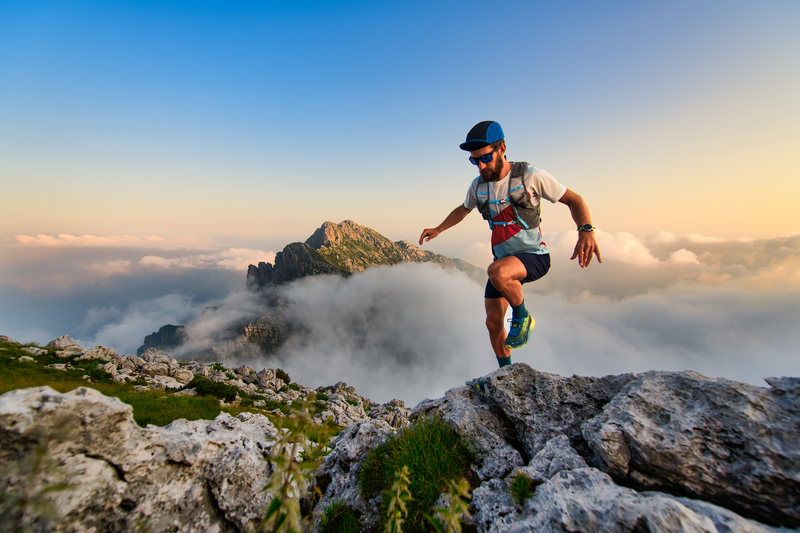
94% of researchers rate our articles as excellent or good
Learn more about the work of our research integrity team to safeguard the quality of each article we publish.
Find out more
ORIGINAL RESEARCH article
Front. Plant Sci. , 09 January 2024
Sec. Functional Plant Ecology
Volume 14 - 2023 | https://doi.org/10.3389/fpls.2023.1279019
Introduction: Goji berry (Lycium barbarum L.) is a famous edible and medicinal herb worldwide with considerable consumption. The recent cultivation of goji berries in the Daodi region was seriously reduced due to increased production costs and the influence of policy on preventing nongrain use of arable land in China. Consequently, production of Daodi goji berry was insufficient to meet market demands for high-quality medicinal materials. Searching for regions similar to the Daodi region was necessary.
Methods: The MaxEnt model was used to predicted the current and future potential regions suitable for goji berry in China based on the environmental characteristics of the Daodi region (including Zhongning County of Zhongwei prefecture-level city, and its surroundings), and the ArcGIS software was used to analyze the changes in its suitable region.
Results: The results showed that when the parameters were FC = LQHP and RM = 2.1, the MaxEnt model was optimal, and the AUC and TSS values were greater than 0.90. The mean temperature and precipitation of the coldest quarter were the most critical variables shaping the distribution of Daodi goji berries. Under current climate conditions, the suitable habitats of the Daodi goji berry were 45,973.88 km2, accounting for 0.48% of China’s land area, which were concentrated in the central and western Ningxia Province (22,589.42 km2), and the central region of Gansu Province (18,787.07 km2) bordering western Ningxia. Under future climate scenarios, the suitable area was higher than that under current climate conditions and reached the maximum under RCP 6.0 (91,256.42 km2) in the 2050s and RCP 8.5 (82,459.17 km2) in the 2070s. The expansion regions were mainly distributed in the northeast of the current suitable ranges, and the distributional centroids were mainly shifted to the northeast. The moderately and highly suitable overlapping habitats were mainly distributed in Baiyin (7,241.75 km2), Zhongwei (6,757.81 km2), and Wuzhong (5, 236.87 km2) prefecture-level cities.
Discussion: In this stduy, MaxEnt and ArcGIS were applied to predict and analyze the suitable habitats of Daodi goji berry in China under climate change. Our results indicate that climate warming is conducive to cultivating Daodi goji berry and will not cause a shift in the Daodi region. The goji berry produced in Baiyin could be used to satisfy the demand for high-quality medicinal materials. This study addresses the insufficient supply and guides the cultivation of Daodi goji berry.
Chinese medicinal materials (CMMs) are the foundation of traditional Chinese medicine (TCM) for disease prevention and treatment, and their quality is closely related to the safety and efficacy of TCM (Wang et al., 2015; Lu et al., 2022). Therefore, more than 60% of CMMs included in the Chinese Pharmacopoeia (2020 edition) must be capable of detecting chemical component concentrations (Xu et al., 2021). However, CMMs have multitudinous pharmacology components that play considerable roles in physiological regulation through synergistic, antagonistic, or unilateral effects. CMMs cannot rely on several mandatory detected components to assess their quality due to the limitations of science and technology (Gao et al., 2011; Wu et al., 2018). Evaluating the comprehensive quality of CMMs based on clinical efficacy has long been a major scientific issue for TCM (Fang et al., 2022). In ancient China, based on the abundant clinical efficacy of CMMs from different regions for long-term practice, TCM practitioners gradually proposed the concept of Daodi CMMs (also known as Didao, authentic, genuine, geoauthentic medicinal materials, etc.) (Zhao et al., 2012; Yang et al., 2018). According to the Law of the People’s Republic of China on TCM implemented in 2017, Daodi CMMs refer to those selected through long-term clinical applications of TCM and produced in specific geographic regions, with better quality and efficacy than others. Thus, Daodi CMMs are widely recognized and have enjoyed a good reputation in the TCM industry for centuries.
TCM plays an ever-increasing role in modern medicine and healthcare, gradually gaining recognition worldwide (Gao et al., 2011). The cultivation area of CMMs has increased enormously with the increasing demand for TCM services. In 2020, the total planting area of CMMs exceeded 56,000 km2 in China (Wang et al., 2022a). However, the synthesis of plant bioactive constituents (mainly secondary metabolites) is influenced by external environmental conditions, such as climate and soil (Ncube et al., 2012; Lei et al., 2018). Different regional environments lead to an uneven quality of CMMs (Liu et al., 2020). In recent years, the Chinese government has issued several documents advocating for enterprises to use Daodi CMMs to ensure the quality of CMMs and the efficacy of TCMs (Standing Committee of the National People's Congress of China, 2019; National Medical Products Administration of China, 2020; National Medical Products Administration of China et al., 2022). However, the supply of CMMs in the Daodi regions is limited, making it challenging to meet the considerable demands of the market. Meanwhile, the Chinese government released a guideline on preventing nongrain use of arable land and stabilizing grain production in 2020 (General Office of the State Council of China, 2020). Such a policy severely restricted the cultivation of CMMs. In 2022, the cultivation area of CMMs decreased by 6.82% compared with 2021 (Insight and Info, 2022), leading to a decrease in the supply of Daodi CMMs.
Even worse, the surface temperature on Earth has increased by 0.85°C over the past 130 years and is predicted to rise continuously due to increased greenhouse gas emissions (Stocker, 2013). The climate has critically impacts on plant growth, development, and distribution. Many plants are expected to shift their geographical ranges for survival in response to global warming (Thuiller, 2007). Therefore, climate change may lead to the shift of CMMs’ suitable ranges. For example, the suitable area of wild jujube [Ziziphus jujuba Mill. var. spinosa (Bunge) Hu ex H. F. Chou] in the future was found to increase except for SSP1-2.6 and SSP5-8.5 in the 2070s, and SSP5-8.5 in the 2090s, and the centroids of its suitable region shifted to the northwest (Zhao et al., 2022a). The suitable habitats of shiny-leaf prickly-ash [Zanthoxylum nitidum (Roxb.) DC.] in the 2050s and 2070s were predicted to gradually reduce southward under different greenhouse gas emission modes (Yang et al., 2023). The formation of high-quality Daodi CMMs is closely related to their growing environments (Zhao et al., 2012; Yang et al., 2018; Liu et al., 2020). Therefore, drastic climate change is likely to result in a shift in the location of Daodi regions. It has been proved that the Daodi region of oriental water plantain [Alisma orientalis (Sam.) Juzep.] has shifted from Hanzhong, Shaanxi Province, in the Song Dynasty to Jianning, Fujian Province, in the Qing Dynasty due to the cooling climate (Peng et al., 2013). Under climate warming, the original Daodi region may no longer be suitable for the distribution of CMMs. However, few studies have focused on the influence of global warming on changes in Daodi regions. Understanding the impact of climate change on the distribution of Daodi CMMs is of great significance to their cultivation and conservation.
The goji berry (Lycium barbarum L.) is a significant medicinal and edible herb with the efficacy of nourishing the liver and kidney, benefiting essence, and improving eyesight (Figure 1) (Ma et al., 2022; Vidović et al., 2022). The annual demand for the herb exceeded 300,000 tons, and the cultivation area covered over 1,200 km2 (Wang et al., 2019a; Ma et al., 2021). These numbers made this species one of the most consumed and cultivated CMMs in China. In recent years, the cultivation area of goji berry in the Daodi region (Zhongning County and its surrounding areas) has decreased to less than 10% of that of China due to increased production costs and the policy of preventing the nongrain use of arable land. Thus, the production in the Daodi region is insufficient to meet market demands. Goji berries from other regions are transported to Zhongning County to disguise Daodi CMMs for sale to meet the considerable market demands (Lv, 2019). As the designated ecological environments are the limited factors influencing the formation of Daodi CMMs, leading to the quality difference between Daodi and non-Daodi CMMs (Zhao et al., 2012; Liu et al., 2020), screening similar production areas based on the environmental characteristics of the Daodi regions is of great significance in meeting the demands of food, Chinese patent medicines, formula granules, decoction pieces, etc. for high-quality goji berry.
Figure 1 (A) The production base of goji berry in the Daodi region and (B) drying processing of the Daodi goji berry.
Species distribution models (SDMs) are powerful tools for predicting the potential geographical distribution of species based on currently known species distribution records and the corresponding site characteristics, which have been widely used in biological introduction and cultivation, species protection, and invasive species prevention (Santana et al., 2019; Zhao et al., 2022a; Yang et al., 2023). Various SDMs, such as Bioclim, Climex, Domain, GARP, and MaxEnt, have been developed to predict the potential distribution of species (Srivastava et al., 2020; Li et al., 2023). The MaxEnt model stands out for its high prediction accuracy with incomplete or small sample datasets, short running time, and easy operation (Pearson et al., 2007; Ortega-Huerta and Townsend Peterson, 2008).
Although the impact of climate change on the distribution of goji berries in China has been previously predicted using the MaxEnt model (Wang et al., 2022b), its effects on Daodi goji berries were still unclear. In this study, the potentially suitable habitats of goji berries under current and future climate scenarios were predicted based on the environmental characteristics of the Daodi region using the optimized MaxEnt model. The major environmental variables influencing its distribution were identified, and the changes in its distribution were analyzed. Our study will hopefully provide guidance for scientific cultivation and contribute to solving the supply shortage of high-quality goji berries.
According to the standard for Daodi medicinal materials, issued by the Chinese Society of TCM (CACM) in 2019, the Daodi region of goji berry is located in Zhongning County and its surrounding areas (Huang et al., 2020). From 2019 to 2021, the distribution of goji berry cultivation bases in Zhongning County and its adjacent surrounding counties, including Shapotou District and Haiyuan County of Zhongwei prefecture-level city, and Tongxin County, Hongsipu District, Litong District and Qingtongxia of Wuzhong prefecture-level city was collected through field investigation. Finally, the distributional information of 55 goji berry cultivation bases in the Daodi region was collected (Figure 2).
Figure 2 Distribution information of goji berry planting bases in the Daodi region of Ningxia Province.
The data on soil were obtained from the World Soil Database (Harmonized World Soil Database v1.2, http://www.fao.org/soils-portal/), and converted to the ASCII format using ArcGIS v10.8 (https://www.esri.com/zh-cn/arcgis/). The dataset contained 18 soil variables. Due to the lack of data on future soil layers, we assumed that future soil layers were consistent with the current ones over these short time frames (Zhang et al., 2020a; Huang et al., 2022). The current (averages for 1960 – 1990) and the future 2050s (averages for 2041 – 2060) and 2070s (averages for 2061 – 2080) climate data were obtained from the World Climate Database (WorldClim v1.4, 2.5-minute resolution, https://www.worldclim.org/) and converted to ASCII format using ArcGIS. The climatic dataset contained 19 climate variables. Future climate data were determined based on the Community Climate System Model v4.0 (CCSM 4.0). The mode had four greenhouse gas emission scenarios (RCP 2.6, 4.5, 6.0, and 8.5) from the 5th Emission Report of the Intergovernmental Panel on Climate Change (IPCC) (Moss et al., 2010). Thirty-six environmental variables (17 soil and 19 climate variables) were initially used to construct the MaxEnt model.
The maps [inspection number: GS Jing (2022) 1061] of the Ningxia Hui Autonomous Region, Gansu Province, Inner Mongolia Autonomous Region, and China were downloaded from the Alibaba Cloud Data Visualization Platform (DataV. GeoAtlas, http://datav.aliyun.com/portal/school/atlas/areaselector/) and converted to shapefiles using Mapshapper (https://mapshaper.org/).
The spatial analysis function of ArcGIS was used to estimate the distance between the distribution points to reduce the effect of spatial autocorrelation and improve prediction accuracy. Consequently, duplicate points were deleted, and only one point was maintained when the straight-line distance between two points was less than 10 km.
The environmental variables were examined for cross-correlation to avoid multicollinearity and improve the MaxEnt model’s accuracy (Jarnevich et al., 2015). First, Pearson’s correlation between the current environmental variables was analyzed using the correlation function of ENMTools v1.3 (https://github.com/danlwarren/ENMTools/). Second, the environmental variable data and the distribution data of goji berry were imported into MaxEnt v3.4.4 (https://biodiversityinformatics.amnh.org/opensource/maxent/) with 25% of the distribution points in the test dataset and the remaining 75% in the training dataset. A jackknife analysis was performed to measure variable importance. The logistic format and a replicate run type bootstrap were also selected as MaxEnt model features. The model runs were repeated 10 times. The environmental variables with zero contribution were removed. Variables with an absolute value of their correlation coefficient greater than 0.8 were retained only when they reached the highest contribution in the jackknife analysis (Li et al., 2022; Zhao et al., 2022b).
The regularization multiplier (RM) and feature classes (FCs) are the key parameters affecting the prediction accuracy of the MaxEnt model (Muscarella et al., 2014). The default settings in MaxEnt produce overfitting results and are typically not optimal. We used the Kuenm package (https://github.com/marlonecobos/kuenm/) in R v3.6.3 (https://www.r-project.org/) to calibrate RM and FCs for the optimal MaxEnt (Cobos et al., 2019). The RM parameter was set to 0.1 – 4.0 at an interval of 0.1. The MaxEnt model included five FCs [linear (L), quadratic (Q), hinge (H), product (P), and threshold (T)] and 31 possible FCs combinations. The Kuenm package was applied to evaluate the 1,240 (40 RMs × 31 FCs) candidate modes. The output file (selected_models.csv) was used to select the optimal candidate modes. The best models were selected under current climate conditions according to the following criteria: (1) significant models with omission rates ≤ 5%, and (2) the lowest delta-corrected Akaike information criterion (AICc) of ≤ 2% (Cobos et al., 2019).
The other MaxEnt parameters, which included “Create responsive curves,” “Do jackknife to measure variable importance,” “Out format logistic,” “Random seed,” “Replicated run type bootstrap,” “Add all samples to the background,” and “Write background predictions” were selected for simulation and prediction (Zhao et al., 2022b). The settings for the remaining parameters were default (Zhang et al., 2018a; Wang et al., 2021; Li et al., 2022). The screening data for environmental variables and distribution points were imported into MaxEnt, with 25% of the distribution points used for model testing and the remaining 75% for model training. The model runs repeated 10 times.
The accuracy of the model prediction results was evaluated using the receiver operation characteristic (ROC) area under the curve (AUC) and true skill statistic (TSS) under current climate conditions. The closer the AUC and TSS values were close to 1, indicating a high prediction accuracy (Hou et al., 2023). The model prediction performance was deemed when the AUC and TSS values were greater than 0.9, and 0.75, respectively (Allouche et al., 2006; Franco et al., 2022).
The ASCII files output by MaxEnt were reclassified and visualized using ArcGIS. The maximum training sensitivity plus specificity logistic threshold (MTSPS) output by MaxEnt under current climatic conditions was employed to classify these ASCII files into unsuitable and suitable for the Daodi goji berry. This threshold is considered simple and effective in determining modeled species’ presence/absence maps (Huang et al., 2022). Areas with suitability values greater than MTSPS were considered suitable for the species (Dai et al., 2023). The distribution areas were divided into unsuitable habitat (0 – MTSPS), lowly suitable habitat (MTSPS – 0.6), moderately suitable habitat (0.6 – 0.7), and highly suitable habitat (0.7 – 1.0) according to the suitable probability using the ArcGIS reclassification function.
The number of grids in different suitable habitats was counted using ArcGIS. The area of each grid was calculated as follows:
The area of different suitable habitats was calculated as follows:
The ASCII files output by MaxEnt were imported into ArcGIS. Then, SDMtoolbox v2.0 (http://www.sdmtoolbox.org/) was employed to convert these ASCII files to binary files (0 unsuitable habitat, 1 suitable habitat) with the threshold value of 0.6. The spatiotemporal changes in the 2050s and 2070s compared with the current and the centroid changes of the moderately and highly suitable habitats under different climate scenarios were analyzed using SDMtoolbox. The spatiotemporal changes in the area were calculated using the method mentioned in Section 2.2.4.
The binary files mentioned in Section 2.2.5 were imported into ArcGIS. Then, the plus function in Spatial Analyst Tools was applied to determine the overlap of moderately and highly suitable habitats under current and future climate scenarios. The suitable overlapping area was calculated using the method mentioned in Section 2.2.4.
The correlation of the environmental variables was shown in Figure 3. After the screening, 26 of 55 distribution points and 14 (five climate variables and nine soil variables) of 36 environmental variables (Table 1) were selected for MaxEnt analysis.
Table 1 Percent contribution and permutation importance of the screening environmental variables under current climate conditions.
Based on the screening distribution points and environmental variables, the potential distribution of Daodi goji berry was simulated and predicted by the MaxEnt model. When we selected default parameters for the model, the delta AICc was 54.85. Based on the results output by the Kuenm package, when RM and FC were separately set to 2.1 and LQPH, delta AICc was observed to be 0. Therefore, RM = 2.1 and FC = LQPH were the optimal combination for MaxEnt modeling (Supplementary Table S1). The 10-repeated average training AUC (Supplementary Figure S1) and the average TSS were 0.999 and 0.945, respectively, indicating that the reconstructed model was highly reliable and could effectively predict the Daodi goji berry suitable habitats.
The dominant environmental variables influencing the distribution of Daodi goji berry under current climate conditions were determined through their contribution rates and the jackknife of regularized training gain (Sun et al., 2012). The variables with the highest contribution rate to the model were bio11 (33.4%) and bio19 (29.5%), with a cumulative contribution rate of 62.9% and permutation importance of 87.8% (Table 1). The results of the jackknife test of variable importance showed that bio11 and bio19, with the highest training gain, were the two critical variables shaping the distribution of the Daodi goji berry (Supplementary Figure S2).
Based on a comprehensive analysis of the contribution rates and the regularized training gain, the dominant variables influencing the distribution of Daodi goji berry were bio11 and bio19. The relationship between the distribution probability and the dominant environmental variables was studied based on the species response curves under current climate conditions. When the potential distribution probability was greater than 0.5473 (MTSPS), the mean temperature (bio11) and precipitation (bio19) of the coldest quarter were from −6.40°C to −2.57°C and less than 13.97 mm, respectively (Supplementary Figure S3).
Under current climate conditions, the actual distribution of goji berry production bases in the Daodi region highly matched the MaxEnt prediction results. 92.31% of the screening distribution points were located in suitable habitats, of which 76.92% were in moderately and highly suitable habitats.
The habitats suitable for the growth of Daodi goji berry were 45,973.88 km2, accounting for 0.48% of China’s land area, which were concentrated in central and western Ningxia province and central Gansu province (Figures 4, 5). The suitable habitats in Ningxia were the largest, accounting for 49.14% of those in China, followed by Gansu (40.86%) and Inner Mongolia (3.27%) (Figure 5).
In Ningxia province, the suitable habitats were mainly distributed in Zhongwei (9,160.21 km2) and Wuzhong (9,004.66 km2) prefecture-level cities, where Daodi goji berry was located, accounting for 80.41% of those in Ningxia, followed by Yinchuan (4,338.14 km2) (Figure 4). In Gansu province, the suitable habitats were mainly located in Baiyin (10,439.18 km2) and Lanzhou (6,135.61 km2) prefecture-level cities, accounting for 88.22% of those in Gansu. The region with the widest highly suitable habitats was Baiyin (3,785.07 km2), followed by Wuzhong (3,508.53 km2), Zhongwei (3,214.71 km2), Lanzhou (1,762.91 km2), and Yinchuan (380.24 km2) (Figure 4).
Under different future climate scenarios, the area of potentially suitable habitats was higher than that under current climate conditions (Figure 5). Additionally, these habitats were mainly distributed in Ningxia, Gansu, and Inner Mongolia provinces (Figure 6; Supplementary Figure S4). In the 2050s, the area of suitable habitats under RCP 2.6, 4.5, and 6.0 climate scenarios expanded with the increased greenhouse gas emissions, except RCP 8.5. Among the four climate scenarios in the 2050s, the suitable area under RCP 6.0 reached the maximum (91,256.42 km2), with an increase of 98.50% compared with the current. However, the changes in suitable area in the 2070s were opposite to those in the 2050s. In the 2070s, the suitable area decreased gradually under RCP 2.6, 4.5, and 6.0 but reached the maximum (82,459.17 km2) under RCP 8.5. The highly suitable area under different future climate scenarios was higher than the current ones, mainly distributed in Ningxia and Gansu provinces, and reached the maximum under RCP 6.0 (22,416.59 km2) in the 2050s and 2.6 (21,224.03 km2) in the 2070s (Figure 5).
In Ningxia, the area of total suitable and highly suitable habitats for the distribution of Daodi goji berry under future climate scenarios was higher than the current ones, and reached the maximum under RCP 8.5 (suitability: 32,907.62 km2; highly suitability: 13,290.94 km2) in the 2070s (Figure 5). The highly suitable habitats were mainly distributed in central and western Ningxia, including Zhongwei and Wuzhong, followed by Yinchuan (Figure 6).
In Gansu, the suitable habitats’ location was consistent with the current and mainly distributed in Baiyin and Lanzhou (Figure 6). The total suitable and highly suitable area reached the maximum under RCP 6.0 (suitability: 2,607.95 km2; highly suitability: 8,814.54 km2) in the 2050s, and reached the maximum under RCP 4.5 (suitability: 2,1051.20 km2) and 2.6 (highly suitability: 9,263.91 km2) in the 2070s, respectively (Figure 5).
In Inner Mongolia, the suitable habitats under future climate scenarios expanded dramatically and increased to 6.14 – 17.18 times compared with the current (Figure 5), which mainly concentrated in the southwest Ordos prefecture-level city bordering with Ningxia, followed by Alxa (Figure 6). The area of highly suitable habitats reached a maximum of 1,590.07 km2 under RCP 8.5 in the 2070, which was 8.36 times larger than the current.
Compared with the current climate conditions, the expansion area of moderately and highly suitable habitats under future 2050s and 2070s climate scenarios was all higher than the contraction, with an increase of 0.84 – 7.31 fold (Figure 7). The expansion regions were mainly distributed in the northeast of the current moderately and highly suitable ranges, including Ordos, Wuzhong, Yinchuan, and Shizuishan prefecture-level cities (Figure 8). On the contrary, the contraction regions were mainly located in Lanzhou prefecture-level city, southwest of the current moderately and highly suitable ranges. Under different future climate scenarios, the expansion area reached the maximum (28,016.41 km2) under RCP 8.5 in the 2070s and was close to the unchanged (stable) area (28,638.62 km2) of moderately and highly suitable habitats (Figure 7).
Figure 7 Changes in the area of moderately and highly suitable habitats under different future climate scenarios compared with those under the current climate conditions.
Figure 8 Spatiotemporal changes in moderately and highly suitable habitats under different future climate scenarios compared with those under current climate conditions.
Under current climate conditions, the moderately and highly suitable habitat centroid was located in Zhongwei (104.96°E, 37.08°N), Ningxia province, bordering Baiyin, Gansu province (Figure 9). Under future climate scenarios, the distributional centroids shifted northeast but still located in Zhongwei, except for RCP 2.6 (104.77°E, 36.65°N) in the 2050s, which was projected to shift southwest into Baiyin. The distance between the centroid of RCP 8.5 (105.87°E, 37.63°N) in the 2070s and the current was 101.16 km, which was larger than the others (Figure 9).
Figure 9 Centroids of moderately and highly suitable habitats under current and future climate scenarios.
Under current and future climate scenarios, the moderately and highly suitable overlapping habitats were mainly concentrated in Ningxia (14,587.20 km2) and Gansu (9,229.34 km2) provinces, accounting for 59.77% and 37.82% of those of China, respectively (Figures 10, 11). In Ningxia, the overlapped regions were mainly distributed in Zhongwei (6,757.81 km2) and Wuzhong (5,236.87 km2) prefecture-level cities, followed by Yinchuan (2,557.94 km2). In Gansu, the overlapped regions were mainly distributed in Baiyin (7,241.75 km2) prefecture-level city, followed by Lanzhou (1,953.03 km2) (Figure 11).
In this study, MaxEnt and ArcGIS were used to predict the suitable habitats of the Daodi goji berry in China and analyze its changes under different greenhouse gas emission modes based on the environmental characteristics of the Daodi region. Our study was significant in guiding the scientific cultivation and meeting the supply of high-quality goji berry. As concentrated distribution points and cross-correlations among the environmental variables led to the overfitting of the MaxEnt model (Zhang et al., 2018a; Wang et al., 2023), we used the spatial analysis function of ArcGIS and ENMTools to screen the distribution points and environmental variables to avoid these issues. Moreover, to improve the MaxEnt prediction accuracy, the key parameters RM and FC were optimized using the Kuenm package. The AUC and TSS values of the optimized model were all larger than 0.90, and more than 90% distribution points were located in the suitable habitats, indicating that the simulation and prediction of the model were excellent and reliable. Thus, the model could be used to predict the geographical distribution of the Daodi goji berry.
The prediction results showed that the potentially suitable habitats for the Daodi goji berry were mainly distributed in central and western Ningxia province and central Gansu province bordering western Ningxia under current climate conditions, which were consistent with the known distribution of the cultivated goji berries (Xu et al., 2014). These regions were located in the upper reaches of the Yellow River with similar environmental characteristics and suitable for cultivating goji berries (Li et al., 2019a). Climate warming affects the adaptability of plants, causing the expansion of thermophilic plants and the retreat of psychrophilic plants to the Antarctic and Arctic (Parmesan and Yohe, 2003; Hegland et al., 2009; Liang et al., 2018). Our results showed that the area suitable for the Daodi goji berry under future climate scenarios was all higher than the current, and the distributional centroids mainly shifted to the northeast of China. Given that goji berries are infinite inflorescence plants, the warming climate will extend the growth period, resulting in increased yields and benefiting the accumulation of active ingredients such as polysaccharides (Li et al., 2019b; Xu et al., 2023). Thus, we believe climate warming is conductive to Daodi goji berry cultivation.
The environmental conditions are the dominant factors influencing the physiology, distribution, and phenology of plants (Xue et al., 2021; Wang et al., 2023). Therefore, climate change may result in the migration of CMMs’ Daodi region (Peng et al., 2013). We identified that the moderately and highly suitable overlapping habitats of Daodi goji berries were primarily distributed in Baiyin, Zhongwei, and Wuzhong. Under climate change, these areas consistently maintained their suitability for cultivating Daodi goji berries. Zhongning County, the focal region for cultivating Daodi goji berries, is situated at the junction of Zhongwei and Wuzhong (Huang et al., 2020). Based on our findings, we propose that climate warming will not lead to the migration of Daodi goji berry location.
Among all the environmental variables, climate, including temperature and precipitation, is the most crucial factors controlling plant regeneration and distribution (Thuiller, 2007). Our results showed that the contribution rate (83.3%) and the permutation importance (99.9%) of climate variables were much higher than the soil, indicating that climate had a much greater impact on Daodi goji berry distribution than soil. This characteristic was also found in many other plants (Hageer et al., 2017). For example, the dominant environmental variables influencing the distribution of mountain pepper Litsea cubeba (Lour.) Pers. were precipitation of the driest quarter, annual precipitation, temperature annual range, and min temperature of the coldest month instead of soil variables (Shi et al., 2023). In temperate ecosystems, winter ecological processes are important drivers of vegetation and ecosystem functioning (Kreyling, 2010). In this study, the mean temperature of the coldest quarter (bio11) was the most crucial environmental variable influencing the distribution of the Daodi goji berry, and the suitable range was −6.40°C – −2.57°C; this was closely related to the habits of the shrub, which was mainly distributed in the arid and cold regions of northwest China (Lu et al., 2020). It has been proved that goji berry was mainly distributed in the suitable range of −10°C to 10°C for the mean temperature in winter (Wang et al., 2022b). When the temperature was below -15°C, the germination of its dormant branches would decrease significantly (Qi et al., 2016).
We found that Baiyin, Gansu province, was always moderately and highly suitable for Daodi goji berries under climate change. Previous studies have shown that although the longitudinal and transverse diameters of goji berries from Baiyin were larger than those from Zhongning County, there were no significant differences in the concentrations of polysaccharides, betaine, carotenoids, flavonoids, total phenols, trace elements, etc. (Lu et al., 2019; Wang et al., 2019a; Wang, 2020). Moreover, Li et al. (2019a) showed that the quality (polysaccharides, protein, vitamins, and naringin) regionalization of goji berry produced in Baiyin was highly consistent with that in Zhongning. Therefore, we suggest that goji berry produced in Baiyin could be used to relieve the insufficient supply of Daodi CMMs. According to the concept of Daodi CMMs in the Law of the People’s Republic of China on TCM, the production areas of Daodi CMMs (Daodi regions) were selected through long-term clinical practice and remained relatively stable. Therefore, we suggest that this region should be included when demarcating the boundary of the Daodi region of goji berry in the future.
In recent years, the cultivation area of goji berry in Qinghai Province has been constantly expanding, primarily concentrated in the Qaidam Basin of China’s Qinghai-Tibet Plateau. As a result, Qinghai has emerged as the largest cultivation province in China, capturing a market share of 38.94% (General Office of the People's Government of Qinghai Province, 2022), and plays a crucial role in the development of goji berry industry. For the regional unique environmental characteristics, the size and yield of Qaidam Basin-produced goji berries are 1.5 – 2.0 times larger than those from the Daodi region, with lower harvesting costs (Wang, 2020; Wang et al., 2020). Our prediction results demonstrated that the Qaidam Basin was unsuitable for Daodi goji berry distribution. This did not mean that the efficacy of Qaidam Basin-produced goji berries was lower than those from the Daodi region. Wang et al. (2020) found that the contents of metabolites, such as organic acids, alkaloids, and polyphenols, in Qaidam Basin-produced goji berry were lower than Daodi region, while the contents of lipids and amino acids were higher. However, other research results demonstrated that there was no significant difference in the contents of carotenoids, total phenols, rutin, and total polysaccharides between the two regions (Lu et al., 2019; Yossa Nzeuwa et al., 2019). Variations in sample collection sites, harvest periods, and varieties might cause such differences. Nonetheless, it is widely acknowledged that Qaidam Basin-produced goji berries are more suitable for fruit consumption compared to those from the Daodi region, owing to their larger size, higher monosaccharides, and lower pesticide residue (Wang et al., 2019a; Wang et al., 2020). Due to the complexity and diversity of the chemical components of CMMs, different components have synergistic, antagonistic, or neutral effects on each other. Therefore, it is not scientifically reasonable to assess the quality of CCMs according to the content of some active components (Liu et al., 2016; Zhang et al., 2018b; Zhang et al., 2020b). Evaluating the quality of goji berries from different regions based on clinical efficacy is significant for guiding scientific cultivation and differential development.
In this study, the current and future potential habitats suitable for goji berry were screened based on the environmental characteristics of the Daodi region for the first time, which was critical in addressing supply shortage and guiding the cultivation of Daodi goji berry. However, different cultivation managements are used in other regions, such as the kinds and doses of fertilizers, which may also cause changes in the synthesis of metabolites (Chung et al., 2010; Shi et al., 2019). Therefore, it is necessary to widely collect goji berries from the Daodi region and its quasi-regions, and conduct a comprehensive and comparative evaluation of their quality based on massive active components and clinical efficacy.
Searching for potential regions suitable for cultivating Daodi goji berry is significant in meeting the market demands for high-quality medicinal materials. These regions were predicted based on the selected environmental characteristics of the Daodi region using the optimized MaxEnt model. Our results indicated that the prediction of the optimized model was highly accurate, and the most important variable affecting the distribution of Daodi goji berries was the mean temperature of the coldest quarter. The suitable habitats were mainly distributed in central and western Ningxia and central Gansu at present and would be expanded to the northest of the current ranges under future climate scenarios. The moderately and highly suitable overlapping habitats were mainly located in Baiyin, Zhongwei, and Wuzhong under climate change. Baiyin, adjacent to the Daodi region, was always suitable for Daodi goji berry distribution at present and in the future. We suggest that climate warming is beneficial to the cultivation of Daodi goji berries but has little influence on the Daodi region’s location, and the goji berries produced in Baiyin could be used as Daodi medicinal materials to meet market demands.
The original contributions presented in the study are included in the article/Supplementary Material. Further inquiries can be directed to the corresponding author.
JL: Conceptualization, Formal analysis, Funding acquisition, Investigation, Methodology, Software, Writing – original draft. CD: Conceptualization, Formal analysis, Methodology, Supervision, Writing – review & editing. GD: Investigation, Resources, Writing – review & editing. ZW: Investigation, Resources, Writing – review & editing. YZ: Investigation, Resources, Writing – review & editing. GF: Funding acquisition, Software, Supervision, Writing – review & editing, Project administration.
The author(s) declare financial support was received for the research, authorship, and/or publication of this article. This work was funded by the Youth Research Fund of Qinghai University (No. 2022-QNY-7), Innovation Fund of Qinghai Academy of Agricultural and Forestry Sciences (No. 2023-NKY-03), and Qinghai Provincial Major Science and Technology Special Project (No. 2023-NK-A4).
Author YZ is employed by the company Qinghai Kunlun Goji Industry Technology Innovation Research Co., Ltd.
The remaining authors declare that the research was conducted in the absence of any commercial or financial relationships that could be construed as a potential conflict of interest.
All claims expressed in this article are solely those of the authors and do not necessarily represent those of their affiliated organizations, or those of the publisher, the editors and the reviewers. Any product that may be evaluated in this article, or claim that may be made by its manufacturer, is not guaranteed or endorsed by the publisher.
The Supplementary Material for this article can be found online at: https://www.frontiersin.org/articles/10.3389/fpls.2023.1279019/full#supplementary-material
Allouche, O., Tsoar, A., Kadmon, R. (2006). Assessing the accuracy of species distribution models: prevalence, kappa and the true skill statistic (TSS). J. Appl. Ecol. 43, 1223–1232. doi: 10.1111/j.1365-2664.2006.01214.x
Chung, R., Chen, C., Ng, L. (2010). Nitrogen fertilization affects the growth performance, betaine and polysaccharide concentrations of Lycium barbarum. Ind. Crop Prod. 32 (3), 650–655. doi: 10.1016/j.indcrop.2010.07.021
Cobos, M. E., Peterson, A. T., Barve, N., Osorio-Olvera, L. (2019). Kuenm: an R package for detailed development of ecological niche models using MaxEnt. PeerJ 7, e6281. doi: 10.7717/peerj.6281
Dai, Y., Huang, H., Qing, Y., Li, J., Li, D. (2023). Ecological response of an umbrella species to changing climate and land use: habitat conservation for Asiatic black bear in the Sichuan-Chongqing region, southwestern China. Ecol. Evol. 13, 10222. doi: 10.1002/ece3.10222
Fang, Z., Wang, F., Han, L., Li, G., Wen, C., Han, J., et al. (2022). Application of "major scientific issues and engineering technology difficulties in traditional Chinese medicine (2019-2021)" in national science and technology layout. Chin. J. Chin. Mater. Med. 5, 1137–1144. doi: 10.19540/j.cnki.cjcmm.20221108.601
Franco, A. C. S., Lorini, M. L., Minsky, E. M. C., Souza Lima Figueiredo, M., Santos, L. N. (2022). Far beyond the amazon: global distribution, environmental suitability, and invasive potential of the two most introduced peacock bass. Biol. Invasions 24, 2851–2872. doi: 10.1007/s10530-022-02814-6
Gao, H., Wang, Z., Li, Y., Qian, Z. (2011). Overview of the quality standard research of traditional Chinese medicine. Front. Med. 5, 195–202. doi: 10.1007/s11684-011-0134-x
General Office of the People's Government of Qinghai Province. (2022). The 14th five year plan for the development of goji berry industry in Qinghai Province. Available at: http://wap.qinghai.gov.cn/zwgk/xxgkml/zfwj/202301/t20230116_191612.html/.
General Office of the State Council of China. (2020). Opinions on preventing " non-grain production" of cultivated land and stabilizing grain production. Available at: http://english.scio.gov.cn/Chinavoices/2020-11/18/content_76921952.htm.
Hageer, Y., Esperón-Rodríguez, M., Baumgartner, J. B., Beaumont, L. J. (2017). Climate, soil or both? Which variables are better predictors of the distributions of Australian shrub species? PeerJ 5, e3446. doi: 10.7717/peerj.3446
Hegland, S. J., Nielsen, A., Lázaro, A., Bjerknes, A., Totland, Ø. (2009). How does climate warming affect plant-pollinator interactions? Ecol. Lett. 12 (2), 184–195. doi: 10.1111/j.1461-0248.2008.01269.x
Hou, J., Xiang, J., Li, D., Liu, X. (2023). Prediction of potential suitable distribution areas of Quasipaa spinosa in China based on MaxEnt optimization model. Biology 12, 366. doi: 10.3390/biology12030366
Huang, R., Du, H., Wen, Y., Zhang, C., Zhang, M., Lu, H., et al. (2022). Predicting the distribution of suitable habitat of the poisonous weed Astragalus variabilis in China under current and future climate conditions. Front. Plant Sci. 13. doi: 10.3389/fpls.2022.921310
Huang, L., Guo, L., Zhan, Z. (2020). Compilation of Daodi medicinal materials standards (Beijing, China: Beijing Science and Technology Press), 406–411.
Insight and Info. (2022). Research report on the current situation and development prospects of the cultivation of traditional Chinese medicine materials, (2022-2029). Available at: https://www.Chinabaogao.com/baogao/202202/575077.html/.
Jarnevich, C. S., Stohlgren, T. J., Kumar, S., Morisette, J. T., Holcombe, T. R. (2015). Caveats for correlative species distribution modeling. Ecol. Inform. 29, 6–15. doi: 10.1016/j.ecoinf.2015.06.007
Kreyling, J. (2010). Winter climate change: a critical factor for temperate vegetation performance. Ecology 91, 1939–1948. doi: 10.1890/09-1160.1
Lei, D., Wu, J., Leon, C., Huang, L., Hawkins, J. A. (2018). Medicinal plants of Chinese Pharmacopoeia and Daodi: insights from phylogeny and biogeography. Chin. Herb. Med. 10, 269–278. doi: 10.1016/j.chmed.2018.06.004
Li, X., Li, D., Duan, X., Liu, Y. (2019b). Variations and effects of climate in growth period of Lycium barbarum L. in Ningxia. Chin. J. Eco-Agric. 27, 1789–1798. doi: 10.13930/j.cnki.cjea.190477
Li, Y., Shao, W., Jiang, J. (2022). Predicting the potential global distribution of Sapindus mukorossi under climate change based on MaxEnt modelling. Environ. Sci. pollut. Res. 29 (15), 21751–21768. doi: 10.1007/s11356-021-17294-9
Li, T., Yang, Y., Wang, Y., Xu, S., Fan, X., Xia, Y., et al. (2023). Predicting potential habitat of Aconitum carmichaeli Debeaux in China based on three species distribution models. Pol. J. Environ. Stud. 32 (2), 1219–1230. doi: 10.15244/pjoes/156358
Li, J., Yu, Y., Guo, L., Huang, L., Zhang, X., Yang, J. (2019a). Study on quality regionalization of Lycii Fructus. Chin. J. Chin. Mater. Med. 44 (6), 1156–1163. doi: 10.19540/j.cnki.cjcmm.2019.0023
Liang, Q., Xu, X., Mao, K., Wang, M., Wang, K., Xi, Z., et al. (2018). Shifts in plant distributions in response to climate warming in a biodiversity hotspot, the Hengduan Mountains. J. Biogeogr. 45 (6), 1334–1344. doi: 10.1111/jbi.13229
Liu, C., Chen, S., Xiao, X., Zhang, T., Hou, W., Miao, M. (2016). A new concept on quality marker of Chinese materia medica: quality control for Chinese medicinal products. Chin. Tradit. Herbal. Drugs 47 (9), 1443–1457. doi: 10.7501/j.issn.0253-2670.2016.09.001
Liu, X., Zhang, Y., Wu, M., Ma, Z., Huang, Z., Tian, F., et al. (2020). The scientific elucidation of Daodi medicinal materials. Chin. Med. 15, 86. doi: 10.1186/s13020-020-00367-1
Lu, Y., Guo, S., Yan, H., Zhang, F., Wang, H., Jin, L., et al. (2020). Regionalization of production of medicinal and edible fruit of Lycium barbarum associated with ecological factors and chemical constituents. Acta Pharm. Sin. 55 (10), 2466–2477. doi: 10.16438/j.0513-4870.2020-0572
Lu, Y., Guo, S., Zhang, F., Yan, H., Qian, D., Wang, H., et al. (2019). Comparison of functional components and antioxidant activity of Lycium barbarum L. fruits from different regions in China. Molecules. 24 (12), 2228. doi: 10.3390/molecules24122228
Lu, X., Jin, Y., Wang, Y., Chen, Y., Fan, X. (2022). Multimodal integrated strategy for the discovery and identification of quality markers in traditional Chinese medicine. J. Pharm. Anal. 12 (5), 701–710. doi: 10.1016/j.jpha.2022.05.001
Lv, W. (2019). Daodi study of Lycii Fructus based on liquid chromatography mass spectrometry. North Minzu University. Yinchuan China. 20. doi: 10.27754/d.cnki.gbfmz.2019.000016
Ma, R., Zhang, X., Ni, Z., Thakur, K., Wang, W., Yan, Y., et al. (2022). Lycium barbarum (Goji) as functional food: a review of its nutrition, phytochemical structure, biological features, and food industry prospects. Crit. Rev. Food Sci. Nutr. 63 (30), 10621–10635. doi: 10.1080/10408398.2022.2078788
Ma, P., Zhu, R., Bai, C., Yu, J. (2021). Investigation on the resources and industrial development of Lycium barbarum. Chin. Tradit. Patent Med. 43 (11), 3245–3248. doi: 10.3969/j.issn.1001-1528.2021.11.061
Moss, R. H., Edmonds, J. A., Hibbard, K. A., Manning, M. R., Rose, S. K., van Vuuren, D. P., et al. (2010). The next generation of scenarios for climate change research and assessment. Nature. 463 (7282), 747–756. doi: 10.1038/nature08823
Muscarella, R., Galante, P. J., Soley Guardia, M., Boria, R. A., Kass, J. M., Uriarte, M., et al. (2014). Enmeval: an R package for conducting spatially independent evaluations and estimating optimal model complexity for maxent ecological niche models. Methods Ecol. Evol. 5, 1198–1205. doi: 10.1111/2041-210X.12261
National Medical Products Adminstration, Ministry of Agriculture and Rural Affairs, National Forestry and Grassland Adminstration, National Adminstration of Traditional Chinese Medicine of China. (2022). Good Agricultural Practice of traditional Chinese medicinal materials. Available at: https://www.nmpa.gov.cn/xxgk/fgwj/xzhgfxwj/20220317110344133.html/ (Accessed 4 July 2023).
National Medical Products Adminstration of China. (2020). Technical guidelines for research on quality control of new medicinal materials of traditional Chinese medicine (Trial). Available at: https://www.nmpa.gov.cn/xxgk/ggtg/ypggtg/ypqtggtg/20201016144518188.html/ (Accessed 29 November 2023).
Ncube, B., Finnie, J. F., Van Staden, J. (2012). Quality from the field: the impact of environmental factors as quality determinants in medicinal plants. S. Afr. J. Bot. 82, 11–20. doi: 10.1016/j.sajb.2012.05.009
Ortega-Huerta, M. A., Townsend Peterson, A. (2008). Modeling ecological niches and predicting geographic distributions: a test of six presence-only methods. Rev. Mex. Biodivers. 79, 205–216.
Parmesan, C., Yohe, G. (2003). A globally coherent fingerprint of climate change impacts across natural systems. Nature 421, 37–42. doi: 10.1038/nature01286
Pearson, R. G., Raxworthy, C. J., Nakamura, M., Townsend Peterson, A. (2007). Predicting species distributions from small numbers of occurrence records: a test case using cryptic geckos in Madagascar. J. Biogeogr. 34, 102–117. doi: 10.1111/j.1365-2699.2006.01594.x
Peng, H., Hao, J., Huang, L. (2013). Effect of climate change on genuine medicinal materials producing areas during last 2 000 years—Alisma orientale and Citrus aurtantium as examples. Chin. J. Chin. Mater. Med. 38 (13), 2218–2222. doi: 10.4268/cjcmm20131337
Qi, Y., Geng, W., Zhou, W., Wang, J., Wang, Q., Wang, W., et al. (2016). Study on the cold resistance of two Chinese wolfberry species. Xinjiang Agr. Sci. 53, 2203–2209. doi: 10.6048/j.issn.1001-4330.2016.12.006
Santana, P. A., Kumar, L., Da Silva, R. S., Pereira, J. L., Picanço, M. C. (2019). Assessing the impact of climate change on the worldwide distribution of Dalbulus maidis (DeLong) using MaxEnt. Pest Manage. Sci. 75 (10), 2706–2715. doi: 10.1002/ps.5379
Shi, X., Wang, J., Zhang, L., Chen, S., Zhao, A., Ning, X., et al. (2023). Prediction of the potentially suitable areas of Litsea cubeba in China based on future climate change using the optimized MaxEnt model. Ecol. Indic. 148, 110093. doi: 10.1016/j.ecolind.2023.110093
Shi, Z., Wei, F., Wan, R., Li, Y., Wang, Y., An, W., et al. (2019). Impact of nitrogen fertilizer levels on metabolite profiling of the Lycium barbarum L. fruit. Molecules. 24 (21), 3879. doi: 10.3390/molecules24213879
Srivastava, V., Griess, V. C., Keena, M. A. (2020). Assessing the potential distribution of Asian gypsy moth in Canada: a comparison of two methodological approaches. Sci. Rep. 10, 22. doi: 10.1038/s41598-019-57020-7
Standing Committee of the National People's Congress of China (2019) Pharmaceutical Administration law of China. Available at: https://www.nmpa.gov.cn/xxgk/fgwj/flxzhfg/20190827083801685.html/ (Accessed 4 July 2023).
Stocker, T. F. (2013). The closing door of climate targets. Science 339 (6117), 280–282. doi: 10.1126/science.1232468
Sun, J., Zhou, G., Sui, X. (2012). Climatic suitability of the distribution of the winter wheat cultivation zone in China. Eur. J. Agron. 43, 77–86. doi: 10.1016/j.eja.2012.05.009
Thuiller, W. (2007). Biodiversity: climate change and the ecologist. Nature 448, 550–552. doi: 10.1038/448550a
Vidović, B. B., Milinčić, D. D., Marčetić, M. D., Djuriš, J. D., Ilić, T. D., Kostić, A.Ž., et al. (2022). Health benefits and applications of goji berries in functional food products development: a review. Antioxidants 11 (2), 248. doi: 10.3390/antiox11020248
Wang, Y. (2020). 'Ningqi 1' wolfberry quality response mechanism to meteorological and soil factors. Beijing Forestry University. Beijing China., 36. doi: 10.26949/d.cnki.gblyu.2020.001646
Wang, R., Jiang, C., Liu, L., Shen, Z., Yang, J., Wang, Y., et al. (2021). Prediction of the potential distribution of the predatory mite Neoseiulus californicus McGregor in China using MaxEnt. Glob. Ecol. Conserv. 29, e01733. doi: 10.1016/j.gecco.2021.e01733
Wang, L., Jiang, P., Xu, D., Wang, R., Sun, Q. (2022b). Analysis of geographic distribution patterns of Lycium barbarum in the context of climate oscillations. Acta Bot. Boreal.-Occident. Sin. 42 (12), 2133–2142. doi: 10.7606/j.issn.1000-4025.2022.12.2133
Wang, Y., Jin, H., Dong, X., Yang, S., Ma, S., Ni, J. (2019b). Quality evaluation of Lycium barbarum (wolfberry) from different regions in China based on polysaccharide structure, yield and bioactivities. Chin. Med. 14, 49. doi: 10.1186/s13020-019-0273-6
Wang, L., Kong, W., Yang, M., Han, J., Chen, S. (2015). Safety issues and new rapid detection methods in traditional Chinese medicinal materials. Acta Pharm. Sin. B 5, 38–46. doi: 10.1016/j.apsb.2014.12.005
Wang, Y., Liang, X., Guo, S., Li, Y., Zhang, B., Yin, Y., et al. (2019a). Evaluation of nutrients and related environmental factors for wolfberry (Lycium barbarum) fruits grown in the different areas of China. Biochem. Syst. Ecol. 86, 103916. doi: 10.1016/j.bse.2019.103916
Wang, Y., Liang, X., Li, Y., Fan, Y., Li, Y., Cao, Y., et al. (2020). Changes in metabolome and nutritional quality of Lycium barbarum fruits from three typical growing areas of China as revealed by widely targeted metabolomics. Metabolites 10 (2), 46. doi: 10.3390/metabo10020046
Wang, H., Zhang, X., Wang, J., Shi, T., Li, M., Wan, X., et al. (2022a). Statistical analysis of planting area of Chinese medicinal materials in China in 2020. Chin. Food Drug Admin. Mag. (1), 4–9. doi: 10.3969/j.issn.1673-5390.2022.01.001
Wang, Y., Zhao, R., Zhou, X., Zhang, X., Zhao, G., Zhang, F. (2023). Prediction of potential distribution areas and priority protected areas of Agastache rugosa based on MaxEnt model and Marxan model. Front. Plant Sci. 14, 1200796. doi: 10.3389/fpls.2023.1200796
Wu, X., Zhang, H., Fan, S., Zhang, Y., Yang, Z., Fan, S., et al. (2018). Quality markers based on biological activity: a new strategy for the quality control of traditional Chinese medicine. Phytomedicine 44, 103–108. doi: 10.1016/j.phymed.2018.01.016
Xu, C., Liu, S., Xu, R., Qiao, H., Chen, J., Jin, H., et al. (2014). Investigation of production status in major wolfberry producing areas of China and some suggestions. Chin. J. Chin. Mater. Med. 39 (11), 1979–1984. doi: 10.4268/cjcmm20141106
Xu, X., Xu, H., Shang, Y., Zhu, R., Hong, X., Song, Z., et al. (2021). Development of the general chapters of the Chinese Pharmacopoeia 2020 edition: a review. J. Pharm. Anal. 11 (4), 398–404. doi: 10.1016/j.jpha.2021.05.001
Xu, R., Yang, J., Liu, J., Yan, W., Ma, G., Ma, J. (2023). Trends and effects of agro-climatic resources and main meteorological disasters during Lycium barbarum L. growing seasons in Ningxia. Chin. J. Eco-Agric. 31 (10), 1645–1656. doi: 10.12357/cjea.20230102
Xue, Y., Cao, B., Liang, H., Yang, J., Gao, P., Mao, M., et al. (2021). Environmental shifts have important impacts on the functional traits and bioactive products of medicinal crop Cornus officinalis. Ind. Crop Prod. 162, 113304. doi: 10.1016/j.indcrop.2021.113304
Yang, Y., He, J., Liu, Y., Zeng, J., Zeng, L., He, R., et al. (2023). Assessment of Chinese suitable habitats of Zanthoxylum nitidum in different climatic conditions by MaxEnt model, HPLC, and chemometric methods. Ind. Crop Prod. 196, 116515. doi: 10.1016/j.indcrop.2023.116515
Yang, X., Tian, X., Zhou, Y., Liu, Y., Li, X., Lu, T., et al. (2018). Evidence-based study to compare Daodi traditional Chinese medicinal material and non-Daodi traditional Chinese medicinal material. Evid.-based Complement Altern. Med. 2018, 6763130. doi: 10.1155/2018/6763130
Yossa Nzeuwa, I. B., Guo, B., Zhang, T., Wang, L., Ji, Q., Xia, H., et al. (2019). Comparative metabolic profiling of Lycium fruits (Lycium barbarum and Lycium chinense) from different areas in China and from Nepal. J. Food Qual. 2019, 4396027. doi: 10.1155/2019/4396027
Zhang, T., Bai, G., Han, Y., Xu, J., Gong, S., Li, Y., et al. (2018b). The method of quality marker research and quality evaluation of traditional Chinese medicine based on drug properties and effect characteristics. Phytomedicine 44, 204–211. doi: 10.1016/j.phymed.2018.02.009
Zhang, W., Wang, S., Kang, C., Lv, C., Zhou, L., Huang, L., et al. (2020b). Pharmacodynamic material basis of traditional Chinese medicine based on biomacromolecules: a review. Plant Methods 16, 26. doi: 10.1186/s13007-020-00571-y
Zhang, L., Wei, Y., Wang, J., Zhou, Q., Liu, F., Chen, Q., et al. (2020a). The potential geographical distribution of Lycium ruthenicum Murr under different climate change scenarios. Chin. J. Appl. Environ. Biol. 26 (4), 969–978. doi: 10.19675/j.cnki.1006-687x.2019.09046
Zhang, K., Yao, L., Meng, J., Tao, J. (2018a). MaxEnt modeling for predicting the potential geographical distribution of two peony species under climate change. Sci. Total Environ. 634, 1326–1334. doi: 10.1016/j.scitotenv.2018.04.112
Zhao, Z., Guo, P., Brand, E. (2012). The formation of Daodi medicinal materials. J. Ethnopharmacol. 140 (3), 476–481. doi: 10.1016/j.jep.2012.01.048
Zhao, X., Lei, M., Wei, C., Guo, X. (2022b). Assessing the suitable regions and the key factors for three Cd-accumulating plants (Sedum alfredii, Phytolacca americana, and Hylotelephium spectabile) in China using MaxEnt model. Sci. Total Environ. 852, 158202. doi: 10.1016/j.scitotenv.2022.158202
Keywords: Lycium barbarum, Daodi, MaxEnt, potential distribution, climate change
Citation: Li J, Deng C, Duan G, Wang Z, Zhang Y and Fan G (2024) Potentially suitable habitats of Daodi goji berry in China under climate change. Front. Plant Sci. 14:1279019. doi: 10.3389/fpls.2023.1279019
Received: 17 August 2023; Accepted: 18 December 2023;
Published: 09 January 2024.
Edited by:
Lucian Copolovici, Aurel Vlaicu University of Arad, RomaniaReviewed by:
Mengfei Li, Gansu Agricultural University, ChinaCopyright © 2024 Li, Deng, Duan, Wang, Zhang and Fan. This is an open-access article distributed under the terms of the Creative Commons Attribution License (CC BY). The use, distribution or reproduction in other forums is permitted, provided the original author(s) and the copyright owner(s) are credited and that the original publication in this journal is cited, in accordance with accepted academic practice. No use, distribution or reproduction is permitted which does not comply with these terms.
*Correspondence: Guanghui Fan, Z3VhbmdodWlfZmFuX21haWxAMTYzLmNvbQ==
†These authors have contributed equally to this work and share first authorship
Disclaimer: All claims expressed in this article are solely those of the authors and do not necessarily represent those of their affiliated organizations, or those of the publisher, the editors and the reviewers. Any product that may be evaluated in this article or claim that may be made by its manufacturer is not guaranteed or endorsed by the publisher.
Research integrity at Frontiers
Learn more about the work of our research integrity team to safeguard the quality of each article we publish.