- 1State Key Laboratory for Conservation and Utilization of Subtropical Agro-bioresources, College of Life Science and Technology, Guangxi University, Nanning, Guangxi, China
- 2Department of Bioinformatics and Biotechnology, Government College University Faisalabad (GCUF), Faisalabad, Pakistan
- 3Key Laboratory of Biology and Genetic Improvement of Oil Crops, Ministry of Agriculture, Oil Crops Research Institute, Chinese Academy of Agricultural Sciences, Wuhan, China
- 4Environment and Plant Protection Institute, Chinese Academy of Tropical Agricultural Sciences, Haikou, China
- 5Department of Pharmacology and Toxicology, College of Pharmacy, King Saud University, Riyadh, Saudi Arabia
The nucleotide-binding site-leucine-rich repeat (NBS–LRR) gene family is the largest group of disease resistance (R) genes in plants and is active in response to viruses, bacteria, and fungi usually involved in effector-triggered immunity (ETI). Pangenome-wide studies allow researchers to analyze the genetic diversity of multiple species or their members simultaneously, providing a comprehensive understanding of the evolutionary relationships and diversity present among them. The draft pan-genome of three Mangifera indica cultivars (Alphonso, Hong Xiang Ya, and Tommy atkins) was constructed and Presence/absence variants (PAVs) were filtered through the ppsPCP pipeline. As a result, 2823 genes and 5907 PAVs from H. Xiang Ya, and 1266 genes and 2098 PAVs from T. atkins were added to the reference genome. For the identification of CC-NBS-LRR (CNL) genes in these mango cultivars, this draft pan-genome study has successfully identified 47, 27, and 36 members in Alphonso, H. Xiang Ya, and T. atkins respectively. The phylogenetic analysis divided MiCNL proteins into four distinct subgroups. All MiCNL genes are unevenly distributed on chromosomes. Both tandem and segmental duplication events played a significant role in the expansion of the CNL gene family. These genes contain cis-elements related to light, stress, hormone, and development. The analysis of protein-protein interactions (PPI) revealed that MiCNL proteins interacted with other defense-responsive proteins. Gene Ontology (GO) analysis indicated that MiCNL genes play a role in defense mechanisms within the organism. The expression level of the identified genes in fruit peel was observed under disease and cold stress which showed that Mi_A_CNL13 and 14 were up-regulated while Mi_A_CNL15, 25, 30, 31, and 40 were down-regulated in disease stress. On the other hand, Mi_A_CNL2, 14, 41, and 45 were up-regulated and Mi_A_CNL47 is down-regulated in cold stress. Subsequently, the Random Forest (RF) classifier was used to assess the multi-stress response of MiCNLs. It was found that Mi_A_CNL14 is a gene that responds to multiple stress conditions. The CNLs have similar protein structures which show that they are involved in the same function. The above findings provide a foundation for a deeper understanding of the functional characteristics of the mango CNL gene family.
1 Introduction
Plants have evolved various mechanisms to protect themselves from both biotic and abiotic stresses (Haak et al., 2017). When they are attacked by pathogens, such as bacteria, viruses, fungi, nematodes, and insects, plants activate their pathogen response mechanisms to prevent further harm (Baker et al., 2010). One key component of this defense system is the plant disease resistance (R) genes. These genes play a role in defense against pathogens and are triggered by pathogen signaling (Belkhadir et al., 2004). They can target specific pathogens and are typically encoded by a type of protein called a nucleotide-binding site-leucine-rich repeat (NBS-LRR) protein. The NBS domain of this protein contains three key motifs: the P-loop, kinase-2, and kinase-3a-binding nucleotide (Tameling et al., 2002). The LRR domain, which typically contains 20-30 amino acid residues, is made up of two segments: a highly conserved segment (HCS) and a variable segment (VS) (Matsushima and Miyashita, 2012). The NBS-LRR gene family is the largest class of R genes and plays multiple roles in host-pathogen recognition and downstream signaling transduction (Wan et al., 2012).
NBS-LRR proteins are a class of plant resistance (R) genes that play a crucial role in protecting plants against pathogens. These proteins are divided into two types based on their conserved functional domains: TIR-domain-containing (TNL) and non-TIR-domain-containing. The non-TIR-domain-containing type, also known as CC-NBS-LRR (CNLs), is characterized by the presence of a coiled-coil domain at the N-terminal instead of a TIR domain (Sukarta et al., 2016). Additionally, other domains such as zinc fingers or RPW8 domains may also be present in the N-terminal of CNL genes. CNL genes are found in both monocotyledons and dicotyledons and are widely present in plants (Tarr et al., 2009).
Furthermore, a large proportion of R genes (approx. 80%) encode the NBS-LRR domain, and more than 50 NBS genes have been shown to play a role in disease resistance (Song et al., 2015). Examples of NBS-LRR proteins include the Pi-ta gene in rice, which directly interacts with the Magnaporthe grisea effector AVR-Pita, and the RRS1 protein in Arabidopsis thaliana, which directly interacts with the bacterial wilt pathogen protein PopP2 (Jia et al., 2000; Deslandes et al., 2003). Additionally, RPS2 and RPM1 resistance genes in Arabidopsis respond to Pseudomonas syringae through indirect interaction with AvrRpm1 and AvrB (DeYoung and Innes, 2006; Gururani et al., 2012). Furthermore, the ectopic overexpression of the Arabidopsis RPW8 gene has been shown to enhance resistance to powdery mildew in grapevine (Hu et al., 2018).
Mangifera indica (Mango) belongs to the Anacardiaceae family, which comprises 73 genera and almost 850 species. This fruit grows in tropical and subtropical regions of the world. Mangoes are renowned for being a natural source of dietary fiber, vitamins, proteins, carbohydrates, and essential minerals. They also have a unique flavor and are very nutritious. Therefore, it is called as “King of Tropical Fruits”. Green, yellow, dark red, and orange are the skin colors of ripe mango fruits (Quintana et al., 2021). The mango’s genome was sequenced in 2020, opening up greater resources for molecular studies on this fruit (Wang et al., 2020). The pan-genome of a species encompasses a collection of genes that can be divided into three categories: core genes that are found in all members of the species, accessory genes that are present in some members but not all, and unique genes that are specific to certain individuals within the species. This concept refers to the genetic diversity within a species, rather than an individual genome.
Since CNLs are involved in the defense mechanism of plants against various pathogens including viruses, bacteria, and fungi, the identification of mango CNLs is necessary to understand their interaction mechanisms and to develop defense-resilient cultivars. Additionally, mangoes are traded internationally, and the presence of diseases can restrict exports due to phytosanitary regulations. Disease resistant mango varieties can open up new markets and enhance international trade opportunities.
In this study, only those mango cultivars were chosen that have both the genome and annotation files available. Using a draft pan-genome, the CNL gene family members were identified in three mango cultivars: Alphonso, Hong Xiang Ya, and Tommy atkins. The structural and functional characteristics, gene structure and motifs, chromosomal distribution, gene duplication, cis-regulatory elements, protein-protein interaction (PPI), and the expression pattern of Mi_A_CNLs at various conditions were analyzed. Furthermore, machine learning techniques were used to identify the multi-stress responsive genes. These results provide worthy clues for further analyzing the biological functions of MiCNLs in various other biotic and abiotic stresses.
2 Materials and methods
2.1 Construction of mango draft pan-genome
The published genomes of three Mangifera indica cultivars named Alphonso, H. Xiang Ya, and T. atkins were downloaded from the MangoBase database (https://mangobase.org/easy_gdb/index.php) (Gómez-Ollé et al., 2023) and a draft pan-genome was constructed based on presence-absence variations (PAVs) using ppsPCP: a plant presence/absence variants scanner and pan-genome construction pipeline (http://cbi.hzau.edu.cn/ppsPCP/) (Ul Qamar et al., 2019). PAVs are the types of Structural Variations (SVs) that are either present or absent in different organisms/genomes. Usually, plants have a PAV length of 100bp. The query genomes were iteratively mapped against reference genome using MUMmer and PAVs were harvested. Next, the harvested PAVs were validated with BLASTn search between the query and reference genomes. Finally, the boundaries of filtered PAVs were corrected and a draft pan-genome was established.
2.2 Identification and physiochemical characterization of mango CNLs
The 51 A. thaliana CNL protein sequences were retrieved from the Ensembl Plants database (https://plants.ensembl.org/index.html) and a tBLASTn search was performed against the draft pan-genome. From the coordinates of each blast hit, using a draft pan-genome GFF file the protein IDs were obtained and protein sequences were retrieved from the proteome of each cultivar. The identified proteins were further searched for the confirmation of the presence of the NB-ARC and LRR domains in Pfam (http://pfam-legacy.xfam.org/) (Bateman et al., 2004), InterPro (https://www.ebi.ac.uk/interpro/) (Hunter et al., 2009), Conserved Domains Database (CDD; https://www.ncbi.nlm.nih.gov/Structure/cdd/cdd.shtml) (Marchler-Bauer et al., 2015), and HMMER (https://www.ebi.ac.uk/Tools/hmmer/) (Finn et al., 2011) databases. In addition, the coiled-coils structure was confirmed on the Paircoil2 website (https://cb.csail.mit.edu/cb/paircoil2/paircoil2.html), and the P-value parameter was set as 0.025 (McDonnell et al., 2006). The proteins having no characteristic conserved domains were excluded from further analysis.
Physicochemical properties including the length of protein sequence (aa), molecular weight (MW), isoelectric point (pI), aliphatic index (AI), Instability index (II), and grand average of hydropathicity (GRAVY) values were predicted using ProtParam tool of Expasy server (https://web.expasy.org/protparam/) (Gasteiger et al., 2005). Additionally, subcellular localization of mango CNL proteins was predicted using an online WoLF PSORT tool (https://wolfpsort.hgc.jp/) (Horton et al., 2007).
2.3 Multiple sequence alignment, phylogenetic analysis, conserved motifs, and gene structure analysis of MiCNLs
To further evaluate the evolutionary link of CNL proteins, a multiple sequence alignment of 51 A. thaliana (AtCNLs), 33 Cucumis sativus L. (CsaCNLs), 10 Citrus sinensis (ScCNLs), 47 Alphonso (Mi_A_CNLs), 27 H. Xiang Ya (Mi_H_CNLs), and 36 T. atkins (Mi_T_CNLs) protein were completed using ClustalW program (Tamura et al., 2021), and a phylogenetic tree was constructed using IQTREE Web Server (http://iqtree.cibiv.univie.ac.at/) (Zameer et al., 2021). The reliability of the constructed tree was verified using 1000 bootstrapping replicates using the maximum likelihood (ML) method. The tree was further edited using the iTOL: Interactive Tree of Life (https://itol.embl.de/) (Letunic and Bork, 2021).
To find common motifs among each mango cultivar, the Multiple Expectation Maximization for Motif Elicitation tool (MEME, https://meme-suite.org/meme/) (Bailey et al., 2015) was applied using protein sequences. Except for setting the motif number to 20, the rest of the parameters were retained by default. TBtools was used to visualize the identified motifs. The GFF file of each mango cultivar was used to analyze the intron and exon pattern of MiCNL genes and the structures were displayed using TBtools (Chen et al., 2018).
2.4 Chromosomal localization, Ka/Ks, and gene duplication analysis
The chromosomal position of each MiCNL gene was acquired from the GFF file of the relative cultivar and mapped using the gene location visualization tool of TBtools software (Chen et al., 2018). MiCNL gene duplication events were determined based on whether the length of the shorter gene covered was equal to or greater than 70% of the longer gene and if the similarity of the two aligned genes was equal to or greater than 70% (Tsai et al., 2012). Tandem and segmental duplications are reported to be the two main mechanisms underlying gene family expansion. Genes located on the same chromosome fragment were considered to be tandem duplicated genes. Genes found to be co-paralogs located on duplicated chromosomal blocks were considered to be segmentally duplicated genes (Flagel and Wendel, 2009). Ka/Ks values can be used to predict selection pressure for replicating genes. DnaSP v.6 software (Rozas et al., 2017) was used to calculate the nonsynonymous (Ka) and synonymous (Ks) nucleotide substitution parameters. If the ratio of Ka/Ks was greater than, equal to, or less than one, this indicated positive, neutral, and purifying selection, respectively (Zia et al., 2022). Moreover, the time of divergence for these gene pairs was calculated using the formula “t = Ks/2λ×10-6”, with λ value of 1.5× 10−8 for dicots to calculate the duplication time in million years (Zameer et al., 2022; Sadaqat et al., 2023).
2.5 Cis-regulatory elements, protein-protein interaction, and gene ontology enrichment analysis
As in the earlier studies, the cis-acting elements in the 2,000 bp upstream sequences in the genomic region of MiCNL genes were retrieved from the genome file using the “samtools faidx” tool in Ubuntu (Li et al., 2009; Hu et al., 2022; Xia et al., 2022; Zhu et al., 2022), and the types, numbers, and functions of these elements were analyzed using PlantCARE database (https://bioinformatics.psb.ugent.be/webtools/plantcare/html/) (Rombauts et al., 1999). Cis-elements were visualized using TBtools software.
Protein sequences of MiCNL were used as input in the STRING database (https://string-db.org/) (Mering et al., 2003) for analyzing PPI. For PPI the level of connection used was tenth and other parameters were kept by default. The PPI network was visualized and edited using Cytoscape software (Shannon et al., 2003). GO enrichment analysis was done using the DAVID database (https://david.ncifcrf.gov/home.jsp) (Dennis et al., 2003) and the components considered were biological processes (BP), cellular components (CC), and molecular function, and KEGG pathways.
2.6 Tissue specific analysis and 3D structure prediction of Mi_A_CNLs
The expression levels of all Mi_A_CNL genes under disease and cold stress were evaluated using transcriptome datasets available at the NCBI Sequence Read Archive (SRA) database (https://www.ncbi.nlm.nih.gov/sra) (Kodama et al., 2012) under BioProject: PRJNA855362 and PRJNA304093 respectively. The genome and annotation files (GFF) were downloaded from the MangoBase database (https://mangobase.org/easy_gdb/index.php) (Gómez-Ollé et al., 2023). The reads quality was checked through the FastQC tool (Brown et al., 2017). Indexes of M. indica (Alphonso) genome sequences were built using Bowtie2 (Langdon, 2015) and high-quality paired-end reads were mapped to the genome. The Htseq-count (Anders et al., 2015) program used abundance estimation of annotated genes. Finally, count values of individual genes were used to generate the heatmap which was illustrated using TBtools software.
To function properly, proteins are needed to be folded into a proper three-dimensional structure. Based on expression patterns, four Mi_A_CNL proteins were selected to predict their structures. Alphafold2 (https://rb.gy/dlamz) was used for this purpose (Jumper et al., 2021). Further, the predicted structures were validated using SAVES (https://saves.mbi.ucla.edu/) (Sawal et al., 2023) and MolProbity (http://molprobity.biochem.duke.edu/) (Davis et al., 2007). PyMOL was used to visualize these structures (Alexander et al., 2011).
2.7 Prediction of multi-stress responsive genes using machine learning
DESeq2 was utilized to investigate both disease and cold stress samples to identify genes with significant expression changes (Anders and Huber, 2012). Based on statistical significance, the identified genes were screened based on their p-value < 0.05 and log2 fold change values (a log2FC value ≥ 0.5 for upregulation, and log2FC ≤ -0.5 for downregulation). Common CNL genes from both datasets were used for testing. To verify the validity of these genes, the random forest (RF) classification algorithm was applied within the R programming environment (Qi, 2012). Model performance assessment usually involves a comparison of the model’s predictions with the known values of the dependent variable within a specific dataset. Count values of disease datasets were taken to train the model and common genes were used for testing. Performance metrics such as accuracy, area under the receiver operating characteristic curve (AUC), specificity, and sensitivity were used to evaluate the effectiveness of the RF classifier, specifically on the dataset containing common multi-stress responsive gene.
3 Results
3.1 Draft pan-genome of three mango cultivars
Three mango genomes of cultivars: Alphonso, H. Xiang Ya, and T. atkins were used to construct a draft pan-genome through ppsPCP. The Alphonso genome was selected as a reference based on its quality and completeness, while H. Xiang Ya and T. atkins were mapped iteratively against the selected reference genome. In the first iteration, the H. Xiang Ya genome contributed 5907 PAVs and 2823 new genes to the reference genome. While, in the second iteration, T. atkins contributed 2092 PAVs and 1266 new genes to the developing draft pan-genome (Table S1). In total, 7999 novel PAVs and 4089 new genes were added to the reference genome and a draft pan-genome assembly was established (Figure 1). The total draft pan-genome assembly size was 470 MB, with a total of 39843 genes in its annotation file. The draft pan-genome assembly fasta (.fa) and annotation (.gff3) files are given in Supplementary Material.
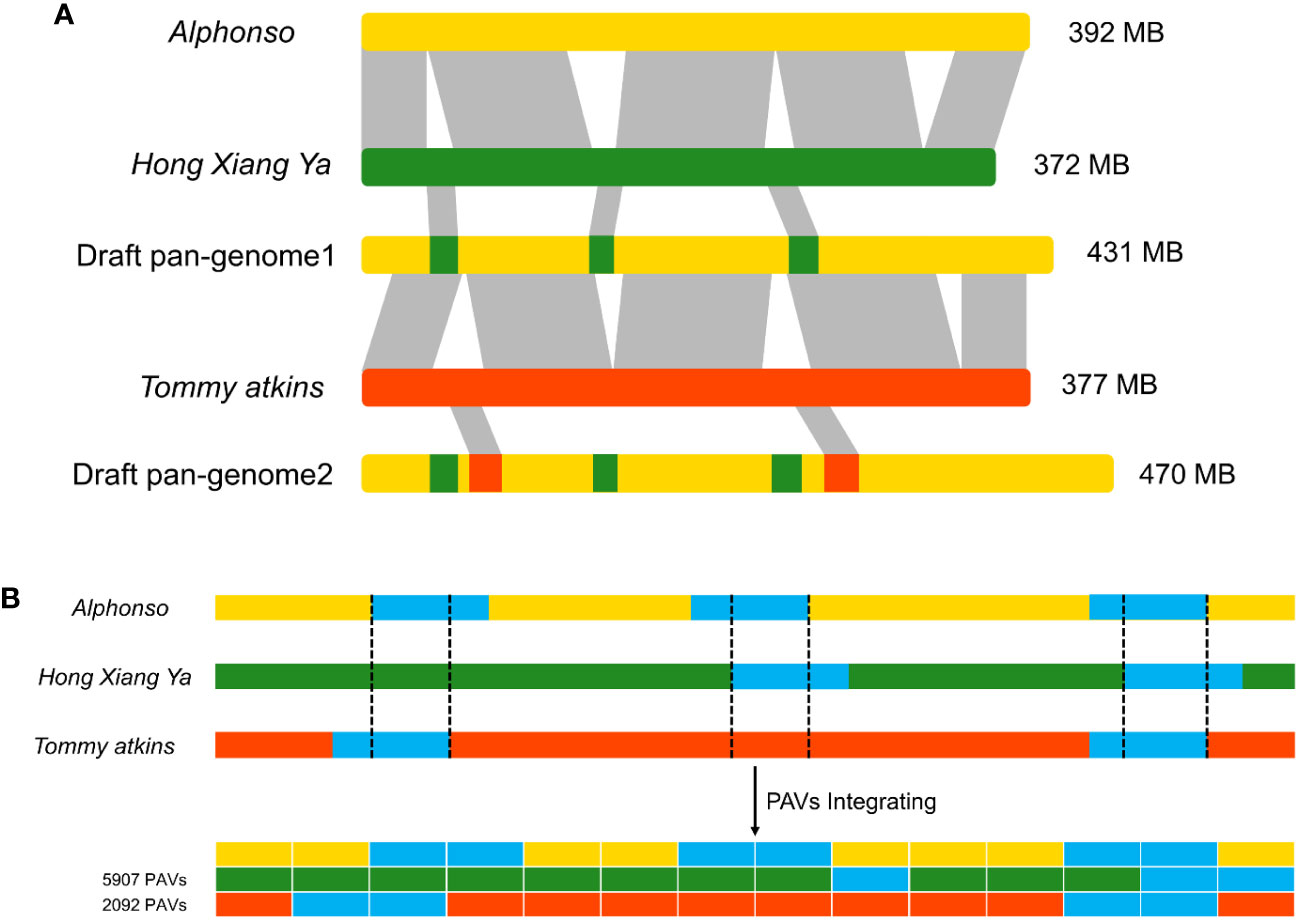
Figure 1 Construction of linearized draft pan-genome. (A) Genomes used for draft pan-genome construction are iteratively aligned between genomes and the starting reference to identify novel segments, then integrate these sequences into the reference genome to construct a draft pan-genome. (B) PAVs scanning and genotyping in the draft pan-genome.
3.2 Identification and physiochemical characteristics of CNL genes in Mangifera indica cultivars
A total of 47, 27, and 36 CNL genes were identified from the genomes of Alphonso (Mi_A_CNLs), H. Xiang Ya (Mi_H_CNLs), and T. atkins (Mi_T_CNLs), respectively. All of the identified MiCNLs were also confirmed for the presence of coil-coil, NB-ARC, and LRR domains (Table S2). The CNLs in Mangifera indica cultivars were relatively less than A. thaliana, Oryza sativa, Medicago truncatula, Helianthus annuus L., and Dioscorea rotundata but higher than C. sinensis, Brassica rapa, Cucumis sativus, and Raphanus sativus (Figure 2).
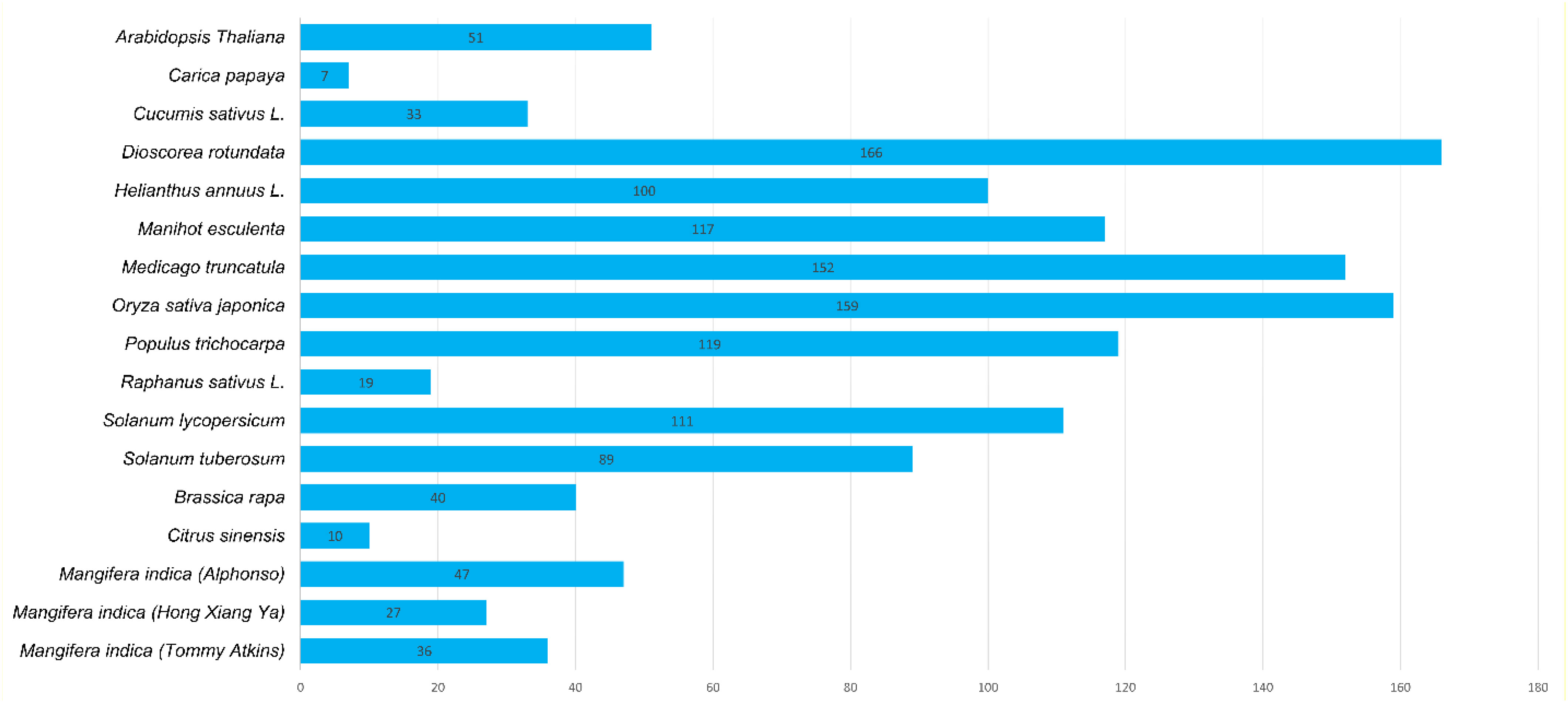
Figure 2 Identified CNL gene family members from Mangifera indica cultivars and other plant species.
The protein names of each cultivar were named from CNL1 onward according to their position on chromosomes, from Chr1 to Chr20 (Table 1).
The physical and chemical properties of all MiCNL proteins were analyzed (Table S3). There were no significant differences in amino acid residue number, molecular weights, isoelectric point instability index, aliphatic index, and GRAVY among the three cultivars. In all cultivars, most of the proteins have an isoelectric point (pI) less than 7 indicating that these proteins have acidic behavior. The instability index (II) values of most proteins indicated that these are unstable in the test tube. Most of the proteins have an aliphatic index (AI) greater than 70 which indicates that these proteins are thermally stable, and negative GRAVY values indicate that these proteins are hydrophilic (Figure 3). The protein’s subcellular localization shows that most of the proteins were present in the cytoplasm and nucleus. Few proteins were present in the chloroplast and endoplasmic reticulum (Table 1).
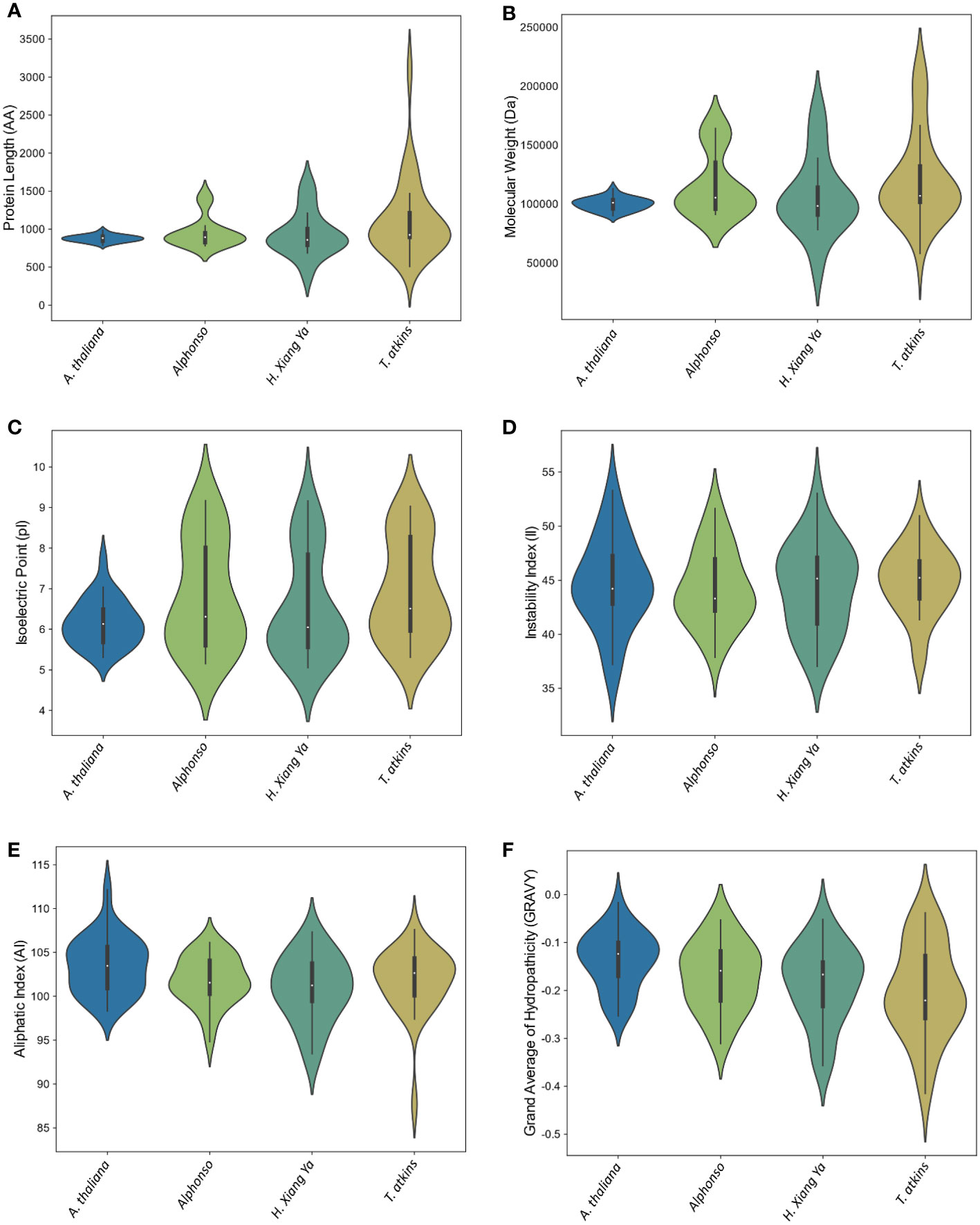
Figure 3 Violin plot of physiochemical properties of A thaliana and three M. indica cultivars. (A) Protein length, (B) Molecular weight, (C) Isoelectric point, (D) Instability index, (E) Aliphatic Index, and (F) Grand average of hydropathicity (GRAVY).
3.3 Phylogenetic relationships of CNL family members from three M. indica cultivars
To analyze the possible evolutionary relationship of the CNL gene family in M. indica cultivars, a phylogenetic tree was constructed using 204 amino acid sequences from six species. All CNL proteins were clustered into four groups. In comparison, group C contained the most CNL gene family members including 27 Mi_A_CNLs, 9 Mi_H_CNLs, 25 Mi_T_CNLs, 7 AtCNLs, 5 CsCNLs, and 23 CsaCNLs followed by group B which contain 13 Mi_A_CNLs, 10 Mi_H_CNLs, 8 Mi_T_CNLs, 23 AtCNLs, 5 CsCNLs, and 7 CsaCNLs. Group A contains 7 Mi_A_CNLs, 5 Mi_H_CNLs, 3 Mi_T_CNLs, 5 AtCNLs, and 3 CsaCNLs. Group D had only 3 members of Mi_H_CNLs and 16 members of AtCNLs. No member of Mi_A_CNLs, Mi_T_CNLs, CsCNLs and CsaCNLs was present in group D (Figure 4).

Figure 4 A maximum likelihood (ML) phylogenetic tree of CNL protein sequences from A. thaliana (At), C. sativus (Csa), C. sinensis, M. indica Alphonso (Mi_A_CNLs), M. indica H. Xiang Ya (Mi_H_CNLs), and M. indica T. atkins (Mi_T_CNLs). Different colors of branches represent different groups.
3.4 Conserved motifs, and gene structure analysis of MiCNLs
Overall, 20 motifs were chosen to analyze the pattern of conserved motifs among the MiCNLs. These motifs were identified through annotation from the Pfam database. The NBS domain consists of 8 motifs. Specifically, motif 1 was identified as the P-loop (Kinase a), motif 3 as GLPL, motif 4 as RNBS-D, motif 6 as MHD, motif 7 as Kinase-2, motif 8 as RNBS-C, motif 10 as RNBS-A, motif 13 as LRR. Out of 20, a total of 12 motifs (1, 3, 4, 6, 7, 8, 9, 10, 11, 13, 14, and 19) were conserved in all proteins of Alphonso. Motifs 2 and 12 were only conserved in the members of group C. Motif 5 was conserved in all proteins except the proteins of group A (Figure 5A). In H. Xiang Ya 8 motifs (1,2,3,4,5,6,7, and 8) were conserved in all proteins expects 2 proteins (Mi_H_CNL21 and Mi_H_CNL23). Motif 18 was only conserved in group B (Figure S1A). In T. atkins 6 motifs (1, 2, 3, 8, 9, and 12) were conserved among all members. Motifs 5 and 16 were only conserved in the members of group C (Figure S2A).
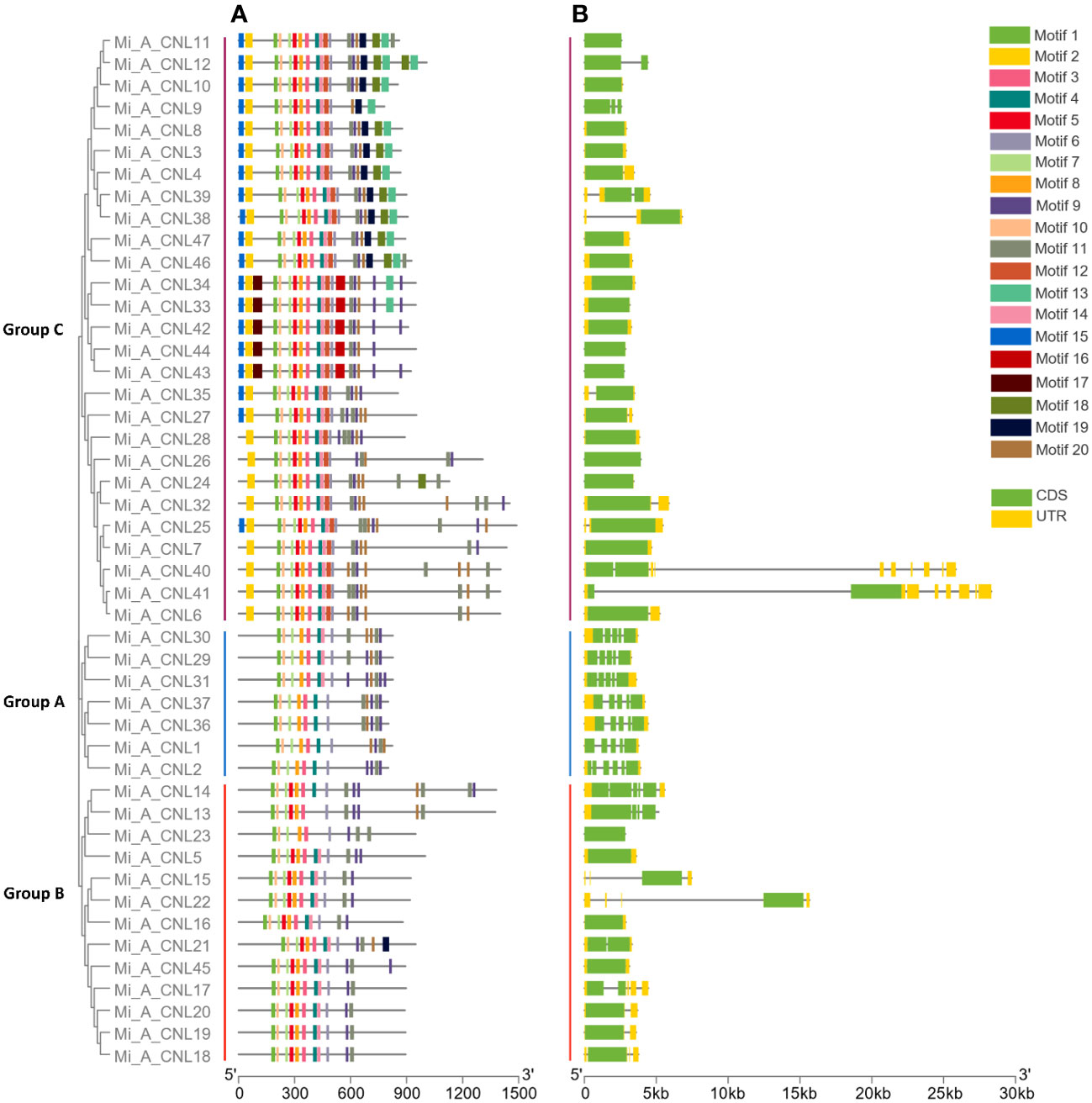
Figure 5 Phylogenetic tree, motif pattern, and gene structure of Mi_A_CNLs constructed using TBtools software. (A) Conserved motifs were determined using the MEME suite. (B) Gene structure was determined to show conservation among genes.
In Alphonso, gene structure varies from one group to another group. In group A, all the members have 5 exons and 4 introns. Group B has 1-4 exons and 0-3 introns, while members of Group C have 1-2 exons and 0-1 introns. Most of the members in group C have only 1 exon and no intron (Figure 5B). In H. Xiang Ya group A exons ranged from 3-13 and introns ranged from 2-12. Group B has 2-5 exons and 1-4 introns. Group C has 1-4 exons and 0-3 introns, while all members of Group D have only 2 exons and 1 intron (Figure S1B). In group A, T. atkins exons had a range from 5-13 and introns had a range from 4-12. Most of the members of group B had 1 exon but few members had a range of 1-3 exons and 0-2 introns. Members of group C have 1-15 exons and 0-14 introns (Figure S2B).
3.5 Chromosomal mapping and gene duplication analysis
In Alphonso, 47 CNL genes were distributed unevenly on 15 out of 20 chromosomes. It had maximum genes (9) at Chr3, and minimum genes (1) at Chr5, 6, 7, 10, 12, and 18. There was no gene on Chr 8, 9, 13, 15, and 19 (Figure 6). In H. Xiang Ya 27 genes were mapped unevenly at 10 out of 20 chromosomes. In this maximum gene (5) were present at chromosome 10 and 12, and minimum gene (1) was present at chromosome 7, 16, and 18. No gene was present at chromosomes 1, 3, 5, 8, 9, 11, 13, 14, and 17 (Figure S3). In T. Atkins 36 genes were present on 13 out of 20 chromosomes and on the scaffold. Chr19 has the maximum number of genes (5 genes) and Chr2, 6, 13, 16, and 17 have minimum numbers of genes (1 gene) on each chromosome. Four genes Mi_T_CNL33-36 were present on the scaffold (10000001). No gene was present on Chr1, 5, 8, 9, 11, and 14 (Figure S4).
Gene duplication events were also analyzed among Mi_A_CNL, Mi_H_CNL, and Mi_T_CNL genes and a total of 37, 8, and 5 duplicated pairs of genes were found among all the members respectively. Most of the members were tandemly duplicated. On the other hand, a few members resulted from segmental duplication. Thus, in line with previous studies, these findings indicated that tandem, as well as segmental duplications, were the main factor causing the increase of the CNL gene family in M. indica cultivars (Table 2).
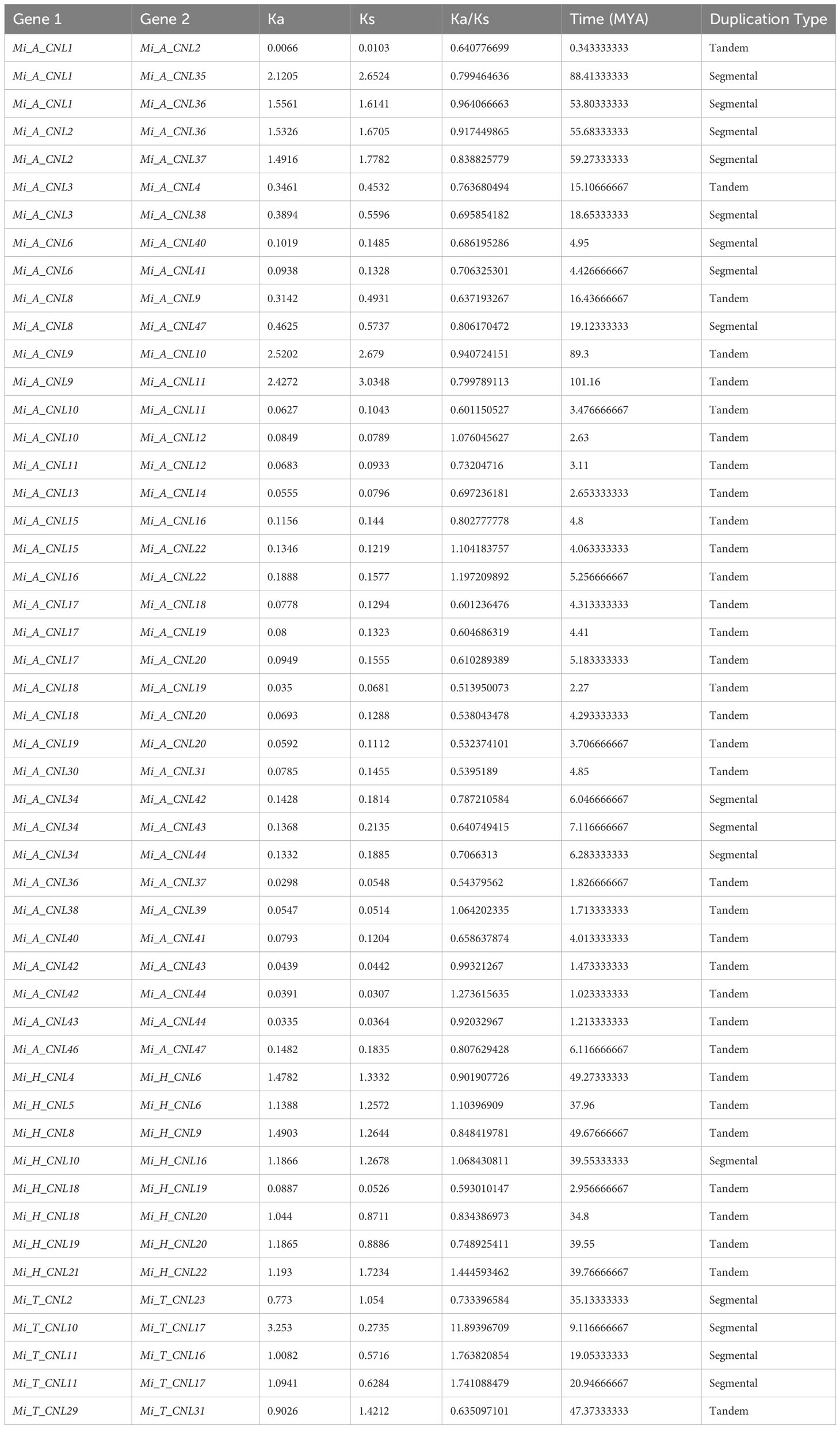
Table 2 Duplication data of three M. indica cultivars genes, rate of synonymous and non-synonymous mutations, duplication time (MYA), and type of duplication between genes.
To analyze the evolutionary constraints of the repeated MiCNL genes, the Ka, Ks, and Ka/Ks ratios of all para-homologous gene pairs were also calculated. In Mi_A_CNLs, Mi_H_CNLs, and Mi_T_CNLs gene pairs had Ka/Ks values ranging from 0.51 to 1.27, 0.59 to 1.44, and 0.63 to 11.89 respectively. Resultantly, the time of divergence of all 50 duplicated gene pairs of Mi_CNLs was between 0.3 to 88.4 million years (MYA).
3.6 Prediction of cis-regulatory elements in the promoter of MiCNL genes
The cis-regulatory elements were analyzed to further predict the involvement of MiCNL genes in the regulation of abiotic stresses. In all M. indica cultivars several cis-elements were found which were further classified into light-related, hormone-related, stress-related, and development-related elements (Table S4). Regarding these elements, for cis-elements Box 4, G-box, GT1-motif, and GATA-motif were found to be involved in light-stress regulation. Five cis-elements were involved with hormone responsiveness: ABRE, CGTCA-motif, TGA-element, P-box, and TCA-element. Further, four cis-elements were found to be involved with stress responsiveness: GC-motif, LTR, TC-rich repeats, and MBS. Five elements including CAT-box, MBSI, circadian, HD-Zip 1, and o2-site were involved in developmental processes. In Mi_A_CNLs light and stress-related cis-elements were mostly present (Figure 7) while in Mi_H_CNLs hormones-related cis-elements were mostly present (Figure S5). Mi_T_CNLs have mostly cis-elements related to hormones, stress, and development (Figure S6).
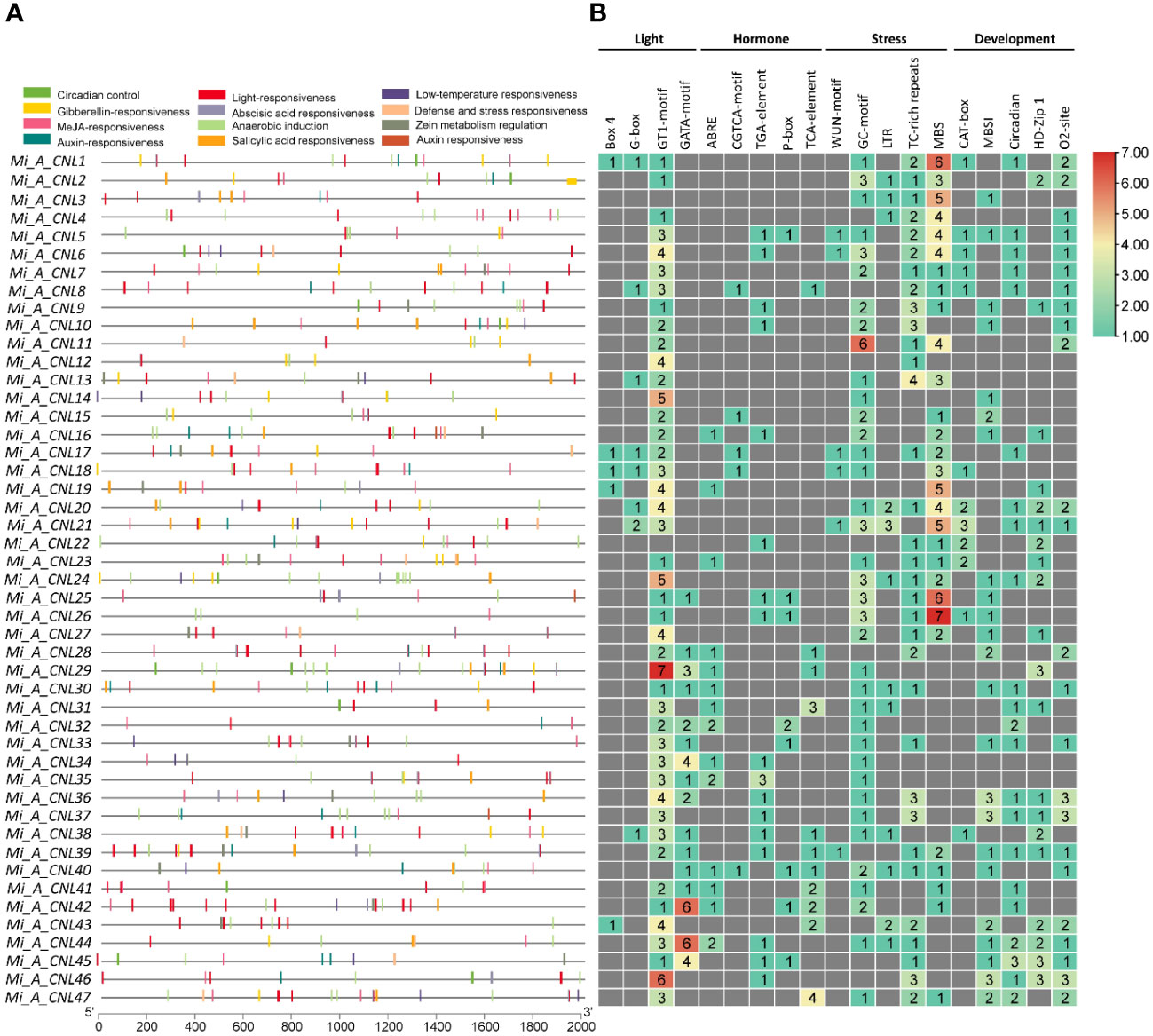
Figure 7 Cis-regulatory elements in the promoter region of Mi_A_CNL genes. (A) Represents the cis-elements and their location on the upstream region of genes. (B) Represents the heatmap with colors showing the number of elements related to various stresses.
3.7 PPI and gene ontology enrichment analysis
MiCNL proteins were evaluated to identify interactions among them to understand their functional interactions. Interacting proteins might be involved in a pathway, thus affecting the roles of other proteins and giving an overall response. Some MiCNL proteins were found to interact with the other CNL as well as the other homologous proteins. Among MiCNLs, Mi_T_CNLs showed the highest interactions. Mi_T_CNL18 and Mi_T_CNL12 were among the highly interacting proteins. Further, Mi_T_CNL9, Mi_T_CNL26, and Mi_H_CNL2 also showed great interactions with other defense-responsive proteins (Figure 8A).
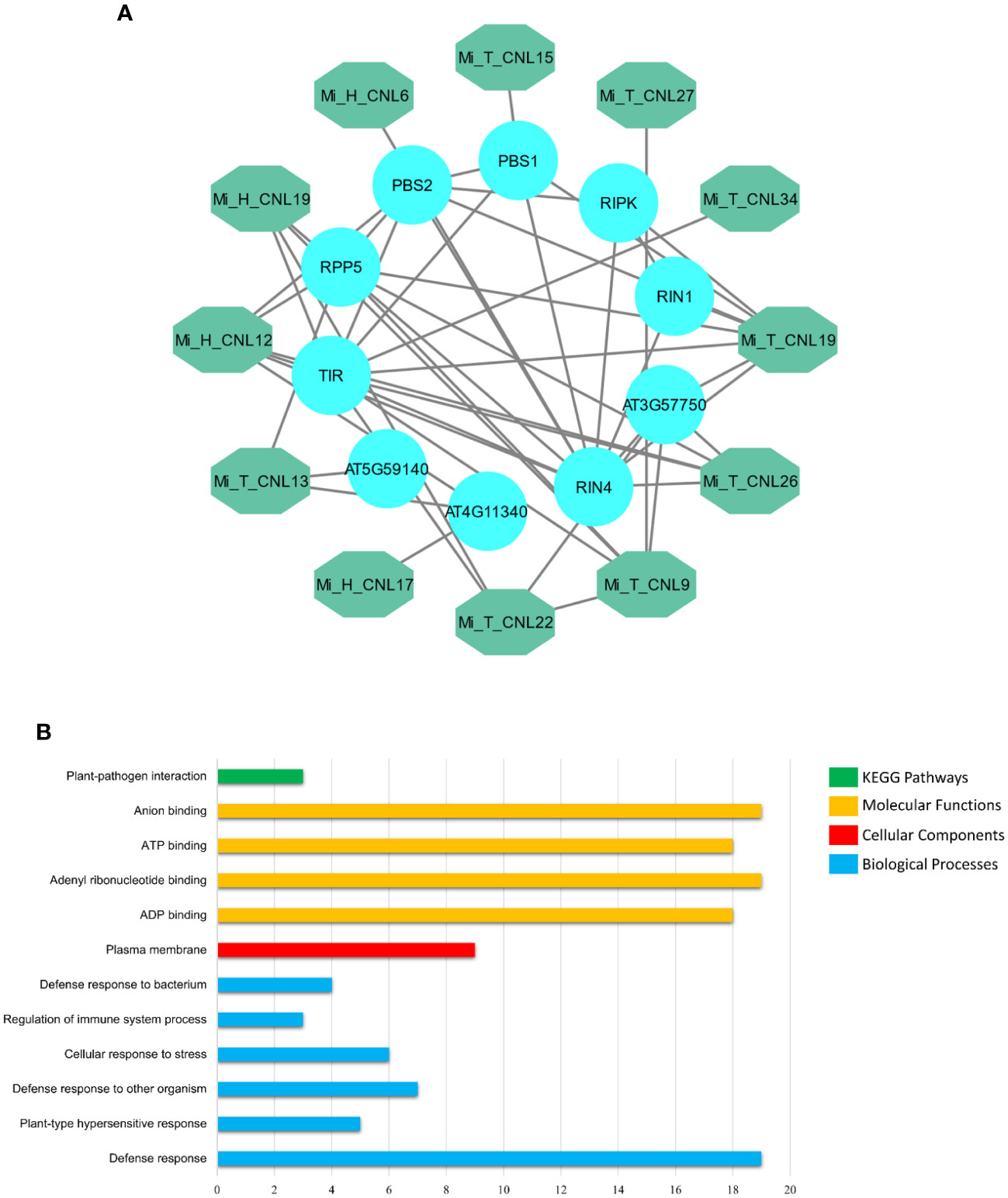
Figure 8 (A) Interactions among Mi_A_CNL and other homologous proteins. The teal color represents the Mi_A_CNL proteins and the cyan color represents the other interacting proteins from different species. (B) Predicted KEGG pathways, Molecular functions, Cellular components, and Biological Processes associated with Mi_A_CNL proteins.
Gene Ontology (GO) enrichment analysis was performed on the MiCNL genes. According to GO analysis, these genes were involved in a KEGG pathway: Plant pathogen interactions (GO: ath04626), Molecular functions including ADP binding (GO:0043531), Adenyl ribonucleotide binding (GO:0032559), ATP binding (GO:0005524) and Anion binding (GO:0043168). Moreover, these proteins were found to be in the plasma membrane (GO:0005886). These proteins also participate in a variety of biological processes including Defense response (GO:0006952), Plant-type hypersensitive response (GO:0009626), Defense response to other organisms (GO:0098542), Cellular response to stress (GO:0033554), Regulation of immune system process (GO:0002682), and Defense response to the bacterium (GO:0042742) (Table S5; Figure 8B).
3.8 Expression analysis of Mi_A_CNL genes
To further investigate the roles of these genes, their expression patterns were observed in disease and cold stress. In the disease stage, few genes showed fluctuated expression as Mi_A_CNL13 and Mi_A_CNL14 were up-regulated in fruit peel and Mi_A_CNL15, 25, 30, 31, 40 were down-regulated (Figure 9A). In cold stress Mi_A_CNL2, 14, 41, 45 were up-regulated and Mi_A_CNL47 is down-regulated (Figure 9B). Overall, the expression level of the remaining genes was found to be similar in each stress and condition.
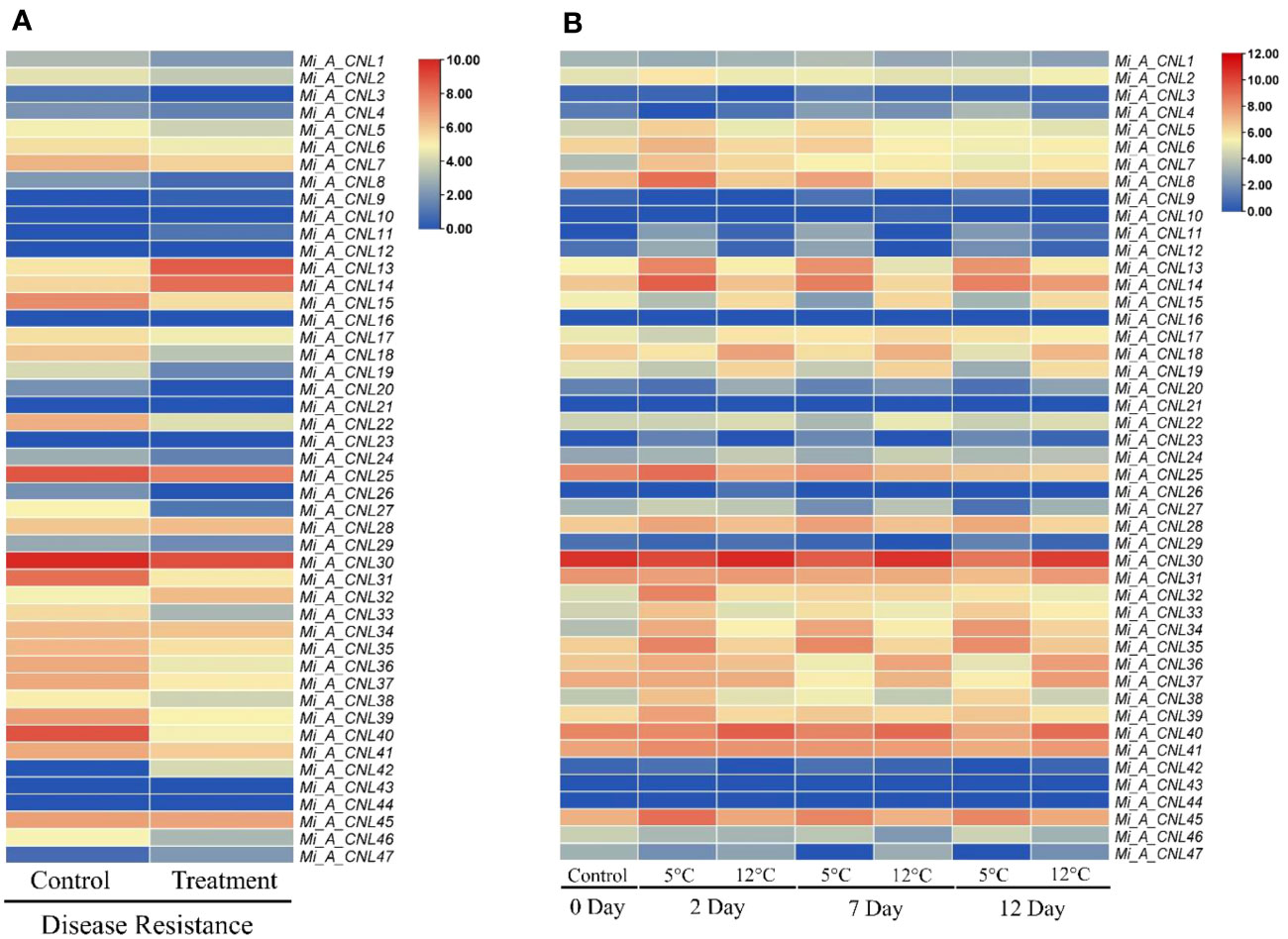
Figure 9 Heatmap regarding the expression pattern of Mi_A_CNL genes in fruit peel at different conditions constructed using count values. (A) Disease stress (B) Cold stress at 2, 7, and 12 days. The red color represents the up-regulated expression and the blue color represents the higher or upregulated expression.
3.9 Structure prediction of Mi_A_CNL proteins
To obtain more structural and ultimately functional insights, the 3D structures of four Mi_A_CNLs proteins (Mi_A_CNL13, 14, 25, and 30) were modeled. All these structures shared almost similar structures of loops, helices, and turns. All these structures contained a great number of helices. The basic structure was similar such as turns on the left side of structures (leucine-rich repeats) are visible in every modeled protein. Moreover, the number of helices in each protein is also the same (Figure 10).
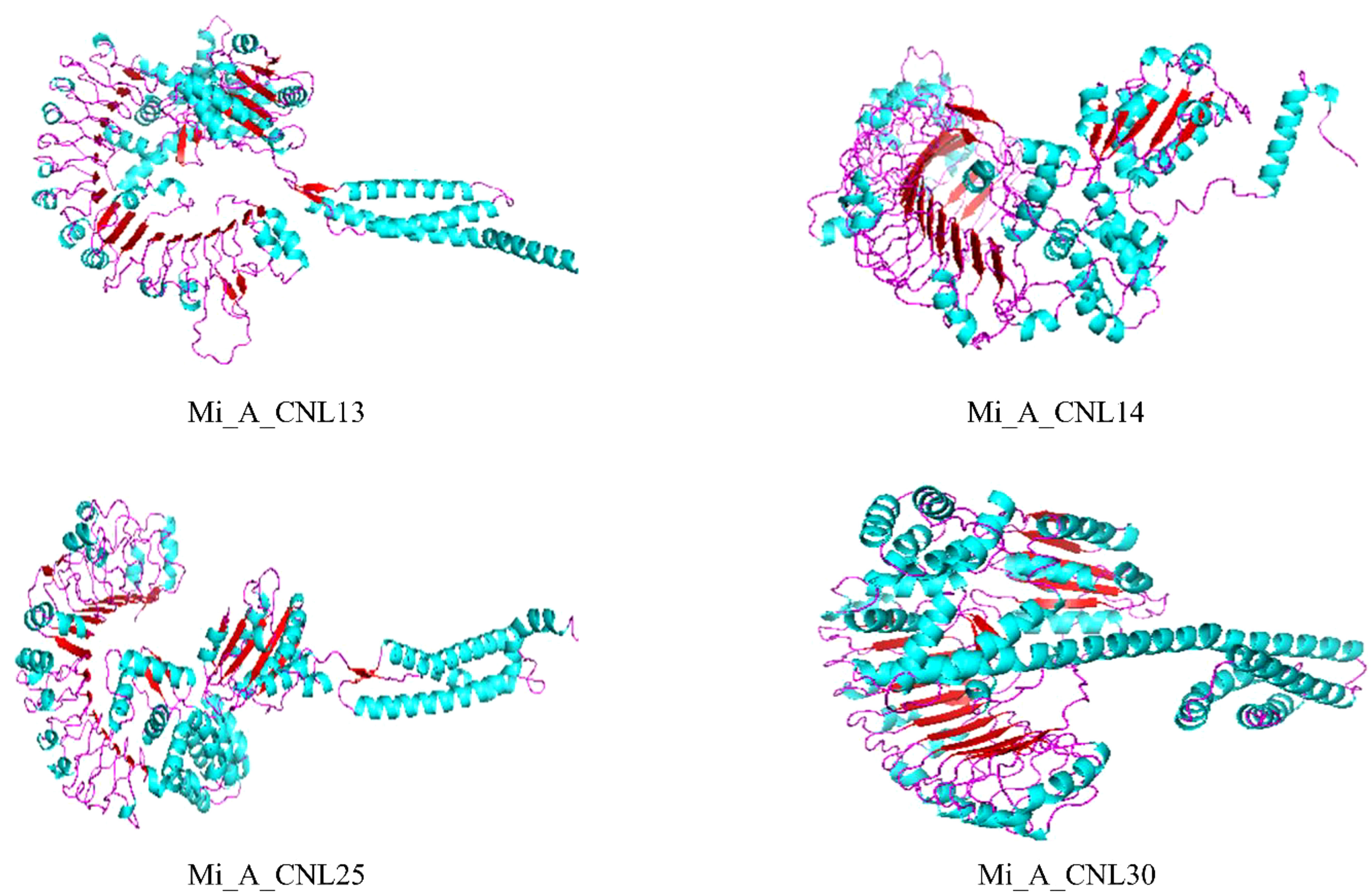
Figure 10 Predicted 3D structures of four Mi_A_CNLs using Alphaflold2 and visualized using PyMOL. Red color represents the helices, cyan color represents the sheets, and pink color represents the loops.
3.10 Performance evaluation of multi-stress responsive genes
A total of 15 genes were found to be present in both disease and cold datasets. A machine learning classifier, a random forest algorithm, was employed to assess their performance (Table 3). Using the count’s data of disease stress as the training dataset, it was analyzed that only one gene (Mi_A_CNL14) was rigorously tested for its multi-stress responsiveness. The classification model’s sensitivity, specificity, and overall accuracy were evaluated using the Receiver Operating Characteristic (ROC) plot. Impressively, Mi_A_CNL14 demonstrated a ROC value of 0.8333, indicating its acceptable performance as a potential multi-stress responsive gene. Figure S7 visually represents the ROC plot for Mi_A_CNL14, providing supporting evidence of its classification efficacy.
4 Discussion
Disease resistance R genes in plants are essential for effector-triggered immunity (ETI) because they have mechanisms for identifying pathogens in plants and protecting the plants directly or indirectly (Yang and Wang, 2015). The NBS-LRR class of these R genes, having most of the NBS and LRR domains at the C-terminal, encodes the largest family of all the five classes of these proteins (Meyers et al., 2003). Two major subfamilies of the NBS-LRR protein family are usually found: toll/interleukin-1 receptor-NBS-LRR (TNL) and coil-coil-NBS-LRR (CNL) (Shao et al., 2014).
In this study, three mango cultivars were analyzed to identify CNL genes in their genome by constructing a draft pan-genome. The CNL gene family is widespread in a variety of plant species such as C. sinensis (Yin et al., 2023), Carica papaya (Porter et al., 2009), C. sativus L. (Zhang et al., 2022), H. annuus L. (Neupane et al., 2018), O. sativa (Zhou et al., 2004), Populus trichocarpa (Kohler et al., 2008), Solanum lycopersicum (Andolfo et al., 2013), Solanum tuberosum (Jupe et al., 2012), and B. rapa (Liu et al., 2021). In this study 47, 27, and 36 CNL genes were found in Alphonso, H. Xiang Ya, and T. atkins respectively. The varying number of CC-NBS-LRR genes, specifically MiCNL genes, among three mango cultivars (ranging from 27 to 47) suggests intraspecific genomic diversity. This phenomenon may be attributed to factors such as genetic drift, environmental pressures, historical hybridization events, gene duplications, and transposon-mediated processes. Similarly, these members showed greater variation in numbers among different plants such as B. rapa which has 40 members [61]. The radish genome had 19 members [55]. Furthermore, A. thaliana has 51 identified members (Meyers et al., 2003).
Pan-genome wide analysis provides a comprehensive overview of diversity at the genomic level involving multiple species, which may lead to the identification of unique genes that are present in specific species instead of being present in all genomes under study (Tahir ul Qamar et al., 2020). Similarly, in this study, three unique genes were identified only in the H. Xiang Ya cultivar including Mi_H_CNL3, 12, and 13. The phylogenetic analysis categorized CNL genes into four groups (A, B, C, and D) using A. thaliana as a reference. The clade of Group C was the largest and Group D was the smallest. All of these genes belonging to the same subgroup were clustered together and shared the same homology, even members from the other species as well. However, none of the CNL genes from Alphonso and T. atkins were found in group D. Similarly, in the case of C. sativus L. no gene was present in group D (Zhang et al., 2022). H. Xiang Ya is the only cultivar that has three members in group D named Mi_H_CNL3, 12, and 13.
The conservation of motifs and gene structures was similar to the ones observed in previous studies like C. sinensis and B. rapa, in which very few such as one exon or intron were found. Similarly, the conservation of motifs among groups was also the same (Kohler et al., 2008). The observed differences in the number of exons and introns among mango cultivars and other species imply the evolutionary changes in gene structures over time, potentially impacting their functional conservation. This suggests diversification of CNL genes. Despite this variation in gene structure, most genes share a similar number of conserved motifs, indicating the preservation of their functions throughout evolution.
Chromosomal mapping indicates that the CNL genes in all three cultivars are distributed unevenly but among all cultivars, most of the genes are present in the form of clusters. The same trend was observed in A. thaliana (Meyers et al., 2003), C. sinensis (Yin et al., 2023), R. sativus L. (Ma et al., 2021), B. rapa (Liu et al., 2021), and O. sativa (Zhou et al., 2004). Most of these genes in three cultivars were found to have undergone tandem duplication. A similar pattern of duplication was observed in B. rapa (Ma et al., 2021) and C. sinensis (Yin et al., 2023). The evaluation of selection pressure on genes involved the use of the Ka/Ks ratio, which represents the ratio of non-synonymous (Ka) to synonymous (Ks) mutations. A Ka/Ks ratio greater than 1 indicates positive selection, while a ratio less than 1 signifies purifying selection. The analysis of mango cultivars revealed evidence of both positive and purifying selection acting on the studied genes.
The promoter region of these genes showed several stress-related elements that further confirm the involvement of these genes in different abiotic and disease-resistant stresses. Other plants have also been shown to have these elements which confer resistance to various environmental stresses. Black rot (BR) is a bacterial disease caused by Xanthomonas campestris pv. campestris dowson, which infects many Brassica species, such as cabbage (Brassica oleracea var), Chinese cabbage (Brassica pekinensis), and oil seed rape (Brassica campestris) (Zeilmaker et al., 2015; Zhang et al., 2018). All these findings help us understand the involvement of these genes in various stresses.
Protein-Protein interaction studies showed that few proteins from mango cultivars interacted with other defense-responsive proteins including TIR, RIN1, RIN4, PBS1, PBS2, and RPP5. GO analysis revealed that most CNL genes are located in the plasma membrane and involved in defense responses, ADP binding, ATP binding, anion binding, and adenyl ribonucleotide binding. In Vitis vinifera, NBS-LRR genes are also involved in defense responses, ADP binding, and ATP binding (Goyal et al., 2020).
The expression profiling of these genes showed their varied expression in disease and cold stress. The expression was analyzed in fruit peel. In response to disease stress, Mi_A_CNL13 and Mi_A_CNL14 were up-regulated, whereas Mi_A_CNL15, 25, 30, 31, and 40 were down-regulated. Conversely, under cold stress, Mi_A_CNL2, 14, 41, and 45 were up-regulated, while Mi_A_CNL47 was down-regulated. In B. rapa most of the CNL genes have the same trend but in C. sativus L. most of the CNL genes were up-regulated in salt and chilling (cold) stress (Liu et al., 2021; Zhang et al., 2022). Based on expression values the 3D structures of four proteins were also predicted to help understand their structural as well as functional conservations and all four proteins have almost the same number of alpha-helices and beta sheets.
Furthermore, random forest, the machine learning approach was utilized to evaluate the genes that were showing multi-stress responses in both disease and cold stress. A total of 15 genes were common in both datasets but only one gene (Mi_A_CNL14) was significantly involved in multi-stress response. Some other studies also utilized the same methods to evaluate genes involved in multi-stress response (Fatima et al., 2023). Therefore, it can be concluded that CNL genes can significantly benefit mango genetic improvement through breeding or genetic manipulation, by conferring disease resistance and enhancing tolerance to abiotic stresses. Their role in multi-stress responsiveness, as suggested by our analysis, makes them valuable candidates for further breeding programs seeking mango varieties with robust adaptability to diverse environmental conditions. Breeding for MiCNL gene related traits could lead to healthier mango plants, reduced pesticide dependency, and improved sustainability in mango cultivation.
5 Conclusion
In this study, a draft pan-genome was constructed and PAVs were scanned through the ppsPCP pipeline using three mango cultivars in which 47 genes in Alphonso, 27 in H. Xiang Ya, and 36 in T. atkins have been identified. These were classified into four groups: A, B, C, and D. All the members from the same group shared greater conservation in motif and gene structure. Few segmental and most tandemly duplicated pairs were found. A large number of cis-regulatory elements related to light, hormones, stress, and development responsive were found in promoter regions of mango CNLs. PPI showed CNL proteins interact with CNL and other defense-responsive proteins. and GO enrichment analysis revealed their interaction and involvement in pathways as well as processes related to defense response. Structure prediction showed high similarity among members of the same groups. Expression profiling of mango fruit peel under disease stress revealed that Mi_A_CNL13 and 14 were up-regulated while Mi_A_CNL15, 25, 30, 31, and 40 were down-regulated. On the other hand, in cold stress Mi_A_CNL2, 14, 41, 45 were up-regulated and Mi_A_CNL47 is down-regulated. Machine learning approaches indicate that out of 15 common genes, only one gene (Mi_A_CNL14) can be a multi-stress responsive gene (Super gene). Our results provide a solid foundation to further investigate the function of CNLs in regulating various abiotic and environmental stress responses and more accessions should be sequenced to improve the quality of the reference genome.
Data availability statement
The original contributions presented in the study are included in the article/Supplementary Material. Further inquiries can be directed to the corresponding authors.
Author contributions
MT: Conceptualization, Data curation, Formal Analysis, Funding acquisition, Investigation, Methodology, Project administration, Software, Visualization, Writing – original draft. MS: Data curation, Investigation, Methodology, Visualization, Writing – original draft. X-TZ: Data curation, Methodology, Software, Validation, Writing – review & editing. HL: Data curation, Investigation, Methodology, Validation, Writing – review & editing. XH: Conceptualization, Data curation, Methodology, Validation, Visualization, Writing – review & editing. KF: Data curation, Investigation, Methodology, Validation, Writing – review & editing. MA: Data curation, Formal analysis, Funding acquisition, Investigation, Resources, Validation, Writing – review & editing. L-LC: Conceptualization, Funding acquisition, Methodology, Project administration, Resources, Supervision, Validation, Writing – review & editing.
Funding
The author(s) declare financial support was received for the research, authorship, and/or publication of this article. This work was supported by the Starting Research Grant for High-level Talents from Guangxi University and Postdoctoral research platform grant of Guangxi University.
Acknowledgments
The researchers supporting project number (RSP2023R494), King Saud University, Riyadh, Saudi Arabia.
Conflict of interest
The authors declare that the research was conducted in the absence of any commercial or financial relationships that could be construed as a potential conflict of interest.
The author(s) declared that they were an editorial board member of Frontiers, at the time of submission. This had no impact on the peer review process and the final decision.
Publisher’s note
All claims expressed in this article are solely those of the authors and do not necessarily represent those of their affiliated organizations, or those of the publisher, the editors and the reviewers. Any product that may be evaluated in this article, or claim that may be made by its manufacturer, is not guaranteed or endorsed by the publisher.
Supplementary material
The Supplementary Material for this article can be found online at: https://www.frontiersin.org/articles/10.3389/fpls.2023.1285547/full#supplementary-material
References
Alexander, N., Woetzel, N., Meiler, J. (2011). “Bcl::Cluster: A method for clustering biological molecules coupled with visualization in the pymol molecular graphics system,” in 2011 IEEE 1st International Conference on Computational Advances in Bio and Medical Sciences, ICCABS. (Orlando, FL, USA: IEEE), 13–18. doi: 10.1109/ICCABS.2011.5729867
Anders, S., Huber, W. (2012). Differential Expression of RNA-Seq Data at the Gene Level–the DESeq Package Vol. 10 (Heidelberg, Germany: European Molecular Biology Laboratory (EMBL), f1000research.
Anders, S., Pyl, P. T., Huber, W. (2015). HTSeq—a python framework to work with high-throughput sequencing data. Bioinformatics 31 (2), 166–169. doi: 10.1093/bioinformatics/btu638
Andolfo, G., Sanseverino, W., Rombauts, S., de Peer, Y. V., Bradeen, J. M., Carputo, D., et al. (2013). Overview of tomato (Solanum lycopersicum) candidate pathogen recognition genes reveals important solanum R locus dynamics. New Phytol. 197 (1), 223–237. doi: 10.1111/j.1469-8137.2012.04380.x
Bailey, T. L., Johnson, J., Grant, C. E., Noble, W. S. (2015). The MEME suite. Nucleic Acids Res. 43 (W1), W39–W49. doi: 10.1093/nar/gkv416
Baker, C. M., Chitrakar, R., Obulareddy, N., Panchal, S., Williams, P., Melotto, M. (2010). Molecular battles between plant and pathogenic bacteria in the phyllosphere. Braz. J. Med. Biol. Res. 43 (8), 698–704. doi: 10.1590/S0100-879X2010007500060
Bateman, A., Coin, L., Durbin, R., Finn, R. D., Hollich, V., Griffiths-Jones, S., et al. (2004). The pfam protein families database. Nucleic Acids Res. 32 (suppl_1), D138–D141. doi: 10.1093/nar/gkh121
Belkhadir, Y., Subramaniam, R., Dangl, J. L. (2004). Plant disease resistance protein signaling: NBS-LRR proteins and their partners. Curr. Opin. Plant Biol. 7 (4), 391–399. doi: 10.1016/j.pbi.2004.05.009
Brown, J., Pirrung, M., McCue, L. A. (2017). FQC dashboard: integrates fastQC results into a web-based, interactive, and extensible FASTQ quality control tool. Bioinformatics 33 (19), 3137–3139. doi: 10.1093/bioinformatics/btx373
Chen, C., Xia, R., Chen, H., He, Y. (2018). TBtools, a toolkit for biologists integrating various HTS-data handling tools with a user-friendly interface. TBtools Toolkit Biologists Integrating Various HTS-Data Handling Tools User-Friendly Interface, 289660. doi: 10.1101/289660
Davis, I. W., Leaver-Fay, A., Chen, V. B., Block, J. N., Kapral, G. J., Wang, X., et al. (2007). MolProbity: all-atom contacts and structure validation for proteins and nucleic acids. Nucleic Acids Res. 35 (SUPPL.2), 375–383. doi: 10.1093/nar/gkm216
Dennis, G., Sherman, B. T., Hosack, D. A., Yang, J., Gao, W., Lane, H.C., et al. (2003). DAVID: database for annotation, visualization, and integrated discovery. Genome Biol. 4 (9), 1–11. doi: 10.1186/gb-2003-4-9-r60
Deslandes, L., Olivier, J., Peeters, N., Feng, D. X., Khounlotham, M., Boucher, C., et al. (2003). Physical interaction between RRS1-R, a protein conferring resistance to bacterial wilt, and popP2, a type III effector targeted to the plant nucleus. Proc. Natl. Acad. Sci. U.S.A. 100 (13), 8024–8029. doi: 10.1073/pnas.1230660100
DeYoung, B. J., Innes, R. W. (2006). Plant NBS-LRR proteins in pathogen sensing and host defense. Nat. Immunol. 7 (12), 1243–1249. doi: 10.1038/ni1410
Fatima, K., Sadaqat, M., Azeem, F., Rao, M. J., Albekairi, N. A., Alshammari, A., et al. (2023). Integrated omics and machine learning-assisted profiling of cysteine-rich-receptor-like kinases from three peanut spp. Revealed their role in multiple stresses. Front. Genet. 14. doi: 10.3389/fgene.2023.1252020
Finn, R. D., Clements, J., Eddy, S. R. (2011). HMMER web server : interactive sequence similarity searching. Nucleic Acid Res. 39, May, 29–37. doi: 10.1093/nar/gkr367
Flagel, L. E., Wendel, J. F. (2009). Gene duplication and evolutionary novelty in plants. New Phytol. 183 (3), 557–564. doi: 10.1111/j.1469-8137.2009.02923.x
Gasteiger, E., Hoogland, C., Gattiker, A., Wilkins, M. R., Appel, R. D., Bairoch, A. (2005). Protein identification and analysis tools on the exPASy server. Proteomics Protoc. Handb., 571–607. doi: 10.1385/1-59259-890-0:571
Gómez-Ollé, A., Bullones, A., Hormaza, J. I., Mueller, L. A., Fernandez-Pozo, N. (2023). MangoBase: A genomics portal and gene expression atlas for mangifera indica. Plants 12 (6), 1273. doi: 10.3390/plants12061273
Goyal, N., Bhatia, G., Sharma, S., Garewal, N., Upadhyay, A., Upadhyay, S. K., et al. (2020). Genome-wide characterization revealed role of NBS-LRR genes during powdery mildew infection in vitis vinifera. Genomics 112 (1), 312–322. doi: 10.1016/j.ygeno.2019.02.011
Gururani, M. A., Venkatesh, J., Upadhyaya, C. P., Nookaraju, A., Pandey, S. K., Park, Se W. (2012). Plant disease resistance genes: current status and future directions. Physiol. Mol. Plant Pathol. 78, 51–65. doi: 10.1016/j.pmpp.2012.01.002
Haak, D. C., Fukao, T., Grene, R., Hua, Z., Ivanov, R., Perrella, G., et al. (2017). Multilevel regulation of abiotic stress responses in plants. Front. Plant Sci. 8 (September). doi: 10.3389/fpls.2017.01564
Horton, P., Park, K.-J., Obayashi, T., Fujita, N., Harada, H., Adams-Collier, C. J., et al. (2007). WoLF PSORT: protein localization predictor. Nucleic Acids Res. 35 (suppl_2), W585–W587. doi: 10.1093/nar/gkm259
Hu, Y., Li, Y., Hou, F., Wan, D., Cheng, Y., Han, Y., et al. (2018). Ectopic expression of arabidopsis broad-spectrum resistance gene RPW8.2 improves the resistance to powdery mildew in grapevine (Vitis vinifera). Plant Sci. 267 (August 2017), 20–31. doi: 10.1016/j.plantsci.2017.11.005
Hu, H., Shi, B., Zhu, W., Zheng, B., Zhou, K., Qian, M., et al. (2022). Genome-wide identification, characterization and expression analysis of mango (Mangifera indica l.) chalcone synthase (CHS) genes in response to light. Horticulturae 8 (10), 968. doi: 10.3390/horticulturae8100968
Hunter, S., Apweiler, R., Attwood, T. K., Bairoch, A., Bateman, A., Binns, D., et al. (2009). InterPro: the integrative protein signature database. Nucleic Acids Res. 37 (suppl_1), D211–D215. doi: 10.1093/nar/gkn785
Jia, Y., McAdams, S. A., Bryan, G. T., Hershey, H. P., Valent, B. (2000). Direct interaction of resistance gene and avirulence gene products confers rice blast resistance. EMBO J. 19 (15), 4004–4014. doi: 10.1093/emboj/19.15.4004
Jumper, J., Evans, R., Pritzel, A., Green, T., Figurnov, M., Ronneberger, O., et al. (2021). Highly accurate protein structure prediction with alphaFold. Nature 596 (7873), 583–589. doi: 10.1038/s41586-021-03819-2
Jupe, F., Pritchard, L., Etherington, G. J., MacKenzie, K., Cock, P. J.A., Wright, F., et al. (2012). Identification and localization of the NB-LRR gene family within the potato genome. BMC Genomics 13 (1), 1–14. doi: 10.1186/1471-2164-13-75
Kodama, Y., Shumway, M., Leinonen, R. (2012). The sequence read archive: explosive growth of sequencing data. Nucleic Acids Res. 40 (D1), 2011–2013. doi: 10.1093/nar/gkr854
Kohler, A., Rinaldi, C., Duplessis, S., Baucher, M., Geelen, D., Duchaussoy, Frédéric, et al. (2008). Genome-wide identification of NBS resistance genes in populus trichocarpa. Plant Mol. Biol. 66 (6), 619–636. doi: 10.1007/s11103-008-9293-9
Langdon, W. B. (2015). “Performance of genetic programming optimized bowtie2 on genome comparison and analytic testing (GCAT) benchmarks.“. BioData Min. 8 (1), 1–7. doi: 10.1186/s13040-014-0034-0
Letunic, I., Bork, P. (2021). Interactive tree of life (ITOL) v5: an online tool for phylogenetic tree display and annotation. Nucleic Acids Res. 49 (W1), W293–W296. doi: 10.1093/nar/gkab301
Li, H., Handsaker, B., Wysoker, A., Fennell, T., Ruan, J., Homer, N., et al. (2009). The sequence alignment/map format and SAMtools. Bioinformatics 25 (16), 2078–2079. doi: 10.1093/bioinformatics/btp352
Liu, Y., Li, D., Yang, N., Zhu, X., Han, K., Gu, R., et al. (2021). Genome-wide identification and analysis of cc-nbs-lrr family in response to downy mildew and black rot in chinese cabbage. Int. J. Mol. Sci. 22 (8). doi: 10.3390/ijms22084266
Ma, Y., Chhapekar, S. S., Lu, L., Oh, S., Singh, S., Kim, C. S., et al. (2021). Genome-wide identification and characterization of NBS-encoding genes in raphanus sativus L. and their roles related to fusarium oxysporum resistance. BMC Plant Biol. 21 (1), 1–17. doi: 10.1186/s12870-020-02803-8
Marchler-Bauer, A., Derbyshire, M. K., Gonzales, N. R., Lu, S., Chitsaz, F., Geer, L. Y., et al. (2015). CDD: NCBI’s conserved domain database. Nucleic Acids Res. 43 (D1), D222–D226. doi: 10.1093/nar/gku1221
Matsushima, N., Miyashita, H. (2012). Leucine-rich repeat (LRR) domains containing intervening motifs in plants. Biomolecules 2 (2), 288–311. doi: 10.3390/biom2020288
McDonnell, A. V., Jiang, T., Keating, A. E., Berger, B. (2006). Paircoil2: improved prediction of coiled coils from sequence. Bioinformatics 22 (3), 356–358. doi: 10.1093/bioinformatics/bti797
Mering, C. v., Huynen, M., Jaeggi, D., Schmidt, S., Bork, P., Snel, B. (2003). STRING: A database of predicted functional associations between proteins. Nucleic Acids Res. 31 (1), 258–261. doi: 10.1093/nar/gkg034
Meyers, B. C., Kozik, A., Griego, A., Kuang, H., Michelmore, R. W. (2003). Genome-wide analysis of NBS-LRR-encoding genes in arabidopsis. Plant Cell 15 (4), 809–834. doi: 10.1105/tpc.009308
Neupane, S., Andersen, E. J., Neupane, A., Nepal, M. P. (2018). Genome-wide identification of NBS-encoding resistance genes in sunflower (Helianthus annuus L.). Genes 9 (8), 384. doi: 10.3390/genes9080384
Porter, B. W., Paidi, M., Ming, R., Alam, M., Nishijima, W. T., Zhu, Y. J. (2009). Genome-wide analysis of carica papaya reveals a small NBS resistance gene family. Mol. Genet. Genomics 281, 609–626. doi: 10.1007/s00438-009-0434-x
Qi, Y. (2012). “Random forest for bioinformatics.“. Ensemble Mach. Learning: Methods Appl. 307–323. doi: 10.1007/978-1-4419-9326-7_11
Quintana, S. E., Salas, S., García-Zapateiro, L. A. (2021). Bioactive compounds of mango (Mangifera indica): A review of extraction technologies and chemical constituents. J. Sci. Food Agric. 101 (15), 6186–6192. doi: 10.1002/jsfa.11455
Rombauts, S., Déhais, P., Montagu, M. V., Rouzé, P. (1999). PlantCARE, a plant cis-acting regulatory element database. Nucleic Acids Res. 27 (1), 295–296. doi: 10.1093/nar/27.1.295
Rozas, J., Ferrer-Mata, A., Sanchez-DelBarrio, J. C., Guirao-Rico, S., Librado, P., Ramos-Onsins, S. E., et al. (2017). DnaSP 6: DNA sequence polymorphism analysis of large data sets. Mol. Biol. Evol. 34 (12), 3299–3302. doi: 10.1093/molbev/msx248
Sadaqat, M., Umer, B., Attia, K. A., Abdelkhalik, A. F., Azeem, F., Javed, M. R., et al. (2023). Genome-wide identification and expression profiling of two-component system (TCS) genes in brassica oleracea in response to shade stress. Front. Genet. 14 (May). doi: 10.3389/fgene.2023.1142544
Sawal, H. A., Nighat, S., Safdar, T., Anees, L. (2023). Comparative in silico analysis and functional characterization of TANK-binding kinase 1–binding protein 1. Bioinf. Biol. Insights 17, 11779322231164828. doi: 10.1177/11779322231164828
Shannon, P., Markiel, A., Ozier, O., Baliga, N. S., Wang, J. T., Ramage, D., et al. (2003). Cytoscape: A software environment for integrated models of biomolecular interaction networks. Genome Res. 13 (11), 2498–2504. doi: 10.1101/gr.1239303
Shao, Z. Q., Zhang, Y. M., Hang, Y. Yu, Xue, J. Yu, Zhou, G. C., Wu, P., et al. (2014). Long-term evolution of nucleotide-binding site-leucine-rich repeat genes: understanding gained from and beyond the legume family. Plant Physiol. 166 (1), 217–234. doi: 10.1104/pp.114.243626
Song, W., Wang, B., Li, X., Wei, J., Chen, L., Zhang, D., et al. (2015). Identification of immune related LRR-containing genes in maize (Zea mays L.) by genome-wide sequence analysis. Int. J. Genomics. 2015, 11. doi: 10.1155/2015/231358
Sukarta, O. C.A., Slootweg, E. J., Goverse, A. (2016). Structure-informed insights for NLR functioning in plant immunity. Semin. Cell Dev. Biol. 56, 134–149. doi: 10.1016/j.semcdb.2016.05.012
Tahir ul Qamar, M., Zhu, X., Khan, M. S., Xing, F., Chen, L.-L. (2020). Pan-genome: A promising resource for noncoding RNA discovery in plants. Plant Genome 13 (3), e20046. doi: 10.1002/tpg2.20046
Tameling, W. I.L., Elzinga, S. D.J., Darmin, P. S., Vossen, J. H., Takken, F. L.W., Haring, M. A., et al. (2002). The tomato R gene products I-2 and mi-1 are functional ATP binding proteins with ATPase activity. Plant Cell 14 (11), 2929–2939. doi: 10.1105/tpc.005793
Tamura, K., Stecher, G., Kumar, S. (2021). MEGA11: molecular evolutionary genetics analysis version 11. Mol. Biol. Evol. 38 (7), 3022–3027. doi: 10.1093/molbev/msab120
Tarr, D., Ellen, K., Alexander, H. M. (2009). TIR-NBS-LRR genes are rare in monocots: evidence from diverse monocot orders. BMC Res. Notes 2, 1–10. doi: 10.1186/1756-0500-2-197
Tsai, Yu C., Weir, N. R., Hill, K., Zhang, W., Kim, H. J., Shiu, S. H., et al. (2012). Characterization of genes involved in cytokinin signaling and metabolism from rice. Plant Physiol. 158 (4), 1666–1684. doi: 10.1104/pp.111.192765
Ul Qamar, M. T., Zhu, X., Xing, F., Chen, L. (2019). PpsPCP: A plant presence/absence variants scanner and pan-genome construction pipeline. Bioinformatics 35 (20), 4156–4158. doi: 10.1093/bioinformatics/btz168
Wan, H., Yuan, W., Ye, Q., Wang, R., Ruan, M., Li, Z., et al. (2012). Analysis of TIR- and non-TIR-NBS-LRR disease resistance gene analogous in pepper: characterization, genetic variation, functional divergence and expression patterns. BMC Genomics 13 (1), 1–15. doi: 10.1186/1471-2164-13-502
Wang, P., Luo, Y., Huang, J., Gao, S., Zhu, G., Dang, Z., et al. (2020). The genome evolution and domestication of tropical fruit mango. Genome Biol. 21 (1), 1–17. doi: 10.1186/s13059-020-01959-8
Xia, L., He, X., Huang, X., Yu, H., Lu, T., Xie, X., et al. (2022). Genome-wide identification and expression analysis of the 14-3-3 gene family in mango (Mangifera indica L.). Int. J. Mol. Sci. 23 (3), 1593. doi: 10.3390/ijms23031593
Yang, X., Wang, J. (2015). Genome-wide analysis of NBS-LRR genes in sorghum genome revealed several events contributing to NBS-LRR gene evolution in grass species. Evol. Bioinf. 12, 9–21. doi: 10.4137/EBO.S36433
Yin, T., Han, P., Xi, D., Yu, W., Zhu, L., Du, C., et al. (2023). Genome-wide identification, characterization, and expression profile of NBS-LRR gene family in sweet orange (Citrus sinensis). Gene 854 (August 2022). doi: 10.1016/j.gene.2022.147117
Zameer, R., Fatima, K., Azeem, F., ALgwaiz, H. I.M., Sadaqat, M., Rasheed, A., et al. (2022). Genome-wide characterization of superoxide dismutase (SOD) genes in daucus carota: novel insights into structure, expression, and binding interaction with hydrogen peroxide (H2O2) under abiotic stress condition. Front. Plant Sci. 13 (June). doi: 10.3389/fpls.2022.870241
Zameer, R., Sadaqat, M., Fatima, K., Fiaz, S., Rasul, S., Zafar, H., et al. (2021). Two-component system genes in sorghum bicolor: genome-wide identification and expression profiling in response to environmental stresses. Front. Genet. 12 (November). doi: 10.3389/fgene.2021.794305
Zeilmaker, T., Ludwig, N. R., Elberse, J., Seidl, M. F., Berke, L., Doorn, A. V., et al. (2015). DOWNY MILDEW RESISTANT 6 and DMR 6-LIKE OXYGENASE 1 are partially redundant but distinct suppressors of immunity in arabidopsis. Plant J. 81 (2), 210–222. doi: 10.1111/tpj.12719
Zhang, B., Li, P., Su, T., Li, P., Xin, X., Wang, W., et al. (2018). BrRLP48, encoding a receptor-like protein, involved in downy mildew resistance in brassica rapa. Front. Plant Sci. 9, 1708. doi: 10.3389/fpls.2018.01708
Zhang, W., Yuan, Q., Wu, Y., Zhang, J., Nie, J. (2022). Genome-wide identification and characterization of the CC-NBS-LRR gene family in cucumber (Cucumis sativus L.). Int. J. Mol. Sci. 23 (9), 1–21. doi: 10.3390/ijms23095048
Zhou, T., Wang, Y., Chen, J. Q., Araki, H., Jing, Z., Jiang, K., et al. (2004). “Genome-wide identification of NBS genes in japonica rice reveals significant expansion of divergent non-TIR NBS-LRR genes.“. Mol. Genet. Genomics 271, 402–415. doi: 10.1007/s00438-004-0990-z
Zhu, J.-w., He, X.-h., Li, Y.-z., Zhang, Y.-l., Yu, H.-x., Xia, L.-m., et al. (2022). Genome-wide analysis of the mango SPL family and overexpression of miSPL13 confers early flowering and stress tolerance in transgenic arabidopsis. Scientia Hortic. 305, 111363. doi: 10.1016/j.scienta.2022.111363
Zia, K., Rao, M. J., Sadaqat, M., Azeem, F., Fatima, K., Qamar, M. T. ul, et al. (2022). Pangenome-wide analysis of cyclic nucleotide-gated channel (CNGC) gene family in citrus spp. Revealed their intraspecies diversity and potential roles in abiotic stress tolerance. Front. Genet. 13. doi: 10.3389/fgene.2022.1034921
Keywords: draft pan-genome, mango, NBS-LRR, multi omics, machine learning
Citation: Tahir ul Qamar M, Sadaqat M, Zhu X-T, Li H, Huang X, Fatima K, Almutairi MM and Chen L-L (2023) Comparative genomics profiling revealed multi-stress responsive roles of the CC-NBS-LRR genes in three mango cultivars. Front. Plant Sci. 14:1285547. doi: 10.3389/fpls.2023.1285547
Received: 30 August 2023; Accepted: 17 October 2023;
Published: 30 October 2023.
Edited by:
Shaojun Dai, Shanghai Normal University, ChinaCopyright © 2023 Tahir ul Qamar, Sadaqat, Zhu, Li, Huang, Fatima, Almutairi and Chen. This is an open-access article distributed under the terms of the Creative Commons Attribution License (CC BY). The use, distribution or reproduction in other forums is permitted, provided the original author(s) and the copyright owner(s) are credited and that the original publication in this journal is cited, in accordance with accepted academic practice. No use, distribution or reproduction is permitted which does not comply with these terms.
*Correspondence: Muhammad Tahir ul Qamar, m.tahirulqamar@hotmail.com; Ling-Ling Chen, llchen@gxu.edu.cn