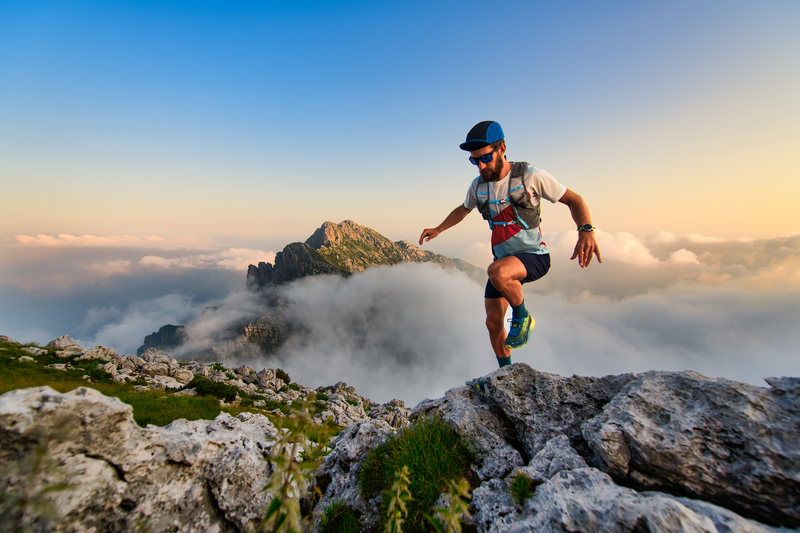
94% of researchers rate our articles as excellent or good
Learn more about the work of our research integrity team to safeguard the quality of each article we publish.
Find out more
OPINION article
Front. Plant Sci. , 07 December 2023
Sec. Plant Bioinformatics
Volume 14 - 2023 | https://doi.org/10.3389/fpls.2023.1309744
With the rapid advancement of Next-Generation Sequencing (NGS) technologies, researchers have gained access to an ever-increasing amount of biological genome data, including many ultra-large genomes (>10 Gb). These ultra-large genomes are typically found in species of gymnosperms, excluding the gnetophytes (Wan et al., 2022). Examples of such species include Cycas (10.5 Gb) (Liu et al., 2022), Ginkgo (10.6 Gb) (Liu et al., 2021), Norway spruce (12.3 Gb) (Nystedt et al., 2013), Chinese pine (25.4 Gb) (Niu et al., 2022), and coast redwood (26.5 Gb) (Scott et al., 2016). However, due to the inherently large genomes of gymnosperms, conducting whole-genome studies has become exceedingly challenging (Wang and Ingvarsson, 2023). Handling these vast genomic datasets typically demands substantial computational resources. Ultra-large genomes in gymnosperms are characterized by an abundance of repetitive sequences, chimeric genes, and intricate structural variations (Nystedt et al., 2013; Wan et al., 2022), rendering existing alignment tools often ineffective in efficiently mapping sequencing reads to these extensive genomes. Given the complexity of ultra-large genomes, the memory requirements for generating alignment indices far exceed the capabilities of many research laboratories and researchers. This has posed formidable obstacles for investigators attempting to address biological questions within the context of these ultra-large genomes.
Coniferous trees hold considerable ecological and economic value, prompting extensive researches into their entire genomes (Neale et al., 2022). Gymnosperms possess distinct genes with unique functions (Ausin et al., 2016), indicating their high potential for functional genomics studies. In order to study gene function in gymnosperms, identifying differentially expressed genes is a primary avenue for exploring functional genes (Costa-Silva et al., 2017), with RNA-seq serving as an indispensable tool for analyzing differential gene expression at the transcriptome level (Stark et al., 2019). Moreover, software tools such as STAR (Dobin et al., 2013) and HISAT2 (Kim et al., 2019) have demonstrated high accuracy and sensitivity in detecting specific transcripts and certain genes when mapping RNA-seq reads to the genome (Sahraeian et al., 2017). However, prior to mapping, the process of indexing genome often consumes a substantial amount of physical memory. For instance, HISAT2 constructs a reference genome index for the 3GB human genome with structure annotation file, it can peak at a memory consumption of up to 200GB (Kim et al., 2019). As genome sizes increase, the memory requirements escalate accordingly. Notably, Paris japonica with a genome size of 145 Gb (Pellicer et al., 2010), represents the largest genomes among eukaryotes, presenting a formidable challenge when it comes to handling large genome alignment tasks that demand significant physical memory.
In light of this, we suggest that when publishing the genomes of gymnosperms, researchers should also provide a version of the genome where repeat regions have been masked or at least provide an annotation file for repeat regions to facilitate their use by other researchers.
In order to effectively map sequencing data to the reference genome for gene function studies in gymnosperms, we have explored some strategies to address the challenges. The most straightforward approach involves increasing physical memory space, but this often entails expensive hardware upgrades. Alternatively, in some special cases, such as when aligning RNA-seq sequencing data, we can employ a reference-free method to handle the sequencing data. However, due to the absence of a reference genome for validation, reference-free analysis may lead to a higher incidence of false positives, where genes or transcripts are erroneously identified as present when they do not actually exist (Lee et al., 2021). Therefore, the optimal scenario is to align the sequencing reads to the index established by the hard-masked genome, reducing memory usage without altering the sequence and positional information of the genes. To assess the effect of using a hard-masked genome in build indices, we employed the STAR (v2.7.8, parameters: –runThreadN 20 –runMode genomeGenerate) to build index for the longest chromosomes of four gymnosperm species. Our findings reveal a notable reduction in maximum resident set size (i.e., the peak memory usage) when building indices using a hard-masked genome (Table 1). Apart from the repeat regions, all other regions are considered as effective areas for index building. Consequently, species with a higher proportion of repeat sequences might exhibit a more pronounced reduction in maximum resident set size during index building. However, this observation is not an absolute rule, as the memory utilization during index creation is also influenced by the complexity of gene structure annotation.
Table 1 Comparison of peak memory usage in unmasked and repeat-masked sequences in the longest chromosomes of four gymnosperm species.
The large sizes of gymnosperm genomes are primarily attributed to the historical and ongoing activity of retrotransposons or transposable elements (TEs), including long terminal repeat (LTR) retrotransposons (Feschotte et al., 2002; Lim et al., 2007). Additionally, in gymnosperms, species with larger genomes tend to have a higher proportion of intact LTRs and a lower proportion of solo LTRs compared to species with smaller genomes (Moffat, 2000; Nystedt et al., 2013; Wan et al., 2018; Xiong et al., 2021; Niu et al., 2022). Therefore, by masking repeat regions in the genome, we can reduce the physical memory usage during the index building process.
Compared to angiosperms, gymnosperms, particularly those with large genomes, are more prone to annotation issues due to a higher proportion of repetitive sequences (Zhang et al., 2023). The presence of repeats can lead to misinterpretation of gene boundaries, affecting the accuracy of gene annotations (Nystedt et al., 2013; Wang and Ingvarsson, 2023). Furthermore, in automated annotation pipelines, tools like BRAKER (Hoff et al., 2019) and MAKER (Campbell et al., 2014) are frequently employed to utilize repeat-masked genome for annotation. This practice aims to avoid noise and reduce computational burden (Pham et al., 2020; Mei et al., 2021; Yang et al., 2022). It’s worth noting that in some specific studies, only a small part of the genome is focused on. For example, in RNA-seq read mapping, researchers primarily focus on transcript regions (Conesa et al., 2016). Therefore, when researchers aim for regions that have been annotated in the genome, mapping sequencing reads to the masked genome may not have a significant impact on the results or outcomes of the specific studies.
Gymnosperms are characterized by the presence of exceptionally long genes (Nystedt et al., 2013; Guan et al., 2016; Niu et al., 2022), often distinguished by their very long introns (Wan et al., 2021). These introns sometimes incorporate LTRs and TEs (Stival Sena et al., 2014). In Chinese pine, it appears that long introns do not significantly affect transcription accuracy (Niu et al., 2022). However, long genes in Picea glauca and Picea tabuliformis tend to exhibit higher expression levels (Ren et al., 2006; Stival Sena et al., 2014). Conversely, some studies in other organisms have suggested that compact genes often display higher expression levels (Castillo-Davis et al., 2002; Stenøien, 2007). The confusion regarding the correlation between gene length and expression level may be attributed to the overrepresented reads from long transcripts, leading to statistical biases in RNA sequencing data (Project et al., 2013; Wan et al., 2022). Therefore, genome-wide masking of repeats has the potential to reduce intron length to some extent while preserving gene structure, thus effectively minimizing alignment errors.
To assist researchers in more easily obtaining a masked genome of ultra-large genome species for genome alignment, we have organized a workflow. It provides researchers with instructions on creating a masked genome either through sequence-based masking or via the annotation of repeat regions within the sequence.
Here are the main steps of this workflow: In the scenario where only the genome sequence file is available, there is a need to perform repeat sequence prediction and masking operations. Firstly, employ Red (Girgis, 2015) to predict repetitive sequences within the genome. Subsequently, transform the genome that has been software-masked into a hard-masked genome. In the situation where both the genome sequence file and repeat annotation are provided, the provided repeat annotation file can be converted into a bed file. Then, utilize the BEDTools (Quinlan and Hall, 2010) to mask repeat regions based on the information within the bed file.
This method provides researchers with a more flexible solution. The code and resources related to this workflow can be found in the GitHub repository (https://github.com/pk-zhu/APMG), for use and further improvement by the scientific community.
Using a repeat-masked genome for alignment, particularly in gymnosperms where genome expansion has been driven by an increase in the proportion of repeats (Wan et al., 2022), can lead to reduced memory usage during the indexing of a genome for downstream analysis. However, this approach may present some potential drawbacks in accurately representing genomic complexity. Firstly, since more sequences are masked in gymnosperms, alignment inaccuracies may occur during whole-genome alignment. These inaccuracies might manifest when using a masked genome for read mapping due to the absence of certain information. For example, some reads might align to both ends of the masked region due to the absence of nucleotides in the sequence, resulting in discrepancies in downstream analyses reliant on alignment, such as estimating transcript abundance. However, this possibility can be mitigated by setting a stringent mismatch tolerance. Furthermore, due to the masking of certain sequences, some genuinely multi-mapped reads may be considered as uniquely mapped reads. However, since the prediction of repeats is based on sequence similarity, only a limited number of reads might experience this situation, and this inaccuracy will be diminished with updates in repeat sequence prediction algorithms. Additionally, for variant calling analysis based on alignment results, masking repeat regions can effectively eliminate false duplicates of a set of related genes, thereby enhancing the accuracy of variant detection (Catreux et al., 2021; Wagner et al., 2022). Given the substantial presence of repeats in gymnosperm genomes, using a masked genome might therefore yield more accurate results.
However, reads generated from whole-genome sequencing techniques such as ATAC-seq (Buenrostro et al., 2013), BS-seq (Krueger et al., 2012), and RAD-seq (Davey and Blaxter, 2011) are not recommended to be used with a masked genome in analysis. These techniques are commonly employed for sequencing across the entire genome, aiming to comprehend the structure, functionality, and variations within the genome. Therefore, these kinds of data might be required for alignment across the whole genome or for computing abundance signals of reads aligned to repeat regions. Additionally, DNA methylation levels are positively correlated with genome size (Novák et al., 2020), and some repeat sequences contribute to the formation of highly methylated heterochromatin (Islam-Faridi et al., 2007; Fedoroff, 2012), which is one of the critical factors for the proper expression of long genes in gymnosperms (Fuchs et al., 2008; Niu et al., 2022). Therefore, data capable of detecting methylation should also not be mapped to the repeat-masked genome.
In conclusion, when adopting the masked genome for analysis, researchers need to carefully balance the reduction in computational resource consumption with the potential loss of genetic information. It’s essential to consider this balance in the context of their research objectives and specific subjects under study. Masked genome offers a flexible and efficient solution for studying gymnosperms but should be used judiciously considering its limitations in specific research scenarios.
PZ: Methodology, Writing – original draft, Writing – review & editing. TH: Funding acquisition, Writing – review & editing. YZ: Resources, Writing – review & editing. LC: Supervision, Writing – review & editing.
The author(s) declare financial support was received for the research, authorship, and/or publication of this article. This research was funded by the National Key Research and Development Program of China (2021YFD2200501), Scientific Research Project of Fujian Province (2023J01478), Fujian Forestry Nursery Technology Tackle Key Issues Project Phase Seven (LZKG-202207) and Forestry Peak Discipline Construction Project from Fujian Agriculture and Forestry University (72202200205).
We appreciate the valuable suggestions provided by editor JM. These suggestions enabled us to improve the quality of our manuscript.
The authors declare that the research was conducted in the absence of any commercial or financial relationships that could be construed as a potential conflict of interest.
All claims expressed in this article are solely those of the authors and do not necessarily represent those of their affiliated organizations, or those of the publisher, the editors and the reviewers. Any product that may be evaluated in this article, or claim that may be made by its manufacturer, is not guaranteed or endorsed by the publisher.
Ausin, I., Feng, S., Yu, C., Liu, W., Kuo, H. Y., Jacobsen, E. L., et al. (2016). DNA methylome of the 20-gigabase Norway spruce genome. Proc. Natl. Acad. Sci. 113, E8106–E8113. doi: 10.1073/pnas.1618019113
Buenrostro, J. D., Giresi, P. G., Zaba, L. C., Chang, H. Y., Greenleaf, W. J. (2013). Transposition of native chromatin for fast and sensitive epigenomic profiling of open chromatin, DNA-binding proteins and nucleosome position. Nat. Methods 10, 1213–1218. doi: 10.1038/nmeth.2688
Campbell, M. S., Law, M., Holt, C., Stein, J. C., Moghe, G. D., Hufnagel, D. E., et al. (2014). MAKER-P: A tool kit for the rapid creation, management, and quality control of plant genome annotations. Plant Physiol. 164, 513–524. doi: 10.1104/pp.113.230144
Castillo-Davis, C. I., Mekhedov, S. L., Hartl, D. L., Koonin, E. V., Kondrashov, F. A. (2002). Selection for short introns in highly expressed genes. Nat. Genet. 31, 415–418. doi: 10.1038/ng940
Catreux, S., Farrell, F., Mehio, R., Murray, L., Parnaby, G., Roddey, C., et al. (2021) Demystifying the versions of GRCh38/hg38 reference genomes, how they are used in DRAGEN and their impact on accuracy. Available at: https://www.illumina.com/science/genomics-research/articles/dragen-demystifying-reference-genomes.html.
Conesa, A., Madrigal, P., Tarazona, S., Gomez-Cabrero, D., Cervera, A., McPherson, A., et al. (2016). A survey of best practices for RNA-seq data analysis. Genome Biol. 17, 13. doi: 10.1186/s13059-016-0881-8
Costa-Silva, J., Domingues, D., Lopes, F. M. (2017). RNA-Seq differential expression analysis: An extended review and a software tool. PloS One 12, e0190152. doi: 10.1371/journal.pone.0190152
Davey, J. W., Blaxter, M. L. (2011). RADSeq: next-generation population genetics. Briefings Funct. Genomics 9, 416–423. doi: 10.1093/bfgp/elq031
Dobin, A., Davis, C. A., Schlesinger, F., Drenkow, J., Zaleski, C., Jha, S., et al. (2013). STAR: ultrafast universal RNA-seq aligner. Bioinformatics 29, 15–21. doi: 10.1093/bioinformatics/bts635
Fedoroff, N. V. (2012). Transposable elements, epigenetics, and genome evolution. Science 338, 758–767. doi: 10.1126/science.338.6108.758
Feschotte, C., Jiang, N., Wessler, S. R. (2002). Plant transposable elements: where genetics meets genomics. Nat. Rev. Genet. 3, 329–341. doi: 10.1038/nrg793
Fuchs, J., Jovtchev, G., Schubert, I. (2008). The chromosomal distribution of histone methylation marks in gymnosperms differs from that of angiosperms. Chromosome Res. 16, 891–898. doi: 10.1007/s10577-008-1252-4
Girgis, H. Z. (2015). Red: an intelligent, rapid, accurate tool for detecting repeats de-novo on the genomic scale. BMC Bioinf. 16, 227. doi: 10.1186/s12859-015-0654-5
Guan, R., Zhao, Y., Zhang, H., Fan, G., Liu, X., Zhou, W., et al. (2016). Draft genome of the living fossil Ginkgo biloba. Gigascience 5, s13742–s13016. doi: 10.1186/s13742-016-0154-1
Hoff, K. J., Lomsadze, A., Borodovsky, M., Stanke, M. (2019). Whole-genome annotation with BRAKER. Gene Predict.: Methods Protoc. 1962, 65–95. doi: 10.1007/978-1-4939-9173-0_5
Islam-Faridi, M. N., Nelson, C. D., Kubisiak, T. L. (2007). Reference karyotype and cytomolecular map for loblolly pine (Pinus taeda L.). Genome 50, 241–251. doi: 10.1139/G06-153
Kim, D., Paggi, J. M., Park, C., Bennett, C., Salzberg, S. L. (2019). Graph-based genome alignment and genotyping with HISAT2 and HISAT-genotype. Nat. Biotechnol. 37, 907–915. doi: 10.1038/s41587-019-0201-4
Krueger, F., Kreck, B., Franke, A., Andrews, S. R. (2012). DNA methylome analysis using short bisulfite sequencing data. Nat. Methods 9, 145–151. doi: 10.1038/nmeth.1828
Lee, S.-G., Na, D., Park, C. (2021). Comparability of reference-based and reference-free transcriptome analysis approaches at the gene expression level. BMC Bioinf. 22, 1–9. doi: 10.1186/s12859-021-04226-0
Lim, K. Y., Kovarik, A., Matyasek, R., Chase, M. W., Clarkson, J. J., Grandbastien, M., et al. (2007). Sequence of events leading to near-complete genome turnover in allopolyploid Nicotiana within five million years. New Phytol. 175, 756–763. doi: 10.1111/j.1469-8137.2007.02121.x
Liu, H., Wang, X., Wang, G., Cui, P., Wu, S., Ai, C., et al. (2021). The nearly complete genome of Ginkgo biloba illuminates gymnosperm evolution. Nat. Plants 7, 748–756. doi: 10.1038/s41477-021-00933-x
Liu, Y., Wang, S., Li, L., Yang, T., Dong, S., Wei, T., et al. (2022). The Cycas genome and the early evolution of seed plants. Nat. Plants 8, 389–401. doi: 10.1038/s41477-022-01129-7
Mei, Y., Jing, D., Tang, S., Chen, X., Chen, H., Duanmu, H., et al. (2021). InsectBase 2.0: a comprehensive gene resource for insects. Nucleic Acids Res. 50, D1040–D1045. doi: 10.1093/nar/gkab1090
Moffat, A. S. (2000). Transposons help sculpt a dynamic genome. Science 289, 1455–1457. doi: 10.1126/science.289.5484.1455
Neale, D. B., Zimin, A. V., Zaman, S., Scott, A. D., Shrestha, B., Workman, R. E., et al. (2022). Assembled and annotated 26.5 Gbp coast redwood genome: a resource for estimating evolutionary adaptive potential and investigating hexaploid origin. G3 12, jkab380. doi: 10.1093/g3journal/jkab380
Niu, S., Li, J., Bo, W., Yang, W., Zuccolo, A., Giacomello, S., et al. (2022). The Chinese pine genome and methylome unveil key features of conifer evolution. Cell 185, 204–217. doi: 10.1016/j.cell.2021.12.006
Novák, P., Guignard, M. S., Neumann, P., Kelly, L. J., Mlinarec, J., Koblížková, A., et al. (2020). Repeat-sequence turnover shifts fundamentally in species with large genomes. Nat. Plants 6, 1325–1329. doi: 10.1038/s41477-020-00785-x
Nystedt, B., Street, N. R., Wetterbom, A., Zuccolo, A., Lin, Y.-C., Scofield, D. G., et al. (2013). The Norway spruce genome sequence and conifer genome evolution. Nature 497, 579–584. doi: 10.1038/nature12211
Pellicer, J., Fay, M. F., Leitch, I. J. (2010). The largest eukaryotic genome of them all? Bot. J. Linn. Soc. 164, 10–15. doi: 10.1111/j.1095-8339.2010.01072.x
Pham, G. M., Hamilton, J. P., Wood, J. C., Burke, J. T., Zhao, H., Vaillancourt, B., et al. (2020). Construction of a chromosome-scale long-read reference genome assembly for potato. Gigascience 9, giaa100. doi: 10.1093/gigascience/giaa100
Project, A. G., Albert, V. A., Barbazuk, W. B., dePamphilis, C. W., Der, J. P., Leebens-Mack, J., et al. (2013). The Amborella genome and the evolution of flowering plants. Science 342, 1241089. doi: 10.1126/science.1241089
Quinlan, A. R., Hall, I. M. (2010). BEDTools: a flexible suite of utilities for comparing genomic features. Bioinformatics 26, 841–842. doi: 10.1093/bioinformatics/btq033
Ren, X.-Y., Vorst, O., Fiers, M. W., Stiekema, W. J., Nap, J.-P. (2006). In plants, highly expressed genes are the least compact. Trends Genet. 22, 528–532. doi: 10.1016/j.tig.2006.08.008
Sahraeian, S. M. E., Mohiyuddin, M., Sebra, R., Tilgner, H., Afshar, P. T., Au, K. F., et al. (2017). Gaining comprehensive biological insight into the transcriptome by performing a broad-spectrum RNA-seq analysis. Nat. Commun. 8, 59. doi: 10.1038/s41467-017-00050-4
Scott, A. D., Stenz, N. W., Ingvarsson, P. K., Baum, D. A. (2016). Whole genome duplication in coast redwood (Sequoia sempervirens) and its implications for explaining the rarity of polyploidy in conifers. New Phytol. 211, 186–193. doi: 10.1111/nph.13930
Stark, R., Grzelak, M., Hadfield, J. (2019). RNA sequencing: the teenage years. Nat. Rev. Genet. 20, 631–656. doi: 10.1038/s41576-019-0150-2
Stenøien, H. (2007). Compact genes are highly expressed in the moss Physcomitrella patens. J. Evol. Biol. 20, 1223–1229. doi: 10.1111/j.1420-9101.2007.01301.x
Stival Sena, J., Giguère, I., Boyle, B., Rigault, P., Birol, I., Zuccolo, A., et al. (2014). Evolution of gene structure in the conifer Picea glauca: a comparative analysis of the impact of intron size. BMC Plant Biol. 14, 1–16. doi: 10.1186/1471-2229-14-95
Wagner, J., Olson, N. D., Harris, L., McDaniel, J., Cheng, H., Fungtammasan, A., et al. (2022). Curated variation benchmarks for challenging medically relevant autosomal genes. Nat. Biotechnol. 40, 672–680. doi: 10.1038/s41587-021-01158-1
Wan, T., Gong, Y., Liu, Z., Zhou, Y., Dai, C., Wang, Q. (2022). Evolution of complex genome architecture in gymnosperms. GigaScience 11, giac078. doi: 10.1093/gigascience/giac078
Wan, T., Liu, Z., Leitch, I. J., Xin, H., Maggs-Kölling, G., Gong, Y., et al. (2021). The Welwitschia genome reveals a unique biology underpinning extreme longevity in deserts. Nat. Commun. 12, 4247. doi: 10.1038/s41467-021-24528-4
Wan, T., Liu, Z.-M., Li, L.-F., Leitch, A. R., Leitch, I. J., Lohaus, R., et al. (2018). A genome for gnetophytes and early evolution of seed plants. Nat. Plants 4, 82–89. doi: 10.1038/s41477-017-0097-2
Wang, X., Ingvarsson, P. K. (2023). Quantifying adaptive evolution and the effects of natural selection across the Norway spruce genome. Mol. Ecol. 32, 5288–5304. doi: 10.1111/mec.17106
Xiong, X., Gou, J., Liao, Q., Li, Y., Zhou, Q., Bi, G., et al. (2021). The Taxus genome provides insights into paclitaxel biosynthesis. Nat. Plants 7, 1026–1036. doi: 10.1038/s41477-021-00963-5
Yang, T., Liu, R., Luo, Y., Hu, S., Wang, D., Wang, C., et al. (2022). Improved pea reference genome and pan-genome highlight genomic features and evolutionary characteristics. Nat. Genet. 54, 1553–1563. doi: 10.1038/s41588-022-01172-2
Keywords: gymnosperm, ultra-large genome, repeats, masked genome, sequencing read mapping
Citation: Zhu P, He T, Zheng Y and Chen L (2023) The need for masked genomes in gymnosperms. Front. Plant Sci. 14:1309744. doi: 10.3389/fpls.2023.1309744
Received: 08 October 2023; Accepted: 27 November 2023;
Published: 07 December 2023.
Edited by:
Jeffrey P. Mower, University of Nebraska-Lincoln, United StatesReviewed by:
Chung-Shien Wu, Academia Sinica, TaiwanCopyright © 2023 Zhu, He, Zheng and Chen. This is an open-access article distributed under the terms of the Creative Commons Attribution License (CC BY). The use, distribution or reproduction in other forums is permitted, provided the original author(s) and the copyright owner(s) are credited and that the original publication in this journal is cited, in accordance with accepted academic practice. No use, distribution or reproduction is permitted which does not comply with these terms.
*Correspondence: Lingyan Chen, ZmFmdWNseUBmYWZ1LmVkdS5jbg==
Disclaimer: All claims expressed in this article are solely those of the authors and do not necessarily represent those of their affiliated organizations, or those of the publisher, the editors and the reviewers. Any product that may be evaluated in this article or claim that may be made by its manufacturer is not guaranteed or endorsed by the publisher.
Research integrity at Frontiers
Learn more about the work of our research integrity team to safeguard the quality of each article we publish.