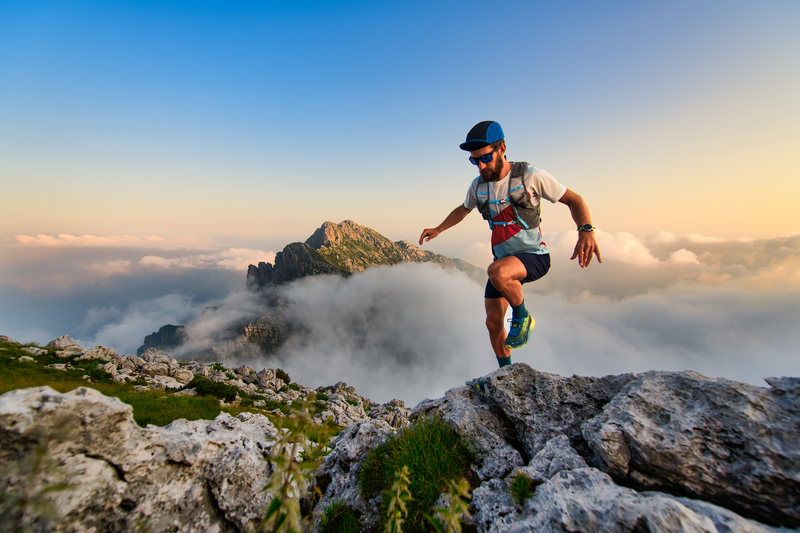
95% of researchers rate our articles as excellent or good
Learn more about the work of our research integrity team to safeguard the quality of each article we publish.
Find out more
ORIGINAL RESEARCH article
Front. Plant Sci. , 29 January 2024
Sec. Plant Biophysics and Modeling
Volume 14 - 2023 | https://doi.org/10.3389/fpls.2023.1283339
This article is part of the Research Topic Plant Architectural Models and Crop Production View all 9 articles
Breeding sorghum to withstand droughts is pivotal to secure crop production in regions vulnerable to water scarcity. Limited transpiration (LT) restricts water demand at high vapor pressure deficit, saving water for use in critical periods later in the growing season. Here we evaluated the hypothesis that LT would increase sorghum grain yield in the United States. We used a process-based crop model, APSIM, which simulates interactions of genotype, environment, and management (G × E × M). In this study, the G component includes the LT trait (GT) and maturity group (GM), the EW component entails water deficit patterns, and the MP component represents different planting dates. Simulations were conducted over 33 years (1986-2018) for representative locations across the US sorghum belt (Kansas, Texas, and Colorado) for three planting dates and maturity groups. The interaction of GT x EW indicated a higher impact of LT sorghum on grain for late drought (LD), mid-season drought (MD), and early drought (ED, 8%), than on well-watered (WW) environments (4%). Thus, significant impacts of LT can be achieved in western regions of the sorghum belt. The lack of interaction of GT × GM × MP suggested that an LT sorghum would increase yield by around 8% across maturity groups and planting dates. Otherwise, the interaction GM × MP revealed that specific combinations are better suited across geographical regions. Overall, the findings suggest that breeding for LT would increase sorghum yield in the drought-prone areas of the US without tradeoffs.
Droughts resulting from changes in precipitation patterns threaten crop production and food security in semiarid areas worldwide (Barbier, 2015). In the United States alone, crop yield loss due to droughts costs ~$9 billion per year (NOAA, 2020). In this respect, breeding for drought-prone environments plays a pivotal role in maintaining crop production (Thornton et al., 2018). Nevertheless, developing crops with less water demand is challenging because drought adaptation traits are complex, difficult to identify, and often involve tradeoffs (Araus et al., 2012; Monneveux et al., 2012). Furthermore, testing the effect of these traits under water stress scenarios is limited since drought events vary over time and geographies (Tang and Piechota, 2009; Pournasiri-Poshtiri et al., 2018). Thus, plant breeding programs require complementary methods to test the effect of any hypothetical drought adaptation trait to design a breeding pipeline (Cooper et al., 2002; Bernardo, 2020; Crossa et al., 2022).
Crop models have become standard tools to assess the impact of new technologies in agriculture and can support plant breeding (van Ittersum et al., 2003; Challinor et al., 2018). These models integrate ecophysiological knowledge to represent the plant-soil-atmosphere system and predict the crop response to soil properties, climatic conditions and crop management practices (Jones et al., 2003). Crop models equip breeding programs with the tools to develop and evaluate hypotheses regarding the performance of new cultivars (G) under environmental (E), and management scenarios (M) (Messina et al., 2011; Chenu et al., 2017). Several crop modeling studies have evaluated theoretical expressions of crop traits linked to cultivar-specific parameters for drought environment (Singh et al., 2014). The most common approach varies cultivar parameters (Messina et al., 2011; Singh et al., 2014) or implements new traits (Sinclair et al., 2005) to evaluate alternative ideotypes for constraint environments. This approach to crop improvement advantages investment of finite resources to defined targets for genetic gain in specific environments.
Sorghum is one of the most drought-adapted crops in semiarid regions used for multiple purposes, including forage, fiber, and food (Doggett and Majisu, 1968; Smith and Frederiksen, 2000). Most of the grain sorghum production worldwide (15%) is grown under rainfed environments in the sorghum belt of the United States that runs from South Dakota to South Texas (Laingen, 2015). Kansas, Texas, and Colorado lead grain sorghum production in the sorghum belt with 50%, 30%, and 6%, respectively (Laingen, 2015). Across this area, water limitation and high vapor pressure deficit (VPD) affect plant transpiration, making sorghum production vulnerable to droughts. Although sorghum harbors drought adaptation (Abdel-Ghany et al., 2020; Abreha et al., 2021), breeding for drought traits has received less attention. Therefore, the full potential of sorghum production under water-limited environments in the sorghum belt of the United States may not yet have been achieved.
Limited transpiration (LT) is a hypothetical trait that restricts water demand in periods of high VPD which occurs around mid-day (Figures 1A, B). This mechanism shifts plant-water demand, conserving water in the soil profile during the vegetative stage and for use during grain filling (Figure 1) (Sinclair et al., 2005). Reducing transpiration (H2O) due to stomatal closure in hours with high VPD would penalize carbon assimilation (CO2). Thus, causing grain yield reductions under well-watered conditions but increasing the grain yield and the effective use of water under moderate water-limited environments (Vadez et al., 2014). This hypothetical physiological mechanism of LT has been extended into process-based models where transpiration was restricted during high VPD hours (Sinclair et al., 2005; Messina et al., 2015; Truong et al., 2017). Crop model simulations under rainfed conditions for sorghum and other crops such as soybean, maize, lentil, chickpea, and wheat indicate a yield increase for a phenotype with LT trait in areas vulnerable to water scarcity (Sinclair et al., 2017; Collins et al., 2021). For sorghum, reports indicated an increase in yield production from 6 to 10% for severe drought scenarios in Australia, semiarid regions of India, and the United States (Texas) (Sinclair et al., 2005; Kholová et al., 2014; Truong et al., 2017).
Figure 1 Hypothetical effect of LT trait in grain sorghum under rainfed conditions. (A) Hourly trajectory of VPD during four days with contrasting, (B) Hourly trajectory of transpiration for sorghum with non-LT and LT traits (GT). The dashed gray line indicates the threshold of VPD at which genotypes express differences in transpiration VPD response. (C) Cumulative transpiration during the growing season, and (D) trajectory of grain yield during the growing season.
Simulations for various crops (Messina et al., 2015; Guiguitant et al., 2017; Collins et al., 2021) suggest that breeding for the LT trait can make a valuable economic contribution in rainfed regions. Yet, its impact on grain yield in sorghum-producing regions of the United States remains unknown. This study uses the APSIM-sorghum growth model to generate hypotheses of the potential benefits and tradeoffs of the LT trait in grain sorghum. Under drought scenarios, we expect an increase in grain yield in rainfed sorghum-producing regions for sorghum with the LT trait (Figure 1). Otherwise, no impacts or detrimental effects on grain yield are expected for non-stress environments. Likewise, we expect these benefits across different combinations of genetic background and management practices. Results indicate that introgressing LT in grain sorghum would increase yield by more than 5% in water-limited scenarios but less than 5% in well-watered settings. Additionally, the LT would benefit grain yield across all combinations of maturity groups and planting dates.
The simulation study was conducted for Kansas, Texas, and Colorado counties that have high sorghum production (Figure 2A) area and are located in contrasting gradients of precipitation and VPD (Table 1, Figure 2D). Across these locations annual precipitation and VPD are inversely associated (Figures 2B, C, E) with declining precipitation and increasing VPD from east to west (https://prism.oregonstate.edu/). Annual precipitation shapes farmer crop management including maturity group adoption (Roozeboom and Fjell, 1998; Shroyer et al., 1998; Ciampitti et al., 2019). Therefore, in these regions plant density of 17 plants m2 and 6 plants m2 are recommended for areas with annual precipitation around 800 mm and 350 mm, respectively (Shroyer et al., 1998). Similarly, full-season hybrids are planted in regions with high annual precipitation while short-season hybrids are grown in regions with low precipitation (Roozeboom and Fjell, 1998; Ciampitti et al., 2019).
Figure 2 Study system to evaluate the impact of the limited transpiration (LT) trait. (A) Geographic distribution of grain sorghum production area (ha-1) in the United States in 2019. (B) Annual precipitation (mm) in sorghum producing areas. (C) Mean of the monthly maximum vapor pressure deficit (VPD, kPa) from May to August in sorghum producing areas. (D) Distribution of grain sorghum in Colorado, Kansas, and Texas and sites for model evaluation and simulation sites. (E) Mean VPD and annual precipitation in sorghum producing regions. Sorghum production areas were obtained from the National Agricultural Statistical Service (NASS, https://www.nass.usda.gov/). Precipitation and vapor pressure deficit information for sorghum-producing areas were acquired from the PRISM Climate Group (https://prism.oregonstate.edu/).
APSIM-Sorghum (Hammer et al., 2010; Hammer et al., 2019a) is a crop model that integrates the intertwined interaction of G × E × M) to simulate plant development and growth on a daily basis (Wang et al., 2002; Keating et al., 2003; Holzworth et al., 2014). The model requires the following input data: daily weather records, soil profile characteristics, crop management, and cultivar-specific parameters. Crop phenology development is estimated as the summation of thermal time for nine phases from germination to physiological maturity. Daily biomass is estimated as the minimum of biomass limited by solar radiation or water availability. The biomass limited by solar radiation is the product of radiation use efficiency (RUE, MJ m-2), solar radiation (MJ m-2), and the fraction of light intercepted. The biomass limited by water availability is the product of transpiration efficiency and soil water supply. The model estimates water, temperature, and nitrogen deficit factors which affect phenology and growth. To estimate the effect of LT on carbon assimilation in hours with high VPD, APSIM-sorghum downscales daily temperature and solar radiation to hourly time steps and estimates relative humidity (RH) on each hour (Parton and Logan, 1981). Temperature and RH are used to calculate VPD on each hour (Murray, 1967; Monteith and Unsworth, 2013), then the model estimates biomass as a function of hourly water supply and demand. Finally, the biomass accumulation is aggregated for each daily timestep. Note, the version of APSIM-sorghum used and LT modifications were made for research purposes and are not in the release version.
Daily weather data at each site, including precipitation (mm), solar radiation (MJ m-2 day-1), maximum (°C), and minimum temperature (°C), were obtained from NASA Prediction of Worldwide Energy Resources (POWER- https://power.larc.nasa.gov/) from 1986 to 2018. The spatial resolution of the data are 1.0° latitude by 1.0° longitude for solar radiation and 0.5° latitude by 0.5° longitude for the remaining variables. Soil profile information such as soil texture (%), bulk density (g ml-1), organic carbon (%), and pH was downloaded from the web soil survey (https://websoilsurvey.sc.egov.usda.gov/App/HomePage.htm). These data were used to estimate the saturation capacity (SAT), field capacity (DUL), and wilting point (LL15) for each layer of the soil profile using the SBuild application of the Decision Support System for Agrotechnology Transfer (DSSAT) program (Hoogenboom et al., 2019). Crop management practices such as planting depth, plant population and planting dates were obtained from experiments or variety trials (Pachta, 2007; Larson et al., 2021; Schnell et al., 2021).
Model testing was conducted in two steps: model calibration and model evaluation (Wallach et al., 2014). In model calibration, specific parameters were iteratively adjusted to fit observations, while model evaluation estimated the accuracy of the model with independent data. For model testing we collected available information on field experiments for hybrid 87G57 from 1997 to 2007 (Figure 1D; Supplementary Table S1). Model calibration was conducted with a high quality experiment that accounts for information of crop management, phenology development, in-season biomass components, and initial soil water (Pachta, 2007). Information of this experiment including weather, soil and crop management was arranged into APSIM-Sorghum. First, a simulation was conducted for the hybrid 86G56 (no calibration), which was available in the library of the model. Next, cultivar parameters were modified, to eliminate the photoperiod sensitivity (photoperiod_slope from 10 to 0), and to match the grain yield components by modifying the parameter relation between biomass accumulated from floral initiation to the start of grain (dm_per_seed from 0.00087 to 0.00099). There was no need to adjust parameters related to phenology development since the model was accurate in predicting flowering time for this experiment (observed: 52, and simulated: 53).
Model evaluation was conducted with variety trial experiments conducted in Garden City, Colby, and Hays (Kansas). These experiments have information of planting date, plant density, flowering time, and grain yield. Environment (daily weather data, soil profile) and crop management practices for these simulations were arranged into APSIM-Sorghum. Each year the crop was simulated to be planted under optimal soil moisture (70% soil available water), and non-nitrogen limitations at plant density of 6–14 plants m-2. Grain yield was expressed assuming 12.5% of moisture content. Model accuracy was analyzed using the root mean square error (RMSE), which indicates the distance from a perfect prediction (Wallach et al., 2014).
Simulations comprised three factors: trait (GT): non-LT and LT trait; maturity group (GM): short, medium, and full-season; and planting dates (MP): early-May, mid-May, and early-June. The model defines a phenotype with an LT trait by assigning the parameter limited maximum transpiration to any value from 0.2 to 0.9 mm h-1 (Supplementary Table S2). Note a phenotype with an LT trait of 0.9 mm h-1 represents a genotype that restricts the transpiration by 10%; by contrast, a phenotype with non-LT had no restrictions. The LT trait was imposed as 0.9 mm h-1 for simulations across all locations. Parameters for hybrid 87G57 corresponded to a commercial short-season sorghum hybrid with 15 leaves. Parameters controlling growth and development, tt_endjuv_to_init, Tpla_prod_coef, and Tpla_inflection, were adjusted to simulate medium-season and late-season sorghum hybrids, each with 17 and 19 leaves, respectively. The number of tillers was kept constant (0.3) for all maturity groups. Simulations started every year on the first of January with initial soil moisture of 60%. In these simulations, the crop was automatically planted at three time intervals, early-May, mid-May, and early-June, a row distance of 76 cm, planting depth of two cm, and fertilized to fully meet plant nitrogen demand. Simulations were conducted every year from 1986 to 2018. We analyzed the grain yield, transpiration, biomass, soil moisture and water productivity for both sorghum phenotypes (non-LT and LT).
We conducted a sensitivity analysis in a representative location to identify initial conditions’ effect on grain yield changes resulting from the LT trait. Therefore, simulations started with initial soil moisture of 20%, 30%, 40%, 50%, 60%, 70%, 80%, and 90% while maintaining the LT trait at 0.9 mm h-1. Otherwise, to determine yield gains resulting from hypothetical genetic variability, we created simulations and varied the LT parameter from 0.2 to 0.9 mm h-1 while maintaining the initial soil moisture at 60%. As previously outlined, these simulations started each year on the first day of January under similar maturity groups and management practices. Absolute and relative change in harvested grain yield for the phenotype with LT trait was calculated for each simulation and averaged over environments.
The APSIM-Sorghum estimates the daily trajectory of soil water supply and plant water demand (waterSD), penalizing crop growth and development on a scale from 0 (stress) to 1 (no stress). The model indicates stress (close to zero) when the soil water supply falls behind plant water demand and no stress (close to 1) when the soil water supply meets the plant water demand (Wang et al., 2002). For the non-LT trait, the daily trajectories of waterSD were aggregated at the centesimal cumulative thermal time for each season. This time series information was analyzed via a hierarchical classification using the cluster (Maechler et al., 2023) library and the clara (Clustering Large Applications clara) method (Kaufman and Rousseeuw, 1990). The number of clusters was determined via the silhouette method (Kassambara, 2017), a measure of similarity for each data point relative to the assigned cluster and separation compared to other clusters. The final water stress environments (EW) resulted as the median of water stress on each cluster.
Statistical analyses of model outputs were performed in the R statistical environment utilizing mixed linear models and the lmer library (Bates et al., 2015) and lmerTest libraries. The analysis quantified the size of fixed effects on dependent variables (simulated grain yield, biomass, transpiration, soil moisture, and water productivity). Factors with fixed effects were trait (GT), maturity group (GM), planting date (MP), water stress environments (EW), and their interaction while factors with random effects were years (EY) nested on each location (EL). First, a backward-elimination stepwise regression (step function) that estimates the Akaike Information Criterion (AIC) was used to select the best linear model. The alternative hypothesis behind each model identified by the stepwise regression is that at least one of these fixed main and interaction effects is not equal to zero, while the null hypothesis is that all fixed effects and interactions are zero. Next, to test the robustness of the stepwise regression, a comparison between the new model and the four-way interaction model (GT × GM × MP × EW) was conducted using the likelihood ratio test (lrtest function). For simulated variables: grain yield, total transpiration, and soil water content, the stepwise regression indicated the model in Equation 1 as the best (α = 0.05), and the likelihood ratio test suggested this model was significantly different (α = 0.05) to the four-way interaction model. For simulated variables: biomass at flowering, transpiration at flowering, and water productivity, linear models resulting from the stepwise regression are detailed in Equations 2, 3, and 4, respectively. The likelihood ratio test coincided with Equation 2 as the best model for biomass (α = 0.05). Nevertheless, Equations 3 and 4 were non-significantly different to a four-way interaction model.
where is the response or dependent variable in the nth location in the mth year in the lth water stress environment in the kth planting date in the jth maturity group in the ith trait, is the grand mean, is the fixed effect of the ith trait, is the fixed effect of the jth maturity group, is the fixed effect of the kth planting date, is the fixed effect of the lth water stress environment, is the two-way interaction effect in the ith trait in the lth water stress environment, is the two-way interaction effect in the ith trait in the jth maturity group, is the two-way interaction effect in the jth maturity group in the kth planting date, is the two-way interaction effect in the lth water stress environment in the kth planting date, is the two-way interaction effect in the jth maturity group in the lth water stress environment, is the three-way interaction effect in the jth maturity group in the lth water stress environment in the kth planting date, is the three-way interaction effect in the ith trait in the lth water stress environment in the kth planting date, is the random effect of mth year nested within the nth location.
Tukey’s test was performed when the F value was below an α = 0.05 significance threshold.
To determine the model accuracy for flowering time and grain yield, we compared the observed data versus the information simulated by the model. For a growing season with hybrid 87G67 in Manhattan, Kansas (Figures 3A–D), the model reproduced the trajectory of dry weight for total biomass, stem, and panicle with an RMSE of 1.1, 0.4, and 0.7 Mg ha-1, respectively. However, a substantial underestimation occurred for dry leaf weight. In this experiment, the observed grain yield was 4.8 Mg ha-1, and the results after calibration were 5.4 Mg ha-1. For experiments in Kansas from 1997 to 2007 in Garden City, Hays, Colby, and Manhattan, the model showed satisfactory predictions for days to anthesis with an RMSE of 5 days (Figure 3E) and grain yield with an RMSE of 2 Mg ha-1 (Figure 3F). Despite the lack of experimental field data for model testing in Texas and Colorado, a comparison of statistical (2.2 to 6.6 Mg ha-1) versus simulated grain yield (1.5 to 6 Mg ha-1) resulted in a RMSE of 1.1 Mg ha-1 (Figure 3G).
Figure 3 Model testing for APSIM-Sorghum in the study system. (A) Observed versus simulated in-season dry biomass for hybrid 87G67 in Manhattan, Kansas (2007). (B) Observed versus simulated in-season stem dry weight for hybrid 87G67 in Manhattan, Kansas (2007). (C) Observed versus simulated in-season leaf dry weight for hybrid 87G67 in Manhattan, Kansas (2007). (D) Observed versus simulated in-season panicle dry weight for hybrid 87G67 in Manhattan, Kansas (2007). (E) Observed versus simulated days to anthesis for hybrid 87G67 under rainfed conditions for experiments in Garden City, Colby, Hays, and Manhattan (Kansas). (F) Observed versus simulated dry grain yield for hybrid 87G67 under rainfed conditions across the Kansas precipitation gradient (Garden City, Colby, Hays, and Manhattan). Each point (E, F) represents results for single seasons between 1997 to 2007. Information of (G) Observed versus simulated grain yield for Kansas, Colorado, and Texas study sites (indicated in Figure 4D). The y axis represents the mean of simulated yields over 33 years (1986-2018), three planting dates, and three maturity groups. The x axis represents the mean of observed data over 21 years (2010 to 2021). Observed sorghum grain yield (G) was obtained from the National Agricultural Statistical Service (NASS, https://www.nass.usda.gov/).
To determine the best GM and MP combination for grain yield in precipitation gradients, we conducted simulations for short-, medium- and full-season sorghums planted in early May (May 01), mid May (May 15) and early June (June 01). Note, around 3% of the simulations were removed for the analysis because they did not complete the vegetative stage (hereafter referred to as “failed seasons”) and the yield was close to zero. This occurred under extreme drought events (Rippey, 2015). For instance, in Colorado in 2012, the annual precipitation was less than 207 mm, and the rainfall during the simulated growing period was less than 130 mm. The number of failed seasons for full-season sorghum either planted early or late was higher in Colorado suggesting that short-season varieties outperform any maturity group under low rainfall, while the frequency of failed seasons in Texas was higher in early planting dates (Supplementary Figure S2).
Grain yield for simulated sorghum with a non-LT trait for different maturity groups and planting dates in Kansas, Texas, and Colorado, are indicated in Figure 4. An average across GM and MP indicated that grain yield varied from 1.7 to 6.5 Mg ha-1 (Figure 4A), with higher and lower yields in eastern and western regions, respectively. Interannual variability for grain yield ranged from 30 to 50% (Figure 4A). The model predicted higher yields in Colorado and Kansas when all maturity groups were planted in early May, followed by planting dates in mid-May and early June. In most Texas locations, the model predicted a higher yield for planting dates in early June (Figure 4B). The seasonal rainfall during each simulated season influenced the performance of different maturity groups for grain yield (Supplementary Figure S1A). On planting dates in June, discrepancies among maturity groups occurred under high precipitation; nevertheless, as the amount of rainfall during the growing season decreases, these differences become negligible (< 1%; Supplementary Figure S1B). By contrast, differences in maturity groups for grain yield across precipitation gradients in early May are insignificant (Supplementary Figure S1B).
Figure 4 Maturity (GM) × planting date (M) combinations to achieve maximum yield for non-LT versus LT sorghum. (A) Average grain yield and interannual variability. (B) Average grain under different planting dates and maturity groups. Each point represents the mean of 33 years (1986-2018), the annual variability (coefficient of variability) is the quotient of the standard deviation and mean. Best GM × M combination for a sorghum with (C) non-LT and (D) LT trait. (E) Relative increase in grain yield for a sorghum with LT trait.
Due to GM × MP × EW interactions, the effect of LT trait (GT) may depend on agronomic options, such as maturity group (GM) of the hybrid and planting date (MP). To identify the best combination (GM × MP) at each site (EL), we obtained the maximum yield for LT sorghum. The model indicated similar combinations for non-LT and LT sorghums (Figures 4C, D). In Colorado and Kansas, higher yields resulted when seasons for all maturity groups started on the first of May. In Colorado, short-season sorghum performed better in northern regions, while medium-season sorghum in southern regions. Full-season sorghum yielded higher across Kansas, except in Colby, where medium-season sorghum outperformed any other combination. In Texas, the model indicated full-season sorghum planted on the first of June as the best combination, with some exceptions in the northern regions (i.e. Hansford, Texas). Overall, sorghum with LT across all locations is expected to increase grain yields from 0 to 15% (Figure 4E). Note, the relative change is lower than 3% in regions with high precipitation and this change increases as declining precipitation amplifies water deficit scenarios in western regions of the study site.
To determine predominant water stress environments (EW) in the study system, we conducted a hierarchical cluster analysis for simulated time series on waterSD and thermal time. The classification indicated four water deficit environments: well-watered or light stress at grain filling (WW), late drought (LD), mid-season drought (MD), and early drought (ED) (Figure 5A). WW and LD predominated in eastern regions of Kansas and Texas, while MD and ED predominated in eastern Colorado and western Texas (Figure 5B). The analysis revealed a strong correlation between seasonal precipitation and water deficit patterns; although it was non-significant (r = 0.9, α = 0.06). On average, WW, LD, MD, and ED, showed seasonal precipitation of 400 mm, 300 mm, 244 mm, and 230 mm, respectively.
Figure 5 Simulated effects of LT (GT) across the US sorghum belt. (A) Water stress environment identified via water supply and demand (WaterSD) at each site. (B) Frequency of water stress environments in Kansas, Texas and Colorado. (C) Distribution of simulated grain yield, transpiration, water productivity, biomass and soil extractable water for a phenotype with a non-LT (dark gray) and LT trait (green) in water stress environments. Each distribution represents simulations for all maturity groups (GM), and planting dates (MP) in all sites over 33 years. Letters indicate significant differences (α< 0.05) of all pairwise comparisons using the Tukey HSD test. WW, Well-watered or light stress at grain filling; LD, late drought; MD, mid-season drought; and ED, early drought.
To determine the significance of fixed factors plus corresponding interaction on dependent variables (simulated variables), we used mixed models (Equations 1–4). We conducted this analysis for six simulated variables: grain yield, total transpiration, total water productivity, biomass at flowering time, transpiration at flowering time and soil water content at flowering time. All individual fixed effects (GT, GM, EW, and MP) had high significance (α = 2×10-16, Supplementary Table S3), and a post hoc analysis suggested that factors on each fixed effect belonged to different groups (Supplementary Table S3). For instance, sorghum’s LT trait increased grain yield by 5%. Full-season sorghum yielded 21% and 10% higher than early and medium-season sorghum. WW favored grain production; while lower yields correspond to ED. Likewise, earlier planting dates outperformed sorghum planted either in mid-May or early-June.
All dependent variables exhibited high significance (α = 2×10-16) in two-way interaction for three combinations: GT × EW, EW × MP, and GM × EW (Supplementary Table S3). The significant interaction for GT × EW, and the pairwise comparison for grain yield, water productivity, biomass at flowering, and soil moisture at flowering indicated that the LT trait outperformed the non-LT trait in all environments (Figure 5C). Note, grain yield for a LT sorghum in a WW environment was lower (4%) than in LD, MD, and ED environments (8%). However, the pairwise comparison for total transpiration and transpiration at flowering confirmed the significance for the interaction GT × Ew. For instance, the non-LT trait exhibited higher total transpiration in WW, while the LT trait improved it in MD and ED (Figure 5C). At flowering time, LT increased transpiration in WW, LD, and MD, but both traits exhibited similar transpiration in ED (Figure 5C). Only for biomass at flowering time the interaction GT × GM had high significance.
Transpiration at flowering exhibited a three-way interaction for GT × GM × EW (α = 0.002, Table S3). The pairwise comparison indicated a lack of differences between LT and non-LT genotypes for each maturity group in ED (Supplementary Figures S4A B, C). By contrast, the LT trait increased transpiration in WW, LD, and MD for each maturity group (Supplementary Figures S4A, B,C). Grain yield, transpiration, and soil water at flowering time and biomass at the flowering time exhibited the following three interactions as significant: GM × EW × MP (α = 0.04). Pairwise comparisons among these interactions for grain yield revealed differences for each maturity group and planting dates in environments WW and LD, but these differences become smaller in MD and ED (Supplementary Figures S4D, E, F). In these environments, for all maturity groups, grain yield for planting dates in early May and mid-May were similar, but these differed (α = 0.01) from the early June planting.
To test the effect of initial water content on the LT trait, we designed simulations and created eight scenarios of initial soil moisture (from 20% to 90%) while maintaining the LT parameter at 0.9 mm h-1. We conducted these simulations in Hays (Kansas), which presented the four environment classes identified in this study (Figures 2D, 5B). Nevertheless, regardless of the initial water content scenario, the model pointed out a yield increase for sorghum with LT, which is more pronounced under low soil moisture (i.e. 20% and 30%). Overall, model predictions indicated that initial soil moisture changes do not affect LT’s positive effect, although the uncertainty of these changes increased under low soil moisture (Figure 6A).
Figure 6 Sensitivity of a sorghum with LT trait to variation of initial conditions and genetic variability. (A) Relative change in grain yield under thresholds of initial soil moisture. The limited maximum transpiration was 0.9 mm h-1. (B) Relative change in grain yield under thresholds of limited maximum transpiration. Initial soil moisture was 60%. The analysis was conducted for a representative location (Hays, Kansas; 1986-2018) at the center of the sorghum belt which presented all four water stress environments. Vertical lines represent the standard deviation of each environment.
To test the hypothetical genetic variability of LT on grain yield, we created simulations for LT sorghum with a limited maximum transpiration rate from 0.2 mm h-1 to 0.9 mm h-1, while maintaining the initial soil moisture at 60%. We conducted these simulations in a central site of the sorghum belt in Kansas (Hays), representing the four water deficit patterns identified in this study. Model predictions indicated that grain yield increases in all environments when LT is lower than 0.9 mm h-1 (Figure 6B), with a greater benefit under drought scenarios. For instance, on average, an LT sorghum with a limited maximum transpiration rate of 0.2 mm h-1 increased the grain yield by 15%, 45%, 70%, and 74% in WW, LD, MD, and ED, respectively. By contrast, an LT sorghum with 0.8 mm h-1 increased the grain yield by 7%, 8%, 10%, and 11% in WW, LD, MD, and ED, respectively. Note that the uncertainty of predictions, represented by the standard deviation, became larger at LT lower than 0.9 mm h-1. In LD, the model predicted a yield increase between 25 to 79% for sorghum with an LT of 0.2 mm h-1. Otherwise, this increase ranged from 6 to 16% for sorghum with an LT of 0.8 mm h-1.
This study takes advantage of the nonlinearity of G×E×M captured by the APSIM-Sorghum to guide breeding programs in developing improved water-use hybrids in the sorghum belt of the United States. Specifically, this study assessed management practices to leverage the performance of sorghum hybrids (Figure 5B), envirotyped the target population of environments with distinctive water stress patterns (Figures 5A, B), and quantified the impact of the LT trait in the production sorghum area. Crop model applications to support breeding exist for different crops and diverse geographies (Technow et al., 2015; Chenu et al., 2017; Hammer et al., 2019b; Jighly et al., 2023). For instance, a study deployed a rice crop model to hypothesize the lack of effectiveness in breeding drought-tolerant upland rice in Brazil (Heinemann et al., 2019), and other studies improved phenomic prediction by integrating crop models and genomic prediction (Cooper et al., 2002; Heslot et al., 2014; Crossa et al., 2022).
The foundation of the model application is testing the prediction accuracy for the evaluated variable. This study showed that APSIM-Sorghum predictions of grain yield for short-season sorghum, obtained from variety trials, resulted in 2 Mg ha-1 (Figure 3F). A comparison of statistical data and simulated yield for all locations (Figure 3G) indicated a RMSE 1.1 Mg ha-1. These results agree with previous studies for sorghum, which show a RMSE of 1 to 2 Mg ha-1 (Carcedo et al., 2022; Ojeda et al., 2022). APSIM-sorghum underestimates the -in-season dynamic of leaf tissue study (Figure 3C), a similar phenomenon is observed in a sorghum study that used the APSIM-sorghum model in Ethiopia (Tirfessa et al., 2023). While we acknowledge this discrepancy, it does not influence the impact of the LT trait. The APSIM-sorghum simulates transpiration on a leaf area basis, the simulated leaf area is identical for non-LT and LT phenotypes (Supplementary Table S2); hence, the relative change resulting from the LT trait is similar to our findings regardless of the model accuracy for leaf tissue. Researchers have applied differing approaches to APSIM-sorghum validation with some studies primarily comparing flowering time, biomass, and grain yield (Carcedo et al., 2022; Della Nave et al., 2022; Ojeda et al., 2022), while others disregard testing the accuracy of the model (Kholová et al., 2014; Ronanki et al., 2022). Overall, the model reliability for grain yield predictions enabled deployment to test the potential impact of the LT trait.
The decision to include a trait within a breeding program clearly depends on the impact of this trait on final grain yield. Breeding programs require that a candidate trait can contribute at least a 5% yield increase to be included in a breeding portfolio. Findings of this study revealed the LT trait can potentially increase grain yield from 3% to 13% in the sorghum belt in the United States (Figure 4E). Therefore, LT is a candidate trait for developing hybrids with improved water-resiliency for western regions of the sorghum belt (Figure 5B).
Although our simulation does not present a full geospatial analysis (Messina et al., 2015), our study shows results for contrasting sites across gradients of VPD and precipitation. Site-specific simulations allowed for handling detailed information on additional variables (Collins et al., 2021) in any growing period, such as soil moisture, transpiration, and biomass (Figure 5C). Otherwise, grid geospatial simulation studies rarely provide information other than yield (Messina et al., 2015; Guiguitant et al., 2017). Despite our study disregarded spatial variability on initial soil moisture, the model reproduced the observed yield (RMSE 1.1 Mg ha-1, Figure 3F). Likewise, a sensitivity analysis revealed that the initial water conditions do not affect the positive impact on LT (Figure 6A).
Current climate variability (33 years) highlights the crop vulnerability (Figure 4A) in western regions characterized by the high frequency of water deficit scenarios (Figure 5B) and where the impact of LT sorghum is more significant (Figure 4E and Figure 5). Climate change scenarios, disregarded in our simulations, project a VPD increase by the end of the century (Yuan et al., 2019). Under high VPD, LT hypothetically leads to stomatal closure (Sinclair et al., 2005); similarly, rising levels of CO2 cause stomatal closure in C3 and C4 crops (Allen et al., 2011). However, it is unknown whether the impact of CO2 and LT would have a synergistic effect or if the stomatal response to CO2 would prevail over LT. Simulations under future scenarios would be needed to elucidate these effects. Nevertheless, a simulation study hypothesized that CO2 and LT can compensate for detrimental impacts of climate change in the wheat belt of Australia (Collins et al., 2021).
The LT trait is expected to restrict water transpiration when soil moisture is at field capacity and high VPD (Sinclair et al., 2005). Therefore, this trait is undesirable for WW conditions because depriving transpiration would penalize carbon fixation and final grain yield (Vadez et al., 2014). Unexpectedly, in our study, simulation studies indicated that an LT sorghum can contribute to an increase in grain yield of 4% for WW environments (Supplementary Table S3, Figure 5C). Under WW environments, predictions for wheat with the APSIM model indicated a yield increase of 0.2% (Collins et al., 2021), while predictions for maize with a simple model indicated a yield decline of -3% in the USA (Messina et al., 2015). Yield gains for WW environments in our study can be due to differences in the model structure. In LD environments, sorghum grain yield increased by around 9% (Figure 5C, Supplementary Table S3), which is slightly higher than predictions for wheat (2 to 7%, Collins et al., 2021) and within the range of 0 to 24% reported for maize (Messina et al., 2015). Our results for MD (10%) and ED (9%) align with the reported yield increase for wheat which ranged between 3 to 13% (Collins et al., 2021). From a breeding perspective, LT sorghum would have a more significant impact on water stress scenarios of the sorghum belt. It is essential to identify the best combination of GT × GM × MP in sorghum since it is planted late and across precipitation gradients (Roozeboom and Fjell, 1998; Shroyer et al., 1998; Ciampitti et al., 2019). Overall, LT sorghum increased grain yield across planting dates and maturity groups by 8%. Although specific combinations of GM × MP (Supplementary Table S3) can maximize crop yield either for a non-LT (Figure 4C) or LT sorghum (Figure 4D).
Variety trials or multi-environment experiments have shown that, unlike full-season varieties, medium- and short-season varieties can complete their growing cycle in regions with low precipitation (Larson et al., 2021; Schnell et al., 2021) and limited growing degree days (GDD) at higher latitudes (Kukal and Irmak, 2018). This strategy has led to the selection of maturity groups that match precipitation and GDD gradients in the sorghum belt (Figures 4C and 4D). Since the impact of LT sorghum is more relevant in western regions (Figure 4E), this study suggests introgressing this trait in medium- and short-season hybrids rather than in full-season hybrids (Figures 4D, 5B). Management practices need to be considered to enhance the performance of LT sorghum. Shifting planting dates can change the frequency of water deficit environments (Chenu et al., 2011; Raymundo et al., 2021) (Supplementary Figure S3) by increasing grain yield in early planting dates, especially in Kansas (Figure 4B). Higher yields in early spring resulted from the synchronization of planting dates with the onset of precipitation, which increased the frequency of WW environments (Supplementary Figure S3). Likewise, simulation and field studies demonstrated yield gains of up to 11% in seasons with higher water availability (Francis et al., 1986; Carcedo et al., 2021; Zander et al., 2021)
Genetic pyramiding for drought adapted phenotypes can be explored via crop modeling (Cooper et al., 2002). A simulation study in sorghum revealed that LT is more effective than stay-green in water scarcity scenarios (Kholová et al., 2014). Higher yields in early spring suggests (Figure 4B and Supplementary Figures S4D–F) a plausible interaction between early chilling tolerance and LT trait. LT increases canopy temperature (Belko et al., 2013), and temperatures beyond 38°C can penalize carbon assimilation and plant growth (Singh et al., 2015). Via experimentation and simulations, crop models provide understanding of the effect of interplay between multiple traits (genes) on harvestable yield. Note phenotypes commonly used in crop modeling differ from phenotypes traditionally evaluated by breeders (Ramirez-Villegas et al., 2020). This mismatch makes large-scale phenotyping deployed by breeding programs unsuitable for crop model purposes (Muller and Martre, 2019). Hence, our study shows how crop models can inform high-throughput phenotyping platforms regarding potential traits contributing to the plant water economy (Boote et al., 2021). Then, integrating field experimentation and crop modeling for ideotyping LT with additional adaptation traits can support breeding programs when developing a trait technology for water-limited scenarios (Chenu et al., 2017).
In this simulation study, the LT trait optimizes water use by reducing transpiration around flowering time, increasing water productivity and grain yield (Figure 5C). Therefore, this trait can benefit farmers’ economies in the sorghum belt. Nevertheless, LT is a hypothetical trait, implemented in crop models (Bates et al., 2015; Messina et al., 2015; Sinclair et al., 2017; Truong et al., 2017), and whose genetic variation is reported and limited to controlled environments (Gholipoor et al., 2010; Vadez et al., 2015; Devi and Reddy, 2018; Medina et al., 2019; Collins et al., 2021). Although variation for LT was reported in controlled environments, the repeatability of this trait has yet to be shown in sorghum-producing regions. Hence, including the LT trait in a sorghum breeding program requires validating this trait under field conditions and testing the effectiveness of phenomic approaches to discriminate this trait in large populations. Potential donors would make feasible developing bi-parental populations to determine quantitative trait loci (QTLs) controlling the phenotypic expression of this trait. Further isolating these QTL can assist in dissecting the underlying physiological and molecular mechanisms of limited transpiration, which remain enigmatic.
The original contributions presented in the study are included in the article/Supplementary Material. Further inquiries can be directed to the corresponding authors.
RR: Conceptualization, Data curation, Formal analysis, Investigation, Methodology, Validation, Visualization, Writing – original draft, Writing – review & editing. GMc: Methodology, Software, Writing – review & editing. SS-B: Funding acquisition, Resources, Writing – review & editing. AL: Writing – review & editing, Formal analysis. GMo: Conceptualization, Funding acquisition, Investigation, Methodology, Resources, Supervision, Writing – review & editing.
The author(s) declare financial support was received for the research, authorship, and/or publication of this article. This study was supported by funding from the Foundation for Food and Agriculture Research - Seeding Solution “CA18-SS-0000000094 – Bridging the Genome-to-Phenome Breeding Gap for Water-Efficient Crop Yields (G2P Bridge)” to GPM and SS-B; the Kansas Department of Agriculture “Collaborative Sorghum Investment Program Water Optimized Sorghum for Kansas” to GPM; and the Kansas Grain Sorghum Commission.
The authors declare that the research was conducted in the absence of any commercial or financial relationships that could be construed as a potential conflict of interest.
The reviewer VJ declared a shared affiliation with the author GM to the handling editor at the time of review.
All claims expressed in this article are solely those of the authors and do not necessarily represent those of their affiliated organizations, or those of the publisher, the editors and the reviewers. Any product that may be evaluated in this article, or claim that may be made by its manufacturer, is not guaranteed or endorsed by the publisher.
The Supplementary Material for this article can be found online at: https://www.frontiersin.org/articles/10.3389/fpls.2023.1283339/full#supplementary-material
Abdel-Ghany, S. E., Ullah, F., Ben-Hur, A., Reddy, A. S. N. (2020). Transcriptome analysis of drought-resistant and drought-sensitive sorghum (Sorghum bicolor) genotypes in response to PEG-induced drought stress. Int. J. Mol. Sci. 21, 772. doi: 10.3390/ijms21030772
Abreha, K. B., Enyew, M., Carlsson, A. S., Vetukuri, R. R., Feyissa, T., Motlhaodi, T., et al. (2021). Sorghum in dryland: morphological, physiological, and molecular responses of sorghum under drought stress. Planta 255, 20. doi: 10.1007/s00425-021-03799-7
Allen, L. H., Kakani, V. G., Vu, J. C. V., Boote, K. J. (2011). Elevated CO2 increases water use efficiency by sustaining photosynthesis of water-limited maize and sorghum. J. Plant Physiol. 168, 1909–1918. doi: 10.1016/j.jplph.2011.05.005
Araus, J. L., Serret, M. D., Edmeades, G. O. (2012). Phenotyping maize for adaptation to drought. Front. Physiol. 3. doi: 10.3389/fphys.2012.00305
Barbier, N. (2015). Vulnerability to water shortages in the 21st Century’s arid and semi-arid American West (L’Ordinaire des Amériques). doi: 10.4000/orda.1956
Bates, D., Maechler, M., Bolker, B. M., Walker, S. C. (2015). Fitting linear mixed-effects models using lme4. J. Stat. Software 67, 1–48. doi: 10.18637/jss.v067.i01
Belko, N., Zaman-Allah, M., Diop, N. N., Cisse, N., Zombre, G., Ehlers, J. D., et al. (2013). Restriction of transpiration rate under high vapour pressure deficit and non-limiting water conditions is important for terminal drought tolerance in cowpea. Plant Biol. (Stuttg) 15, 304–316. doi: 10.1111/j.1438-8677.2012.00642.x
Bernardo, R. (2020). Reinventing quantitative genetics for plant breeding: something old, something new, something borrowed, something BLUE. Heredity 125, 375–385. doi: 10.1038/s41437-020-0312-1
Boote, K. J., Jones, J. W., Hoogenboom, G. (2021). Incorporating realistic trait physiology into crop growth models to support genetic improvement. silico Plants 3, diab002. doi: 10.1093/insilicoplants/diab002
Carcedo, A. J. P., Cejas, E., Gambin, B. L. (2021). Adapting sorghum sowing date and genotype maturity to seasonal rainfall variation in a temperate region. silico Plants 3, diab007. doi: 10.1093/insilicoplants/diab007
Carcedo, A. J. P., Mayor, L., Demarco, P., Morris, G. P., Lingenfelser, J., Messina, C. D., et al. (2022). Environment characterization in sorghum (Sorghum bicolor L.) by modeling water-deficit and heat patterns in the great plains region, United States. Front. Plant Sci. 13. doi: 10.3389/fpls.2022.768610
Challinor, A. J., Müller, C., Asseng, S., Deva, C., Nicklin, K. J., Wallach, D., et al. (2018). Improving the use of crop models for risk assessment and climate change adaptation. Agric. Syst. 159, 296–306. doi: 10.1016/j.agsy.2017.07.010
Chenu, K., Cooper, M., Hammer, G. L., Mathews, K. L., Dreccer, M. F., Chapman, S. C. (2011). Environment characterization as an aid to wheat improvement: interpreting genotype-environment interactions by modelling water-deficit patterns in North-Eastern Australia. J. Exp. Bot. 62, 1743–1755. doi: 10.1093/jxb/erq459
Chenu, K., Porter, J. R., Martre, P., Basso, B., Chapman, S. C., Ewert, F., et al. (2017). Contribution of crop models to adaptation in wheat. Trends Plant Sci. 22, 472–490. doi: 10.1016/j.tplants.2017.02.003
Ciampitti, I. A., Prasad, P. V. V., Schlegel, A. J., Haag, L., Schnell, R. W., Arnall, B., et al. (2019). Genotype × Environment × Management interactions: US sorghum cropping systems, in: sorghum. John Wiley Sons Ltd pp, 277–296. doi: 10.2134/agronmonogr58.c13
Collins, B., Chapman, S., Hammer, G., Chenu, K. (2021). Limiting transpiration rate in high evaporative demand conditions to improve Australian wheat productivity. silico Plants 3, diab006. doi: 10.1093/insilicoplants/diab006
Cooper, M., Chapman, S. C., Podlich, D. W., Hammer, G. L. (2002). The GP problem: quantifying gene-to-phenotype relationships. In Silico Biol. 2, 151–164.
Crossa, J., Montesinos-López, O. A., Pérez-Rodríguez, P., Costa-Neto, G., Fritsche-Neto, R., Ortiz, R., et al. (2022). “Genome and Environment Based Prediction ModelsPrediction models and Methods of Complex TraitsComplex traits Incorporating Genotype × Environment Interaction,” in Genomic prediction of complex traits: methods and protocols, methods in molecular biology. Eds. Ahmadi, N., Bartholomé, J. (New York, NY: Springer US), 245–283. doi: 10.1007/978-1-0716-2205-6_9
Della Nave, F. N., Ojeda, J. J., Irisarri, J. G. N., Pembleton, K., Oyarzabal, M., Oesterheld, M. (2022). Calibrating APSIM for forage sorghum using remote sensing and field data under sub-optimal growth conditions. Agric. Syst. 201, 103459. doi: 10.1016/j.agsy.2022.103459
Devi, M. J., Reddy, V. R. (2018). Transpiration response of cotton to vapor pressure deficit and its relationship with stomatal traits. Front. Plant Sci. 9. doi: 10.3389/fpls.2018.01572
Doggett, H. J., Majisu, B. N. (1968). Disruptive selection in crop development. Heredity 23, 1–23. doi: 10.1038/hdy.1968.1
Francis, C. A., Moomaw, R. S., Rajewski, J. F., Saeed, M. (1986). Grain sorghum hybrid interactions with tillage system and planting dates1. Crop Sci. 26, cropsci1986.0011183X002600010046x. doi: 10.2135/cropsci1986.0011183X002600010046x
Gholipoor, M., Prasad, P. V. V., Mutava, R. N., Sinclair, T. R. (2010). Genetic variability of transpiration response to vapor pressure deficit among sorghum genotypes. Field Crops Res. 119, 85–90. doi: 10.1016/j.fcr.2010.06.018
Guiguitant, J., Marrou, H., Vadez, V., Gupta, P., Kumar, S., Soltani, A., et al. (2017). Relevance of limited-transpiration trait for lentil (Lens culinaris Medik.) in South Asia. Field Crops Res. 209, 96–107. doi: 10.1016/j.fcr.2017.04.013
Hammer, G., McLean, G., Doherty, A., van Oosterom, E., Chapman, S. (2019a). Sorghum crop modeling and its utility in agronomy and breeding, in: sorghum. John Wiley Sons Ltd pp, 215–239. doi: 10.2134/agronmonogr58.c10
Hammer, G., Messina, C., Wu, A., Cooper, M. (2019b). Biological reality and parsimony in crop models—why we need both in crop improvement! silico Plants 1, diz010. doi: 10.1093/insilicoplants/diz010
Hammer, G. L., van Oosterom, E., McLean, G., Chapman, S. C., Broad, I., Harland, P., et al. (2010). Adapting APSIM to model the physiology and genetics of complex adaptive traits in field crops. J. Exp. Bot. 61, 2185–2202. doi: 10.1093/jxb/erq095
Heinemann, A. B., Ramirez-Villegas, J., Rebolledo, M. C., Costa Neto, G. M. F., Castro, A. P. (2019). Upland rice breeding led to increased drought sensitivity in Brazil. Field Crops Res. 231, 57–67. doi: 10.1016/j.fcr.2018.11.009
Heslot, N., Akdemir, D., Sorrells, M. E., Jannink, J.-L. (2014). Integrating environmental covariates and crop modeling into the genomic selection framework to predict genotype by environment interactions. Theor. Appl. Genet. 127, 463–480. doi: 10.1007/s00122-013-2231-5
Holzworth, D. P., Huth, N. I., deVoil, P. G., Zurcher, E. J., Herrmann, N. I., McLean, G., et al. (2014). APSIM – Evolution towards a new generation of agricultural systems simulation. Environ. Model. Software 62, 327–350. doi: 10.1016/j.envsoft.2014.07.009
Hoogenboom, G., Porter, C. H., Boote, K. J., Shelia, V., Wilkens, P. W., Singh, U., et al. (2019). “The DSSAT crop modeling ecosystem,” in Advances in crop modeling for sustainable agriculture (Cambridge, United Kingdom: Burleigh Dodds Science Publishing), 173–216.
Jighly, A., Thayalakumaran, T., O’Leary, G. J., Kant, S., Panozzo, J., Aggarwal, R., et al. (2023). Using genomic prediction with crop growth models enables the prediction of associated traits in wheat. J. Exp. Bot. 74, 1389–1402. doi: 10.1093/jxb/erac393
Jones, J. W., Hoogenboom, G., Porter, C. H., Boote, K. J., Batchelor, W. D., Hunt, L. A., et al. (2003). The DSSAT cropping system model. Eur. J. Agronomy Model. Cropping Systems: Science Software Appl. 18, 235–265. doi: 10.1016/S1161-0301(02)00107-7
Kassambara, A. (2017). Practical guide to cluster analysis in R: unsupervised machine learning (STHDA).
Kaufman, L., Rousseeuw, P. (1990). “Clustering large applications (Program CLARA),” in Finding groups in data (John Wiley & Sons, Ltd), 126–163. doi: 10.1002/9780470316801.ch3
Keating, B. A., Carberry, P. S., Hammer, G. L., Probert, M. E., Robertson, M. J., Holzworth, D., et al. (2003). An overview of APSIM, a model designed for farming systems simulation. Eur. J. Agronomy Model. Cropping Systems: Science Software Appl. 18, 267–288. doi: 10.1016/S1161-0301(02)00108-9
Kholová, J., Murugesan, T., Kaliamoorthy, S., Malayee, S., Baddam, R., Hammer, G. L., et al. (2014). Modelling the effect of plant water use traits on yield and stay-green expression in sorghum. Funct. Plant Biol. 41, 1019–1034. doi: 10.1071/FP13355
Kukal, M. S., Irmak, S. (2018). U.S. Agro-climate in 20th century: growing degree days, first and last frost, growing season length, and impacts on crop yields. Sci. Rep. 8, 6977. doi: 10.1038/s41598-018-25212-2
Laingen, C. (2015). A spatiotemporal analysis of sorghum in the United States. Papers Appl. Geogr. 1, 307–311. doi: 10.1080/23754931.2015.1084359
Larson, K. J., Jones-Diamond, S. M., Johnson, J. J., Bartolo, M. E., Tanabe, K. J., Pettinger, B. T. (2021). . 2021 sorghum hybrid performance in eastern colorado (No. TR22-1) (Colorado State University).
Maechler, M., Rousseeuw, P., Struyf, A., Hubert, M., Hornik, K. (2023). cluster: Cluster Analysis Basics and Extensions. R package version 2.1.6 — For new features, see the 'NEWS' and the 'Changelog' file in the package source. Available at: https://CRAN.R-project.org/package=cluster.
Medina, S., Vicente, R., Nieto-Taladriz, M. T., Aparicio, N., Chairi, F., Vergara-Diaz, O., et al. (2019). The plant-transpiration response to vapor pressure deficit (VPD) in durum wheat is associated with differential yield performance and specific expression of genes involved in primary metabolism and water transport. Front. Plant Sci. 9. doi: 10.3389/fpls.2018.01994
Messina, C. D., Podlich, D., Dong, Z., Samples, M., Cooper, M. (2011). Yield–trait performance landscapes: from theory to application in breeding maize for drought tolerance. J. Exp. Bot. 62, 855–868. doi: 10.1093/jxb/erq329
Messina, C. D., Sinclair, T. R., Hammer, G. L., Curan, D., Thompson, J., Oler, Z., et al. (2015). Limited-transpiration trait may increase maize drought tolerance in the US corn belt. Agron. J. 107, 1978–1986. doi: 10.2134/agronj15.0016
Monneveux, P., Jing, R., Misra, S. (2012). Phenotyping for drought adaptation in wheat using physiological traits. Front. Physiol. 3. doi: 10.3389/fphys.2012.00429
Monteith, J. L., Unsworth, M. H. (2013). “Chapter 2 - properties of gases and liquids,” in Principles of environmental physics (Fourth edition). Eds. Monteith, J. L., Unsworth, M. H. (Boston: Academic Press), 5–23. doi: 10.1016/B978-0-12-386910-4.00002-0
Muller, B., Martre, P. (2019). Plant and crop simulation models: powerful tools to link physiology, genetics, and phenomics. J. Exp. Bot. 70, 2339–2344. doi: 10.1093/jxb/erz175
Murray, F. W. (1967). On the computation of saturation vapor pressure. J. Appl. Meteorology Climatology 6, 203–204. doi: 10.1175/1520-0450(1967)006<0203:OTCOSV>2.0.CO;2
NOAA. (2020). The high cost of drought (Drought.gov). Available at: https://www.drought.gov/news/high-cost-drought (Accessed 12.7.22).
Ojeda, J. J., Hammer, G., Yang, K.-W., Tuinstra, M. R., deVoil, P., McLean, G., et al. (2022). Quantifying the effects of varietal types × management on the spatial variability of sorghum biomass across US environments. GCB Bioenergy 14, 411–433. doi: 10.1111/gcbb.12919
Pachta, C. J. (2007). Improving irrigated cropping systems on the high plains using crop simulation models (Thesis) (Kansas State University).
Parton, W. J., Logan, J. A. (1981). A model for diurnal variation in soil and air temperature. Agric. Meteorology 23, 205–216. doi: 10.1016/0002-1571(81)90105-9
Pournasiri-Poshtiri, M., Towler, E., Pal, I. (2018). Characterizing and understanding the variability of streamflow drought indicators within the USA. Hydrological Sci. J. 63, 1791–1803. doi: 10.1080/02626667.2018.1534240
Ramirez-Villegas, J., Molero Milan, A., Alexandrov, N., Asseng, S., Challinor, A. J., Crossa, J., et al. (2020). CGIAR modeling approaches for resource-constrained scenarios: I. Accelerating crop breeding for a changing climate. Crop Sci. 60, 547–567. doi: 10.1002/csc2.20048
Raymundo, R., Sexton-Bowser, S., Ciampitti, I. A., Morris, G. P. (2021). Crop modeling defines opportunities and challenges for drought escape, water capture, and yield increase using chilling-tolerant sorghum. Plant Direct 5, e349. doi: 10.1002/pld3.349
Rippey, B. R. (2015). The U.S. drought of 2012. Weather and climate extremes, USDA research and programs on extreme events, Vol. 10. 57–64. doi: 10.1016/j.wace.2015.10.004
Ronanki, S., Pavlík, J., Masner, J., Jarolímek, J., Stočes, M., Subhash, D., et al. (2022). An APSIM-powered framework for post-rainy sorghum-system design in India. Field Crops Res. 277, 108422. doi: 10.1016/j.fcr.2021.108422
Roozeboom, K., Fjell, D. (1998). “Selection of grain sorghum hybrids,” in Grain sorghum production handbook (Kansas State University).
Schnell, R. W., Horn, K., Biar, E., Rooney, W. L. (2021). 2021 texas grain sorghum performance variety trials (No. SCS-2021-13). The texas A&M university systems, college station, texas.
Sinclair, T. R., Devi, J., Shekoofa, A., Choudhary, S., Sadok, W., Vadez, V., et al. (2017). Limited-transpiration response to high vapor pressure deficit in crop species. Plant Sci. 260, 109–118. doi: 10.1016/j.plantsci.2017.04.007
Sinclair, T. R., Hammer, G. L., Oosterom, E. J. (2005). Potential yield and water-use efficiency benefits in sorghum from limited maximum transpiration rate. Funct. Plant Biol. 32, 945–952. doi: 10.1071/FP05047
Singh, P., Nedumaran, S., Traore, P. C. S., Boote, K. J., Rattunde, H. F. W., Prasad, P. V. V., et al (2014). Quantifying potential benefits of drought and heat tolerance in rainy season sorghum for adapting to climate change. Agricultural and Forest Meteorology 185, 37–48. doi: 10.1016/j.agrformet.2013.10.012
Singh, V., Nguyen, C. T., van Oosterom, E. J., Chapman, S. C., Jordan, D. R., Hammer, G. L. (2015). Sorghum genotypes differ in high temperature responses for seed set. Field Crops Res. 171, 32–40. doi: 10.1016/j.fcr.2014.11.003
Smith, C. W., Frederiksen, R. A. (2000). Sorghum: origin, history, technology, and production (John Wiley & Sons).
Tang, C., Piechota, T. C. (2009). Spatial and temporal soil moisture and drought variability in the Upper Colorado River Basin. J. Hydrology 379, 122–135. doi: 10.1016/j.jhydrol.2009.09.052
Technow, F., Messina, C. D., Totir, L. R., Cooper, M. (2015). Integrating crop growth models with whole genome prediction through approximate bayesian computation. PloS One 10, e0130855. doi: 10.1371/journal.pone.0130855
Thornton, P., Dinesh, D., Cramer, L., Loboguerrero, A. M., Campbell, B. (2018). Agriculture in a changing climate: Keeping our cool in the face of the hothouse. Outlook Agric. 47, 283–290. doi: 10.1177/0030727018815332
Tirfessa, A., Getachew, F., McLean, G., van Oosterom, E., Jordan, D., Hammer, G. (2023). Modeling adaptation of sorghum in Ethiopia with APSIM—opportunities with G×E×M. Agron. Sustain Dev. 43, 15. doi: 10.1007/s13593-023-00869-w
Truong, S. K., McCormick, R. F., Mullet, J. E. (2017). Bioenergy sorghum crop model predicts VPD-limited transpiration traits enhance biomass yield in water-limited environments. Front. Plant Sci. 8. doi: 10.3389/fpls.2017.00335
Vadez, V., Kholová, J., Hummel, G., Zhokhavets, U., Gupta, S. K., Hash, C. T. (2015). LeasyScan: a novel concept combining 3D imaging and lysimetry for high-throughput phenotyping of traits controlling plant water budget. J. Exp. Bot. 66, 5581–5593. doi: 10.1093/jxb/erv251
Vadez, V., Kholova, J., Medina, S., Kakkera, A., Anderberg, H. (2014). Transpiration efficiency: new insights into an old story. J. Exp. Bot. 65, 6141–6153. doi: 10.1093/jxb/eru040
van Ittersum, M. K., Leffelaar, P. A., van Keulen, H., Kropff, M. J., Bastiaans, L., Goudriaan, J. (2003). On approaches and applications of the Wageningen crop models. Eur. J. Agronomy Model. Cropping Systems: Science Software Appl. 18, 201–234. doi: 10.1016/S1161-0301(02)00106-5
Wallach, D., Makowski, D., Jones, J. W., Brun, F. (2014). “Chapter 9 - model evaluation,” in Working with dynamic crop models, 2nd ed. Eds. Wallach, D., Makowski, D., Jones, J. W., Brun, F. (San Diego: Academic Press), 345–406. doi: 10.1016/B978-0-12-397008-4.00009-5
Wang, E., Robertson, M. J., Hammer, G. L., Carberry, P. S., Holzworth, D., Meinke, H., et al. (2002). Development of a generic crop model template in the cropping system model APSIM. Eur. J. Agronomy Process Simulation Appl. Cropping System Models 18, 121–140. doi: 10.1016/S1161-0301(02)00100-4
Yuan, W., Zheng, Y., Piao, S., Ciais, P., Lombardozzi, D., Wang, Y., et al. (2019). Increased atmospheric vapor pressure deficit reduces global vegetation growth. Sci. Adv. 5, eaax1396. doi: 10.1126/sciadv.aax1396
Keywords: stress tolerance, crop growth model, crop adaptation, plant breeding, limited transpiration, sorghum
Citation: Raymundo R, Mclean G, Sexton-Bowser S, Lipka AE and Morris GP (2024) Crop modeling suggests limited transpiration would increase yield of sorghum across drought-prone regions of the United States. Front. Plant Sci. 14:1283339. doi: 10.3389/fpls.2023.1283339
Received: 25 August 2023; Accepted: 21 December 2023;
Published: 29 January 2024.
Edited by:
Veronique Letort, Paris-Saclay University, FranceReviewed by:
Germano Costa-Neto, Cornell University, United StatesCopyright © 2024 Raymundo, Mclean, Sexton-Bowser, Lipka and Morris. This is an open-access article distributed under the terms of the Creative Commons Attribution License (CC BY). The use, distribution or reproduction in other forums is permitted, provided the original author(s) and the copyright owner(s) are credited and that the original publication in this journal is cited, in accordance with accepted academic practice. No use, distribution or reproduction is permitted which does not comply with these terms.
*Correspondence: Rubí Raymundo, ci5yYXltdW5kb19jYXJodWFwb21hQGNvbG9zdGF0ZS5lZHU=; Geoffrey P. Morris, R2VvZmYuTW9ycmlzQGNvbG9zdGF0ZS5lZHU=
Disclaimer: All claims expressed in this article are solely those of the authors and do not necessarily represent those of their affiliated organizations, or those of the publisher, the editors and the reviewers. Any product that may be evaluated in this article or claim that may be made by its manufacturer is not guaranteed or endorsed by the publisher.
Research integrity at Frontiers
Learn more about the work of our research integrity team to safeguard the quality of each article we publish.