rate constant. The alfalfa was sown on April 16, 2022, with a seeding rate of 22.5 kg ha-1, a seeding depth of 2 cm, and a row spacing of 15 cm. The irrigation method used in this experiment was surface micro-sprinkler irrigation, with three irrigation treatments: 525 mm (W1, 5250 m3 ha-1), 675 mm (W2, 6750 m3 ha-1), and 750 mm (W3, 7500 m3 ha-1). The irrigation schedule can be seen in Table 1.
3.2 Data acquisition
32.1 Alfalfa growth data acquisition
In this study, three random plants were selected from each plot for measurement after each cutting of alfalfa regrowth (Figure 1A). To eliminate measurement errors caused by water loss, stem diameter, plant height, leaf length, leaf width, and leaf area were all measured on the same day of sampling. Additionally, the number of leaves was counted. The measurement methods differed slightly between 2017-2018 and 2022. (i) In 2017-2018, we randomly selected intact and healthy leaves in layers, with 6 leaves selected from each layer. Leaf length and leaf width were measured using a vernier caliper with an accuracy of 0.01 mm (Figure 1E). The leaf area was calculated using the leaf area estimation formula (Faverjon et al., 2017). The specific calculation formula is shown in Equation (1). (ii) In 2022, we used an HP M126a scanner (Figure 1D) and Image J software to measure leaf length, leaf width, and leaf area (Sun et al., 2015). In this method, the leaves were flattened and placed on a scanner bed (HP M126a) and scanned sequentially at a resolution of 300 dpi, with the images saved in jpg format. Since the stems were not removed during scanning, the stem portion was manually removed using the drawing tool in Image J software before calculating the leaf area (Figure 1F). For leaf area measurement, the color mode was changed to 8-bit grayscale, and the “Size” value in the “Analyze Particles” function was set to 20-Infinity, considering areas below 20 mm² as impurities and excluding them from area recognition. The calculation method of LAI is shown in Equation (2) (Gower et al., 1999). Figure 2 shows the LAI of alfalfa under different water and nitrogen treatments in 2018.
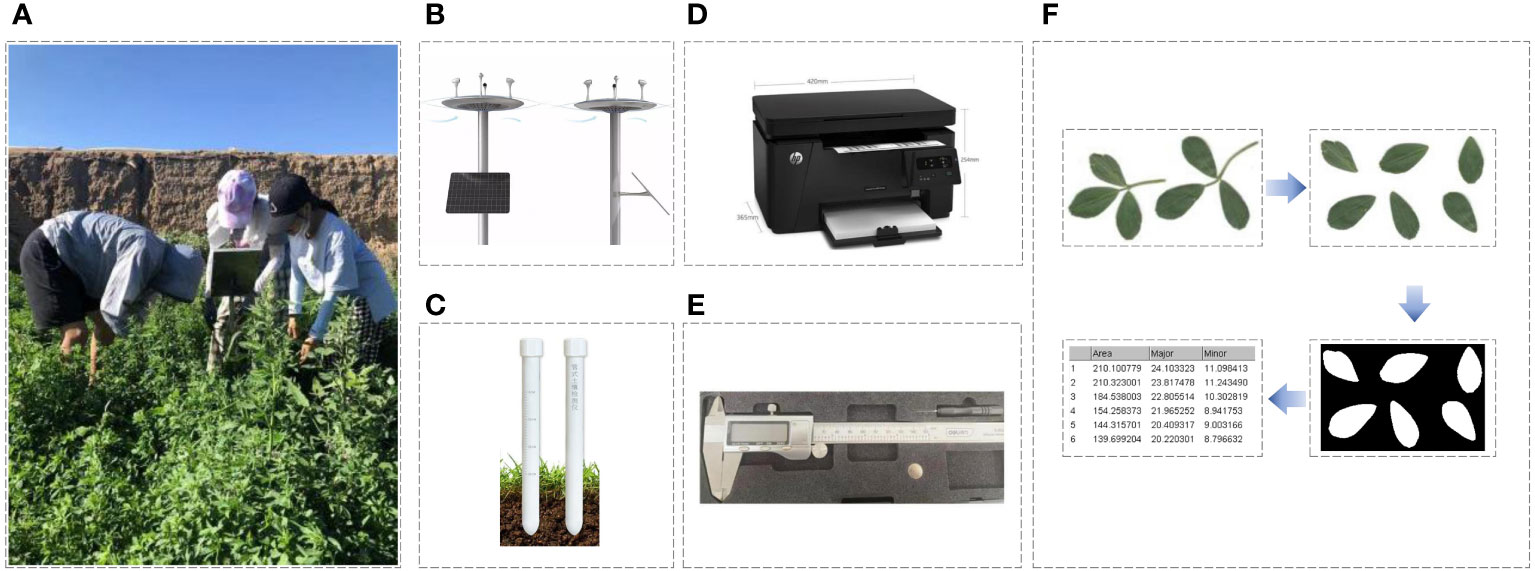
Figure 1 The experimental scenario and equipment deployed. (A) Data collection scenario; (B) Meteorological stations; (C) soil moisture sensors; (D) Scanner; (E) vernier caliper; (F) Leaf area measurement process.
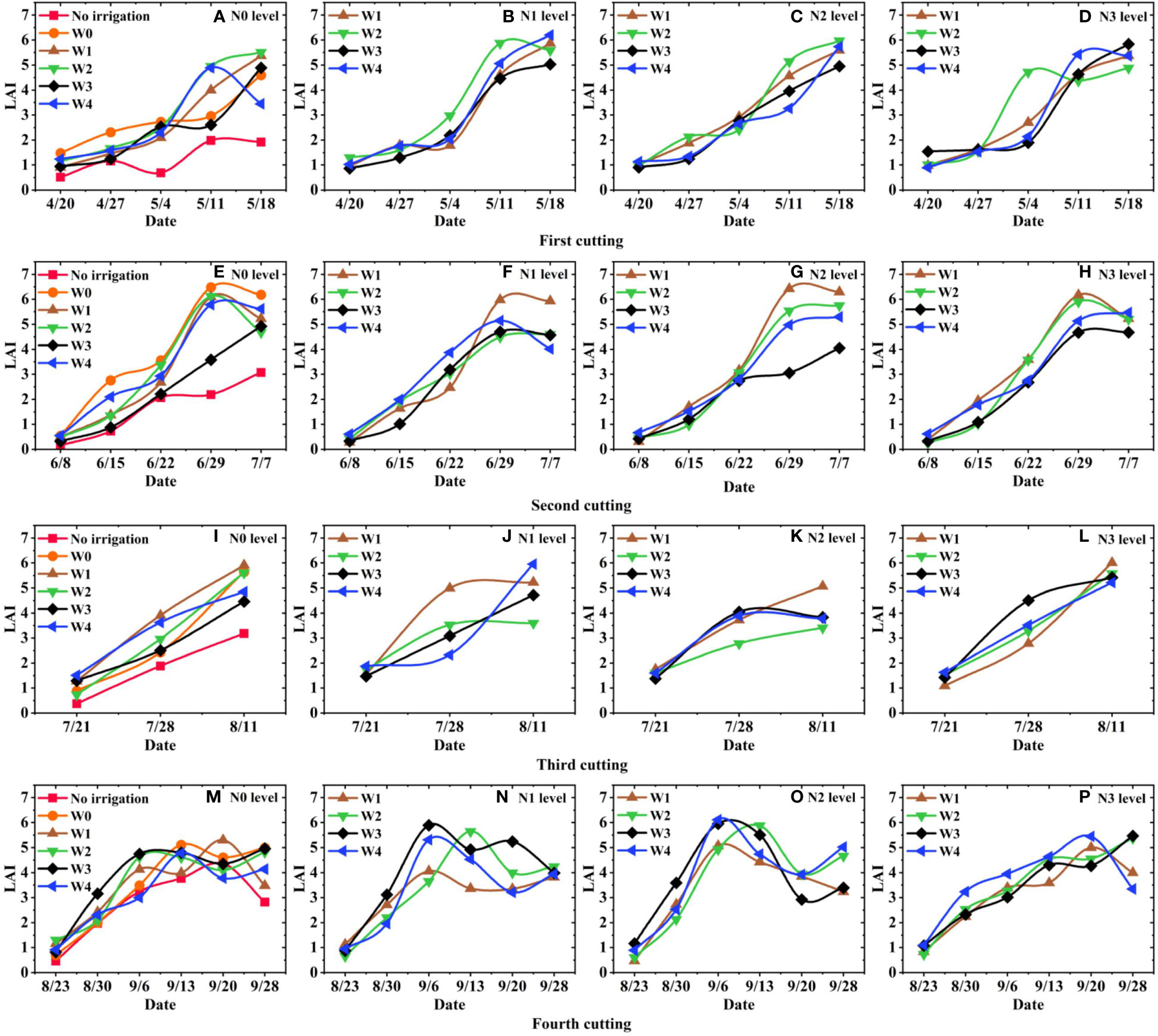
Figure 2 The LAI of alfalfa under different water and nitrogen treatments in 2018. (A, E, I, M) represents the LAI at N0, (B, F, J, N) represents the LAI at N1, (C, G, K, O) represent the LAI at N2, and (D, H, L, P) represents the LAI at N3.
Where LA represents the leaf area; L and W represent the leaf length and the leaf width (mm), respectively; and K represents the correction coefficient.
Where LAI represents the leaf area index, AVGLA is the average leaf area per plant, ρ is the planting density, and S is the planting area.
32.2 Yield data acquisition
Yield refers to the total biomass of a crop per unit of land area, excluding the root system. It reflects alfalfa productivity, and a higher yield signifies greater productivity. It is directly influenced by meteorological circumstances as well as diverse water and nitrogen management strategies. During the growth period of the early flowering stage, we carefully selected alfalfa plants with uniform and consistent growth for harvest. Use the diagonal method to take 3 sample areas in each plot, with each sample area measuring 1 m2. The stubble height was maintained 5 cm. We measured the fresh yield of alfalfa after removing other weeds. Additionally, we took about 300 g of fresh alfalfa samples back to the laboratory and let them naturally dry in the wind until they reach below 20% moisture content. Then, we estimated forage dry matter yield.
32.3 Meteorological and soil data acquisition
In this study, we deployed the ET007 Tianqi micro meteorological station (Figure 1B) and the ET40 intelligent soil moisture sensor (Figure 1C), which are specialized equipment designed for scientific research, to collect environmental and soil data.
Meteorological data were collected using Campbell Scientific data recorders, with a measurement interval of 60 seconds and data output of every 30 minutes. The weather station recorded various parameters including temperature (°C), net radiation (MJ m-2), CO2 concentration (mg m-3), soil moisture (m3 m-3), relative humidity (%), wind direction and wind speed (m s-1). According to the records of the meteorological station, the average temperatures during the alfalfa growth period measured in the experimental area were 20.6°C, 20.2°C, and 23.0°C in 2017, 2018, and 2022, respectively. The daily average temperature and effective accumulated temperature were estimated using Python 3.6 and the PyCharm program (Linge and Langtangen, 2020).
Soil moisture sensors were deployed at depths of 0-10 cm, >10-20 cm, and >20-30 cm with a data collection interval of 10 minutes for real-time monitoring over 24 hours. Due to limited experimental conditions, soil moisture was measured in seven different treatments in 2017-2018. These treatments included W2N0, W2N1, W2N2 and W2N3 under the same irrigation amount, as well as W1N2, W3N2 and W4N2 under the same nitrogen application rate.
4 Description and analysis of dataset
The dataset contains a total of five files. The dataset is fairly straightforward for setting up because we put data in files that correspond to the proper annotations. The dataset provides growth data of alfalfa under different water and nitrogen treatments. A total of 5118 alfalfa samples were collected, and the length, width, and LA of 76688 leaves were measured. The detailed information of the dataset is shown in Table 2.
LAI is a key indicator for evaluating the growth structure of individual alfalfa plants or entire populations (Hammond et al., 2023). This study used Origin software to analyze the effects of different water regimes and nitrogen rates on the leaf area index of Juneng 7 alfalfa in 2018. Figure 2 shows the dynamic trend of alfalfa LAI under different water and nitrogen treatments in 2018 of the datasets. Figure 2 shows that the overall trend of LAI changes in alfalfa is essentially consistent with that of alfalfa harvested for forage purposes. After the plant turned green, alfalfa grew rapidly, and the LAI increased rapidly as the temperature rose. Due to influence of temperature during the growth period, the LAI of the second and third cuttings rapidly increased after regrowth stage, while the LAI of the first and fourth cuttings showed slower growth (Figure 2). The LAI of the fourth cutting exhibited a declining trend in the later growth period, which aligned with Bai and Bao (2002)’s findings that as autumn approaches, the LAI of alfalfa typically decreases during the later development stage (after the early flowering stage) (Figures 2M-P). Additionally, the maximum LAI of the first, second, and third cuttings can reach or approach 6, whereas the maximum LAI of the fourth cutting in the observed region was around 5 (Figure 2). This result consistent with Gao and Hannaway (1985)’s research who stated that the maximum LAI of the fourth cutting indicates that unpredictable climate factors affect alfalfa growth during autumn.
Moreover, the growth of alfalfa is influenced and restricted by water and nitrogen. Additionally, previous research has shown that water has a greater impact on alfalfa yield compared to nitrogen (Sun et al., 2005). Through analyzing the dataset, it was found that different irrigation treatments have different effects on the alfalfa LAI (Figure 2). The overall performance of alfalfa LAI under W2 irrigation treatment was higher compared to other treatments, indicating that an appropriate amount of irrigation can impact the stem-leaf ratio of alfalfa and consequently affect the crude protein content of alfalfa (Su et al., 2011). However, under the same irrigation treatment, the impact of different nitrogen application rates on alfalfa LAI was not observed significant. Compared to other nitrogen treatments, the LAI performance under low nitrogen application rates was better. Upon analyzing the dataset, we observed that the response of LAI to nitrogen application varied across different alfalfa planting years (Figure 2). Notably, 2018 marked the third year of alfalfa planting. Based on the analysis of Figure 2, it is found that the impact of different nitrogen application rates on alfalfa LAI is not significant. This finding aligned with the research conclusion that applying nitrogen fertilizer during the year of alfalfa planting can improve plant growth and enhance winter survival. However, after more than two years of planting, alfalfa can rely on its own rhizobia nitrogen fixation to meet its nitrogen needs (Peters and Stritzke, 1970; Eardly et al., 1985; Gao et al., 2020). Additionally, significant variations were observed in the response of LAI to water and nitrogen across different alfalfa varieties and cuttings.
5 Potential use
The dataset holds significant practical importance within the context of deep learning advancements. It has the potential to assist in establishing models for estimating alfalfa leaf area and predicting leaf area index in complex physical environments. Furthermore, this dataset provides vital data support for alfalfa yield estimation and optimization of water and nitrogen strategy in field management.
Data availability statement
The datasets presented in this study can be found in online repositories. The names of the repository/repositories and accession number(s) can be found below: https://github.com/im1robot/leaf-area-and-leaf-area-index.git.
Author contributions
SY: Data curation, Writing – original draft, Writing – review & editing. YG: Data curation, Funding acquisition, Investigation, Writing – review & editing. JW: Supervision, Writing – review & editing. RL: Data curation, Supervision, Writing – review & editing. DT: Supervision, Writing – review & editing. AL: Supervision, Writing – review & editing. ZZ: Supervision, Writing – review & editing.
Funding
The author(s) declare financial support was received for the research, authorship, and/or publication of this article. This work was supported in part by National natural science foundation of China (62262052, 62162052), in part by Key Research and Development Project of Ningxia Province (2021BEB04016, 2022BDE03007), Natural Science Foundation of Ningxia (2021AAC03041, 2022AAC03004).
Conflict of interest
The authors declare that the research was conducted in the absence of any commercial or financial relationships that could be construed as a potential conflict of interest.
Publisher’s note
All claims expressed in this article are solely those of the authors and do not necessarily represent those of their affiliated organizations, or those of the publisher, the editors and the reviewers. Any product that may be evaluated in this article, or claim that may be made by its manufacturer, is not guaranteed or endorsed by the publisher.
References
Adeluyi, O., Harris, A., Verrelst, J., Foster, T., Clay, G. D. (2021). Estimating the phenological dynamics of irrigated rice leaf area index using the combination of prosail and gaussian process regression. Int. J. Of. Appl. Earth Observation. And. Geoinform. 102, 102454. doi: 10.1016/J.Jag.2021.102454
Bai, W., Bao, X. (2002). Simulation alfalfa growth in wulanbuhe sandy region. Chin. J. Of. Appl. Ecol. 13 (12), 1605–1609.
Baral, R., Lollato, R. P., Bhandari, K., Min, D. (2022). Yield gap analysis of rainfed alfalfa in the United States. Front. In. Plant Sci. 13, 931403. doi: 10.3389/fpls.2022.931403
Castro-Valdecantos, P., Apolo-Apolo, O. E., Pérez-Ruiz, M., Egea, G. (2022). Leaf area index estimations by deep learning models using Rgb images anddata fusion in maize. Precis. Agric. 23 (6), 1949–1966. doi: 10.1007/S11119-022-09940-0
Eardly, B. D., Hannaway, D. B., Bottomley, P. J. (1985). Nitrogen nutrition and yield of seedling alfalfa as affected by ammonium nitrate fertilization. Agron. J. 77 (1), 57–62. doi: 10.2134/agronj1985.00021962007700010014x
Faverjon, L., Escobar-Gutiérrez, A. J., Litrico, I., Louarn, G. (2017). A conserved potential development framework applies to shoots of legume species with contrasting morphogenetic strategies. Front. In. Plant Sci. 8. doi: 10.3389/Fpls.2017.00405
Feng, Y., Shi, Y., Zhao, M., Shen, H., Xu, L., Luo, Y., et al. (2022). Yield and quality properties of alfalfa (Medicago sativa L.) and their influencing factors in China. Eur. J. Of. Agron. 141, 126637. doi: 10.1016/J.Eja.2022.126637
Fink, K. P., Grassini, P., Rocateli, A., Bastos, L. M., Kastens, J., Ryan, L. P., et al. (2022). Alfalfa water productivity and yield gaps in the U.S. central Great Plains. Field Crops Res. 289, 108728. doi: 10.1016/j.fcr.2022.108728
Gao, L., Hannaway, D. (1985). Alfamod: an agroclimatology computer model of alfalfa production. J. Of. Agric. Sci. 1 (2), 1–11.
Gao, L., Su, J., Tian, Q., Shen, Y. (2020). Effects of nitrogen application on nitrogen accumulation and root nitrogenase activity in medicago sativa at different soil water contents. Acta Pratacult. Sin. 29 (3), 130–136. doi: 10.11686/cyxb2019268
Gower, S. T., Kucharik, C. J., Norman, J. M. (1999). Direct and indirect estimation of leaf area index, fapar, and net primary production of terrestrialecosystems. Remote Sens. Of. Environ. 70 (1), 29–51. doi: 10.1016/S0034-4257(99)00056-5
Hammond, K., Kerry, R., Jensen, R. R., Spackman, R., Hulet, A., Hopkins, B. G., et al. (2023). Assessing within-field variation in alfalfa leaf area index using Uav visible vegetation indices. Agronomy 13 (5), 1289. doi: 10.3390/Agronomy13051289
Hui, Z., Jimin, W., Angdong, H. (2021). An analysis of the current supply and demand for feed grains in China. Agecon Search, 215–228. doi: 10.1016/J.Compag.2023.107775
Jin, X., Li, Z., Feng, H., Ren, Z., Li, S. (2020). Deep neural network algorithm for estimating maize biomass based on simulated sentinel 2a vegetation indices and leaf area index. Crop J. 8 (1), 87–97. doi: 10.1016/J.Cj.2019.06.005
Laroche, J. P., Gervais, R., Lapierre, H., Ouellet, D. R., Tremblay, G. F., Halde, C., et al. (2022). Milk production and efficiency of utilization of nitrogen, metabolizable protein, and amino acids are affected by protein and energy supplies in dairy cows fed alfalfa-based diets. J. Dairy. Sci. 105 (1), 329–346. doi: 10.3168/jds.2021-20923
Linge, S., Langtangen, H. P. (2020). Programming for computations-python: a gentle introduction to numerical simulations with python 3.6 (Berlin, Germany: Springer Nature), 332. doi: 10.1007/978-3-030-16877-3
Liu, M., Wang, Z., Mu, L., Xu, R., Yang, H. (2021). Effect of regulated deficit irrigation on alfalfa performance under two irrigation systems in the inland arid area of midwestern China. Agric. Water Manage. 248, 106764. doi: 10.1016/J.Agwat.2021.106764
Liu, M., Wu, X., Yang, H. (2022). Evapotranspiration characteristics and soil water balance of alfalfa grasslands under regulated deficit irrigation in the inland arid area of midwestern China. Agric. Water Manage. 260, 107316. doi: 10.1016/J.Agwat.2021.107316
Lv, Y., Wang, J., Yin, M., Kang, Y., Ma, Y., Jia, Q., et al. (2023). Effects of planting and nitrogen application patterns on alfalfa yield, quality, water–nitrogen use efficiency, and economic benefits in the yellow river irrigation region of Gansu Province, China. Water 15 (2), 251. doi: 10.3390/W15020251
Ma, J., Huangfu, W., Yang, X., Xu, J., Zhang, Y., Wang, Z., et al. (2022). “King of the forage”—Alfalfa supplementation improves growth, reproductive performance, health condition and meat quality of pigs. Front. Vet. Sci. 9. doi: 10.3389/fvets.2022.1025942
Moghaddam, A., Mofidian, S. M. A. (2022). Comparison of forage yield and leaf area index of some local and foreign alfalfa (Medicago sativa L.) genotypes. Iranian. J. Of. Rangelands. And. Forests. Plant Breed. And. Genet. Res. 29 (2), 329–317. doi: 10.22092/Ijrfpbgr.2022.356780.1398
Motsinger, L. A. (2022). Effects of providing novel feedstuffs to livestock on production and skeletal muscle growth (Doctoral dissertation, Utah: Utah State University). doi: 10.3389/fmicb.2022.1056315
Ojaghlou, H., Ojaghlou, F., Jafari, M. M., Misaghi, F., Nazari, B., Karamidehkordi, E. (2023). Effect of irrigation management on water productivity indicators of alfalfa. Water And. Soil 37 (2), 165–185. doi: 10.22067/Jsw.2023.79145.1211
Peters, E., Stritzke, J. (1970). Herbicides and nitrogen fertilizer for the establishment of three varieties of spring-sown alfalfa. Agron. J. 62 (2), 259–262. doi: 10.2134/agronj1970.00021962006200020025x
Qi, H., Zhu, B., Wu, Z., Liang, Y., Li, J., Wang, L., et al. (2020). Estimation of peanut leaf area index from unmanned aerial vehicle multispectral images. Sensors 20 (23), 6732. doi: 10.3390/S20236732
Qian, Z. H., Han, B. F., Liu, Z. T., Cai, W., Fu, B. Z., Ma, H. B. (2020). Effects of infiltration irrigation on growth characters and water use efficiency of alfalfa in the ningxia yellow river diversion irrigation area. Acta Pratacult. Sin. 29 (3), 147. doi: 10.11686/Cyxb2019254
Su, Y., Zhang, L., Sun, Q., Wu, Y., Yang, X., Lu, N. (2011). Effects of variable water and nutrient regimes on nutrients of alfalfa. Acta Agrestia. Sin. 19 (5), 821–824. doi: 10.11733/j.issn.1007-0435.2011.05.018
Sun, Z., Wang, Y., Ji, S., Wang, K., Zhao, Y. (2015). Computer‐aided analysis with Image J for quantitatively assessing psoriatic lesion area. Skin Research and Technology 21 (4), 437–443. doi: 10.1111/srt.12211
Sun, H., Liu, G., Zhang, Y., Gao, F., Lu, T., Han, J. (2005). Water requirement, water consumption, water requirement rate, water consumption rate and water use efficiency of alfalfa. Pratacult. Sci. 22 (12), 24–30.
Tian, H., Wang, P., Tansey, K., Zhang, J., Zhang, S., Li, H. (2021). An lstm neural network for improving wheat yield estimates by integrating remote sensing data and meteorological data in the Guanzhong plain, pr China. Agric. And. For. Meteorol. 310, 108629. doi: 10.1016/J.Agrformet.2021.108629
Wan, W., Li, Y., Li, H. (2022). Yield and quality of alfalfa (Medicago sativa L.) in response to fertilizer application in China: A meta-analysis. Front. Plant Sci. 13. doi: 10.3389/fpls.2022.1051725
Wang, E., He, D., Wang, J., Lilley, J. M., Christy, B., Hoffmann, M. P., et al. (2022). How reliable are current crop models for simulating growth and seed yield of canola across global sites and under future climate change? Climatic. Change 172 (1-2), 20. doi: 10.1007/S10584-022-03375-2
Wang, Y., Liu, C., Cui, P., Su, D. (2021). Effects of partial root-zone drying on alfalfa growth, yield and quality under subsurface drip irrigation. Agric. Water Manage. 245, 106608. doi: 10.1016/J.Agwat.2020.06608
Wang, X., Shen, L., Liu, T., Wei, W., Zhang, S., Tuerti, T., et al. (2023). Juvenile plumcot tree can improve fruit quality and economic benefits by intercropping with alfalfa in semi-arid areas. Agric. Syst. 205, 103590. doi: 10.1016/J.Agsy.2022.103590
Wang, B., Yang, Y. Q., Li, M. Y., Ni, W., Hai, Y. R., Zhang, S. X., et al. (2022). The effect of sowing rate and row spacing on the yield and quality of alfalfa in the ningxia yellow river irrigation area. Acta Pratacult. Sin. 31 (2), 147. doi: 10.11686/Cyxb2021236
Zhang, J., Cheng, T., Guo, W., Xu, X., Qiao, H., Xie, Y., et al. (2021). Leaf area index estimation model for Uav image hyperspectral data based on wavelength variable selection and machine learning methods. Plant Methods 17 (1), 1–14. doi: 10.1186/S13077-021-00750-5
Keywords: leaf area estimation, leaf area index prediction, meteorological data, soil moisture data, alfalfa, deep learning
Citation: Yang S, Ge Y, Wang J, Liu R, Tang D, Li A and Zhu Z (2024) A dataset for estimating alfalfa leaf area and predicting leaf area index. Front. Plant Sci. 15:1290920. doi: 10.3389/fpls.2024.1290920
Received: 08 September 2023; Accepted: 24 January 2024;
Published: 13 February 2024.
Edited by:
Yuanyuan Zha, Wuhan University, ChinaReviewed by:
Georgios Tsaniklidis, Hellenic Agricultural Organization – ELGO, GreeceRudra Baral, University of Missouri, United States
Copyright © 2024 Yang, Ge, Wang, Liu, Tang, Li and Zhu. This is an open-access article distributed under the terms of the Creative Commons Attribution License (CC BY). The use, distribution or reproduction in other forums is permitted, provided the original author(s) and the copyright owner(s) are credited and that the original publication in this journal is cited, in accordance with accepted academic practice. No use, distribution or reproduction is permitted which does not comply with these terms.
*Correspondence: Yongqi Ge, Z2V5b25ncWlAbnh1LmVkdS5jbg==