- 1Department of Agroecology and Crop Production, Faculty of Agrobiology, Food and Natural Resources, Czech University of Life Sciences Prague, Suchdol, Czechia
- 2Department of Crop Sciences and Agroforestry, Faculty of Tropical AgriSciences, Czech University of Life Sciences Prague, Suchdol, Czechia
Omics techniques, including genomics, transcriptomics, proteomics, and metabolomics have smoothed the researcher’s ability to generate hypotheses and discover various agronomically relevant functions and mechanisms, as well as their implications and associations. With a significant increase in the number of cases with resistance to multiple herbicide modes of action, studies on herbicide resistance are currently one of the predominant areas of research within the field of weed science. High-throughput technologies have already started revolutionizing the current molecular weed biology studies. The evolution of herbicide resistance in weeds (particularly via non-target site resistance mechanism) is a perfect example of a complex, multi-pathway integration-induced response. To date, functional genomics, including transcriptomic and metabolomic studies have been used separately in herbicide resistance research, however there is a substantial lack of integrated approach. Hence, despite the ability of omics technologies to provide significant insights into the molecular functioning of weeds, using a single omics can sometimes be misleading. This mini-review will aim to discuss the current progress of transcriptome-based and metabolome-based approaches in herbicide resistance research, along with their systematic integration.
1 Introduction
Herbicide resistance among weeds has become a major reason for food security problems since the early years of synthetic herbicide development. Since then, there has been a continuous increase in the frequency of herbicide resistance cases, a major plant protection challenge encountered by farmers across the planet. Herbicides have been used to control weeds and increase the quality and quantity of major crops since their discovery. However, repeated use of similar herbicidal modes of action (MOAs) and the associated selection pressure have led to the evolution of herbicide resistance in varieties of economically important weedy species such as Alopecurus myosuroides (Lan et al., 2022; Goldberg-Cavalleri et al., 2023), Amaranthus palmeri (Manicardi et al., 2023), Apera spica venti (Košnarová et al., 2021; Papapanagiotou et al., 2022), Bromus sterilis (Sen et al., 2021), Lolium spp (Tehranchian et al., 2019; Ma et al., 2020; Zhu et al., 2023). Recent studies showed that the majority of these weeds were also developing multiple resistances against herbicides with more than one mode of action (Tehranchian et al., 2019; Ma et al., 2020; Košnarová et al., 2021; Lan et al., 2022; Papapanagiotou et al., 2022; Zhu et al., 2023). From a molecular point of view, herbicide resistance mechanisms might be categorized into target-site based resistance (TSR) and non-target-site based resistance (NTSR) (Torra and Alcántara-de la Cruz, 2022). Briefly, TSR comprises single or multiple nucleotide polymorphisms (point mutations), codon deletion (till date there is only one evidence in the case of protoporphyrinogen oxidase in Amaranthus sp.) and target gene overexpression (either via transcriptional regulatory mechanisms or via gene amplification) (Gaines et al., 2020). On the other hand, NTSR constitutes enhanced metabolism [via cytochrome P450s (Cyp450s), glutathione S-transferases (GSTs) and glycosyltransferases (GTs)], reduced uptake and translocation, vacuolar sequestration and increased ability to deal with oxygen radicals (Gaines et al., 2020). More details on these mechanisms can be found in Gaines et al., 2020. Reports show that the NTSR is more complicated and trickier to understand than the TSR (Jugulam and Shyam, 2019). Nevertheless, irrespective of the specific resistance mechanism, herbicide-resistant weeds, if left uncontrolled, will pose a significant threat to the global agricultural sector.
High-throughput molecular technologies (such as genomics, proteomics, metabolomics, transcriptomics etc.) have the potential to offer great analytical opportunities to understand and identify the mechanisms of herbicide resistance, thus leading to the identification of novel herbicidal MOAs (Ravet et al., 2018). Development of herbicide resistance in weeds is similar to that of abiotic stress resistance development in plants and can provide insights into the stress resistance mechanisms in plants in general (Hamouzová et al., 2023). Even though during the last decade, continuous progress has been made in the field of DNA, RNA, and protein-based omics studies in herbicide resistance, substantial research areas are yet to be explored. Additionally, to our knowledge, inadequate initiatives have been taken to integrate multiple omics-based studies to elucidate the mechanisms of herbicide resistance in economically significant weeds. Hence, in this mini-review, we will confine our discussion to transcriptomics, metabolomics and the need for their systematic integration to unravel the molecular mechanisms of herbicide resistance in weeds. The transcriptome and metabolome represent two key layers of biological information that can provide novel insights into how weeds develop resistance against herbicides. While transcriptomics provides a global overview on the expression profile of the genes, metabolomics provides a global overview on metabolic pathways and their regulation (Maroli et al., 2018a).
2 Weeds’ response to herbicide stress: effects of herbicide stress on weeds’ metabolic pathways and relevant genes involved
Among the metabolic pathways, the most important ones are carbohydrate metabolism, amino acid metabolism, polyamine metabolism, and lipid metabolism (Arbona et al., 2013). Carbohydrate metabolism is directly linked to photosynthetic performance and is the primary source of energy during stressful periods. Photosystem inhibitors such as atrazine, chlorotoluron and metribuzin are known to be involved in the disruption of the light-capturing reactions and electron transport chain in chloroplasts. These herbicides inhibit the production of ATP and NADPH (via competitive binding to the plastoquinone binding site (QB) on the D1 protein, which is encoded by the PsbA gene), which eventually affects carbohydrate metabolism in the weeds (Ma et al., 2020; Košnarová et al., 2021; Yang et al., 2022a). As of 2022 (according to https://www.weedscience.org/Home.aspx, accessed on July 23rd, 2023), 87 cases of resistance against PSII-inhibiting herbicides have been reported globally. Mutations in the PsbA gene and increased target gene and/or protein expression were identified as the mechanisms of resistance (Košnarová et al., 2021; Yang et al., 2022b). Besides interfering with the electron transport chain, herbicides can also have adverse effects on starch accumulation, sucrose transport and plant’s respiration process, which eventually will affect carbohydrate metabolism pathways. In addition to these, accumulation of sugars with no energetic roles (such as oligosaccharides) has also been detected in several plant species, as a response to abiotic stresses. These compounds are well-known for their indirect associations with reactive oxygen species (ROS) scavenging (Arbona et al., 2013). ROS scavenging is a vital mechanism while preventing oxidative stress and associated cellular damage. In the context of herbicide resistance, metabolism of herbicides via metabolic enzymes leads to oxidative stress within the weed. In answer to this, resistant weeds activate enzymes such as superoxide dismutase, catalase, and peroxidases and perform ROS scavenging, which eventually provides them survival advantages over their susceptible counterparts. However, very few such research on herbicide resistant-weeds have been conducted till date. Apart from carbohydrate metabolism, amino acid and lipid metabolism are also significant metabolic pathways. Acetolactate synthase and 5-enolpyruvylshikimate-3-phosphate synthase are among the most important enzymes involved in amino acid metabolic pathways and targeted by commercially important herbicides (Tan et al., 2006). Both of these enzymes are involved in the synthesis of aromatic amino acids (phenylalanine, tyrosine, and tryptophan). These amino acids play important roles during protein synthesis as well as in the production of various plant defense-related secondary metabolites. Among the important enzymes involved in fatty acid metabolism targeted by herbicides is Acetyl-CoA carboxylase (ACCase). ACCase plays a critical role in fatty acid synthesis (Takano et al., 2020). During stress periods, lipids play vital roles, which includes membrane fluidity and integrity, as signaling molecules and oxidative stress management (Liu et al., 2019).
3 Integrated transcriptomics-metabolomics: understanding the adaptation, tolerance, and resistance in plants
In the era of systems biology research, biological processes and gene regulatory networks can be dynamic and hard to interpret. Advanced omics technologies such as metabolomics and transcriptomics, have contributed significantly to explicating the molecular mechanisms of plant responses to different stresses. In the last few decades, information derived from next-generation sequencing technologies paired with advanced statistical and computational approaches has allowed for the characterization of genes involved in plant abiotic stress (Mehta et al., 2019; Sahoo et al., 2020; Aruna Kumara and Thiruchchelvan, 2021). Previously, thorough characterization of genes involved in stress responses was limited to crops and model systems because of the requisite genomic resources, but recent advancements permit the study of non-model species such as weeds. Despite challenges like the development of suitable methods and pipelines, RNA-seq transcriptome sequencing has been extensively used for the identification of gene families involved in herbicide resistance, particularly the NTSR (Zhao et al., 2017; Bai et al., 2020; Chen et al., 2021). Till date, there are many evidence of the use of transcriptomics and metabolomics in herbicide-resistance research. While transcriptomics can identify the differentially expressed genes, the metabolites are the ultimate result of controlled gene transcription and hence the metabolome can be considered the basis of the system’s observed phenotype. In the study conducted by Wrzesińska-Krupa et al., the authors used a de novo transcriptome of Apera spica-venti to perform RNA-sequencing analysis of pinoxaden-resistant and susceptible plants. They identified several important genes responsible for herbicide resistance, such as isoforms of UDP-GTs and GSTs (along with others) as the prime candidate genes (Wrzesińska-Krupa et al., 2023). In another study, the authors used a comparative RNA-seq transcriptomic approach to understand how florpyrauxifen-benzyl treatment can affect phytohormone biosynthesis and signal transduction in resistant and susceptible Echinochloa crus-galli (L.) P. Beauv (Jin et al., 2023). Zhao et al., used transcriptome profiling and identified twenty-four contigs (including isoforms of CytP450s, GSTs, GTs, ABC-transporters, and others) involved in mesosulfuron-methyl resistance in Alopecurus aequalis (Zhao et al., 2017).
In addition to the RNA-seq studies, metabolomics has also been used in herbicide-resistance studies. The metabolome refers to the complete set of metabolites present within a biological sample. Even though the metabolome represents the smallest domain (among genome, transcriptome, proteome, and metabolome), it is more chemically complex and diverse than its counterparts (Kumar et al., 2017). In the context of understanding herbicide resistance in weeds, the metabolome can provide additional information since it represents additional levels of regulation as well as the end products of regulatory processes (Boonchaisri et al., 2020). For example, in the study conducted by Zulet-Gonzalez et al., the authors used non-targeted gas chromatography-mass spectrometry (GC-MS) and liquid chromatography–mass spectrometry (LC–MS) metabolomic profiling and examined the phytotoxic effects of glyphosate on sensitive and resistant populations of Amaranthus palmeri S. Wats. They didn’t find any differences in the metabolic profiles in the absence of herbicide treatment. However, upon treatment with glyphosate, they detected reduced concentrations of quercetin and its derivatives only in the resistant plants (Zulet-Gonzalez et al., 2023). In another study conducted by Tafoya-Razo et al., the authors conducted metabolic fingerprinting of susceptible and resistant common Avena fatua L. populations using Desorption Electrospray Ionization-Mass Spectrometry (DIESI-MS). The authors found that metabolomic fingerprinting can be successfully used to study the diversities of herbicide-resistance mechanisms, which can be used to study the “geographic mosaic of resistance” (Tafoya-Razo et al., 2022). From the perspective of NTSR mechanisms, metabolites can regulate the constitutive and induced expression of Cyp450s, GSTs, and GTs, thus leading to modulation of their activities during herbicide stress. In a different way, metabolites can also regulate the expression of the NTSR genes by altering the chromatin structure of the gene’s regulatory regions. To date, such studies are yet to be conducted. Comparative metabolomics among the treated and untreated resistant and susceptible plants will enable us to discover the detailed metabolites that are involved in herbicide detoxification, along with determining their relative abundance and activities. Table 1 summarizes the list of transcriptomics and metabolomics-based research works related to herbicide sensitivities.
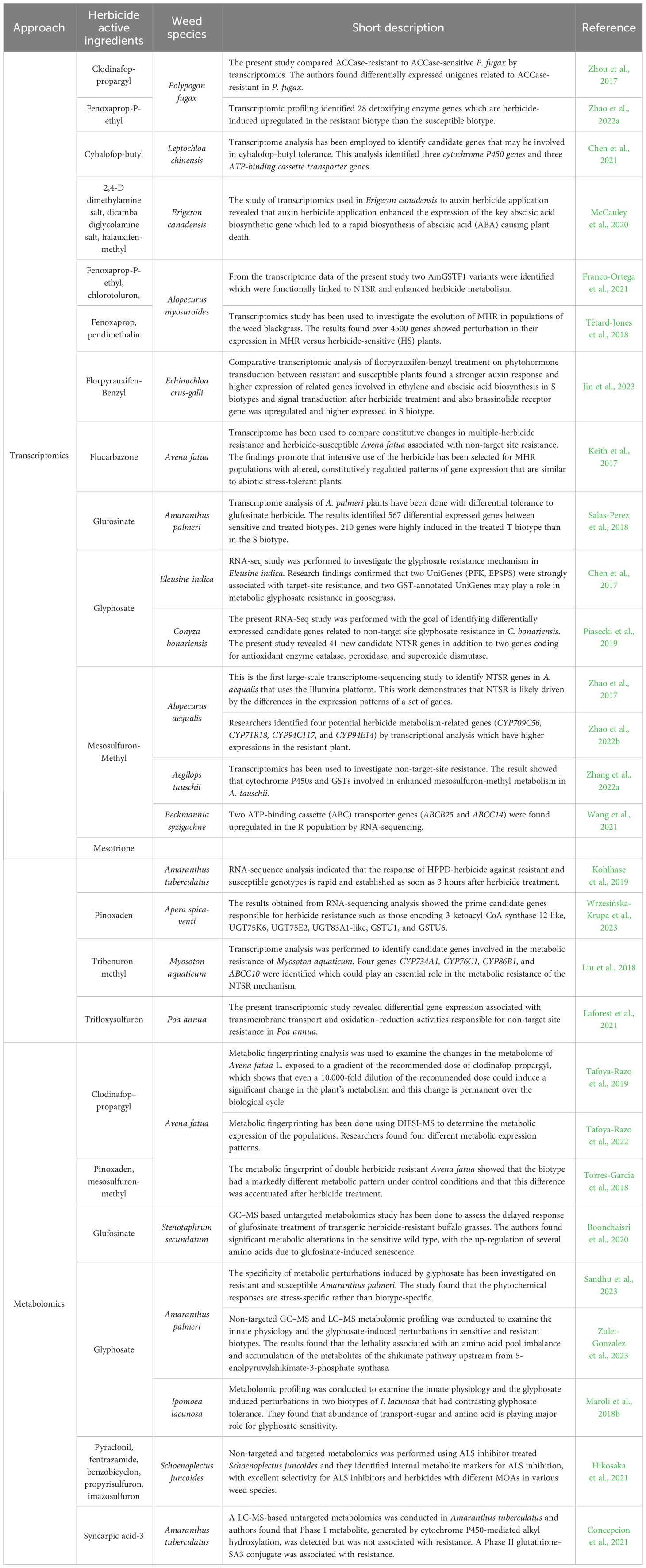
Table 1 Some important transcriptomics and metabolomics-related research works identified in major weedy species.
It is a well-known fact that, within a biological system, transcript abundance does not always translate to protein abundance due to several biological and technical factors. These factors might include post-transcriptional and translational regulatory factors, mRNA stability, protein degradation and turnover, etc. Additionally, even if a protein is formed, it may not inevitably be functional (Liu et al., 2016; Manavella et al., 2023). In this context, correlating the transcriptome and the proteome might be misleading. Therefore, we strongly advise systematically integrating the transcriptome and metabolome data instead. However, it is important to note that post-translational modifications (such as phosphorylation and acetylation) cannot be identified through transcriptomics and metabolomics alone. In such cases, mounting up more data (from other omics such as proteomics and genomics) can boost our understanding of biological complexities and minimize bias. To date, even though there is a lack of research on using the transcriptome and metabolome data for integrated analysis in herbicide resistance research, a few such studies have been conducted in other plants. For example, in a study conducted by Xu et al. (2023a), the authors combined transcriptome and metabolome data from two contrasting apple species (cold-resistant vs cold-sensitive cultivars) to identify the key metabolic pathways involved in response to cold stress. They identified differentially expressed genes (DEGs) and differentially expressed metabolites (DEMs), which provided novel insights into the mechanisms of cold resistance in apple trees in response to cold stress during dormancy (Xu et al., 2023a). In another study conducted in 2023, the authors used integrated transcriptomic-metabolomic analysis to investigate the possible roles of flavonoids involved in resistance to powdery mildew in wheat. They identified DEGs and differentially accumulated flavonoids and validated them using qRT-PCR and biochemical analyses (flavonoids and malondialdehyde content measurements, antioxidant enzyme activities), respectively (Xu et al., 2023b).
Similarly, among the herbicide resistance mechanisms, NTSRs are always believed to be a cumulative effect of several gene families (Ghanizadeh and Harrington, 2017). Hence, the transcriptome can only identify the expression of the important genes, which is often inadequate when studying the weeds as a system. Additionally, the relationship between metabolite and transcript levels might go beyond merely the fact that gene expression affects the global metabolite levels. Alternatively, gene expression might also be regulated by the metabolites, indicating an interconnection between the two (Anjali et al., 2023). Apart from the functional prediction of genes and coexpression analyses, integrated transcriptome-metabolome can also be used to analyze the organizational principle of the whole system (such as systems characterization of pathways) (Xue et al., 2021). In the current scenario, correlating the transcriptome and the metabolome data might allow us to discover the key genes and the metabolic pathways associated with the herbicide resistance in the system. Parallel analysis of transcript and metabolite profiling can expose novel gene-to-metabolite networks and hence can realistically screen candidate genes involve in herbicide resistance (for example, exactly which isoforms of Cyp450s or GSTs or GTs) (Fukushima et al., 2009; Nakabayashi and Saito, 2015). Currently, there are quite a few comprehensible gene-coexpression tools that are specifically designed for plant genomics research. Some of them include Arabidopsis Coexpression Tool (ACT), Arabidopsis thaliana transcription factor and protein interaction database (ATTED-II), Genevestigator, Co-expression Platform (CoP), PlantPAN 3.0 and GENEVESTIGATOR V4 (Nakabayashi and Saito, 2015; Zogopoulos et al., 2021). Apart from the mentioned comprehensible gene-coexpression tools, there are also several publicly available tools and software platforms that could assist in the parallel analysis of transcriptome and metabolome data. Such tools include MetaboAnalyst (current version: MetaboAnalyst 5.0), GEMINI (Gene Expression and Metabolism Integrated for Network Inference), Plant MetGenMAP, mixOmics, etc. MetaboAnalyst (https://www.metaboanalyst.ca/MetaboAnalyst/home.xhtml) is a user-friendly web-based platform designed exclusively for metabolomics data analysis that offers a wide range of capabilities, from raw MS spectra processing to comprehensive data normalization and integration with other omics data (Pang et al., 2022). Plant MetGenMAP (http://bioinfo.bti.cornell.edu/cgi-bin/MetGenMAP/home.cgi) is a web-based system that allows the user to identify the changed pathways from gene expression and/or metabolite profile data. This system enables data visualization in a biochemical pathway context (Joung et al., 2009). GEMINI is a software tool designed to infer regulatory networks that connect genes and metabolites, and hence can be considered as an important tool for the integrated analysis of transcriptomics and metabolomics data (Chandrasekaran, 2019). mixOmics is a collaborative R package-based project (between Australia, France, and Canada) that focuses on multi-omics data integration. This tool can be used for integrative analysis of transcriptomics and metabolomics data, with a special emphasis on dimension reduction (Rohart et al., 2017). Figure 1 describes a schematic framework of the experimental design based on integrated transcriptomics and metabolomics in weeds. Following the analysis of individual transcriptome and metabolome data, statistical and bioinformatic techniques, such as correlation analysis, can be used to integrate them. The coexpression of genes and the production of metabolites can be measured by different types of correlation coefficients such as Pearson correlation coefficient (r), Spearman rank correlation (ρ), Kendall’s Tau (τ) and weighted gene coexpression network analysis (WGCNA). For example, in a study conducted by Yang et al., the authors had used Pearson correlation coefficients and their corresponding p-values to discover metabolites and related genes from a combined metabolomic and transcriptomic analysis (Yang et al., 2022b). In another study conducted by Zhang et al., the authors had successfully identified key structural genes responsible for anthocyanin biosynthesis mechanisms in Vaccinium corymbosum L. using integrated transcriptome and metabolome analysis. They had also used Pearson correlation coefficient values for screening out the genes of interest (Zhang et al., 2022b). In the context of herbicide resistance mechanism discovery, these tools can be used to assess how changes in gene expression levels correspond to variations in metabolite abundances, and vice versa. Based on the integration results, the genes showing significant correlations with specific metabolites can be identified. Prior knowledge of metabolic pathways in combination with the metabolome result can be used to assess the possible biological relevance of these identified genes to herbicide resistance.
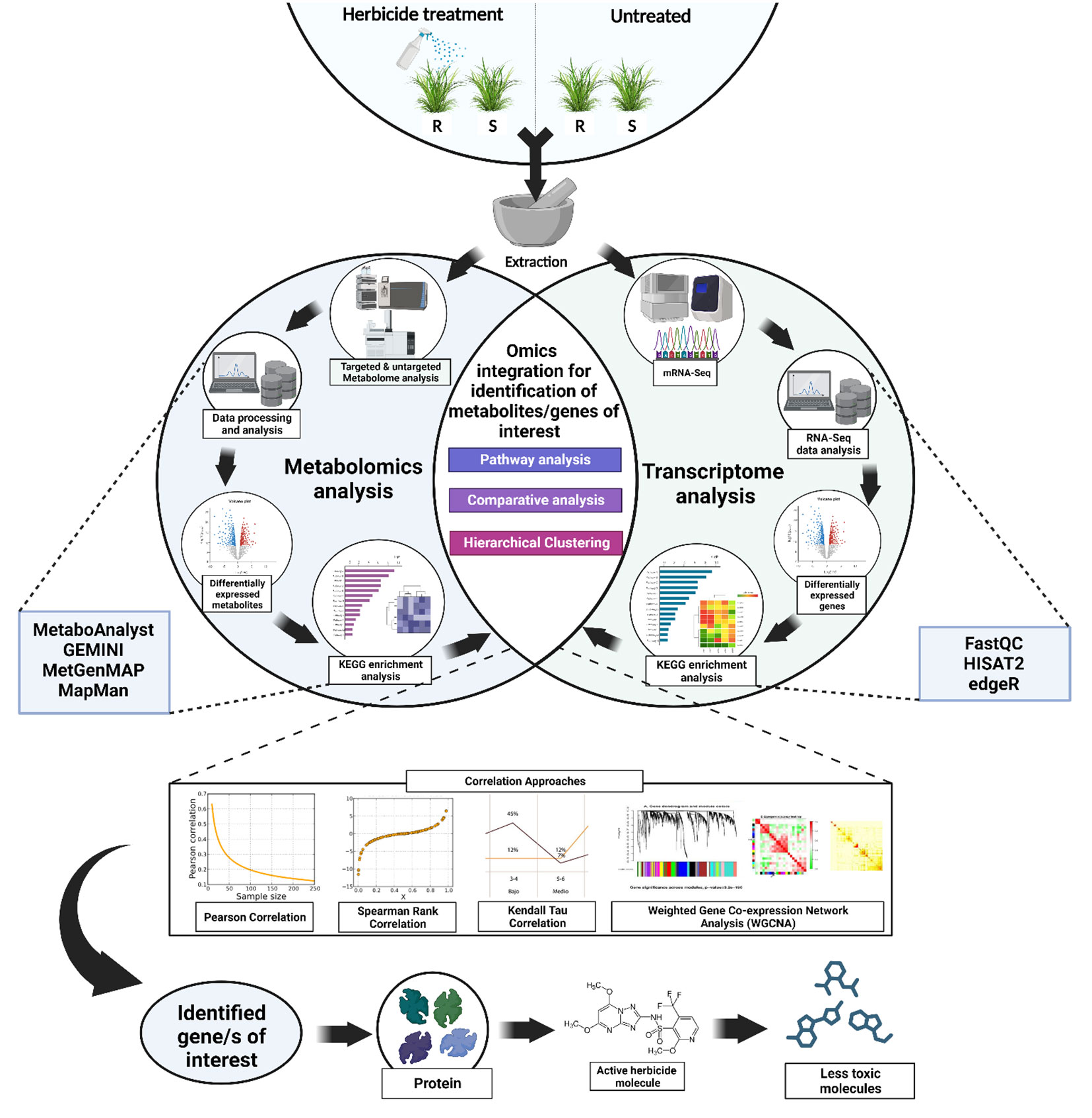
Figure 1 A simplified framework showing the possible experimental design based on integrated transcriptomics and metabolomics in weeds. The RNA-Seq transcriptome analysis will help to identify the differentially expressed genes under herbicide stress (such as genes encoding cytochrome P450s, GSTs, GTs, transporter proteins, oxidases, peroxidases etc.). Thereafter, the metabolite profiling using metabolomics [using Gas Chromatography/Mass Spectrometry (GC/MS) or Liquid Chromatography/Mass Spectrometry (LC/MS)] will further confirm the involvement of these differentially produced metabolic enzymes. Following the analysis of individual omics data, statistical and bioinformatic techniques, such as correlation analysis using Pearson correlation coefficient, Spearman rank correlation (ρ), kendall’s Tau (τ) and weighted gene coexpression network analysis (WGCNA)., can be used to integrate them. Suitable significance thresholds can be set to identify the significant associations between gene expression and metabolite abundance. The integration of these omics approaches can establish a link between phenotypic outcome to genotypic expression and hence can provide a system-level understanding of herbicide resistance mechanisms in weeds.
4 Summary and outlook: current challenges and way forward
Individually, both metabolomics and transcriptomics are powerful tools for understanding the molecular basis of herbicide resistance. However, determining whether expression of a particular gene or increased concentration of a particular metabolite is the cause or consequence of herbicide adaptation is a difficult task. Such questions can be answered by integrated transcriptome-metabolome studies. Despite significant advances in omics-based studies of herbicide resistance, to the best of our knowledge, to date there have been inadequate efforts made to investigate the power and potential of transcriptome-metabolome correlation analysis in herbicide resistance. This can likely be attributed to the limited availability of genomic and metabolome resources in weeds. Even though RNA-seq studies have been successfully used to study evolutionary processes in weeds, they have their own challenges. The main issue to address is the proportion of mismatches, especially when genetic differences between species are high. In such cases, the portion of the reads mapping ambiguously to more than one contig increases, which might lead to erroneous result interpretation and further downstream processes (Fukushima et al., 2009). Hence, high-resolution genomic analyses of weedy plants will be needed in the future. Alongside transcriptome studies, metabolome studies on weeds also have numerous challenges, which might range from constrained genomic resources and reference databases to the complexity of the metabolite profiles. In weeds, the lack of reference databases can make it difficult to identify and annotate all the metabolites. Furthermore, there might be many anonymous metabolites, which can also impede precise result interpretations and hence make biomarker discovery difficult in weeds.
Besides individual omics, the application of integrated omics approaches to weeds can also be challenging and requires careful consideration. One of the most important data integration challenges is that the transcriptome and metabolite information do not correlate for some of the genes and metabolites. This might be due to complicated regulatory networks ranging from feedback loops to co-regulation and compensatory mechanisms (Stelling et al., 2002; Vemuri and Aristidou, 2005). Additionally, metabolites within cells can have varying turnover rates. Hence, even if gene expression remains constant, that does not imply that metabolite levels will also remain constant (Li et al., 2022). Hence, in these cases, gene-metabolite correlations may not be evident from short time scale experiments. Also, there can be some technical factors and threshold effects involved, which can initiate weak correlations. For example, minor changes in gene expression might not lead to measurable changes in metabolite levels until a certain threshold is reached, or there might be some abnormalities regarding the normalization methods and data preprocessing choices that might obscure the true correlations. Addressing these challenges might require sophisticated and refined statistical analysis methods, and weeds might have unknown statistical challenges that need to be addressed (Kumar et al., 2017). Another challenge might be the huge diversity among the weed species, resulting in substantial physiological and genetic heterogeneity even within a single species. Due to species diversity, each might have its own unique genetic makeup and biological characteristics, and hence the resistance mechanisms might also vary significantly (Owen, 2016). High species diversity might also limit the broader applicability of research findings from multi-omics data since the perceptions gained from one environment may not apply directly to another. This scenario might demand more advanced bioinformatics pipelines to accommodate the unique genomic features of each species. This will require specialized expertise. However, the most crucial challenge in integrated transcriptomic-metabolomic analyses is based on the fact that there is no direct connection between metabolite and transcript. The differentially expressed transcripts cannot be mapped to a single metabolome database. Hence, the data analysis becomes more complicated; however, this impasse can be overcome by reducing the number of metabolites. Comparative metabolomic analyses, such as comparison between herbicide-resistant and susceptible weeds and identification of the specific features, can be implied to reduce the undesirable metabolites. Nevertheless, despite the challenges, transcriptomic-metabolomic integration is a powerful combination and a fascinating field of research that undeniably requires additional exploration and the involvement of more geneticists and weed scientists.
Author contributions
MKS: Conceptualization, Data curation, Formal analysis, Investigation, Supervision, Writing – original draft, Writing – review & editing. SB: Data curation, Writing – original draft, Writing – review & editing. RB: Data curation, Formal analysis, Investigation, Software, Writing – original draft, Writing – review & editing. KH: Formal analysis, Supervision, Validation, Writing – review & editing. JS: Formal analysis, Funding acquisition, Project administration, Resources, Supervision, Validation, Writing – review & editing.
Funding
The author(s) declare financial support was received for the research, authorship, and/or publication of this article. This work was supported by grant from the Long-term conceptual development of research organization [The Ministry of Education, Youth and Sports (Czech Republic)]. Rohit Bharati was supported by the Internal Grant Agency, grant number 20233105, Faculty of Tropical AgriSciences, Czech University of Life Sciences in Prague.
Conflict of interest
The authors declare that the research was conducted in the absence of any commercial or financial relationships that could be construed as a potential conflict of interest.
Publisher’s note
All claims expressed in this article are solely those of the authors and do not necessarily represent those of their affiliated organizations, or those of the publisher, the editors and the reviewers. Any product that may be evaluated in this article, or claim that may be made by its manufacturer, is not guaranteed or endorsed by the publisher.
References
Anjali, Kumar, S., Korra, T., Thakur, R., Arutselvan, R., Kashyap, A. S., et al. (2023). Role of plant secondary metabolites in defence and transcriptional regulation in response to biotic stress. Plant Stress 8, 100154. doi: 10.1016/j.stress.2023.100154
Arbona, V., Manzi, M., de Ollas, C., Gómez-Cadenas, A. (2013). Metabolomics as a tool to investigate abiotic stress tolerance in plants. Int. J. Mol. Sci. 14, 4885–4911. doi: 10.3390/ijms14034885
Aruna Kumara, U. M., Thiruchchelvan, N. (2021). “Omics technology for plant stress management,” in Microbiological Activity for Soil and Plant Health Management. Eds. Soni, R., Suyal, D. C., Bhargava, P., Goel, R. (Singapore: Springer), 375–412. doi: 10.1007/978-981-16-2922-8_16
Bai, S., Zhao, Y., Zhou, Y., Wang, M., Li, Y., Luo, X., et al. (2020). Identification and expression of main genes involved in non-target site resistance mechanisms to fenoxaprop-p-ethyl in Beckmannia syzigachne. Pest Manage. Sci. 76, 2619–2626. doi: 10.1002/ps.5800
Boonchaisri, S., Stevenson, T., Dias, D. A. (2020). Utilization of GC–MS untargeted metabolomics to assess the delayed response of glufosinate treatment of transgenic herbicide resistant (HR) bffalo grasses (Stenotaphrum secundatum L.). Metabolomics 16, 22. doi: 10.1007/s11306-020-1644-9
Chandrasekaran, S. (2019). A protocol for the construction and curation of genome-scale integrated metabolic and regulatory network models. Methods Mol. Biol. 1927, 203–214. doi: 10.1007/978-1-4939-9142-6_14
Chen, J., Huang, H., Wei, S., Huang, Z., Wang, X., Zhang, C. (2017). Investigating the mechanisms of glyphosate resistance in goosegrass (Eleusine indica (L.) Gaertn.) by RNA sequencing technology. Plant J. 89, 407–415. doi: 10.1111/tpj.13395
Chen, K., Peng, Y., Zhang, L., Wang, L., Mao, D., Zhao, Z., et al. (2021). Whole transcriptome analysis resulted in the identification of Chinese sprangletop (Leptochloa chinensis) genes involved in cyhalofop-butyl tolerance. BMC Genomics 22, 521. doi: 10.1186/s12864-021-07856-z
Concepcion, J. C. T., Kaundun, S. S., Morris, J. A., Hutchings, S.-J., Strom, S. A., Lygin, A. V., et al. (2021). Resistance to a nonselective 4-hydroxyphenylpyruvate dioxygenase-inhibiting herbicide via novel reduction-dehydration-glutathione conjugation in Amaranthus tuberculatus. New Phytol. 232, 2089–2105. doi: 10.1111/nph.17708
Franco-Ortega, S., Goldberg-Cavalleri, A., Walker, A., Brazier-Hicks, M., Onkokesung, N., Edwards, R. (2021). Non-target site herbicide resistance is conferred by two distinct mechanisms in black-grass (Alopecurus myosuroides). Front. Plant Sci. 12. doi: 10.3389/fpls.2021.636652
Fukushima, A., Kusano, M., Redestig, H., Arita, M., Saito, K. (2009). Integrated omics approaches in plant systems biology. Curr. Opin. Chem. Biol. 13, 532–538. doi: 10.1016/j.cbpa.2009.09.022
Gaines, T. A., Duke, S. O., Morran, S., Rigon, C. A. G., Tranel, P. J., Küpper, A., et al. (2020). Mechanisms of evolved herbicide resistance. J. Biol. Chem 30, 10307–10330. doi: 10.1074/jbc.REV120.013572
Ghanizadeh, H., Harrington, K. C. (2017). Non-target site mechanisms of resistance to herbicides. Crit. Rev. Plant Sci. 36, 24–34. doi: 10.1080/07352689.2017.1316134
Goldberg-Cavalleri, A., Onkokesung, N., Franco-Ortega, S., Edwards, R. (2023). ABC transporters linked to multiple herbicide resistance in blackgrass (Alopecurus myosuroides). Front. Plant Sci. 14. doi: 10.3389/fpls.2023.1082761
Hamouzová, K., Sen, M. K., Bharati, R., Košnarová, P., Chawdhery, M. R. A., Roy, A., et al. (2023). Calcium signaling in weeds under herbicide stress: An outlook. Front. Plant Sci. 14. doi: 10.3389/fpls.2023.1135845
Hikosaka, M., Iwahashi, F., Yamato, S. (2021). Metabolomic analysis of Schoenoplectus juncoides reveals common markers of acetolactate synthase inhibition among paddy weeds. Pesticide Biochem. Physiol. 174, 104827. doi: 10.1016/j.pestbp.2021.104827
Jin, W., Sun, J., Tang, W., Yang, Y., Zhang, J., Lu, Y., et al. (2023). Comparative transcriptome analysis of the differential effects of florpyrauxifen-benzyl treatment on phytohormone transduction between florpyrauxifen-benzyl-resistant and -susceptible barnyard grasses (Echinochloa crus-galli (L.) P. Beauv). Agronomy 13, 702. doi: 10.3390/agronomy13030702
Joung, J.-G., Corbett, A. M., Fellman, S. M., Tieman, D. M., Klee, H. J., Giovannoni, J. J., et al. (2009). Plant metGenMAP: an integrative analysis system for plant systems biology. Plant Physiol. 151, 1758–1768. doi: 10.1104/pp.109.145169
Jugulam, M., Shyam, C. (2019). Non-target-site resistance to herbicides: recent developments. Plants 8, 417. doi: 10.3390/plants8100417
Keith, B. K., Burns, E. E., Bothner, B., Carey, C. C., Mazurie, A. J., Hilmer, J. K., et al. (2017). Intensive herbicide use has selected for constitutively elevated levels of stress-responsive mRNAs and proteins in multiple herbicide-resistant Avena fatua L. Pest Manag Sci. 73, 2267–2281. doi: 10.1002/ps.4605
Kohlhase, D. R., O’Rourke, J. A., Owen, M. D. K., Graham, M. A. (2019). Using RNA-seq to characterize responses to 4-hydroxyphenylpyruvate dioxygenase (HPPD) inhibitor herbicide resistance in waterhemp (Amaranthus tuberculatus). BMC Plant Biol. 19, 182. doi: 10.1186/s12870-019-1795-x
Košnarová, P., Hamouz, P., Hamouzová, K., Linn, A., Sen, M. K., Mikulka, J., et al. (2021). Apera spica-venti in the Czech Republic develops resistance to three herbicide modes of action. Weed Res. 61, 420–429. doi: 10.1111/wre.12500
Kumar, R., Bohra, A., Pandey, A. K., Pandey, M. K., Kumar, A. (2017). Metabolomics for plant improvement: status and prospects. Front. Plant Sci. 8. doi: 10.3389/fpls.2017.01302
Laforest, M., Soufiane, B., Patterson, E. L., Vargas, J. J., Boggess, S. L., Houston, L. C., et al. (2021). Differential expression of genes associated with non-target site resistance in Poa annua with target site resistance to acetolactate synthase inhibitors. Pest Manag Sci. 77, 4993–5000. doi: 10.1002/ps.6541
Lan, Y., Sun, Y., Liu, Z., Wei, S., Huang, H., Cao, Y., et al. (2022). Mechanism of resistance to pyroxsulam in multiple-resistant Alopecurus myosuroides from China. Plants 11, 1645. doi: 10.3390/plants11131645
Li, H., Barbour, J. A., Zhu, X., Wong, J. W. H. (2022). Gene expression is a poor predictor of steady-state metabolite abundance in cancer cells. FASEB J. 36, e22296. doi: 10.1096/fj.202101921RR
Liu, W., Bai, S., Zhao, N., Jia, S., Li, W., Zhang, L., et al. (2018). Non-target site-based resistance to tribenuron-methyl and essential involved genes in Myosoton aquaticum (L.). BMC Plant Biol. 18, 225. doi: 10.1186/s12870-018-1451-x
Liu, X., Ma, D., Zhang, Z., Wang, S., Du, S., Deng, X., et al. (2019). Plant lipid remodeling in response to abiotic stresses. Environ. Exp. Bot. 165, 174–184. doi: 10.1016/j.envexpbot.2019.06.005
Liu, Y., Beyer, A., Aebersold, R. (2016). On the dependency of cellular protein levels on mRNA abundance. Cell 165, 535–550. doi: 10.1016/j.cell.2016.03.014
Ma, H., Lu, H., Han, H., Yu, Q., Powles, S. (2020). Metribuzin resistance via enhanced metabolism in a multiple herbicide resistant Lolium rigidum population. Pest Manage. Sci. 76, 3785–3791. doi: 10.1002/ps.5929
Manavella, P. A., Godoy Herz, M. A., Kornblihtt, A. R., Sorenson, R., Sieburth, L. E., Nakaminami, K., et al. (2023). Beyond transcription: compelling open questions in plant RNA biology. Plant Cell 35, 1626–1653. doi: 10.1093/plcell/koac346
Manicardi, A., Milani, A., Scarabel, L., Mora, G., Recasens, J., Llenes, J. M., et al. (2023). First report of glyphosate resistance in an Amaranthus palmeri population from Europe. Weed Res. doi: 10.1111/wre.12579
Maroli, A. S., Gaines, T. A., Foley, M. E., Duke, S. O., Doğramacı, M., Anderson, J. V., et al. (2018a). Omics in weed science: A perspective from genomics, transcriptomics, and metabolomics approaches. Weed Sci. 66, 681–695. doi: 10.1017/wsc.2018.33
Maroli, A. S., Nandula, V. K., Duke, S. O., Gerard, P., Tharayil, N. (2018b). Comparative metabolomic analyses of ipomoea lacunosa biotypes with contrasting glyphosate tolerance captures herbicide-induced differential perturbations in cellular physiology. J. Agric. Food Chem. 66, 2027–2039. doi: 10.1021/acs.jafc.7b04722
McCauley, C. L., McAdam, S. A. M., Bhide, K., Thimmapuram, J., Banks, J. A., Young, B. G. (2020). Transcriptomics in Erigeron canadensis reveals rapid photosynthetic and hormonal responses to auxin herbicide application. J. Exp. Bot. 71, 3701–3709. doi: 10.1093/jxb/eraa124
Mehta, S., James, D., Reddy, M. K. (2019). “Omics technologies for abiotic stress tolerance in plants: current status and prospects,” in Recent approaches in omics for plant resilience to climate change. Ed. Wani, S. H. (Cham: Springer International Publishing), 1–34. doi: 10.1007/978-3-030-21687-0_1
Nakabayashi, R., Saito, K. (2015). Integrated metabolomics for abiotic stress responses in plants. Curr. Opin. Plant Biol. 24, 10–16. doi: 10.1016/j.pbi.2015.01.003
Owen, M. D. K. (2016). Diverse approaches to herbicide-resistant weed management. Weed Sci. 64, 570–584. doi: 10.1614/WS-D-15-00117.1
Pang, Z., Zhou, G., Ewald, J., Chang, L., Hacariz, O., Basu, N., et al. (2022). Using MetaboAnalyst 5.0 for LC–HRMS spectra processing, multi-omics integration and covariate adjustment of global metabolomics data. Nat. Protoc. 17, 1735–1761. doi: 10.1038/s41596-022-00710-w
Papapanagiotou, A. P., Damalas, C. A., Bosmali, I., Madesis, P., Menexes, G., Eleftherohorinos, I. (2022). Multiple resistance of silky windgrass to acetolactate synthase- and acetyl-CoA synthase–inhibiting herbicides. Weed Technol. 36, 334–343. doi: 10.1017/wet.2022.24
Piasecki, C., Yang, Y., Benemann, D. P., Kremer, F. S., Galli, V., Millwood, R. J., et al. (2019). Transcriptomic analysis identifies new non-target site glyphosate-resistance genes in Conyza bonariensis. Plants (Basel) 8, 157. doi: 10.3390/plants8060157
Ravet, K., Patterson, E. L., Krähmer, H., Hamouzová, K., Fan, L., Jasieniuk, M., et al. (2018). The power and potential of genomics in weed biology and management. Pest Manage. Sci. 74, 2216–2225. doi: 10.1002/ps.5048
Rohart, F., Gautier, B., Singh, A., Cao, K.-A. L. (2017). mixOmics: An R package for ‘omics feature selection and multiple data integration. PloS Comput. Biol. 13, e1005752. doi: 10.1371/journal.pcbi.1005752
Sahoo, J. P., Behera, L., Sharma, S. S., Praveena, J., Nayak, S. K., Samal, K. C. (2020). Omics studies and systems biology perspective towards abiotic stress response in plants. Am. J. Plant Sci. 11, 2172–2194. doi: 10.4236/ajps.2020.1112152
Salas-Perez, R. A., Saski, C. A., Noorai, R. E., Srivastava, S. K., Lawton-Rauh, A. L., Nichols, R. L., et al. (2018). RNA-Seq transcriptome analysis of Amaranthus palmeri with differential tolerance to glufosinate herbicide. PloS One 13, e0195488. doi: 10.1371/journal.pone.0195488
Sandhu, P. K., Leonard, E., Nandula, V., Tharayil, N. (2023). Global metabolome of palmer amaranth (Amaranthus palmeri) populations highlights the specificity and inducibility of phytochemical responses to abiotic stress. J. Agric. Food Chem. 71, 3518–3530. doi: 10.1021/acs.jafc.2c07162
Sen, M. K., Hamouzová, K., Mikulka, J., Bharati, R., Košnarová, P., Hamouz, P., et al. (2021). Enhanced metabolism and target gene overexpression confer resistance against acetolactate synthase-inhibiting herbicides in Bromus sterilis. Pest Manage. Sci. 77, 2122–2128. doi: 10.1002/ps.6241
Stelling, J., Klamt, S., Bettenbrock, K., Schuster, S., Gilles, E. D. (2002). Metabolic network structure determines key aspects of functionality and regulation. Nature 420, 190–193. doi: 10.1038/nature01166
Tafoya-Razo, J. A., Mora-Munguía, S. A., Torres-García, J. R. (2022). Diversity of herbicide-resistance mechanisms of avena fatua L. @ to acetyl-coA carboxylase-inhibiting herbicides in the Bajio, Mexico. Plants 11, 1644. doi: 10.3390/plants11131644
Tafoya-Razo, J. A., Oregel-Zamudio, E., Velázquez-Márquez, S., Torres-García, J. R. (2019). 10,000-times diluted doses of ACCase-inhibiting herbicides can permanently change the metabolomic fingerprint of susceptible Avena fatua L. Plants. Plants (Basel) 8, 368. doi: 10.3390/plants8100368
Takano, H. K., Ovejero, R. F. L., Belchior, G. G., Maymone, G. P. L., Dayan, F. E. (2020). ACCase-inhibiting herbicides: mechanism of action, resistance evolution and stewardship. Sci. Agric. (Piracicaba Braz.) 78, e20190102. doi: 10.1590/1678-992X-2019-0102
Tan, S., Evans, R., Singh, B. (2006). Herbicidal inhibitors of amino acid biosynthesis and herbicide-tolerant crops. Amino Acids 30, 195–204. doi: 10.1007/s00726-005-0254-1
Tehranchian, P., Nandula, V. K., Matzrafi, M., Jasieniuk, M. (2019). Multiple herbicide resistance in California Italian ryegrass (Lolium perenne ssp. multiflorum): characterization of ALS-inhibiting herbicide resistance. Weed Sci. 67, 273–280. doi: 10.1017/wsc.2019.1
Tétard-Jones, C., Sabbadin, F., Moss, S., Hull, R., Neve, P., Edwards, R. (2018). Changes in the proteome of the problem weed blackgrass correlating with multiple-herbicide resistance. Plant J. 94, 709–720. doi: 10.1111/tpj.13892
Torra, J., Alcántara-de la Cruz, R. (2022). Molecular mechanisms of herbicide resistance in weeds. Genes 13, 2025. doi: 10.3390/genes13112025
Torres-García, J. R., Tafoya-Razo, J. A., Velázquez-Márquez, S., Tiessen, A. (2018). Double herbicide-resistant biotypes of wild oat (Avena fatua) display characteristic metabolic fingerprints before and after applying ACCase- and ALS-inhibitors. Acta Physiol. Plant 40, 119. doi: 10.1007/s11738-018-2691-y
Vemuri, G. N., Aristidou, A. A. (2005). Metabolic engineering in the -omics era: elucidating and modulating regulatory networks. Microbiol. Mol. Biol. Rev. 69, 197–216. doi: 10.1128/MMBR.69.2.197-216.2005
Wang, J., Chen, J., Li, X., Cui, H. (2021). RNA-Seq transcriptome analysis to identify candidate genes involved in non-target site-based mesosulfuron-methyl resistance in Beckmannia syzigachne. Pesticide Biochem. Physiol. 171, 104738. doi: 10.1016/j.pestbp.2020.104738
Wrzesińska-Krupa, B., Szmatoła, T., Praczyk, T., Obrępalska-Stęplowska, A. (2023). Transcriptome analysis indicates the involvement of herbicide-responsive and plant-pathogen interaction pathways in the development of resistance to ACCase inhibitors in Apera spica-venti. Pest Manag Sci. 79, 1944–1962. doi: 10.1002/ps.7370
Xu, G., Li, L., Zhou, J., Lyu, D., Zhao, D., Qin, S. (2023a). Comparison of transcriptome and metabolome analysis revealed differences in cold resistant metabolic pathways in different apple cultivars under low temperature stress. Hortic. Plant J. 9, 183–198. doi: 10.1016/j.hpj.2022.09.002
Xu, W., Xu, X., Han, R., Wang, X., Wang, K., Qi, G., et al. (2023b). Integrated transcriptome and metabolome analysis reveals that flavonoids function in wheat resistance to powdery mildew. Front. Plant Sci. 14. doi: 10.3389/fpls.2023.1125194
Xue, J., Lu, D., Wang, S., Lu, Z., Liu, W., Wang, X., et al. (2021). Integrated transcriptomic and metabolomic analysis provides insight into the regulation of leaf senescence in rice. Sci. Rep. 11, 14083. doi: 10.1038/s41598-021-93532-x
Yang, C., Wu, P., Yao, X., Sheng, Y., Zhang, C., Lin, P., et al. (2022b). Integrated Transcriptome and Metabolome Analysis Reveals Key Metabolites Involved in Camellia oleifera Defense against Anthracnose. Int. J. Mol. Sci. 23, 536. doi: 10.3390/ijms23010536
Yang, J., Yu, H., Cui, H., Chen, J., Li, X. (2022a). PsbA gene over-expression and enhanced metabolism conferring resistance to atrazine in Commelina communis. Pesticide Biochem. Physiol. 188, 105260. doi: 10.1016/j.pestbp.2022.105260
Zhang, J., Li, S., An, H., Zhang, X., Zhou, B. (2022b). Integrated transcriptome and metabolome analysis reveals the anthocyanin biosynthesis mechanisms in blueberry (Vaccinium corymbosum L.) leaves under different light qualities. Front. Plant Sci. 13. doi: 10.3389/fpls.2022.1073332
Zhang, D., Li, X., Bei, F., Jin, T., Jia, S., Bu, R., et al. (2022a). Investigating the metabolic mesosulfuron-methyl resistance in aegilops tauschii coss. By transcriptome sequencing combined with the reference genome. J. Agric. Food Chem. 70, 11429–11440. doi: 10.1021/acs.jafc.2c04529
Zhao, N., Li, W., Bai, S., Guo, W., Yuan, G., Wang, F., et al. (2017). Transcriptome profiling to identify genes involved in mesosulfuron-methyl resistance in Alopecurus aequalis. Front. Plant Sci. 8. doi: 10.3389/fpls.2017.01391
Zhao, N., Yan, Y., Liu, W., Wang, J. (2022b). Cytochrome P450 CYP709C56 metabolizing mesosulfuron-methyl confers herbicide resistance in Alopecurus aequalis. Cell Mol. Life Sci. 79, 205. doi: 10.1007/s00018-022-04171-y
Zhao, N., Yang, J., Jiang, M., Liao, M., Cao, H. (2022a). Identification of essential genes involved in metabolism-based resistance mechanism to fenoxaprop-P-ethyl in Polypogon fugax. Pest Manag Sci. 78, 1164–1175. doi: 10.1002/ps.6733
Zhou, F., Zhang, Y., Tang, W., Wang, M., Gao, T. (2017). Transcriptomics analysis of the flowering regulatory genes involved in the herbicide resistance of Asia minor bluegrass (Polypogon fugax). BMC Genomics 18, 953. doi: 10.1186/s12864-017-4324-z
Zhu, G., Wang, H., Gao, H., Liu, Y., Li, J., Feng, Z., et al. (2023). Multiple resistance to three modes of action of herbicides in a single italian ryegrass (Lolium multiflorum L.) population in China. Agronomy 13, 216. doi: 10.3390/agronomy13010216
Zogopoulos, V. L., Saxami, G., Malatras, A., Angelopoulou, A., Jen, C.-H., Duddy, W. J., et al. (2021). Arabidopsis Coexpression Tool: a tool for gene coexpression analysis in Arabidopsis thaliana. iScience 24, 102848. doi: 10.1016/j.isci.2021.102848
Keywords: abiotic stresses, herbicide resistance, metabolomics, omics technologies, transcriptomic regulation
Citation: Sen MK, Bhattacharya S, Bharati R, Hamouzová K and Soukup J (2023) Comprehensive insights into herbicide resistance mechanisms in weeds: a synergistic integration of transcriptomic and metabolomic analyses. Front. Plant Sci. 14:1280118. doi: 10.3389/fpls.2023.1280118
Received: 19 August 2023; Accepted: 25 September 2023;
Published: 11 October 2023.
Edited by:
Dinesh Adhikary, University of Alberta, CanadaReviewed by:
Wei Zhu, Chinese Academy of Sciences (CAS), ChinaQijie Guan, University of Mississippi, United States
Aleya Ferdausi, University of Alberta, Canada
Copyright © 2023 Sen, Bhattacharya, Bharati, Hamouzová and Soukup. This is an open-access article distributed under the terms of the Creative Commons Attribution License (CC BY). The use, distribution or reproduction in other forums is permitted, provided the original author(s) and the copyright owner(s) are credited and that the original publication in this journal is cited, in accordance with accepted academic practice. No use, distribution or reproduction is permitted which does not comply with these terms.
*Correspondence: Madhab Kumar Sen, c2VubUBhZi5jenUuY3o=; Katerina Hamouzová, aGFtb3V6b3ZhQGFmLmN6dS5jeg==