- 1Department of Biological Sciences, The University of North Carolina at Charlotte, Charlotte, NC, United States
- 2Key Laboratory of Plant Resources Conservation and Sustainable Utilization, South China Botanical Garden, Chinese Academy of Sciences, Guangzhou, China
- 3Center of Conservation Biology, Core Botanical Gardens, Chinese Academy of Sciences, Guangzhou, China
- 4David H. Murdock Research Institute, Kannapolis, NC, United States
- 5Department of Bioinformatics and Genomics, The University of North Carolina at Charlotte, Charlotte, NC, United States
Glyceollins, a family of phytoalexins elicited in legume species, play crucial roles in environmental stress response (e.g., defending against pathogens) and human health. However, little is known about the genetic basis of glyceollin elicitation. In the present study, we employed a metabolite-based genome-wide association (mGWA) approach to identify candidate genes involved in glyceollin elicitation in genetically diverse and understudied wild soybeans subjected to soybean cyst nematode. In total, eight SNPs on chromosomes 3, 9, 13, 15, and 20 showed significant associations with glyceollin elicitation. Six genes fell into two gene clusters that encode glycosyltransferases in the phenylpropanoid pathway and were physically close to one of the significant SNPs (ss715603454) on chromosome 9. Additionally, transcription factors (TFs) genes such as MYB and WRKY were also found as promising candidate genes within close linkage to significant SNPs on chromosome 9. Notably, four significant SNPs on chromosome 9 show epistasis and a strong signal for selection. The findings describe the genetic foundation of glyceollin biosynthesis in wild soybeans; the identified genes are predicted to play a significant role in glyceollin elicitation regulation in wild soybeans. Additionally, how the epistatic interactions and selection influence glyceollin variation in natural populations deserves further investigation to elucidate the molecular mechanism of glyceollin biosynthesis.
1 Introduction
Plants synthesize a wide array of specialized metabolites, also referred to as secondary metabolites or phytochemicals. These compounds play crucial roles in facilitating plant adaptation to dynamic environments, ensuring survival, and presenting potential applications for human use (Ahmed and Kovinich, 2021). Phytoalexins are specialized metabolites synthesized de novo in response to various biotic and abiotic stresses. Examples include indole alkaloid camalexin in Arabidopsis, phenolic aldehyde gossypol in cotton, phenylpropanoid stilbenes in grapevines, isoflavonoid-derived glyceollins in legume, and momilactones and phytocassanes terpenoids in rice (Jeandet et al., 2002; Wang et al., 2009; Donnez et al., 2011; Saga et al., 2012; Yamamura et al., 2015; Jahan et al., 2019; Jeandet et al., 2020). Among these phytoalexins, isoflavonoids have been of research interest due to the various pharmacological properties and essential roles in plant defense (Dixon and Steele, 1999). The major isoflavones identified in soybeans are comprised of genistein, daidzein, and glycitein (Murphy et al., 2002). It has been reported that trace amounts of glyceollins are produced transiently from daidzein under the influence of both abiotic and biotic stresses. This observation suggests that the production of glyceollins, to a significant extent, is contingent upon external stress factors (Subramanian et al., 2006; Aisyah et al., 2013; Lygin et al., 2013; Bamji and Corbitt, 2017; Jahan and Kovinich, 2019; Jahan et al., 2019). In this regard, producing glyceollins contributes to multiple beneficial effects, such as fostering symbiosis between soybean and Bradyrhizobium japonicum and inhibiting the growth of various microbes (Graham and Graham, 1996; Subramanian et al., 2006). Moreover, they have properties that are beneficial to human health, such as anti-cancer, antioxidant, and neuroprotective (Kim et al., 2012; Nwachukwu et al., 2013; Bamji & Corbitt, 2017; Seo et al., 2018; Pham et al., 2019). However, studies on glyceollins are mainly focused on their medicinal properties, and to the best of our knowledge, little is known about how their elicitation is regulated.
To date, few genes have been identified associated with glyceollin biosynthesis. For example, two key transcription factors, known as GmNAC42-1 and GmMYB29A2, were identified play a crucial role in the biosynthesis of glyceollin I in soybeans, and they contribute to resistance against Phytophthora sojae (Jahan et al., 2019; Jahan et al., 2020). In a study conducted by Jahan and colleagues in 2019, acidity stress was employed to elicit the biosynthesis of glyceollin. They observed that the overexpression of GmNAC42-1 in hairy roots resulted in a remarkable increase of over 10-fold in glyceollin production. The NAC-family transcription factor GmNAC42-1 plays a crucial role in regulating certain glyceollin biosynthesis genes, though not all. This suggests that there is still unidentified essential transcription factor(s) within the glyceollin gene regulatory network (Jahan et al., 2019). In a separate investigation conducted by Jahan and colleagues in 2020, it was revealed that upon stimulation with wall glucan from P. sojae, GmMYB29A2 interacted with the promoters of two glyceollin I biosynthesis genes in vitro and in vivo. This interaction led to the accumulation of glyceollin I and the expression of resistance against Phytophthora (Jahan et al., 2020). Given that glyceollins are produced in trace amounts and transiently under stress conditions, finely adjusting these transcription factors emerges as a promising strategy to enhance their production efficiently.
Phytoalexins have been considered the target of natural selection due to their activities in biotic and abiotic stress responses in natural environments (Pichersky and Gang, 2000; Qi et al., 2004; Miyamoto et al., 2016). Research has shown that genomic approaches in crop wild relatives can reveal genes responsible for target metabolites (Zhang et al., 2017b). Improvements can be achieved by manipulating the metabolic pathway in crops. Examples of this phenomenon include 7-epizingiberene synthase (ShZIS), a sesquiterpene synthase specific to trichomes that is involved in the naturally optimized sesquiterpene biosynthetic pathway in wild tomatoes. This enzyme enhances cultivated tomato resistance against various herbivores when subjected to genetic engineering (Bleeker et al., 2012). Mipeshwaree Devi et al. (2023) have comprehensively summarized recent advancements in the realm of metabolic engineering, specifically focusing on plant-specialized metabolites. Notably, Zhang and colleagues (2022) employed CRISPR/Cas9 for targeted mutagenesis in GmUGT, a UDP-glycosyltransferase pivotal in flavonoid biosynthesis. This targeted mutagenesis resulted in enhanced resistance against leaf-chewing insects (Zhang et al., 2022). Therefore, understanding the metabolic pathways and their regulatory mechanisms is essential for targeted metabolite engineering to achieve crop improvement. However, there is limited reported progress in the field of metabolic engineering (Mipeshwaree Devi et al., 2023).
Furthermore, the study of metabolic gene clusters, which are groups of co-localized and potentially coregulated non-homologous genes involved in specific metabolic pathways, has gained attention (Nützmann et al., 2016; Töpfer et al., 2017). While these clusters have long been observed in microbial genetics, their existence in plant metabolic pathways has only recently been explored (Zheng et al., 2002; Rocha, 2008; Koonin, 2009). A study by Chae et al. (2014) focusing on metabolic gene clusters in Arabidopsis, soybean, sorghum, and rice suggested that approximately one-third of all the metabolic genes in Arabidopsis, soybean, and sorghum, and one-fifth in rice were rich in gene clusters across primary and specialized metabolic pathways (Chae et al., 2014). There is compelling evidence indicating that the highly plastic plant genome itself generates metabolic gene clusters via gene duplication, neofunctionalization, divergence, and genome reorganization instead of horizontal gene transfer from microbes (Osbourn and Field, 2009). This suggests that plants rewire their genome to gain new adaptive functions driven by the need to survive in distinct environments. Mining and functional validation of the candidate genes in such clusters will facilitate the discovery of new enzymes and chemistries that render pathway prediction. Moreover, metabolic gene clusters are likely to be located within dynamic chromosomal regions, and thus, many identified so far may be due to recent evolution (Qi et al., 2004; Field et al., 2011; Matsuba et al., 2013). If so, investigation of these clusters can provide insights into their evolutionary history. The vast and diverse array of specialized metabolites produced through multi-step metabolic pathways plays an essential role in plant adaptation to various ecological niches. However, the occurrence, prevalence, and evolution of such gene clusters in plants are largely unknown. Thus, the study of plant metabolic gene clusters has implications for molecular biology and evolutionary genomics (Yeaman and Whitlock, 2011; Takos and Rook, 2012; Nützmann et al., 2016; Chavali and Rhee, 2018).
To the best of our knowledge, due to the extraordinary metabolic diversity, less than 50 plant-specialized metabolic pathways have been biochemically and genetically identified to date (Nützmann et al., 2016). Metabolomic GWAS (mGWAS) offers an effective approach to understanding the genetic basis of metabolites and their associated traits (Chan et al., 2010; Chan et al., 2011; Riedelsheimer et al., 2012; Luo, 2015). mGWAS allows the identification of common polymorphic regions controlling complex metabolic traits by substantially increasing association panel and genome-wide molecular markers. Besides elucidating genetic architecture, mGWAS can also be used to infer gene functions (Luo, 2015). Hence, mGWAS provides a comprehensive approach to discovering candidate genes. Thus far, it has been used to uncover the genetic basis of variations of a number of different metabolites. For example, Chen et al. (2014) carried out a rice mGWAS study that identified 36 candidate genes influencing the variation of metabolites with physiological and nutritional importance (Chen et al., 2014). Additionally, Petersen et al. (2012) illustrated that in an association study (i.e., mGWAS), a ratio between two metabolite concentrations provides more insightful information than the concentrations of the two metabolites individually. Implementing this innovative approach in mGWAS proves to be valuable for revealing novel and biologically significant associations. They emphasized several studies in which the incorporation of metabolite ratios in both genome-wide and metabolite-wide association studies significantly strengthened the associations (Petersen et al., 2012). For instance, Gieger et al. (2008); Illig et al. (2010), and Suhre et al. (2011) illustrated that the utilization of metabolite ratios in GWAS studies resulted in a substantial increase in the power of association, reaching tens of orders of magnitude (Gieger et al., 2008; Illig et al., 2010; Suhre et al., 2011).
The isoflavonoid pathway has been relatively well studied (Yoneyama et al., 2016; Sukumaran et al., 2018). However, a gap in our understanding of the genetic basis of glyceollin elicitation remains. As of now, researchers have identified transcription factors crucial for the regulation of glyceollin biosynthesis, such as GmNAC42-1 and GmMYB29A2 (Jahan et al., 2019; Jahan et al., 2020). In the present study, we selected wild soybean (Glycine soja), a wild relative of soybean (Glycine max), to delineate the genetic basis and evolution of glyceollin accumulation resulting from biotic stress, i.e., soybean cyst nematode (SCN), the most devastating soybean pest worldwide (Tylka and Marett, 2021). Wild soybeans thrive in diverse habitats and harbor much higher, underexplored genetic diversity than cultivated soybeans (Zhang et al., 2019). Hence, it is an ideal system to understand the genetic basis and evolution of glyceollin variation. Eventually, the essential genes identified in wild soybeans can be used for metabolic engineering or in a breeding program to develop nutrition-rich biofortified soybean cultivars as they exhibit similar genome size and content with no reproductive barriers (Singh and Hymowitz, 1999). In this study, we aim to address these three questions: (1) What is the genetic basis of variation in glyceollin elicitation by SCN? (2) Are there any gene clusters and transcription factors involved in glyceollin variation? (3) Are epistatic interactions and natural selection important evolutionary factors influencing the variation of glyceollin elicitation in natural populations? Our study is the first to employ genomic and evolutionary approaches to understand the genetic basis and selection of glyceollin elicitation. The results provide a fundamental basis for the long-term goal of developing glyceollin-fortified soybean cultivars.
2 Materials and methods
2.1 Plant materials
A total of 265 accessions of wild soybean, Glycine soja, from a wide geographic range, originally collected from China, Japan, Russia, and South Korea, were utilized (Supplementary Table 1). The seeds of these ecotypes were obtained from the USDA National Germplasm resources laboratory (https://www.ars-grin.gov/).
2.2 Plant preparation, SCN inoculation, and sample collection
Seed preparation, germination, transplanting, and soybean cyst nematode (SCN, Heterodera glycines Ichinohe, HG type 1.2.5.7) inoculation were performed following a previously developed protocol (Zhang and Song, 2017; Zhang et al., 2017a). Specifically, each wild soybean ecotype seed underwent surface sterilization using a 0.5% sodium hypochlorite solution for one minute, followed by thorough rinsing. These sterilized seeds were then germinated on sterile filter paper in petri dishes containing an appropriate amount of sterile water for a duration of 3 to 4 d. Once germinated, it was transplanted into a cone-tainer (Greenhouse Megastore, Danville, IL, USA), utilizing sterile sand as the growth medium. The arrangement of cone-tainers in a cone-tainer tray (Greenhouse Megastore, Danville, IL, USA) followed a randomized complete block design. To ensure optimal growth conditions, all the plants were kept within a growth chamber maintained at a temperature of 27°C, with a relative humidity of 50%, and subjected to a long-day photoperiod of 16 h of light followed by 8 h of darkness. The seedlings received regular daily watering to maintain adequate moisture levels for healthy growth.
For SCN inoculation, the HG type 1.2.5.7 nematodes stocks were maintained in a controlled greenhouse environment, with a consistent temperature of 27°C and a photoperiod of 16 h of light followed by 8 hof darkness, spanning over 30 generations. To isolate female nematodes, they were carefully extracted from the roots of soybean cv. Hutcheson by gently massaging the roots in water and then filtering the solution through nested sieves with mesh sizes of 850 and 250 micrometers. The collected female nematodes were then crushed using a rubber stopper in an 8-inch diameter sieve with a 250-micrometer mesh, releasing the eggs, which were subsequently collected using a 25-micrometer mesh sieve. For further purification, the eggs underwent a modified sucrose flotation method (Matthews et al., 2003).
Following purification, the eggs were placed on moist paper tissues and placed in a plastic tray filled with 1 centimeter of water. The tray was covered with aluminum foil and maintained at a temperature of 27°C. Three days after hatching, the second-stage juvenile nematodes (referred to as J2) were harvested and concentrated to achieve a final concentration of 1,800 J2 per milliliter in a 0.09% agarose suspension. After three days of transplantation, when the seedlings were healthy and displayed uniform growth, they were inoculated with 1 milliliter of the J2 nematode inoculum. Concurrently, seedlings inoculated with a 0.09% agarose solution served as the control group.
Whole root tissues were collected and weighed five days post-infection (dpi). The 5 dpi time point was chosen because our previous study suggested a significant inhibition in SCN development in a resistant genotype compared to normal growth in a susceptible genotype (Zhang et al., 2017a). All samples were flash-frozen in liquid nitrogen and stored at -80°C. Four biological replicates per wild soybean genotype were used, eventually a total of 1,020 samples.
2.3 Metabolite extraction and quantification
We employed the extraction method of metabolites from root tissue described in Strauch et al. (2015) (Strauch et al., 2015). The soybean root samples underwent homogenization within a ball mill homogenizer, utilizing an extraction solvent that featured daidzein-d6 (Biotek, catalog#BT-387818) as an internal standard. The metabolite profiling was provided by the service from David H. Murdock Research Institute at the North Carolina Research Campus employing UPLC-MS/MS (ultraperformance liquid chromatography-tandem mass spectrometry). Method development and analysis were conducted using a Waters ACQUITY UPLC-Quattro Premier XE MS. The UPLC and MS/MS parameters were established through experimentation with test samples and analytical standards of glyceollin (chemically synthesized by Dr. P. Erhardt at University of Toledo), daidzein (Sigma Aldrich, catalog#D7802), and daidzein-d6 (LGCstandards). The MS/MS acquisition parameters were optimized based on the analytical standards. Additionally, optimized UPLC gradient conditions were determined to effectively separate the glyceollin and daidzein peaks. Peaks that were consistently detected in at least three biological replicates within each genotype were used for downstream analyses. Each metabolite was confirmed using pure standard compounds, including daidzein, daidzein-d6, and glyceollin. Due to the low concentrations of these compounds and the small sample masses of the wild soybean root samples that had been collected, we used a signal-to-noise ratio of ≥10 for the measurement of the peaks for glyceollin and daidzein. Our method successfully measured daidzein (μg/g root) and glyceollin (unitless) in 264 accessions of wild soybean G. soja roots quantitatively and semi-quantitatively, respectively. Following method development, optimization, and analyses of the test samples, calibration curves were designed using at least six different concentrations of daidzein, created in triplicate to quantify known concentrations of daidzein and glyceollin. A second-degree polynomial was derived from the known concentrations of the standard curve samples and the mass spectrometer response (daidzein/internal standard) from the standard curve data. The resulting polynomial was used to calculate the concentrations of daidzein in the experimental samples. Low, medium, and high QC (quality control) samples were created to assess the accuracy of the calculations. We used the ratio of glyceollin (unitless, a semi-quantitative measurement of glyceollin) to daidzein (μg/g root) (GVSD) as our phenotypic trait (Supplementary Table 1 and Supplementary Figure 2). This phenotype henceforth is denoted GVSD. The justification for employing the ratio is to enhance statistical power by minimizing variability in the metabolomic data and mitigating experimental errors associated with data noise (Petersen et al., 2012).
2.4 Genotypic data
Genotype data for the 264 accessions were obtained from SoySNP50K (Song et al., 2013), which was downloaded from SoyBase (SoyBase.org). After the filter, the genotype included 32,976 genome-wide single nucleotide polymorphic markers (SNPs) with a minor allele frequency (MAF) of at least 5% and a missingness rate of less than 10%.
2.5 Metabolite-based genome-wide association study and linkage disequilibrium estimation
Our genome-wide association analysis was conducted on GVSD (a ratio of glyceollin mean to daidzein mean) in response to SCN infection on all 264 ecotypes using the GAPIT R package (2.0) (Tang et al., 2016). To minimize false-positive associations, we controlled population structure among genotypes with four principal components as calculated with the GAPIT. Heritability estimate and SNP effect were calculated by running GWAS applying CMLM and MLM methods, respectively, implemented in the GAPIT R package (2.0) (Tang et al., 2016).
The Manhattan plot was generated using the R package qqman (Turner, 2018). In addition to the genome-wide significant threshold, we also calculated the chromosome-wide Bonferroni thresholds using independent SNPs estimated on each chromosome following the method of Li and Ji (2005) (Li and Ji, 2005). Linkage disequilibrium (LD) was calculated across the panel with the TASSEL program, version 5 (Bradbury et al., 2007), for the significant SNPs identified from the GWAS analysis. LD was measured using squared correlation R-squared (r2) of 0.2 (upper right in the LD plot) and p-value < 0.05 (the lower left in the LD plot). A pairwise LD was generated following the R function described by Shin et al. (2006) (Shin et al., 2006). Genes within LD blocks containing significant SNPs were identified as potential sources of candidates for further analyses.
2.6 Identification of candidate genes
For extensive gene mining, a pairwise linkage disequilibrium (LD) analysis was initially used for potential candidate gene identification. Then, genes in each LD block were examined as potential candidate genes, and their annotations were obtained from the Phytozome v13 database (Goodstein et al., 2011). Afterward, a GO enrichment analysis of the identified candidate genes was performed using ShinyGO v0.66: Gene Ontology Enrichment Analysis (p-value cutoff (FDR, false discovery rate) = 0.05) (Ge et al., 2020), SoyBase GO Enrichment Data (Grant et al., 2010). To investigate the involvement of these potential candidate genes in metabolic pathways, a database search was performed through an annotation file from Phytozome v13 (Goodstein et al., 2011), SoyBase (Grant et al., 2010), SoyCyc 10.0 Soybean Metabolic Pathway (Hawkins et al., 2021), and Pathview databases (Luo et al., 2017). Finally, a PMN plant metabolic cluster viewer was applied to categorize enzymes into classes (signature or tailoring) and metabolic domains (Hawkins et al., 2021).
2.7 Analysis of epistatic interactions
For any significant SNPs uncovered in the GWAS analysis, it is useful to test whether, beyond their direct effects, they also exhibited interactive effects on GVSD. To accomplish this, we first produced numerically formatted genotypes, in which the homozygous genotype index value is 1 and -1 and the heterozygous 0. This allows us to test for epistasis for each pairwise combination in a simple general linear model with 1 degree of freedom for the additive effects of each of the two SNPs and their interaction. We included the first four principal components from the GAPIT analysis in the model to be consistent with the GWAS scan, where these components were used to adjust for structural relatedness (see below). The significance of all interactions was evaluated with the sequential Bonferroni procedure. To illustrate the interactions of SNP pairs, we also calculated regressions of GVSD on each SNP, but at each of the three genotypes (using the -1, 0, and 1 index values) of the second SNP involved in the significant interaction.
2.8 Extended haplotype homozygosity analysis
To test allele-specific selection patterns of the identified significant SNPs, we analyzed extended haplotype homozygosity (EHH, (Sabeti et al., 2002)) for each significant SNP. The EHH analysis was conducted in SELSCAN v.1.2.0a (Szpiech and Hernandez, 2014) with default parameters, and only SNPs with MAF > 0.05 was used in this analysis.
3 Results
3.1 Genomic dissection of glyceollin accumulation upon biotic elicitation
To investigate the genetic basis of glyceollin elicitation, we performed a metabolite-based genome-wide association study (mGWAS) of glyceollin content in wild soybean roots infected with soybean cyst nematode (SCN). The mGWAS identified a total of eight significant SNPs, with four (ss715603454, ss715603455, ss715603462, and ss715603471) located on chromosome 9 and ss715585948, ss715615975, ss715620269 and ss715636844 on chromosomes 3, 13, 15, and 20, respectively (Figure 1A; Table 1). These significant SNPs were identified based on both genome-wide Bonferroni threshold of 5.104 and chromosome-wide Bonferroni thresholds that varied narrowly from 3.79 to 3.82 among the 20 chromosomes (3.803 on chromosome 9) (Figures 1A, B; Supplementary Table 2). The mGWAS are visualized with the Manhattan and Q-Q (quantile-quantile) plots as shown in Figure 1. The four significant SNPs ss715603454, ss715603455, ss715603462, and ss715603471 on chromosome 9 at positions 30262482, 30191235, 30393285, and 30725658, respectively, are located closely to each other within a 535-kb genomic region (Supplementary Table 2). The heritability for glyceollin was estimated at 35%, suggesting that glyceollin elicitation was genetically controlled (Supplementary Table 2).
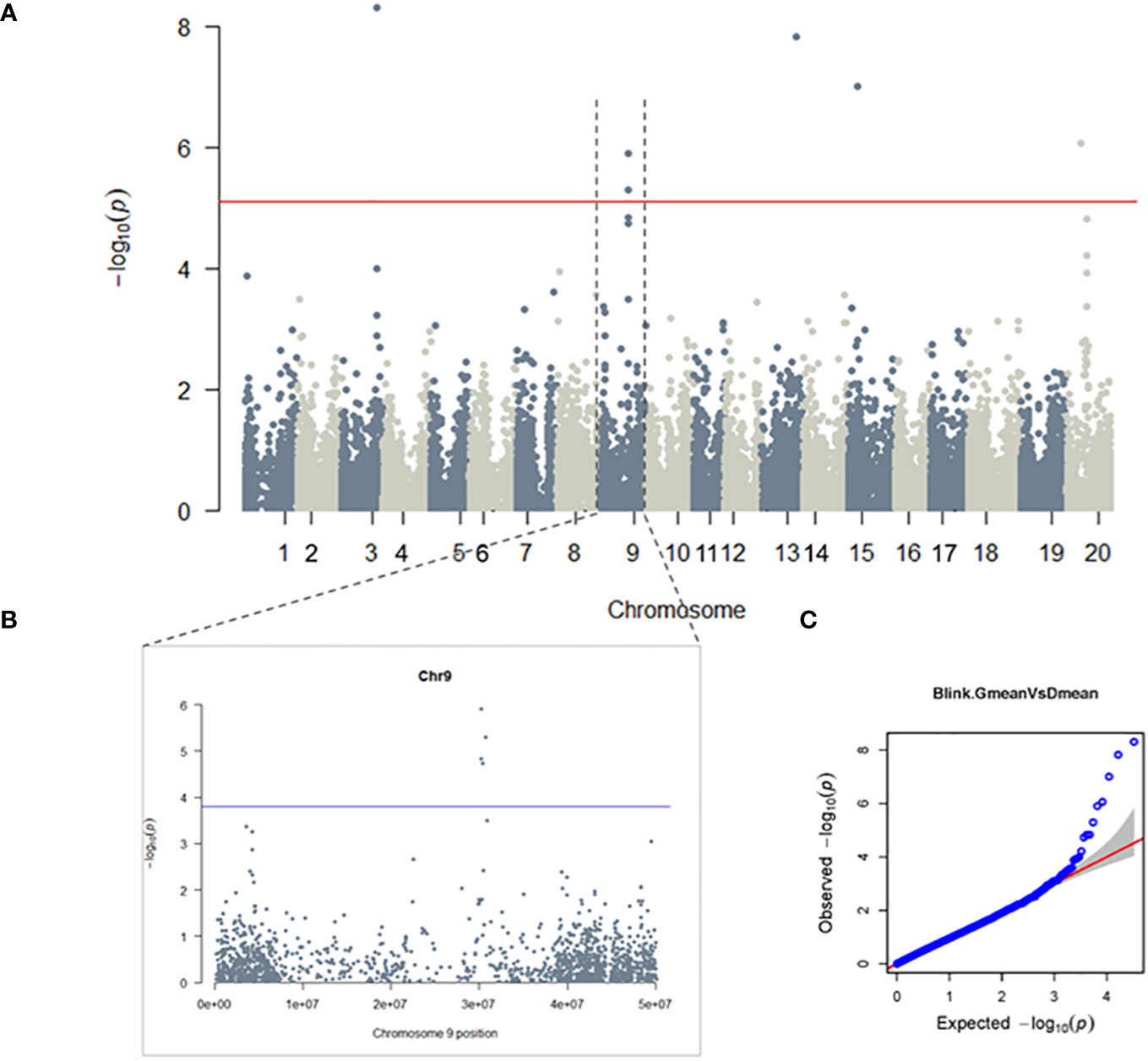
Figure 1 GWAS of Glyceollin elicitation with SCN stress: A genome-wide (A) and chromosome-wide (B) Manhattan plots, with thresholds of 5.104 and 3.803, respectively; (C) quantile-quantile (QQ) plot. Significant SNPs are found on chromosomes 3, 9, 13, 15 and 20 at a 5% genome-wide threshold, the probability of 7.86×10-6 resulted in a threshold of 5.01 (solid red line in the genome-wide Manhattan plot) (A). The 5% chromosome-wide LOD threshold resulted in significant p-values of 1.57×10-4 (threshold 3.803, solid blue line) (B).
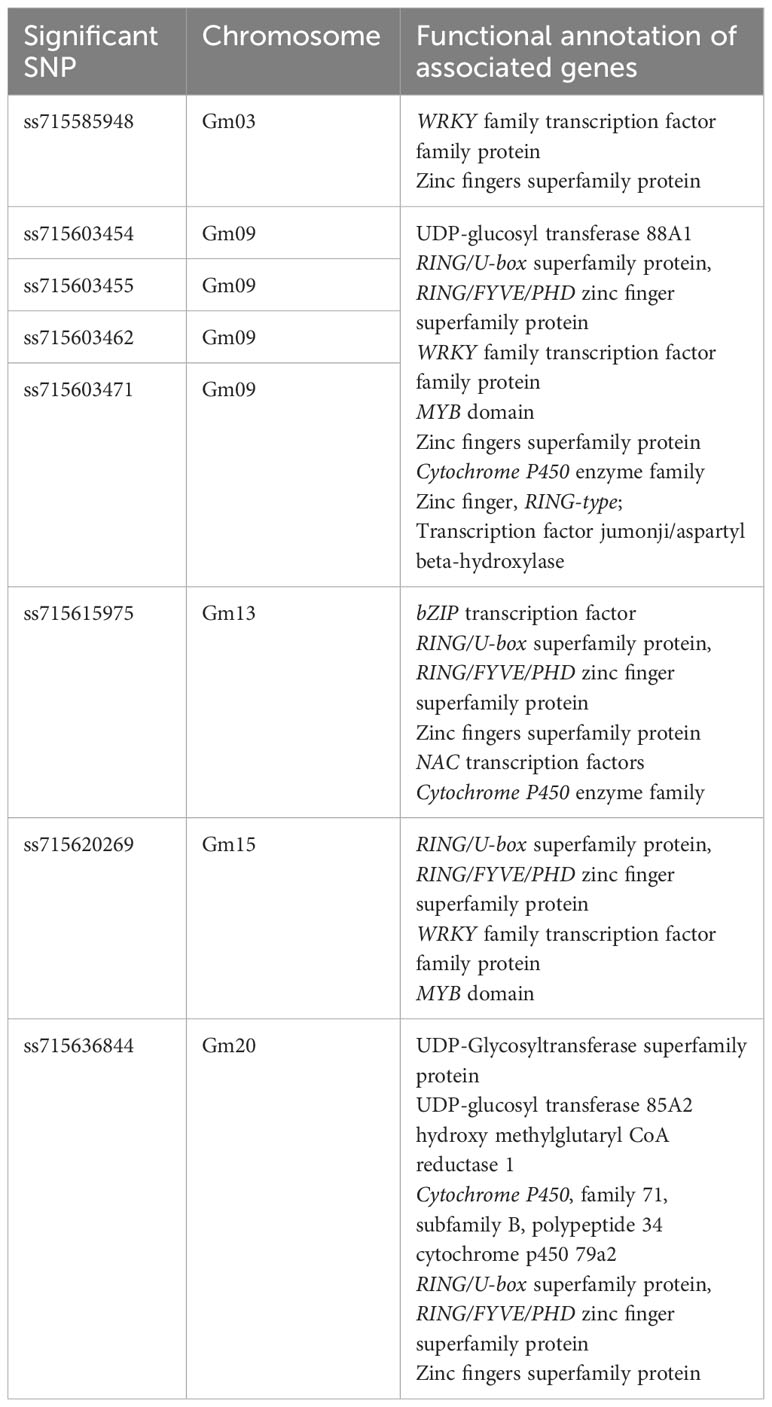
Table 1 Identification of significant SNPs and functional annotation of the plausible candidate genes.
3.2 Candidate gene identification
We employed a pairwise linkage disequilibrium (LD) analysis to identify potential candidate genes. For candidate gene determination, we considered r2>0.2 as a cutoff for our LD analysis, where r2 is the extent of allelic association between a pair of sites (Weir, 1990). Figure 2A shows the LD decay plot in the studied panel. We identified a total of 666 possible candidate genes within either side of 200 kb covering linkage disequilibrium (LD) blocks of the eight significant SNPs (soybean reference genome Glycine max Wm82.a2.v1) (Goodstein et al., 2011; Zhou et al., 2015). Further refining our selection, we narrowed the list to 51 candidate genes, focusing on the eight significant SNPs within the mentioned LD block region. Another criterion for this selection was the alignment with our pathway of interest, demonstrating a strong correlation with the target metabolites (Supplementary Table 3). The LD block within either side of the 200 kb region on chromosome 9 showed the strongest LD compared to the LD blocks for other significant SNPs identified on chromosomes 3, 13, 15 and 20 (Figure 2B; Supplementary Figure 1). Specifically, the candidate gene Glyma.09G128200 exhibits the highest level of linkage disequilibrium (LD) near the significant SNP ss715603454 on chromosome 9 in comparison to the LD block associated with the remaining significant SNPs on this chromosome (Figure 2B). The functional annotation of the candidate genes on chromosome 9 (i.e., Glyma.09G127700, Glyma.09G128200, Glyma.09G128300, and Glyma.09G128400) within this block is biosynthetic enzymes, mainly glycosyltransferase involved in isoflavonoid pathway, as well as regulatory genes such as WRKY and MYB transcription factors (Table 1; Supplementary Tables 3-5). Their likely role as regulatory genes suggests their potential involvement at the transcriptional level in glyceollin elicitation in response to SCN stress (Colinas and Goossens, 2018).
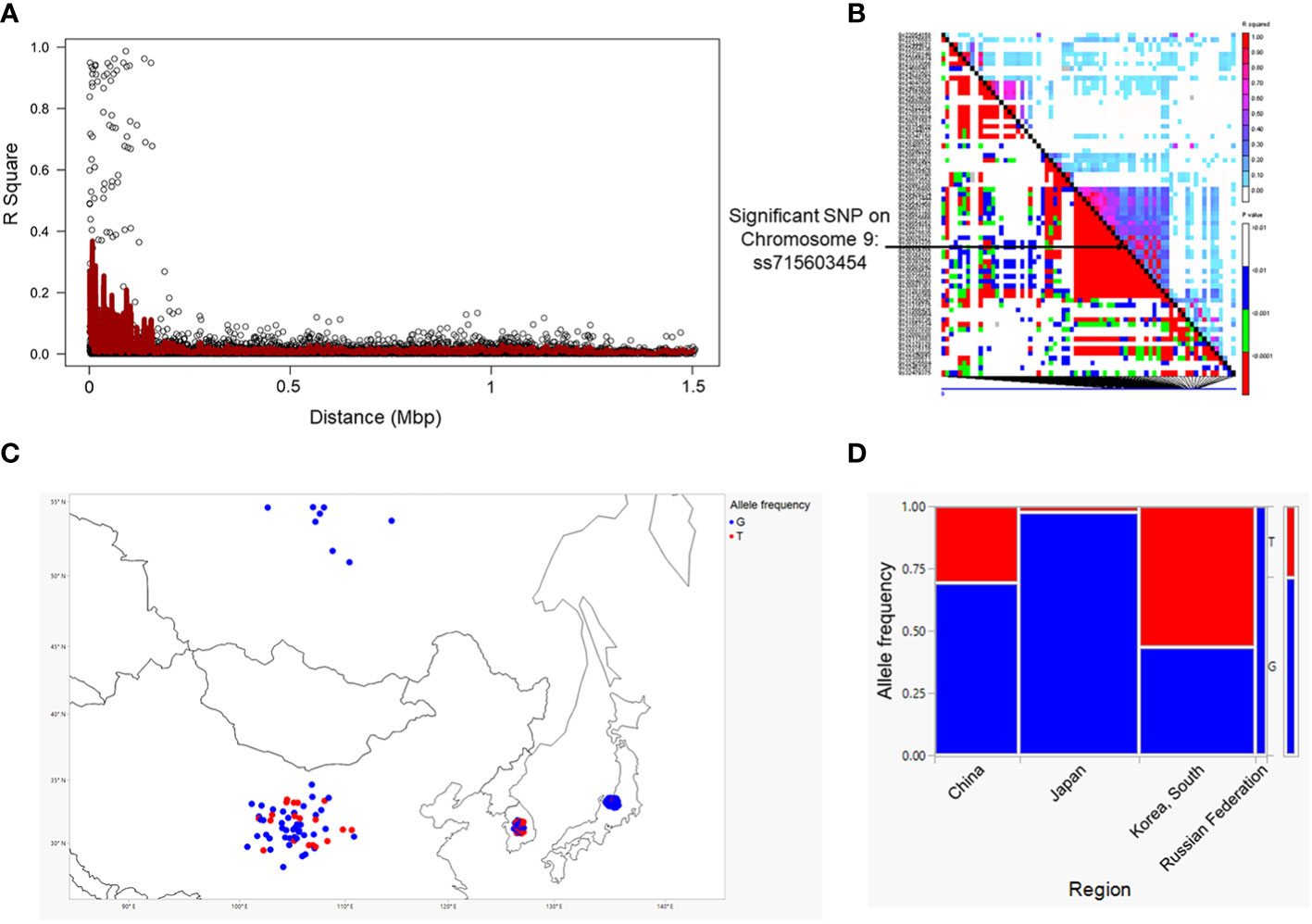
Figure 2 Linkage disequilibrium (LD) decay measured as R2 as a function of marker distance in the studied panel (A) and LD plot for chromosome 9 for significant SNPs. The black diagonal denotes LD between each site and itself (B). Geographic range of the alleles of significant SNPs close to the gene clusters on chromosome 9 (C). Allele frequency in each population. Allele frequency in different geographic regions for a significant SNP was generated using JMP®, Version 15. SAS Institute Inc., Cary, NC, 1989–2021. (D).
We also found putative genes encoding enzymes involved in the specialized metabolic pathways within the LD blocks of the significant SNPs on chromosomes 3, 13, 15, and 20. The enriched GO category includes the phenylpropanoid metabolic process (GO:0055085,GO:0016021,GO:0008308,GO:0006873), linamarin biosynthesis (GO:0055114,GO:0020037,GO:0016705,GO:0005506), and terpenoid biosynthesis (GO:0016829,GO:0010333,GO:0008152,GO:0000287) (Supplementary Table 3). Apart from the biosynthetic enzymes on these chromosomes, we also found transcription factor genes, such as WRKY, MYB, and NAC on chromosomes 3, 9, 13, and 15. For instance, candidate genes within the WRKY family transcription factor group include Glyma.03G176600, Glyma.09G129100, Glyma.09G127100, Glyma.15G139000, and Glyma.15G135600. In the MYB transcription factor category, promising candidate genes include Glyma.09G113000, Glyma.09G113100, and Glyma.15G134100. Additionally, the NAC transcription factors include Glyma.13G274300, Glyma.13G279900, and Glyma.13G280000 as potential candidate genes (Table 1, Supplementary Table 3).
3.3 Metabolic gene clusters identification
We were particularly interested in the candidate genes in the branch from daidzein to glyceollin in the isoflavonoid biosynthesis pathway (Lozovaya et al., 2007). We found that the identified candidate genes on chromosome 9 are clustered together based on our analysis using the PMN plant metabolic cluster viewer, and they fall into two clusters. These two clusters belong to the tailoring enzyme glycosyltransferase within the phenylpropanoid specialized metabolic domain (Supplementary Table 4) (Hawkins et al., 2021). Six genes that belong to these two clusters are within the branch of the isoflavonoid biosynthesis pathway. Two of these six genes, Glyma.09G127200 and Glyma.09G127300, are called cluster 1, while the other four (Glyma.09G127700, Glyma.09G128200, Glyma.09G128300, and Glyma.09G128400) are called cluster 2 (Supplementary Table 4).
Through further investigation of annotation of these candidate genes within the gene clusters (Supplementary Table 5), we found the candidate gene Glyma.09G127200 encodes a glucosyltransferase. Interestingly, the four genes within cluster 2 have a similar functional annotation as Glyma.09G127200 and Glyma.09G127300 in cluster 1, and all these four genes could be isogenes suggesting their origin from genome duplication events (Supplementary Table 5) (Bharadwaj et al., 2021).
3.4 Epistatic interactions among all significant SNPs
The results of the epistasis tests for each of the 28 pairwise combinations of the eight significant SNPs are shown in Table 2. Three probabilities, all associated with the SNP on chromosome 20, were not estimable (Table 2). Among the remaining 25 SNP pairs, 20 show statistical significance. Particularly noticeable is the high significance for all interactions of the SNPs on chromosomes 3, 13, and 15. Three of the six pairs among the four SNPs on chromosome 9, all involving ss715603462, are also statistically significant. In general, therefore, this is evidence for substantial epistasis among these SNPs affecting GVSD.
These epistatic interactions of the SNP pairs are illustrated in Figure 3 for each of the four chosen combinations. For example, in panel A (Figure 3A), it can be seen that regression slopes of GVSD on ss715603454 are close to 0 for ss71585948 CC genotype but are positive for TC and especially TT genotypes. In panel D (Figure 3D), regression slopes of GVSD on ss715603471 are negative for ss715603462 AA and GA genotypes but positive for GG genotypes. With no epistasis, these slopes would be expected to be roughly parallel, but in fact, they diverge considerably from parallelism in these four examples, indicating epistasis.
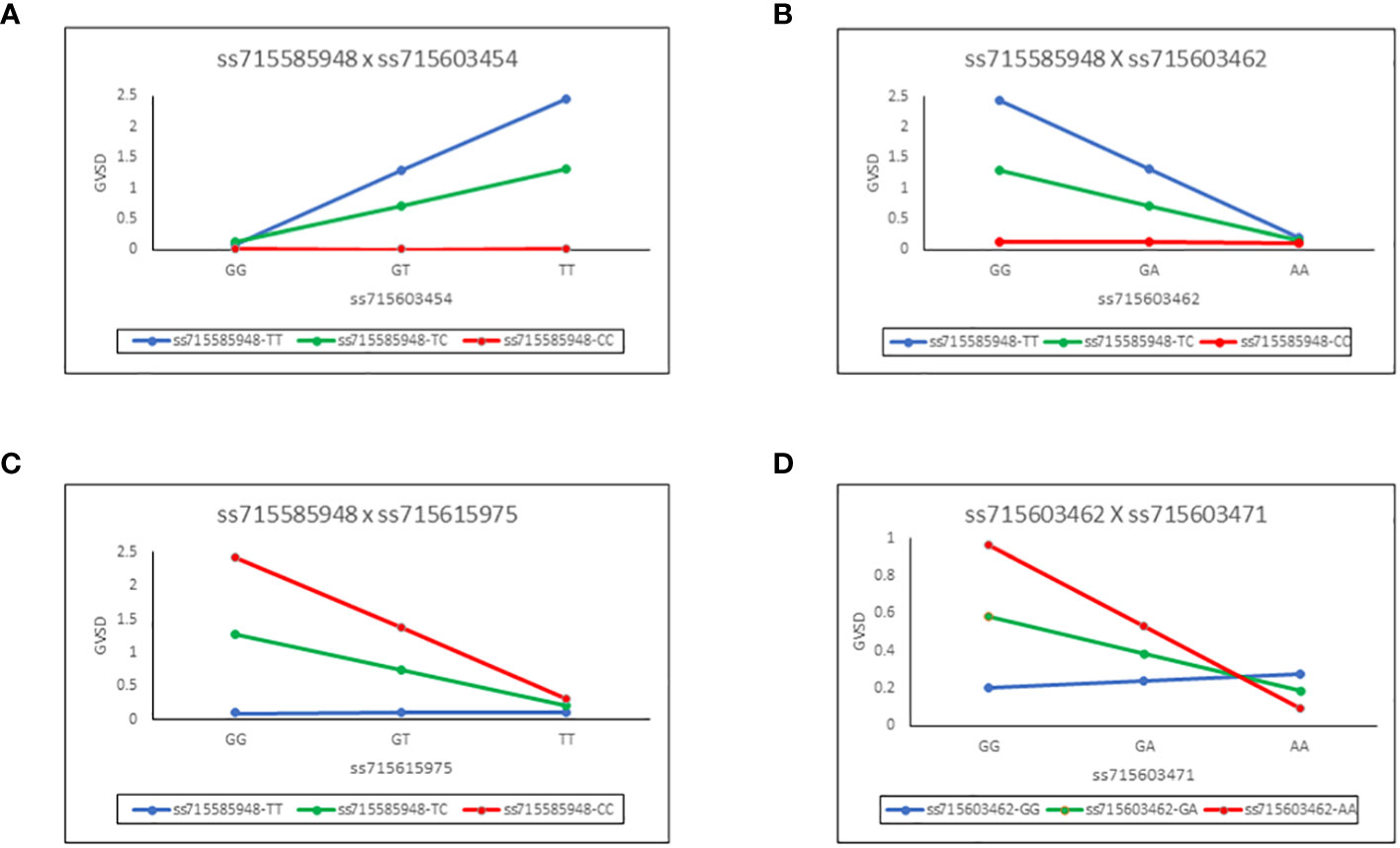
Figure 3 Epistatic interactions of the SNP pairs for each of four chosen combinations. Regression slopes of GVSD on ss715603454 are close to 0 for ss715603454 CC genotypes but are positive for TC and especially TT genotypes (A). Regression slopes of GVSD on ss715603462 are close to 0 for ss715585948 CC genotypes but are negative for TC and especially TT genotypes (B). Regression slopes of GVSD on ss715615975 are close to 0 for ss715585948 TT genotypes but are negative for TC and especially CC genotypes (C). Regression slopes of GVSD on ss715603471 are negative in sign for ss715603462 AA and GA genotypes, but positive in sign for GG genotypes (D).
3.5 Significant SNPs exhibited extended haplotype homozygosity
To examine allele-specific selection patterns associated with the identified significant SNPs, we conducted an analysis of extended haplotype homozygosity (EHH) for each of these SNPs, as proposed by Sabeti et al. (2002) (Sabeti et al., 2002). The extended homozygosity analysis (EHH) analyses revealed allele specific EHH values of the significant SNPs (ss715603454, ss715603455, ss715603462, and ss715603471) on chromosomes 9 (Figure 4). For example, the T allele of ss715603454 showed a much higher EHH value than the G allele. Alleles of significant SNPs on the other chromosomes showed compatible EHH values (Figure 4).
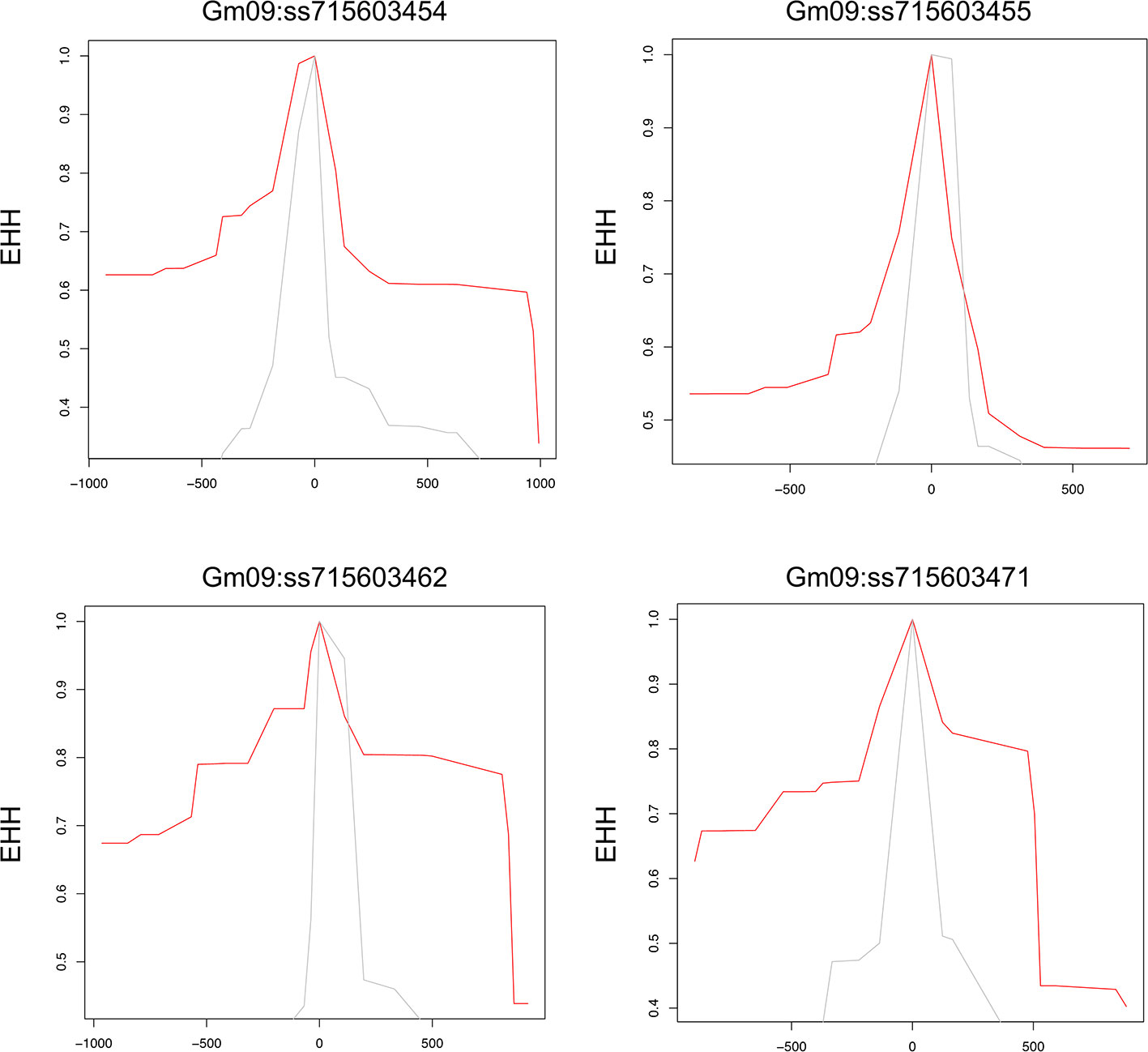
Figure 4 Allele-specific Extended Haplotype Homozygosity (EHH) for the four significant SNPs on chromosome 9. Haplotype lengths are shown flanking the T (red) and G (grey) allele.
4 Discussion
4.1 Metabolic gene clusters in glyceollin elicitation
Gene clusters have been reported to play important roles in phytochemical diversity in Arabidopsis, sorghum, soybean, tomato and rice (Chae et al., 2014; Fan et al., 2020), as well as their roles in important ecological functions in plants i.e., antibacterial, anti-herbivore, antifungal, and insecticidal activities (Polturak and Osbourn, 2021; Polturak et al., 2022). However, their roles in regulating metabolic variation in wild species are relatively less investigated. Even though the isoflavonoid biosynthesis pathway is relatively well studied, the genetic basis of glyceollin elicitation is unclear. Particularly, the contribution, prevalence, and occurrence of gene clusters in plant metabolic diversity are largely unclear. Our mGWAS results suggest there are two probable gene clusters with functionally related but non-homologous genes, which may involve glyceollin elicitation in wild soybean. Thus far, to the best of our knowledge, the genes within these plausible clusters are the first reported candidate genes located on chromosome 9 involved in glyceollin accumulation induced by biotic stimuli in wild soybean. To date, the reported glyceollin biosynthesis genes are located on chromosomes 1, 2, 3, 4, 6, 7, 10, 11, 13, 15, 19 and 20 (Akashi et al., 2009; Yoneyama et al., 2016; Sukumaran et al., 2018; Jahan et al., 2020). Our predicted gene clusters suggest that glyceollin may be synthesized where the enzyme-encoding genes are adjacent to each other on the same chromosome (Chavali and Rhee, 2018). Physical clustering of genes with similar functions can facilitate co-inheritance of alleles with favorable combinations and their coordinated regulations at chromatin level (Osbourn, 2010a; Chu et al., 2011). Besides, such clusters incline to locate in the sub-telomeric regions (Gierl and Frey, 2001; Qi et al., 2004; Sakamoto et al., 2004), near the ends of chromosomes that are known to harbor mutations. For example, an examination of the complete genome sequence revealed that the maize DIMBOA cluster is located close to the end of chromosome 4 (Farman, 2007; Jonczyk et al., 2008). Thus, identifying the positions of the genes can contribute to inferences of possible mechanisms underlying chemical diversity in natural populations.
Beyond gene clusters playing critical roles in phytochemical diversity, tailoring enzymes, such as methyltransferases, glycosyltransferases, CYPs, dehydrogenases/reductases, and acyltransferases, are reported to be responsible for modifying the chemical backbone of specialized metabolites (Osbourn, 2010b). The genes in these two plausible clusters are annotated with tailoring or regulating glycosyltransferase enzymes. One of the common plant defense mechanisms involves glycosylation of secondary metabolites with these enzymes (Mylona et al., 2008). Therefore, the clustering of the genes encoding glycosyltransferase on chromosome 9 might be very critical in the formation of glyceollin, the stress-induced (i.e., SCN stress in our study) protective compounds in legumes. For example, the cyclic hydroxamic acid (DIBOA) in maize (Frey et al., 1997; Gierl and Frey, 2001), the triterpene avenacin in oat (Qi et al., 2004; Qi et al., 2006; Field and Osbourn, 2008; Mugford et al., 2009), and two gene clusters associated with diterpene (momilactone and phytocassane) synthesis in rice, which may be pre-formed or synthesized after stress elicitation for plant defense. Disruption of such gene clusters may compromise pest and disease resistance and lead to the accumulation of toxic pathway intermediates (Chu et al., 2011). In the multi-step plant specialized metabolic pathways, rapid adaptation to a particular environmental niche could result in highly diverse and rapidly evolving metabolic gene clusters (Osbourn and Field, 2009). Hence, the level of conservation of the identified gene clusters across different legume species may shed light on the evolutionary insight of these clusters (Field and Osbourn, 2008). Synthetic biology and functional genetics can further help investigate the organization and contribution of these clusters in metabolite diversity, as well as decipher the mechanism of adaptive evolution and genome plasticity (Osbourn, 2010b; Chu et al., 2011).
4.2 Plausible transcriptional factors in glyceollin elicitation
Advancement of genetics, genomics, and bioinformatic approaches facilitate the prediction and identification of a large number of genes, including transcription factors associated with plant-specialized metabolic pathways (Anarat-Cappillino and Sattely, 2014; Moore et al., 2019). However, the transcriptional regulators of specialized metabolism are less well characterized (Shoji and Yuan, 2021). The regulation of plant-specialized metabolic pathways is dynamic, reflecting the inherent adaptability of these pathways to the ever-changing environment. Such regulation generally occurs at transcription level, and thus, it requires coordinated regulation mediated by transcription factors (TFs) (Colinas and Goossens, 2018; Shoji, 2019). For instance, MYB and basic helix-loop-helix (bHLH) TF family genes were reported to regulate anthocyanin and related flavonoid biosynthetic pathways in a wide range of species (Chezem and Clay, 2016). Moreover, significant modifications of these regulatory genes give rise to the vast diversity in plant specialized metabolism (Huang et al., 2018; Springer et al., 2019).
It is possible that transcription factors, such as MYB and WRKY TFs on chromosome 9, may influence glyceollin elicitation. The regulation of glyceollin elicitation with SCN stress may involve a highly complex interplay among multiple genes and pathways. Previous studies reported that gene families of transcription factors, such as NAC, MYB, bHLH, and WRKY, exhibited conservative patterns among Arabidopsis, cotton, grapevine, maize, and rice (Xu et al., 2004; Zheng et al., 2006; Saga et al., 2012; Ibraheem et al., 2015; Yamamura et al., 2015; Ogawa et al., 2017). These plant species produce various phytoalexins, such as indole alkaloids, terpenoid aldehydes, stilbenoids, deoxyanthocyanidins, and momilactones/phytocassanes, respectively. The investigation of TFs binding promoter regions can give insights if the pathways are co-opted into stress-inducible regulation by the respective TFs such as NAC TF gene GmNAC42–1 and MYB TF gene GmMYB29A2 regulates glyceollin biosynthesis (Jahan et al., 2019; Jahan et al., 2020). The transcription factor gene GmNAC42-1 plays a crucial role as a positive regulator in glyceollin biosynthesis. Jahan and colleagues (Jahan et al., 2019) showed that elevating the expression of GmNAC42-1 in hairy roots has the potential to amplify glyceollin yields by more than tenfold when elicited. Furthermore, the TF gene GmMYB29A2, as identified by Jahan et al. (2020) (Jahan et al., 2020), plays a crucial role in both the accumulation of glyceollin I and the expression of resistance against Phytophthora. It would be intriguing to explore whether the transcription factor genes we’ve identified exhibit homology across different plant species. The homology of TFs among different plant species can facilitate metabolic engineering of a wide variety of crop plants to produce phytoalexins in greater amounts (Ahmed and Kovinich, 2021).
In addition to enzyme-encoding genes, TF genes can also be found as gene clusters. For example, the gene cluster of TF ERF (jasmonate (JA)- responsive ethylene response factor) consists of five ERF genes in tomato (Cárdenas et al., 2016; Thagun et al., 2016), eight in potato (Cárdenas et al., 2016), five in tobacco (Kajikawa et al., 2017), five in C. roseus (Singh et al., 2020), four in Calotropis gigantea (Singh et al., 2020), and four in Glesemium sempervirens (Singh et al., 2020). Besides, TFs involved in plant specialized metabolism can be found in arrays (Zhou et al., 2016; Shoji and Yuan, 2021). Thus, it is possible that the TFs we identified are located in the same genomic neighborhood as arrays or biosynthetic gene clusters (BGCs). The co-regulation hypothesis of gene clusters poses that clustering of TFs can co-regulate genes in a pathway. Although co-regulation of metabolic pathways also occurs un-clustered, clustering may accelerate the recruitment of genes into a regulon (Wisecaver et al., 2017; Smit and Lichman, 2022).
4.3 Epistasis and plausible selection on glyceollin elicitation
Metabolic traits have been reported with low heritability due to environmental effects on their accumulations (Rowe et al., 2008). Recent studies have shown strong epistatic interactions of genes influencing variation of plant specialized metabolites, which may impact fitness in the field (Brachi et al., 2015; Kerwin et al., 2015; Kerwin et al., 2017). For example, numerous epistatic interactions influence the highly complex genetic architecture responsible for Arabidopsis metabolism (Kliebenstein, 2001; Kliebenstein et al., 2001). Moreover, a mixture of positive and negative epistatic interactions can assist identifying significant QTLs located within a biosynthetic pathway (Rowe et al., 2008). Compared to expression regulations, the power of epistasis in metabolomics is that they can better indicate the interconnectedness of metabolites within the metabolic pathway (Fell and Wagner, 2000; Jeong et al., 2000; Arita, 2004). The widespread interactive effects found among our identified significant SNPs affecting targeted metabolic traits may be a consequence of the interconvertibility between daidzein and glyceollin. As an example, the study conducted by Farrell et al. (2017) demonstrated that there is an augmentation in the biosynthesis of glyceollin I from daidzein when there is an elevation in the degradation of 6”-O-malonyldaidzin, an isoflavone conjugate produced from daidzein (Farrell et al., 2017).
Genes containing causal variation for plant defensive compounds may influence field fitness and thus are likely under natural selection (Kroymann, 2011). For example, Benderoth et al. (2006) detected positive selection in glucosinolate diversification in Arabidopsis thaliana and its relatives (Benderoth et al., 2006). Prasad et al. (2012) showed positive selection for a mutation on a metabolic pathway gene could enhance resistance to herbivory in natural populations of a rocky mountain cress species (Prasad et al., 2012). We detected strong signals of selection on the SNPs significantly associated with glyceollin phenotypes with EHH and LD analyses (Figure 4; Figure 2B). For example, the LD surrounding the significant SNP ss715603454 that is next to the identified gene clusters is more extensive, suggesting strong selection in this region (Figure 2B). Meanwhile, the two alleles of this significant SNP, G and T, showed different EHH values, with T exhibiting much longer haplotype homozygosity. This indicates that this T allele may be under recent positive selection. Interestingly, the T allele is significantly associated with higher elicitation of glyceollin and has a higher frequency in South Korea (Figures 2C, D). The allele specific EHH pattern and their geographic distribution may be due to heterogeneous selection pressure in nature.
4.4 Perspectives and future directions of our study
Plant specialized metabolites exhibit extreme quantitative and qualitative variation. Therefore, high-throughput metabolite profiling, such as LC-MS analysis coupled with GWAS (as applied here) can facilitate understanding the genetic contributions to metabolic diversity in natural populations. A common assumption is that biological variables or traits should show a normal distribution, and skewed data may indicate measurement error. However, the scenario is different in metabolomics, especially in secondary metabolism. For instance, a ratio of two related compounds, rather than their separate values, may provide a comprehensive understanding of the underlying enzymatic process (Byrne et al., 1996; McMullen et al., 1998; Yencho et al., 1998; Kliebenstein, 2001; Kliebenstein et al., 2001; Kliebenstein, 2007; Chan et al., 2011; Petersen et al., 2012; Prasad et al., 2012). We used a ratio of glyceollin and daidzein concentrations as the phenotypic trait for our association study. The use of a metabolic ratio also may produce: (1) a reduction in the variability of the data collected for the biological replicates and thus increase statistical power, and (2) a reduction in overall noise in the dataset by canceling out systemic experimental errors. Most importantly for our purposes, the glyceollin to daidzein metabolite ratio is correlated to the corresponding reaction rate under optimal steady-state assumptions, as this metabolite pair is connected in the phenylpropanoid biosynthetic pathway (Suhre et al., 2011; Petersen et al., 2012).
The natural world has a lot to offer in tackling diseases and global food scarcity. There is a need to develop new medicines and future value-increased food by unlocking the uncharted gene pools of wild plants. Our chosen study system crop wild relative of soybean poses much higher and underexplored genetic diversity than its domesticated descendants. Given that glyceollin is produced in trace amounts, it is an exciting challenge to define the plant metabolic gene clusters and transcriptional regulators in the glyceollin biosynthesis pathway. Besides complex cancer treatment and therapies, the rise of different types of tumors and tumor subtypes urges the need for new drugs. Along with glyceollin’s role in plant defense, it has been well-documented for anti-cancer activities. Our follow-up studies will apply transcriptomics and functional validation of the candidate genes, which can expand our focus to explore associations of genes in clusters to understand their involvement in regulating glyceollin biosynthesis at the systems level. As phytochemical variation can be caused by both structural genes and their expression differences, it will be interesting to explore the role of pathway-specific regulators (i.e., transcription factors) in glyceollin elicitation (Osbourn, 2010b). Our results suggest that improving our fundamental knowledge of plant specialized metabolic gene clusters and regulators will facilitate metabolic engineering with improved metabolic traits for sustainable agriculture and novel pharmaceuticals.
Data availability statement
The datasets presented in this study can be found in online repositories. The names of the repository/repositories and accession number(s) can be found in the article/Supplementary Material.
Author contributions
B-HS conceived the idea and initiated the project. FY, HZ, LL, BW, JW, RR, and CB performed experiments and analyzed data. FY, HZ, LL, BW, and B-HS wrote and improved the manuscript. FY and HZ contributed equally. All authors contributed to the article and approved the submitted version.
Funding
The author(s) declare financial support was received for the research, authorship, and/or publication of this article. Research in B-HS lab was supported by the National Institute of General Medical Sciences, Award Number: R15GM122029, R15AT011603; National Science Foundation, Award Number: 2318746; North Carolina Biotechnology Center, Award Number: 2020-FLG-3806 and 2023-FLG-0045; and the University of North Carolina at Charlotte. Work by FY was (partially) supported by the Schlumberger Foundation Faculty for the Future program.
Acknowledgments
We thank T. Mitchell-Olds for the constructive comments and suggestions. We thank X. Li and J. Kofsky for their help with methods and discussions. We also thank Song lab members M. Hatley and N. Mittal for their help with maintaining SCN stocks.
Conflict of interest
The authors declare that the research was conducted in the absence of any commercial or financial relationships that could be construed as a potential conflict of interest.
Publisher’s note
All claims expressed in this article are solely those of the authors and do not necessarily represent those of their affiliated organizations, or those of the publisher, the editors and the reviewers. Any product that may be evaluated in this article, or claim that may be made by its manufacturer, is not guaranteed or endorsed by the publisher.
Supplementary material
The Supplementary Material for this article can be found online at: https://www.frontiersin.org/articles/10.3389/fpls.2024.1240981/full#supplementary-material
References
Ahmed, S., Kovinich, N. (2021). Regulation of phytoalexin biosynthesis for agriculture and human health. Phytochem. Rev. 20 (2), 483–505. doi: 10.1007/s11101-020-09691-8
Aisyah, S., Gruppen, H., Madzora, B., Vincken, J.-P. (2013). Modulation of isoflavonoid composition of Rhizopus oryzae elicited soybean (Glycine max) seedlings by light and wounding. J. Agric. Food Chem. 61 (36), 8657–8667. doi: 10.1021/jf4020203
Akashi, T., Sasaki, K., Aoki, T., Ayabe, S., Yazaki, K. (2009). Molecular cloning and characterization of a cDNA for pterocarpan 4-dimethylallyltransferase catalyzing the key prenylation step in the biosynthesis of glyceollin, a soybean phytoalexin. Plant Physiol. 149 (2), 683–693. doi: 10.1104/pp.108.123679
Anarat-Cappillino, G., Sattely, E. S. (2014). The chemical logic of plant natural product biosynthesis. Curr. Opin. Plant Biol. 19, 51–58. doi: 10.1016/j.pbi.2014.03.007
Arita, M. (2004). The metabolic world of Escherichia coli is not small. Proc. Natl. Acad. Sci. 101, 1543–1547. doi: 10.1073/pnas.0306458101
Bamji, S. F., Corbitt, C. (2017). Glyceollins: Soybean phytoalexins that exhibit a wide range of health-promoting effects. J. Funct. Foods 34, 98–105. doi: 10.1016/j.jff.2017.04.020
Benderoth, M., Textor, S., Windsor, A. J., Mitchell-Olds, T., Gershenzon, J., Kroymann, J. (2006). Positive selection driving diversification in plant secondary metabolism. Proc. Natl. Acad. Sci. 103, 9118–9123. doi: 10.1073/pnas.0601738103
Bharadwaj, R., Kumar, S. R., Sharma, A., Sathishkumar, R. (2021). Plant metabolic gene clusters: evolution, organization, and their applications in synthetic biology. Front. Plant Sci. 12, 697318. doi: 10.3389/fpls.2021.697318
Bleeker, P. M., Mirabella, R., Diergaarde, P. J., VanDoorn, A., Tissier, A., Kant, M. R., et al. (2012). Improved herbivore resistance in cultivated tomato with the sesquiterpene biosynthetic pathway from a wild relative. Proc. Natl. Acad. Sci. 109 (49), 20124–20129. doi: 10.1073/pnas.1208756109
Brachi, B., Meyer, C. G., Villoutreix, R., Platt, A., Morton, T. C., Roux, F., et al. (2015). Coselected genes determine adaptive variation in herbivore resistance throughout the native range of Arabidopsis thaliana. Proc. Natl. Acad. Sci. 112, 4032–4037. doi: 10.1073/pnas.1421416112
Bradbury, P. J., Zhang, Z., Kroon, D. E., Casstevens, T. M., Ramdoss, Y., Buckler, E. S. (2007). TASSEL: Software for association mapping of complex traits in diverse samples. Bioinformatics 23 (19), 2633–2635. doi: 10.1093/bioinformatics/btm308
Byrne, P., Mcmullen, M., Snook, M., Musket, T., Theuri, J., Widstrom, N., et al. (1996). Quantitative trait loci and metabolic pathways: Genetic control of the concentration of maysin, a corn earworm resistance factor, in maize silks. Proc. Natl. Acad. Sci. 93, 8820–8825. doi: 10.1073/pnas.93.17.8820
Cárdenas, P. D., Sonawane, P. D., Pollier, J., Bossche, R. V., Dewangan, V., Weithorn, E., et al. (2016). GAME9 regulates the biosynthesis of steroidal alkaloids and upstream isoprenoids in the plant mevalonate pathway. Nat. Commun. 7, 1–16. doi: 10.1038/ncomms10654
Chae, L., Kim, T., Nilo-Poyanco, R., Rhee, S. Y. (2014). Genomic signatures of specialized metabolism in plants. Science 344, 510–513. doi: 10.1126/science.1252076
Chan, E. K., Rowe, H. C., Corwin, J. A., Joseph, B., Kliebenstein, D. J. (2011). Combining genome-wide association mapping and transcriptional networks to identify novel genes controlling glucosinolates in Arabidopsis thaliana. PLoS Biol. 9, 1001125. doi: 10.1371/journal.pbio.1001125
Chan, E. K., Rowe, H. C., Hansen, B. G., Kliebenstein, D. J. (2010). The complex genetic architecture of the metabolome. PLoS Genet. 6, 1001198. doi: 10.1371/journal.pgen.1001198
Chavali, A. K., Rhee, S. Y. (2018). Bioinformatics tools for the identification of gene clusters that biosynthesize specialized metabolites. Briefings Bioinf. 19, 1022–1034. doi: 10.1093/bib/bbx020
Chen, W., Gao, Y., Xie, W., Gong, L., Lu, K., WANG, W., et al. (2014). Genome-wide association analyses provide genetic and biochemical insights into natural variation in rice metabolism. Nat. Genet. 46, 714–721. doi: 10.1038/ng.3007
Chezem, W. R., Clay, N. K. (2016). Regulation of plant secondary metabolism and associated specialized cell development by MYBs and bHLHs. Phytochemistry 131, 26–43. doi: 10.1016/j.phytochem.2016.08.006
Chu, H. Y., Wegel, E., Osbourn, A. (2011). From hormones to secondary metabolism: The emergence of metabolic gene clusters in plants. Plant J. 66, 66–79. doi: 10.1111/j.1365-313X.2011.04503.x
Colinas, M., Goossens, A. (2018). Combinatorial transcriptional control of plant specialized metabolism. Trends Plant Sci. 23, 324–336. doi: 10.1016/j.tplants.2017.12.006
Dixon, R. A., Steele, C. L. (1999). Flavonoids and isoflavonoids – a gold mine for metabolic engineering. Trends Plant Sci. 4 (10), 394–400. doi: 10.1016/S1360-1385(99)01471-5
Donnez, D., Kim, K.-H., Antoine, S., Conreux, A., Luca, V. D., Jeandet, P., et al. (2011). Bioproduction of resveratrol and viniferins by an elicited grapevine cell culture in a 2 L stirred bioreactor. Process Biochem. 46, 1056–1062. doi: 10.1016/j.procbio.2011.01.019
Fan, P., Wang, P., Lou, Y.-R., Leong, B. J., Moore, B. M., Schenck, C. A., et al. (2020). Evolution of a plant gene cluster in Solanaceae and emergence of metabolic diversity. Elife 9, 56717. doi: 10.7554/eLife.56717.sa2
Farman, M. L. (2007). Telomeres in the rice blast fungus Magnaporthe oryzae: The world of the end as we know it. FEMS Microbiol. Lett. 273, 125–132. doi: 10.1111/j.1574-6968.2007.00812.x
Farrell, K., Jahan, M., Kovinich, N. (2017). Distinct mechanisms of biotic and chemical elicitors enable additive elicitation of the anticancer phytoalexin glyceollin I. Molecules 22 (8), 1261. doi: 10.3390/molecules22081261
Fell, D. A., Wagner, A. (2000). The small world of metabolism. Nat. Biotechnol. 18, 1121–1122. doi: 10.1038/81025
Field, B., Fiston-Lavier, A.-S., Kemn, A., Geisler, K., Quesneville, H., Osbourn, A. E. (2011). Formation of plant metabolic gene clusters within dynamic chromosomal regions. Proc. Natl. Acad. Sci. 108, 16116–16121. doi: 10.1073/pnas.1109273108
Field, B., Osbourn, A. E. (2008). Metabolic diversification—Independent assembly of operon-like gene clusters in different plants. Science 320, 543–547. doi: 10.1126/science.1154990
Frey, M., Chomet, P., Glawischnig, E., Stettner, C., Grun, S., Winklmair, A., et al. (1997). Analysis of a chemical plant defense mechanism in grasses. Science 277, 696–699. doi: 10.1126/science.277.5326.696
Ge, S. X., Jung, D., Yao, R. (2020). ShinyGO: a graphical gene-set enrichment tool for animals and plants. Bioinformatics 36, 2628–2629. doi: 10.1093/bioinformatics/btz931
Gieger, C., Geistlinger, L., Altmaier, E., Hrabé De Angelis, M., Kronenberg, F., Meitinger, T., et al. (2008). Genetics meets metabolomics: a genome-wide association study of metabolite profiles in human serum. PLoS Genet. 4 (11), e1000282. doi: 10.1371/journal.pgen.1000282
Gierl, A., Frey, M. (2001). Evolution of benzoxazinone biosynthesis and indole production in maize. Planta 213, 493–498. doi: 10.1007/s004250100594
Goodstein, D. M., Shu, S., Howson, R., Neupane, R., Hayes, R. D., Fazo, J., et al. (2011). Phytozome: A comparative platform for green plant genomics. Nucleic Acids Res. 40, 1178–1186. doi: 10.1093/nar/gkr944
Graham, T. L., Graham, M. Y. (1996). Signaling in soybean phenylpropanoid responses (dissection of primary, secondary, and conditioning effects of light, wounding, and elicitor treatments). Plant Physiol. 110, 1123–1133. doi: 10.1104/pp.110.4.1123
Grant, D., Nelson, R. T., Cannon, S. B., Shoemaker, R. C. (2010). SoyBase, the USDA-ARS soybean genetics and genomics database. Nucleic Acids Res. 38, 843–846. doi: 10.1093/nar/gkp798
Hawkins, C., Ginzburg, D., Zhao, K., Dwyer, W., Xue, B., Xu, A., et al. (2021). Plant Metabolic Network 15: A resource of genome-wide metabolism databases for 126 plants and algae. J. Integr. Plant Biol. 63, 1888–1905. doi: 10.1111/jipb.13163
Huang, D., Wang, X., Tang, Z., Yuan, Y., Xu, Y., He, J., et al. (2018). Subfunctionalization of the Ruby2–Ruby1 gene cluster during the domestication of citrus. Nat. Plants 4, 930–941. doi: 10.1038/s41477-018-0287-6
Ibraheem, F., Gaffoor, I., Tan, Q., Shyu, C.-R., Chopra, S. (2015). A sorghum MYB transcription factor induces 3-deoxyanthocyanidins and enhances resistance against leaf blights in maize. Molecules 20, 2388–2404. doi: 10.3390/molecules20022388
Illig, T., Gieger, C., Zhai, G., Römisch-Margl, W., Wang-Sattler, R., Prehn, C., et al. (2010). A genome-wide perspective of genetic variation in human metabolism. Nat. Genet. 42 (2), 137–141. doi: 10.1038/ng.507
Jahan, M. A., Harris, B., Lowery, M., Coburn, K., Infante, A. M., Percifield, R. J., et al. (2019). The NAC family transcription factor GmNAC42–1 regulates biosynthesis of the anticancer and neuroprotective glyceollins in soybean. BMC Genomics 20 (1), 149. doi: 10.1186/s12864-019-5524-5
Jahan, M. A., Harris, B., Lowery, M., Infante, A. M., Percifield, R. J., Kovinich, N. (2020). Glyceollin transcription factor GmMYB29A2 regulates soybean resistance to Phytophthora sojae. Plant Physiol. 183 (2), 530–546. doi: 10.1104/pp.19.01293
Jahan, M. A., Kovinich, N. (2019). Acidity stress for the systemic elicitation of glyceollin phytoalexins in soybean plants. Plant Signaling Behav. 14 (7), 1604018. doi: 10.1080/15592324.2019.1604018
Jeandet, P., Douillet-Breuil, A.-C., Bessis, R., Debord, S., Sbaghi, M., Adrian, M. (2002). Phytoalexins from the Vitaceae: Biosynthesis, phytoalexin gene expression in transgenic plants, antifungal activity, and metabolism. J. Agric. Food Chem. 50, 2731–2741. doi: 10.1021/jf011429s
Jeandet, P., Sobarzo-Sánchez, E., Silva, A. S., Clément, C., Nabavi, S. F., Battino, M., et al. (2020). Whole-cell biocatalytic, enzymatic and green chemistry methods for the production of resveratrol and its derivatives. Biotechnol. Adv. 39, 107461. doi: 10.1016/j.biotechadv.2019.107461
Jeong, H., Tombor, B., Albert, R., Oltvai, Z. N., Barabási, A.-L. (2000). The large-scale organization of metabolic networks. Nature 407, 651–654. doi: 10.1038/35036627
Jonczyk, R., Schmidt, H., Osterrieder, A., Fiesselmann, A., Schullehner, K., Haslbeck, M., et al. (2008). Elucidation of the final reactions of DIMBOA-glucoside biosynthesis in maize: Characterization of Bx6 and Bx7. Plant Physiol. 146, 1053–1063. doi: 10.1104/pp.107.111237
Kajikawa, M., Sierro, N., Kawaguchi, H., Bakaher, N., Ivanov, N. V., Hashimoto, T., et al. (2017). Genomic insights into the evolution of the nicotine biosynthesis pathway in tobacco. Plant Physiol. 174, 999–1011. doi: 10.1104/pp.17.00070
Kerwin, R., Feusier, J., Corwin, J., Rubin, M., Lin, C., Muok, A., et al. (2015). Natural genetic variation in Arabidopsis thaliana defense metabolism genes modulates field fitness. Elife 4, e05604. doi: 10.7554/eLife.05604.045
Kerwin, R. E., Feusier, J., Muok, A., Lin, C., Larson, B., Copeland, D., et al. (2017). Epistasis× environment interactions among Arabidopsis thaliana glucosinolate genes impact complex traits and fitness in the field. New Phytol. 215, 1249–1263. doi: 10.1111/nph.14646
Kim, H. J., Lim, J.-S., Kim, W.-K., Kim, J.-S. (2012). Soyabean glyceollins: Biological effects and relevance to human health. Proc. Nutr. Soc. 71 (1), 166–174. doi: 10.1017/S0029665111003272
Kliebenstein, D. (2001). Gene duplication and the diversification of secondary metabolism: Side chain modification of glucosinolates in Arabidopsis thaliana. Plant Cell 13, 681–693. doi: 10.1105/tpc.13.3.681
Kliebenstein, D. J. (2007). Metabolomics and Plant Quantitative Trait Locus Analysis–The optimum genetical genomics platform? In: Concepts in Plant Metabolomics eds. Nikolau, B. J., Wurtele, E. S. (Dordrecht: Springer), 29–44. doi: 10.1007/978-1-4020-5608-6_3
Kliebenstein, D. J., Gershenzon, J., Mitchell-Olds, T. (2001). Comparative quantitative trait loci mapping of aliphatic, indolic and benzylic glucosinolate production in Arabidopsis thaliana leaves and seeds. Genetics 159, 359–370. doi: 10.1093/genetics/159.1.359
Koonin, E. V. (2009). Evolution of genome architecture. Int. J. Biochem. Cell Biol. 41, 298–306. doi: 10.1016/j.biocel.2008.09.015
Kroymann, J. (2011). Natural diversity and adaptation in plant secondary metabolism. Curr. Opin. Plant Biol. 14, 246–251. doi: 10.1016/j.pbi.2011.03.021
Li, J., Ji, L. (2005). Adjusting multiple testing in multilocus analyses using the eigenvalues of a correlation matrix. Heredity 95, 221–227. doi: 10.1038/sj.hdy.6800717
Lozovaya, V. V., Lygin, A. V., Zernova, O. V., Ulanov, A. V., Li, S., Hartman, G. L., et al. (2007). Modification of phenolic metabolism in soybean hairy roots through down regulation of chalcone synthase or isoflavone synthase. Planta 225, 665–679. doi: 10.1007/s00425-006-0368-z
Luo, J. (2015). Metabolite-based genome-wide association studies in plants. Curr. Opin. Plant Biol. 24, 31–38. doi: 10.1016/j.pbi.2015.01.006
Luo, W., Pant, G., Bhavnasi, Y. K., Blanchard, S. G. Jr, Brouwer, C. (2017). Pathview Web: User friendly pathway visualization and data integration. Nucleic Acids Res. 45 (W1), W501–W508. doi: 10.1093/nar/gkx372
Lygin, A. V., Zernova, O. V., Hill, C. B., Kholina, N. A., Widholm, J. M., Hartman, G. L., et al. (2013). Glyceollin is an important component of soybean plant defense against Phytophthora sojae and Macrophomina phaseolina. Phytopathology® 103 (10), 984–994. doi: 10.1094/PHYTO-12-12-0328-R
Matsuba, Y., Nguyen, T. T., Wiegert, K., Falara, V., Gonzales-Vigil, E., Leong, B., et al. (2013). Evolution of a complex locus for terpene biosynthesis in Solanum. Plant Cell 25, 2022–2036. doi: 10.1105/tpc.113.111013
Matthews, B. F., Macdonald, M. H., Thai, V. K., Tucker, M. L. (2003). Molecular characterization of arginine kinases in the soybean cyst nematode (Heterodera glycines). J. Nematol. 35 (3), 252–258.
McMullen, M., Byrne, P., Snook, M., Wiseman, B., Lee, E., Widstrom, N., et al. (1998). Quantitative trait loci and metabolic pathways. Proc. Natl. Acad. Sci. 95, 1996–2000. doi: 10.1073/pnas.95.5.1996
Mipeshwaree Devi, A., Khedashwori Devi, K., Premi Devi, P., Lakshmipriyari Devi, M., Das, S. (2023). Metabolic engineering of plant secondary metabolites: Prospects and its technological challenges. Front. Plant Sci. 14, 1171154. doi: 10.3389/fpls.2023.1171154
Miyamoto, K., Fujita, M., Shenton, M. R., Akashi, S., Sugawara, C., Sakai, A., et al. (2016). Evolutionary trajectory of phytoalexin biosynthetic gene clusters in rice. Plant J. 87, 293–304. doi: 10.1111/tpj.13200
Moore, B. M., Wang, P., Fan, P., Leong, B., Schenck, C. A., Lloyd, J. P., et al. (2019). Robust predictions of specialized metabolism genes through machine learning. Proc. Natl. Acad. Sci. 116, 2344–2353. doi: 10.1073/pnas.1817074116
Mugford, S. T., Qi, X., Bakht, S., Hill, L., Wegel, E., Hughes, R. K., et al. (2009). A serine carboxypeptidase-like acyltransferase is required for synthesis of antimicrobial compounds and disease resistance in oats. Plant Cell 21, 2473–2484. doi: 10.1105/tpc.109.065870
Murphy, P. A., Barua, K., Hauck, C. C. (2002). Solvent extraction selection in the determination of isoflavones in soy foods. J. Chromatogr. B 777 (1–2), 129–138. doi: 10.1016/S1570-0232(02)00342-2
Mylona, P., Owatworakit, A., Papadopoulou, K., Jenner, H., Qin, B., Findlay, K., et al. (2008). Sad3 and Sad4 are required for saponin biosynthesis and root development in oat. Plant Cell 20, 201–212. doi: 10.1105/tpc.107.056531
Nützmann, H. W., Huang, A., Osbourn, A. (2016). Plant metabolic clusters–from genetics to genomics. New Phytol. 211, 771–789. doi: 10.1111/nph.13981
Nwachukwu, I. D., Luciano, F. B., Udenigwe, C. C. (2013). The inducible soybean glyceollin phytoalexins with multifunctional health-promoting properties. Food Res. Int. 54 (1), 1208–1216. doi: 10.1016/j.foodres.2013.01.024
Ogawa, S., Miyamoto, K., Nemoto, K., Sawasaki, T., Yamane, H., Nojiri, H., et al. (2017). OsMYC2, an essential factor for JA-inductive sakuranetin production in rice, interacts with MYC2-like proteins that enhance its transactivation ability. Sci. Rep. 7, 1–11. doi: 10.1038/srep40175
Osbourn, A. (2010a). Gene clusters for secondary metabolic pathways: An emerging theme in plant biology. Plant Physiol. 154, 531–535. doi: 10.1104/pp.110.161315
Osbourn, A. (2010b). Secondary metabolic gene clusters: Evolutionary toolkits for chemical innovation. Trends Genet. 26, 449–457. doi: 10.1016/j.tig.2010.07.001
Osbourn, A. E., Field, B. (2009). Operons. Cell. Mol. Life Sci. 66, 3755–3775. doi: 10.1007/s00018-009-0114-3
Petersen, A.-K., Krumsiek, J., Wägele, B., Theis, F. J., Wichmann, H.-E., Gieger, C., et al. (2012). On the hypothesis-free testing of metabolite ratios in genome-wide and metabolome-wide association studies. BMC Bioinf. 13, 1–7. doi: 10.1186/1471-2105-13-120
Pham, T. H., Lecomte, S., Efstathiou, T., Ferriere, F., Pakdel, F. (2019). An update on the effects of glyceollins on human health: Possible anticancer effects and underlying mechanisms. Nutrients 11 (1), 79. doi: 10.3390/nu11010079
Pichersky, E., Gang, D. R. (2000). Genetics and biochemistry of secondary metabolites in plants: An evolutionary perspective. Trends Plant Sci. 5, 439–445. doi: 10.1016/S1360-1385(00)01741-6
Polturak, G., Dippe, M., Stephenson, M. J., Misra, R. C., Owen, C., Ramirez-Gonzalez, R. H., et al. (2022). Pathogen-induced biosynthetic pathways encode defense-related molecules in bread wheat. Proc. Natl. Acad. Sci. 119, 2123299119. doi: 10.1073/pnas.2123299119
Polturak, G., Osbourn, A. (2021). The emerging role of biosynthetic gene clusters in plant defense and plant interactions. PLoS Pathog. 17, 1009698. doi: 10.1371/journal.ppat.1009698
Prasad, K. V. S. K., Song, B.-H., Olson-Manning, C., Anderson, J. T., Lee, C.-R., Schranz, M. E., et al. (2012). A gain-of-function polymorphism controlling complex traits and fitness in nature. Science 337 (6098), 1081–1084. doi: 10.1126/science.1221636
Qi, X., Bakht, S., Leggett, M., Maxwell, C., Melton, R., Osbourn, A. (2004). A gene cluster for secondary metabolism in oat: Implications for the evolution of metabolic diversity in plants. Proc. Natl. Acad. Sci. 101, 8233–8238. doi: 10.1073/pnas.0401301101
Qi, X., Bakht, S., Qin, B., Leggett, M., Hemmings, A., Mellon, F., et al. (2006). A different function for a member of an ancient and highly conserved cytochrome P450 family: From essential sterols to plant defense. Proc. Natl. Acad. Sci. 103, 18848–18853. doi: 10.1073/pnas.0607849103
Riedelsheimer, C., Lisec, J., Czedik-eysenberg, A., Sulpice, R., Flis, A., Grieder, C., et al. (2012). Genome-wide association mapping of leaf metabolic profiles for dissecting complex traits in maize. Proc. Natl. Acad. Sci. 109, 8872–8877. doi: 10.1073/pnas.1120813109
Rocha, E. P. (2008). The organization of the bacterial genome. Annu. Rev. Genet. 42, 211–233. doi: 10.1146/annurev.genet.42.110807.091653
Rowe, H. C., Hansen, B. G., Halkier, B. A., Kliebenstein, D. J. (2008). Biochemical networks and epistasis shape the Arabidopsis thaliana metabolome. Plant Cell 20, 1199–1216. doi: 10.1105/tpc.108.058131
Sabeti, P. C., Reich, D. E., Higgins, J. M., Levine, H. Z., Richter, D. J., Schaffner, S. F., et al. (2002). Detecting recent positive selection in the human genome from haplotype structure. Nature 419, 832–837. doi: 10.1038/nature01140
Saga, H., Ogawa, T., Kai, K., Suzuki, H., Ogata, Y., Sakurai, N., et al. (2012). Identification and characterization of ANAC042, a transcription factor family gene involved in the regulation of camalexin biosynthesis in Arabidopsis. Mol. Plant-Microbe Interact. 25, 684–696. doi: 10.1094/MPMI-09-11-0244
Sakamoto, T., Miura, K., ITOH, H., Tatsumi, T., Ueguchi-tanaka, M., Ishiyama, K., et al. (2004). An overview of gibberellin metabolism enzyme genes and their related mutants in rice. Plant Physiol. 134, 1642–1653. doi: 10.1104/pp.103.033696
Seo, J., Kim, B., Oh, J., Kim, J.-S. (2018). Soybean-derived phytoalexins improve cognitive function through activation of Nrf2/HO-1 signaling pathway. Int. J. Mol. Sci. 19 (1), 268. doi: 10.3390/ijms19010268
Shin, J.-H., Blay, S., Mcneney, B., Graham, J. (2006). LDheatmap: An R function for graphical display of pairwise linkage disequilibria between single nucleotide polymorphisms. J. Stat. Software 16, 1–10. doi: 10.18637/jss.v016.c03
Shoji, T. (2019). The recruitment model of metabolic evolution: Jasmonate-responsive transcription factors and a conceptual model for the evolution of metabolic pathways. Front. Plant Sci. 10, 560. doi: 10.3389/fpls.2019.00560
Shoji, T., Yuan, L. (2021). ERF gene clusters: Working together to regulate metabolism. Trends Plant Sci. 26, 23–32. doi: 10.1016/j.tplants.2020.07.015
Singh, R. J., Hymowitz, T. (1999). Soybean genetic resources and crop improvement. Genome 42, 605–616. doi: 10.1139/g99-039
Singh, S. K., Patra, B., Paul, P., Liu, Y., Pattanaik, S., Yuan, L. (2020). Revisiting the ORCA gene cluster that regulates terpenoid indole alkaloid biosynthesis in Catharanthus roseus. Plant Sci. 293, 110408. doi: 10.1016/j.plantsci.2020.110408
Smit, S. J., Lichman, B. R. (2022). Plant biosynthetic gene clusters in the context of metabolic evolution. Natural Product Rep. 39, 1465–1482. doi: 10.1039/D2NP00005A
Song, Q., Hyten, D. L., Jia, G., Quigley, C. V., Fickus, E. W., Nelson, R. L., et al. (2013). Development and evaluation of SoySNP50K, a high-density genotyping array for soybean. PloS One 8, 54985. doi: 10.1371/journal.pone.0054985
Springer, N., León, D. E., Grotewold, E. (2019). Challenges of translating gene regulatory information into agronomic improvements. Trends Plant Sci. 24, 1075–1082. doi: 10.1016/j.tplants.2019.07.004
Strauch, R. C., Svedin, E., Dilkes, B., Chapple, C., Li, X. (2015). Discovery of a novel amino acid racemase through exploration of natural variation in Arabidopsis thaliana. Proc. Natl. Acad. Sci. 112, 11726–11731. doi: 10.1073/pnas.1503272112
Subramanian, S., Stacey, G., Yu, O. (2006). Endogenous isoflavones are essential for the establishment of symbiosis between soybean and Bradyrhizobium japonicum. Plant J. 48 (2), 261–273. doi: 10.1111/j.1365-313X.2006.02874.x
Suhre, K., Shin, S.-Y., Petersen, A.-K., Mohney, R. P., Meredith, D., Wägele, B., et al. (2011). Human metabolic individuality in biomedical and pharmaceutical research. Nature 477, 54–60. doi: 10.1038/nature10354
Sukumaran, A., Mcdowell, T., Chen, L., Renaud, J., Dhaubhadel, S. (2018). Isoflavonoid-specific prenyltransferase gene family in soybean: GmPT01, a pterocarpan 2-dimethylallyltransferase involved in glyceollin biosynthesis. Plant J. 96, 966–981. doi: 10.1111/tpj.14083
Szpiech, Z. A., Hernandez, R. D. (2014). selscan: An efficient multithreaded program to perform EHH-based scans for positive selection. Mol. Biol. Evol. 31, 2824–2827. doi: 10.1093/molbev/msu211
Takos, A. M., Rook, F. (2012). Why biosynthetic genes for chemical defense compounds cluster. Trends Plant Sci. 17, 383–388. doi: 10.1016/j.tplants.2012.04.004
Tang, Y., Liu, X., Wang, J., Li, M., Wang, Q., Tian, F., et al. (2016). GAPIT version 2: An enhanced integrated tool for genomic association and prediction. Plant Genome 9, 2015. doi: 10.3835/plantgenome2015.11.0120
Thagun, C., Imanishi, S., Kudo, T., Nakabayashi, R., Ohyama, K., Mori, T., et al. (2016). Jasmonate-responsive ERF transcription factors regulate steroidal glycoalkaloid biosynthesis in tomato. Plant Cell Physiol. 57, 961–975. doi: 10.1093/pcp/pcw067
Töpfer, N., Fuchs, L. M., Aharoni, A. (2017). The PhytoClust tool for metabolic gene clusters discovery in plant genomes. Nucleic Acids Res. 45, 7049–7063. doi: 10.1093/nar/gkx404
Turner, S. D. (2018). qqman: An R package for visualizing GWAS results using Q-Q and Manhattan plots. J. Open Source Softw. 3, 731. doi: 10.21105/joss.00731
Tylka, G. L., Marett, C. C. (2021). Known distribution of the soybean cyst nematode, Heterodera glycines, in the United States and Canada in 2020. Plant Health Prog. 22, 72–74. doi: 10.1094/PHP-10-20-0094-BR
Wang, X., Howell, C. P., Chen, F., Yin, J., Jiang, Y. (2009). Gossypol-a polyphenolic compound from cotton plant. Adv. Food Nutr. Res. 58, 215–263. doi: 10.1016/S1043-4526(09)58006-0
Weir, B. S. (1990). Genetic data analysis. Methods for discrete population genetic data (Sunderland, MA: Sinaucer Associates, Inc. Publishers).
Wisecaver, J. H., Borowsky, A. T., Tzin, V., Jander, G., Kliebenstein, D. J., Rokas, A. (2017). A global coexpression network approach for connecting genes to specialized metabolic pathways in plants. Plant Cell 29, 944–959. doi: 10.1105/tpc.17.00009
Xu, Y.-H., Wang, J.-W., Wang, S., Wang, J.-Y., Chen, X.-Y. (2004). Characterization of GaWRKY1, a cotton transcription factor that regulates the sesquiterpene synthase gene (+)-δ-cadinene synthase-A. Plant Physiol. 135, 507–515. doi: 10.1104/pp.104.038612
Yamamura, C., Mizutani, E., Okada, K., Nakagawa, H., Fukushima, S., Tanaka, A., et al. (2015). Diterpenoid phytoalexin factor, a bHLH transcription factor, plays a central role in the biosynthesis of diterpenoid phytoalexins in rice. Plant J. 84, 1100–1113. doi: 10.1111/tpj.13065
Yeaman, S., Whitlock, M. C. (2011). The genetic architecture of adaptation under migration–selection balance. Evolution: Int. J. Organic Evol. 65, 1897–1911. doi: 10.1111/j.1558-5646.2011.01269.x
Yencho, G., Kowalski, S., Kobayashi, R., Sinden, S., Bonierbale, M., Deahl, K. (1998). QTL mapping of foliar glycoalkaloid aglycones in Solanum tuberosum× S. berthaultii potato progenies: Quantitative variation and plant secondary metabolism. Theor. Appl. Genet. 97, 563–574. doi: 10.1007/s001220050932
Yoneyama, K., Akashi, T., Aoki, T. (2016). Molecular characterization of soybean pterocarpan 2-dimethylallyltransferase in glyceollin biosynthesis: Local gene and whole-genome duplications of prenyltransferase genes led to the structural diversity of soybean prenylated isoflavonoids. Plant Cell Physiol. 57, 2497–2509. doi: 10.1093/pcp/pcw178
Zhang, Y., Guo, W., Chen, L., Shen, X., Yang, H., Fang, Y., et al. (2022). CRISPR/Cas9-mediated targeted mutagenesis of GmUGT enhanced soybean resistance against leaf-chewing insects through flavonoids biosynthesis. Front. Plant Sci. 13, 802716. doi: 10.3389/fpls.2022.802716
Zhang, H., Kjemtrup-lovelace, S., Li, C., Luo, Y., Chen, L. P., Song, B.-H. (2017a). Comparative RNA-seq analysis uncovers a complex regulatory network for soybean cyst nematode resistance in wild soybean (Glycine soja). Sci. Rep. 7, 1–14. doi: 10.1038/s41598-017-09945-0.
Zhang, H., Mittal, N., Leamy, L. J., Barazani, O., Song, B. (2017b). Back into the wild—Apply untapped genetic diversity of wild relatives for crop improvement. Evolutionary Appl. 10 (1), 5–24. doi: 10.1111/eva.12434
Zhang, H., Song, B. H. (2017). RNA-seq data comparisons of wild soybean genotypes in response to soybean cyst nematode (Heterodera glycines). Genom Data 14, 36–39. doi: 10.1016/j.gdata.2017.08.001
Zhang, H., Yasmin, F., Song, B.-H. (2019). Neglected treasures in the wild—Legume wild relatives in food security and human health. Curr. Opin. Plant Biol. 49, 17–26. doi: 10.1016/j.pbi.2019.04.004
Zheng, Z., Qamar, S. A., Chen, Z., Mengiste, T. (2006). Arabidopsis WRKY33 transcription factor is required for resistance to necrotrophic fungal pathogens. Plant J. 48, 592–605. doi: 10.1111/j.1365-313X.2006.02901.x
Zheng, Y., Szustakowski, J. D., Fortnow, L., Roberts, R. J., Kasif, S. (2002). Computational identification of operons in microbial genomes. Genome Res. 12, 1221–1230. doi: 10.1101/gr.200602
Zhou, Z., Jiang, Y., Wang, Z., Gou, Z., Lyu, J., Li, W., et al. (2015). Resequencing 302 wild and cultivated accessions identifies genes related to domestication and improvement in soybean. Nat. Biotechnol. 33, 408–414. doi: 10.1038/nbt.3096
Keywords: epistasis, mGWAS, phytoalexin, candidate gene, gene cluster, Glycine soja
Citation: Yasmin F, Zhang H, Leamy L, Wang B, Winnike J, Reid RW, Brouwer CR and Song B-H (2024) Genetic basis and selection of glyceollin elicitation in wild soybean. Front. Plant Sci. 15:1240981. doi: 10.3389/fpls.2024.1240981
Received: 15 June 2023; Accepted: 29 January 2024;
Published: 28 February 2024.
Edited by:
Sagarika Mishra, United Arab Emirates University, United Arab EmiratesReviewed by:
Nik Kovinich, York University, CanadaBo-Keun Ha, Chonnam National University, Republic of Korea
Copyright © 2024 Yasmin, Zhang, Leamy, Wang, Winnike, Reid, Brouwer and Song. This is an open-access article distributed under the terms of the Creative Commons Attribution License (CC BY). The use, distribution or reproduction in other forums is permitted, provided the original author(s) and the copyright owner(s) are credited and that the original publication in this journal is cited, in accordance with accepted academic practice. No use, distribution or reproduction is permitted which does not comply with these terms.
*Correspondence: Bao-Hua Song, YnNvbmc1QGNoYXJsb3R0ZS5lZHU=; Hengyou Zhang, emhhbmdoZW5neW91QGlnYS5hYy5jbg==
†Present address: Hengyou Zhang, State Key Laboratory of Black Soils Conservation and Utilization, Northeast Institute of Geography and Agroecology, Chinese Academy of Sciences, Harbin, China
‡These authors have contributed equally to this work