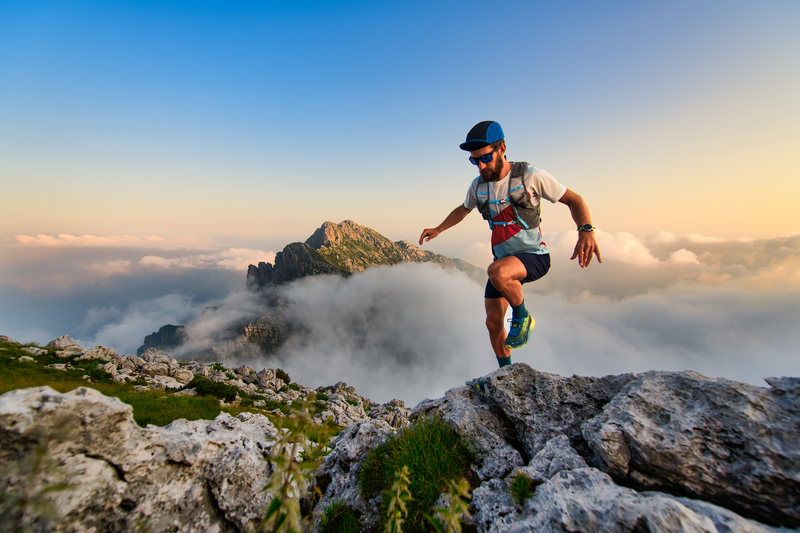
94% of researchers rate our articles as excellent or good
Learn more about the work of our research integrity team to safeguard the quality of each article we publish.
Find out more
ORIGINAL RESEARCH article
Front. Plant Sci. , 12 December 2023
Sec. Functional Plant Ecology
Volume 14 - 2023 | https://doi.org/10.3389/fpls.2023.1239190
This article is part of the Research Topic Plant Diversity: The Key to Ecosystem Resilience in a Changing World View all 10 articles
Plant functional traits serve as a bridge between plants, the environment, and ecosystem function, playing an important role in predicting the changes in ecosystem function that occur during ecological restoration. However, the response of grassland ecosystem function to plant functional traits in the context of ecological restoration in areas of karst desertification remains unclear. Therefore, in this study, we selected five plant functional traits [namely, plant height (H), specific leaf area (SLA), leaf dry matter content (LDMC), root length (RL), and root dry matter content (RDMC)], measured these along with community-weighted mean (CWM) and functional trait diversity, and combined these measures with 10 indexes related to ecosystem function in order to investigate the differences in plant functional traits and ecosystem function, as well as the relationship between plant functional traits and ecosystem functions, under four ecological restoration models [Dactylis glomerata (DG), Lolium perenne (LP), Lolium perenne + Trifolium repens (LT), and natural grassland (NG)]. We found that: 1) the Margalef index and Shannon–Wiener index were significantly lower for plant species in DG and LP than for those in NG (P<0.05), while the Simpson index was significantly higher in the former than in NG (P<0.05); 2) CWMH, CWMLDMC, and CWMRDMC were significantly higher in DG, LP, and LT than in NG, while CWMSLA was significantly lower in the former than in NG (P<0.05). The functional richness index (FRic) was significantly higher in DG and LP than in NG and LT, but the functional dispersion index (FDis) and Rao’s quadratic entropy index (RaoQ) were significantly lower in DG and LP than in NG and LT (P<0.05), and there was no significant difference between DG and LP, or between NG and LT (P>0.05); 3) ecosystem function, including ecosystem productivity, carbon storage, water conservation and soil conservation, was highest in LT and lowest in NG; and 4) CWMLDMC (F=56.7, P=0.024), CWMRL (F=28.7, P=0.024), and CWMH (F=4.5, P=0.048) were the main factors affecting ecosystem function. The results showed that the mixed pasture of perennial ryegrass and white clover was most conductive to restoration of ecosystem function. This discovery has important implications for the establishment of vegetation, optimal utilization of resources, and the sustainable development of degraded karst ecosystems.
The findings of the Millennium Ecosystem Assessment report show that, globally, approximately 60% of the services provided by nature are currently being degraded or are in an unsustainable state. Furthermore, the report reveals that 78% of the benefits that humans derive from nature are rapidly declining (MEA, 2005). The sustainability of ecosystem services has become one of the most important issues for natural resource and environmental management in recent decades (Sharafatmandrad and Mashizi, 2020; Sardar et al., 2023). Biodiversity plays a crucial role in maintaining ecosystem services and human well-being, and the relationship between biodiversity and ecosystem functioning has become a hot topic in ecological research (Cardinale et al., 2012; Gamfeldt and Roger, 2017; Plas, 2019). Biodiversity is considered to be one of the fundamental indicators of ecosystem restoration (Liu M. et al., 2022). Restoring biodiversity and ecosystem function has become the primary objective in ecological restoration (Zirbel et al., 2017; Teng et al., 2023). Not only is an understanding of the relationship between biodiversity and ecosystem function helpful in better coping with the ecological consequences of biodiversity loss under a scenario of global climate change and increasing disturbance arising from human activities, but such an understanding also has practical implications for achieving sustainable management of ecosystems, ensuring the provision of ecosystem services, and realizing the goal of ecological restoration (Hector and Bagchi, 2007; Fry et al., 2018; Gonzalez et al., 2020; Miao et al., 2023). However, the outcomes of restoring biodiversity and ecosystem function are often unpredictable, and the question of how to link changes in community composition to ecosystem function is a major challenge facing current work in ecology and environmental management (Siwicka et al., 2020; Shabaan et al., 2022). Functional trait-based approaches provide a useful framework for studying plant resource acquisition, population survival strategies, and changes in ecosystem processes and functions during restoration (McCormack et al., 2017).
The term functional traits refers to morphological, physiological, and life history traits that indirectly influence plant fitness by affecting growth, reproduction, and survival at the individual level; these traits can be divided into response traits (traits related to the response of organisms to environmental factors, such as resources and disturbances) and effect traits (traits that determine the impact of organisms on ecosystem functions) (Violle et al., 2007). Functional traits are considered to be measurable characteristics that determine the interaction between plants and their environment (Diaz and Cabido, 2001), and have been used to study plant adaptive strategies and their effects on ecosystem function (Garnier et al., 2015). Plant functional traits, which serve as physiological and ecological indicators of plant uptake, use, and maintenance of resources, reflect plant adaptation to different environments and the physiological or evolutionary trade-offs between different functions within plants; these traits are bridges between plants, environments, and ecosystem functions, and play an important role in determining ecosystem functions (Liu et al., 2021). A growing body of research-based evidence shows that plant functional traits are closely related to ecosystem function and can, to some extent, indicate changes in ecosystem function (Faucon et al., 2015; Hobbie, 2015; Roumet et al., 2016). For example, traits related to plant structure and physiology, such as specific leaf area (SLA), stem dry matter content, and leaf nutrient concentration, determine the quality and quantity of litter produced, which in turn indirectly affects leaf carbon storage and decomposition rates (Kazakou et al., 2006). There are also related studies showing that leaf traits (e.g., SLA or carbon-to-nitrogen (C:N) ratio) and root traits related to nutrient access (e.g., diameter, nutrient content, branching intensity) may exert different effects on resource access, carbon storage, and pathogen communities in the soil (Albert et al., 2012; McCormack et al., 2015). However, since there are numerous plant species in nature and various types and intensities of climate change and disturbances arising from human activity, it has become crucial to determine how to utilize plant functional traits to accurately reflect and predict changes in plant communities and ecosystem functions in the process of ecological restoration (Lei et al., 2016).
Two hypotheses can explain the relationship between plant functional traits and ecosystem function (Cadotte, 2017). One is the “mass-ratio hypothesis”, which states that the relative biomass of dominant species in the plant community and their specific traits are the dominating factors in the dynamic changes that occur in ecosystem processes (Grime, 1998); this is often characterized using the community-weighted mean (CWM) (Garnier et al., 2004; Vile et al., 2006; Díaz et al., 2007) for measures such as primary productivity (Finegan et al., 2015; Duffy et al., 2017) and soil carbon storage (Cong et al., 2014; Lange et al., 2015). The other is the “niche differentiation hypothesis”, which suggests that trait differences between species maximize the diversity of resource-use strategies, enhance ecosystem processes by reducing niche overlap, and subsequently influence ecosystem functions, which are considered to be an important component of biodiversity (Petchey and Gaston, 2002; Díaz et al., 2007). Measures of the diversity of plant functional traits, including the functional richness index, functional evenness index, and functional divergence index, are typically used to assess this hypothesis (Tilman et al., 1997). For example, Qi et al. (2022) found that species diversity, functional diversity, and biodiversity in phylogenetic space were generally positively related to productivity in their examation of the relationship between biomass and species diversity, functional diversity, and phylogenetic diversity of each community in the grasslands of the Qinghai-Tibet Plateau. Zhang et al. (2023) investigated the contribution of intraspecific variability to ecosystem function and found that a community with high interspecific variation in plant height and individuals with large leaf area could exhibit improved productivity through niche complementarity and dominance effects, respectively. Because functional diversity can better quantify the trait differences that define species interactions, is more sensitive to environmental stress or disturbance, and is more indicative of changes in ecosystem function, an increasing number of studies have used functional diversity measures to examine the relationship between plant functional traits and ecosystem function (Flynn et al., 2011; Chollet et al., 2014; Gross et al., 2017; Rosenfield and Müller, 2020). However, the mass ratio hypothesis and the niche differentiation hypothesis are not mutually exclusive; they jointly explain the construction of plant communities and ecosystem functions or processes, and have different relative importance in explaining ecosystem functions under different environmental conditions and vegetation types (Schleuter et al., 2010; Mouillot et al., 2013; Kraft et al., 2015; Jiang M. et al., 2022). It is therefore crucial to screen for plant functional traits that are associated with specific ecosystem processes, taking into account regional differences and ecosystem variation.
The karst ecosystem is a significant component of the terrestrial ecosystem, covering approximately 15% of the world’s land area (Yuan, 1991). Among these regions, the South China Karst, with Guizhou as its center, is one of the three major areas of concentrated karst distribution in the world, and it is also the main area of karst ecosystem in China. Due to the fragility of the karst ecosystem itself, following long-term, unsustainable levels of development and use by humans, the ecosystem function is damaged and a rocky, desertified landscape appears (Xiong et al., 2002). The degradation and alteration of the ecological environment due to karst desertification result in decreased stability, weakened resistance to disturbances, and reduced biodiversity in the karst ecosystem. Consequently, the sustainable development of this region has attracted much attention from scholars (Xiong et al., 2012; Canedoli et al., 2021; Yang et al., 2021; Gu et al., 2022). In order to control karst desertification, a large number of ecological restoration projects have been carried out in the karst areas of southern China. Ecological restoration is the core task of karst desertification control, and its goal is to restore biodiversity and ecosystem functions (Benayas et al., 2009; Xiong et al., 2022). Realizing the sustainable development of the karst ecological environment is an important issue for current karst desertification control (He et al., 2019; Wang K. et al., 2020; Xiao and Xiong, 2022). The use of grassland is a pioneer strategy in terms of plant community ecosystems for ecological restoration, and “grain for green” and the establishment of artificial grassland are important components of the project to restore degraded ecosystems; these approaches play an irreplaceable role in the ecological restoration process (Chi et al., 2020). The results of ecological restoration and control over many years have shown that grasslands involved in rocky desertification control have a significant positive effect in terms of the restoration of degraded soil, biodiversity, and ecosystem function (Song et al., 2022). Therefore, a comprehensive and in-depth study on the response of grassland ecosystem function to plant functional traits under different restoration models in karst desertification control areas can provide a better understanding of the degradation and restoration processes of the karst ecosystem. Additionally, such work can provide a theoretical basis for the practice of ecological restoration.
So far, although scientists have carried out extensive research on the functional characteristics of karst plants in the context of ecological restoration, they have mainly focused on adaptive strategies. For example, Zhang S. et al. (2020) conducted a statistical analysis of the relationships among leaf functional traits, plant characteristics, and environmental factors in order to explore the ecological strategies and driving factors of dominant plants in different succession stages of the ecosystem under karst desertification. Liu L. et al. (2022) comprehensively investigated four forests (three natural secondary forests and one artificial forest) in a trough-valley karst watershed in northern Guizhou Province, southwest China, to examine the community-level adaptation strategies of karst forests. Zhou et al. (2022) studied the differences in species composition and functional characteristics between dolomite and limestone karst natural forests to clarify the adaptability of vegetation to desertified karst environments. However, there are fewer studies on the relationship between plant functional traits and ecosystem function, and research on the response of grassland ecosystem function to plant functional traits in the context of ecological restoration in areas of karst desertification is also at an exploratory stage. Therefore, there is an urgent need to explore the response mechanism of grassland ecosystem function to plant functional traits in the context of ecological restoration in areas of karst desertification. On this basis, the objectives of this study were: (1) to investigate the differences in plant functional traits and ecosystem functions between natural and artificially restored grassland ecosystems; and (2) to evaluate the relationship between plant functional traits and grassland ecosystem function. We hypothesized that CWM and functional trait diversity in plant functional traits in grassland ecosystems under different vegetation restoration models would be found to contribute equally to changes in grassland ecosystem function. To address this hypothesis, we selected five indicators of plant functional traits, namely plant height (H), specific leaf area (SLA), leaf dry matter content (LDMC), root length (RL), and root dry matter content (RDMC). We assessed CWM and functional trait diversity, and combined these measures with 10 ecosystem function-related indicators to comparatively analyze the response of grassland ecosystem function to plant functional traits under four ecological restoration models [Dactylis glomerata (DG) grassland, Lolium perenne (LP) grassland, Lolium perenne + Trifolium repens (LT) grassland, and natural grassland (NG)] employed in areas of karst desertification, with the aim of providing a scientific basis for vegetation construction, resource maximization, and sustainable development of degraded karst ecosystems.
The study area is located in Salaxi Town and Yejiao Town, Qixingguan District, Bijie City, Guizhou Province, China (105°02′01′′–105°08′09′′E, 27°11′36′′–27°16′51′′N). The area of the region is 86.27 km2, and the area of rocky desertification is 55.931 km2, accounting for 64.93% of the total area. This area is a typical karst plateau mountain area with light-to-moderate rocky desertification. The study area has a subtropical humid monsoon climate, which is warm and humid in the summer and autumn, and cold and dry in the winter and spring. The average annual temperature is 12°C, the average annual rainfall is 984.40 mm, and rainfall is concentrated in the period from June to September. With a maximum elevation of 2,200 m and a minimum elevation of 1,495m, the terrain in the area varies greatly. The surface is fragmented and there are many peaks and depressions. The soil is mainly zonal yellow soil. The primary vegetation in the area has essentially been destroyed and the secondary vegetation now dominates; this includes Cyclobalanopsis glauca, Pyracantha fortuneana, Pinus massoniana, Rhododendron simsii, Juglans regia, Rosa roxburghii, Artemisia lavandulaefolia, Chenopodium glaucum, Clinopodium chinense, Plantago asiatica, Stellaria media, Digitaria sanguinalis, and Polygonum hydropiper. In order to restore the damaged karst ecosystem, based on the previous practice of the research group, artificial grassland was planted in the study area in 2012 by selecting artificial forage that would be suitable for the climatic environment of the region and would produce better ecological and economic benefits. The established forage consists mainly of Lolium perenne, Trifolium repens, Dactylis glomerata, etc. The variety of Trifolium repens is “Haifa”, the variety of Dactylis glomerata is “Qiangrass No. 4”, and the variety of Lolium perenne is “Yaqing”; the three kinds of forage seeds were provided by Guizhou Shennong Seed Industry Co., Ltd. (Guiyang, China) and Lvyi Seed Industry Co., Ltd. (Guiyang, China) (Song et al., 2022). The established artificial grassland has both single and mixed seeding. The seeding rates for Trifolium repens, Dactylis glomerata, and Lolium perenne have been found to be 2.0 g/m2, 3.0 g/m2, and 3.0g/m2, respectively. A base fertiliser (N-P2O5-K2O) was applied before planting at 0.225 g/m2. In addition, naturally restored grassland was also present. Therefore, the overall grassland ecosystem formed in the process of karst desertification control includes the natural grassland ecosystem and the artificial grassland ecosystem.
Dactylis glomerata monoculture, Lolium perenne monoculture, and a mixture of Dactylis glomerata + Trifolium repens are common establishment practices in the study area, so three grassland types with similar site conditions were selected as test plots: a Dactylis glomerata grassland plot (DG), a Lolium perenne grassland plot (LP), and a Dactylis glomerata + Trifolium repens mixed seeding grassland plot (LT). A natural grassland plot (NG) was selected as a control (Table 1). DG, LP, and LT were mowed four times a year and fertilized with N-P2O5-K2O at the time of the first noticeable rainfall after the first and the third mowings, at a rate of 0.1125 g/m2. No agricultural activities or human disturbances were carried out at NG. Six sampling plots measuring 10m × 10m were set up for each experimental plot, resulting in a total of 24 sampling plots. Due to the fragmented nature of the surface of the study area, sampling plots were spaced more than 10 meters apart. Five 1m × 1m quadrats were set up in each sampling plot for acquisition of the vegetation community and biomass. The quadrat locations within the sampling plots were chosen randomly, but the locations of each quadrat were 2 m away from each other to avoid edge effects. Vegetation survey and sampling were conducted in mid-August 2021, at the peak of biomass and species diversity, and the names of the species occurring within each quadrat and basic information on them, such as height, coverage, and density, were recorded to assess the richness and diversity of each plant community. The above-ground parts of each species in each quadrat were mowed flush with the ground in their entirety to form a mixed sample, and after removal of any dead parts (litter) adhering to the soil, stones, and other impurities, this sample was oven-dried at 75°C for 48 hours to a constant weight, to be used for calculation of the aboveground biomass.
Based on the basic characteristics of the sample plots and the results of a previous study (Brown and Anand, 2022), we selected five traits that are closely related to plant life strategies and functional trade-offs: plant height (H, cm), specific leaf area (SLA, cm2.g-1), leaf dry matter content (LDMC, g·g-1), root length (RL, cm), and root dry matter content (RDMC, g·g-1). In each quadrat, 3–5 dominant species were randomly selected for measurement of plant height. Leaf samples from healthy and mature plants were taken, in four directions (east, south, west, and north) from each plant, using scissors; approximately 12 leaves were randomly selected for measurement of leaf area; five small sample plots of leaves within each sampling plot were completely and uniformly mixed to form a mixed sample, and young leaves, old leaves, and leaves affected by diseases and insect pests were removed from the sampling process. After removal of the litter layer from the soil surface, soil and root samples were taken from the surface layer (0–20cm) using a soil auger and a root auger, respectively, at the same location. Five soil and root samples from each quadrat were mixed to form a composite sample. If the soil depth was less than 20 cm, samples were taken from the full depth. In total, 24 samples each of leaves, soil, and roots were taken back to the laboratory. The root samples were taken out of the root auger and the root was thoroughly washed; 3–5 root systems were then randomly selected for measurement of the root length with a tape measure. The collected leaves and roots were placed in water and stored in a dark environment at 5°C for 12 h. After the leaves and roots were removed from the water, the water on the surface of the leaves and roots was immediately absorbed with absorbent paper, and the saturated fresh weight of the leaves and roots was obtained by weighing them on an electronic balance at 1/10,000. The dry weight of the fully mixed leaf and root samples was measured by drying at 75°C for 48 h to constant weight; this measurement was used to calculate the dry matter content of the leaves and roots (belowground biomass), respectively. The collected soil samples were divided into two parts: one part was used for the determination of soil field capacity and soil porosity, and the other was used for the determination of soil nutrients. The samples used for the determination of soil nutrients were naturally air-dried indoors (Li et al., 2020). Oven-dried plant samples (leaves and roots) and air-dried soil samples were sieved through 2 mm sieves for the determination of plant and soil nutrient properties, respectively.
Leaf area was measured using a leaf area meter (LI-COR, 3100C Area Meter, USA). SLA is the ratio of leaf area to leaf dry weight; LDMC is the ratio of leaf dry weight to leaf saturated fresh weight; and RDMC is the ratio of root dry weight to root saturated fresh weight. Soil field capacity and soil porosity were determined by the oven-drying method. The potassium dichromate–external heating method was used to determine the organic carbon content of plants and soil; the sulfuric acid catalyst digestion–Kjeldahl method was used to determine the total nitrogen (TN) content of soil; the concentrated sulfuric acid digestion–Mo-Sb colorimetric method was used to determine the total phosphorus (TP) content of soil; and the flame photometric method was used to determine the total potassium (TK) content of soil (Zhang, 2011). TN was determined using a continuous flow analyzer (FLOWSYS, SYSTEA, Italy). TP was determined with an ultraviolet-visible spectrophotometer (Specord 200 PLUS, Analytik, Germany). Finally, TK was determined using an atomic absorption spectrometer (ICE3500, Thermo Fisher, USA).
In accordance with the MEA description of ecosystem function (MEA, 2005) and the ecological restoration goal of control of karst desertification, we selected indicators of grassland ecosystem function such as ecosystem productivity, carbon storage, water conservation, and soil conservation for analysis in this study. Ecosystem productivity was expressed as aboveground biomass and belowground biomass (Wu et al., 2017). Carbon storage was expressed as aboveground plant carbon content, soil carbon content, and root carbon content (Wu et al., 2017). Water conservation was expressed as soil field capacity and soil porosity (Fan et al., 2019). Finally, soil fertility conservation was expressed as TN, TP, and TK (Wu et al., 2017).
We used the Margalef index, the Shannon–Wiener index, the Pielou index, and the Simpson index to assess species richness and diversity in the grassland ecosystems. The Margalef index (R), Shannon–Wiener index (H), Pielou index (E), and Simpson index (C) were calculated for each ecosystem using the following formulae:
where is the total number of species in the community, is the frequency of species in the quadrat, is the number of individuals of species in the quadrat, and is the total number of individuals of all species in the quadrat.
Plant functional traits in the grassland ecosystems were analyzed on the basis of CWM and functional trait diversity. The CWM for each trait in each sample was calculated based on the trait value for the species and the relative abundance of the species or aboveground biomass as weights (Garnier et al., 2004). The calculation formula was as follows:
where is the total number of species in the community, is the aboveground biomass or relative abundance of species in the quadrat, and is the trait value of the species in the quadrat.
The functional richness index (FRic) indicates the degree of species use of the ecological space; the functional dispersion index (FDis) indicates the degree of spatial dispersion of plant traits; and Rao’s quadratic entropy index (RaoQ) integrates information on species richness and differences in functional characteristics between species (Casanoves et al., 2011). These three indices were chosen to characterize plant functional diversity in this study. They were all calculated in R using the FD package (Mammola et al., 2021).
In order to better compare grassland ecosystem functions and assess the impact of different restoration models, we use the comprehensive index of ecosystem function to evaluate this, following the research method of Kearney et al. (2019). First, the data for each ecosystem function index were standardized to fall within a range between 0.1 and 1.
where is the change value of evaluation index , is the original observation value of evaluation index , and and are the maximum observation value and minimum observation value of evaluation index , respectively.
The comprehensive index of grassland ecosystem function is calculated as the weighted sum of all transformation variables in each group. The weights are determined based on the relative contribution of each variable to the variance within the ecosystem function group using principal component analysis (PCA). The formula used for this calculation was as follows:
where is the comprehensive index of ecosystem function, is the value converted from Equations 7 or 8 for each evaluation index (containing n variables), and represents the factor scores on the first and second principal component axes. Finally, each composite index of ecosystem function was again adjusted to a range of 0.1–1 using Equation 7.
One-way analysis of variance (ANOVA) and Tukey pairwise comparisons were performed using IBM SPSS Statistics (version 19.0 for Windows; SPSS, Chicago, IL, USA); these tests were used to analyze the effects of different grassland restoration models on species diversity, CWM, functional trait diversity, and ecosystem function. Tukey pairwise comparisons were considered statistically significant at P<0.05. Pearson correlation analysis was conducted to assess the relationships among species diversity, CWM, and functional trait diversity using Origin 2021(version 2021 for Windows; OriginLab, MAS, Hampton, USA). Redundancy analysis (RDA) based on forward selection was carried out to evaluate the effects of species diversity and plant functional traits on ecosystem function using Canoco (version 5.0 for Windows; Ithaca, NY, USA), and the Monte Carlo permutation test was performed to select explanatory factors that had significant effects on changes in ecosystem function (P<0.05).
We conducted ANOVA and Tukey pairwise comparisons to analyze species diversity, CWM, and functional trait diversity in grassland ecosystems under different restoration models. In terms of species diversity (Table 2), the Margalef index and the Shannon–Wiener index were significantly lower in DG and LP than in NG (P<0.05), while the Simpson index was significantly higher in the former two ecosystems than in NG (P<0.05), but there was no significant difference between DG and LP (P>0.05). In addition, there were no significant differences (P>0.05) between NG and LT in terms of the Margalef index, Shannon–Wiener index, Pielou index, or Simpson index. Furthermore, the differences in Pielou index between treatments were also not significant (P>0.05).
The CWM of grassland ecosystems under different restoration models varied significantly (Figure 1). CWMH ranged from 28.02 to 101.30 cm. The distribution of CWMH was more concentrated in LT, while the variance of CWMH was greatest in DG. Compared to NG, CWMH was significantly higher in DG, LP, and LT (P<0.05). The median variation in CWMLDMC ranged from 21.49 to 66.28 g·g-1. CWMLDMC was highest in LT and lowest in NG. CWMSLA varied from 0.61 to 1.85 cm2·g-1. The distribution of CWMSLA was more concentrated in LT, while the variance of CWMSLA was greatest in NG. CWMSLA was significantly higher in NG than in other grassland ecosystems (P<0.05), but there were no significant differences among DG, LP, and LT (P>0.05). The median variation in CWMRL ranged from 10.37 to 12.71 cm. CWMRL was highest in LP (P<0.05), but there was no significant difference in CWMRL between NG and DG, or between DG and LT (P>0.05). CWMRDMC ranged from 3.58 to 10.80 g·g-1. CWMRDMC was significantly higher in DG, LP, and LT than in NG (P<0.05), but there was no significant difference between LT and LP (P>0.05).
Figure 1 CWM of grassland ecosystems under different restoration models. Different letters in the same panel indicate significant differences between treatments based on Tukey pairwise comparisons (p<0.05). CWMH, CWMLDMC, CWMSLA, CWMRL, and CWMRDMC represent the CWM for plant height, leaf dry matter content, specific leaf area, root length, and root dry matter content, respectively.DG, Dactylis glomerata grassland ecosystem; LP, Lolium perenne grassland ecosystem; LT, Lolium perenne + Trifolium repens grassland ecosystem; NG, natural grassland ecosystem.
It was not difficult to observe that functional trait diversity in grassland ecosystems varied greatly among different restoration models (Figure 2). FRic was significantly higher in DG and LP than in NG and LT (P<0.05), but there was no significant difference between DG and LP, and there was also no significant difference between NG and LT (P>0.05). FDis and RaoQ were significantly lower in DG and LP than in NG (P<0.05), but there was no significant difference between DG and LP (P>0.05), and there was also no significant difference between NG and LT (P>0.05).
Figure 2 Functional trait diversity of grassland ecosystems under different restoration models. Different letters in the same panel indicate significant differences between treatments based on Tukey pairwise comparisons (p<0.05). FRic, FDis, and RaoQ represent the functional richness index, the functional dispersion index, and Rao’s quadratic entropy index, respectively. DG, Dactylis glomerata grassland ecosystem; LP, Lolium perenne grassland ecosystem; LT, Lolium perenne + Trifolium repens grassland ecosystem; NG, natural grassland ecosystem.
We performed correlation analyses of species diversity, CWM, and functional trait diversity in grassland ecosystems under different restoration models (Figure 3). Margalef index was positively correlated with Shannon–Wiener index, FDis, and RaoQ, but negatively correlated with Simpson index and CWMRL (P<0.05). Shannon–Wiener index was positively correlated with Pielou index, FDis, and RaoQ (P<0.05). Shannon–Wiener index and Pielou index were negatively correlated with Simpson index and CWMRL (P<0.05). Simpson index was positively correlated with CWMRL (P<0.05), but negatively correlated with FDis (P<0.05). CWMH was positively correlated with CWMLDMC, CWMRL, CWMRDMC, and FRic, but negatively correlated with CWMSLA, FDis, and RaoQ (P<0.05). CWMLDMC was positively correlated with CWMRDMC, but negatively correlated with CWMSLA and FDis (P<0.05). CWMSLA was positively correlated with FDis but negatively correlated with CWMRDMC and FRic (P<0.05). CWMRL was positively correlated with FRic but negatively correlated with FDis and RaoQ (P<0.05). FRic was negatively correlated with FDis and RaoQ (P<0.05). Finally, FDis was positively correlated with RaoQ (P<0.05).
Figure 3 Correlation analyses of species diversity, CWM, and functional trait diversity in grassland ecosystems under different restoration models. * indicates significance at the 0.05 probability level. The labels Margalef, Shannon, Pielou, and Simpson represent the Margalef index, the Shannon–Wiener index, the Pielou index, and the Simpson index, respectively. CWM.H, CWM.LDMC, CWM.SLA, CWM.RL, and CWM.RDMC represent the CWM of plant height, leaf dry matter content, specific leaf area, root length, and root dry matter content, respectively. FRic, FDis, and RaoQ represent the functional richness index, the functional dispersion index, and Rao’s quadratic entropy index, respectively.
We used ANOVA and Tukey pairwise comparisons to analyze indices of grassland ecosystem function under different restoration models (Table 3). Aboveground biomass was greatest in LT, but there was no significant difference between DG and LP (P>0.05), and there was also no significant difference between DG and NG (P>0.05). Compared to NG, the aboveground biomass in DG, LP, and LT was greater by approximately 20%, 30%, and 44%, respectively. Belowground biomass was greatest in LT and lowest in NG, but there was no significant difference between DG and LP (P>0.05). Compared to NG, the belowground biomass in DG, LP, and LT was greater by approximately 32%, 35%, and 56%, respectively. Plant carbon content was significantly higher in DG, LP, and LT than in NG (P<0.05), but there were no significant differences among DG, LP, and LT (P>0.05). Carbon content in the roots was lowest in NG, but there was no significant difference between DG and LP, and there was also no significant difference between DG and LT (P>0.05). Soil organic carbon, soil field capacity, soil porosity, TN, TP, and TK were significantly higher in LT than under the other treatments (P<0.05). These indices were lowest in NG, but there was no significant difference between DG and LP (P>0.05), except in the case of TP.
Table 3 Analysis results for each evaluation index for grassland ecosystem functions under different restoration models.
The results of PCA showed that the first and second principal components together explained 84.11% of the variance in each ecosystem function group for each evaluation indicator (Figure 4). Based on the explanatory power of the principal component and using this as a weight to calculate the comprehensive index of ecosystem function via Equation 9, we obtained values for the comprehensive index of ecosystem function for grassland ecosystems under different restoration models (Table 4). The same trends in terms of differences between restoration models were observed across multiple components of the comprehensive index of grassland ecosystem function: that is, ecosystem productivity, carbon storage, water conservation, and soil fertility were highest in LT and lowest in NG, and there was no significant difference between DG and LP (P>0.05).
Figure 4 Principal component analysis of each indicator for evaluation of grassland ecosystem function under different restoration models.
Redundancy analysis of plant functional traits and ecosystem function showed that the first and second axes explained 90.68% and 2.74% of the variance in ecosystem function, respectively (Figure 5). CWMLDMC, CWMRDMC, CWMH, the Margalef index, and the Shannon-Wiener index were positively correlated with ecosystem productivity, carbon storage, water conservation, and soil fertility conservation. CWMSLA and CWMRL were negatively correlated with ecosystem productivity, carbon storage, water conservation, and soil fertility conservation. FRic, FDis, and RaoQ were not correlated with ecosystem productivity, carbon storage, water conservation, or soil fertility conservation. Further analysis via the Monte Carlo permutation test showed that CWMLDMC, CWMRL, and CWMH had larger effects on ecosystem function than other plant functional traits, explaining 76.20% (F=56.7, P=0.024), 17.7% (F=28.7, P=0.024), and 2.2% (F=4.5, P=0.048) of the variance, respectively, indicating that CWMLDMC, CWMRL, and CWMH were the main factors affecting ecosystem function.
Figure 5 Redundancy analysis of plant functional traits and grassland ecosystem function. The labels Margalef, Shannon, Pielou, and Simpson represent the Margalef index, the Shannon–Wiener index, the Pielou index, and the Simpson index, respectively. CWM.H, CWM.LDMC, CWM.SLA, CWM.RL, and CWM.RDMC represent the CWM of plant height, leaf dry matter content, specific leaf area, root length, and root dry matter content, respectively. FRic, FDis, and RaoQ represent the functional richness index, the functional dispersion index, and Rao’s quadratic entropy index, respectively. EP, CS, WC, and SFC represent ecosystem productivity, carbon storage, water conservation, and soil fertility conservation, respectively.
Species diversity represents the expression of biodiversity at the species level. As a fundamental characteristic of community functional structure, this measure plays a crucial role in indicating community composition, the dynamics of community change, and strategies for vegetation restoration (Crawley and Harral, 2001). Under various restoration models, variation has been observed in species composition and structure among plant communities, resulting in significant disparities in species diversity (Wang et al., 2022a). In this study, plant species diversity under the three artificial restoration models varied greatly. The Margalef index and Shannon index were highest in LT, and the species diversity of the LT ecosystem was closest to that of NG, which is consistent with the findings of Wu et al. (2019) in the degraded grassland of the Three Rivers source area and those of Zhang H. et al. (2020) in the degraded grassland of the Yellow River headwaters. This indicates that, for degraded ecosystems, planting artificial grassland by mixed sowing can promote the vegetation succession of plant communities and restore species diversity in the degraded ecosystem, which is an important way to restore degraded ecosystems. However, there was no significant difference in Margalef index, Shannon–Wiener index, or Pielou index between the DG and LP ecosystems; this finding was similar to the results of Hou et al. (2015) in the Poa pratensis grassland of Qinghai, but different from the results of Hao et al. (2023) in the sandy grassland of Hulunbeir. This phenomenon could potentially be attributed to the similarity in dominant species between DG and LP, as well as the nature of the study conducted by Hou et al. (2015), in which both areas featured perennial grasses. Perennial grasses share commonalities in terms of morphology, physiology, and life history, which may explain the observed similarities.
Plant functional traits determine plant survival, growth, and reproduction; not only can these characteristics strongly influence ecosystem processes, but they also reflect the response process of ecosystems to environmental changes (Wright et al., 2004; Kraft et al., 2015; Li et al., 2021). The adaptability of plants to the environment and their own plasticity leads to major differences in functional traits among plants, with species themselves often acting as indicators and predictors of environmental change through their adoption of morphological and physiological traits to adapt to environmental changes and access to limited resources (Freschet et al., 2018; Hu L. et al., 2021). We found that the five CWM measures responded very differently to different restoration models during the ecosystem restoration process for management of karst desertification. CWMH, CWMLDMC, and CWMRDMC were significantly higher in artificial grassland ecosystems than in the natural grassland ecosystem, which can be explained by the fact that artificial planting promotes forage with rapid growth strategies as dominant species, and that local dominance of relatively tall plants can lead to higher plant biomass and higher resource storage capacity (Conti and Díaz, 2013; Zuo et al., 2017). However, the CWMSLA of plants in artificial grassland ecosystems was lower, which may be due to the fact that the karst ecosystem in southwest China has specific habitat conditions consisting of karst aridity and high soil calcium, and the plants have acquired resilience to this by altering their own structure and their physiological and biochemical processes to adapt to the environment in the course of survival (Xiong et al., 2002; Xi et al., 2011; Zhong et al., 2018). While SLA is related to stress tolerance and growth rate, species with lower SLA can compete for limited nutrients and have higher stress tolerance (Wright et al., 2004), which also proves that artificially planted grass is better adapted to the particular nature of the karst environment. This result was similar to those of Mason et al. (2016) in grazed grassland in New Zealand. Plants obtain nutrients and water from the soil, mainly through the root system, to support their growth and development, and the root system is the area where the most intense plant–soil interactions take place, largely controlling nutrient activation, water use efficiency, and soil health (Kong et al., 2014; Plaza-Bonilla et al., 2014). In this study, CWMRL was significantly higher in DG and LP than in LT, which is consistent with the results of Li et al. (2022) in grassland in Inner Mongolia. This may be attributable to the fact that gramineous grasses have well-developed fibrous roots with a strong ability to compete for water, and water competition intensifies the growth of gramineous grasses roots in monoculture (Bessler et al., 2012).
The concept of functional trait diversity is intricately linked to the functions of species and ecosystems, as it involves the complementary utilization of resources (Mason et al., 2005). This approach provides a clear and intuitive means of characterizing the magnitude, distribution range, and degree of niche differentiation of functional traits within an ecosystem community (Villéger et al., 2008). The FRic reflects the utilization rate of resources by vegetation by quantifying the niche space occupied by existing species in the community (Jäschke et al., 2020). This study showed that FRic was significantly higher in DG and LP than in NG and LT, indicating that the dominant species of perennial gramineous grasses in the community occupied a larger niche space, the niche differentiation of each species was higher, and resource utilization was more efficient (Petchey and Gaston, 2006; Garcia-Cervigon et al., 2021). This result is inconsistent with the findings of Perring et al. (2018) in European temperate grassland and Freitag et al. (2021) in the Eurasian steppe, which may be caused by the particular nature of the habitat environment of karst (Huang et al., 2022). FDis and RaoQ can be used to measure the degree of niche differentiation and resource competition among plants within a community (Carmona et al., 2016), with higher index values indicating stronger ecological niche complementarity among species, weaker competition, and more efficient resource use (Dong et al., 2019). In this study, FDis and RaoQ were significantly higher in NG and LT than in DG and LP, which is consistent with the results of a related study by Huang et al. (2020) in Chongqing City in China. This may be due to the fact that natural and mixed-seeding grassland ecosystems have a great variety and number of species, producing differences in niches between species, and leading to less niche overlap in resource utilization by individuals in natural and mixed-seeding grassland ecosystems compared to single-seeding grassland ecosystems (Yang et al., 2022).
In this study, the Margalef index and Shannon index were higher in NG and LT than in DG and LP, but FRic did not show the same trend. This may be due to the fact that, in degraded karst ecosystems where species are under environmental stress, species trait show convergence in their functional traits, species trait composition is limited to traits adapted to the selective pressures of that environment, and continued increases in species richness only lead to further spatial differentiation of ecological niches, resulting in reduced interspecific trait variability and no further increase in functional diversity (Sasaki et al., 2009; Khasanova et al., 2019), as well as weakened competition between species. These findings are consistent with the results of Dong et al. (2019) on the adaptive strategies of plants in grassland ecosystems of the Tibetan Plateau and with those of Zhang Z. et al. (2021) in nine forest ecosystems from the tropical to boreal zones in China. The results of this study confirmed the following findings: 1) FDis and RaoQ were significantly higher in NG and LT compared to DG and LP; 2) the Margalef index and Shannon index were significantly positively correlated with FDis and RaoQ, but not significantly correlated with FRic; 3) CWMH and CWMRL were significantly negatively correlated with FDis and RaoQ. In addition, the relationship between the Margalef index and Shannon index, as well as functional trait diversity, varied across different types of plant communities. This was confirmed by Weng et al. (2017) through their study of forest communities, suggesting that the relationship between species diversity and functional diversity has originally varied across environmental contexts, even when these are unaffected by external disturbance.
In this study, CWMH was significantly and positively correlated with CWMLDMC, CWMRL, CWMRDMC, and FRic. This may be due to the fact that plant height affects the ability of plants to acquire light and to photosynthesize; specifically, the greater a plant’s height, the greater its ability to so, which contributes to an increase in leaf dry matter content, root length, and root dry matter content. In turn, these also contribute to an increase in the diversity of plant functional traits (Angassa, 2014). These results are similar to those obtained by Maire et al. (2015) in their study of global terrestrial ecosystems. SLA is an important indicator for measurement of the growth status and light energy utilization efficiency of species, and LDMC mainly reflects the ability to retain plant nutrient elements (Hao et al., 2019). Related studies have shown that SLA can reflect the ability of plants to obtain resources, and SLA is usually negatively correlated with LDMC (Shen et al., 2019), which may explain the findings of a significant negative correlation between CWMLDMC and CWMSLA in this study. This is consistent with the results of Niu et al. (2016) in Tibetan alpine meadows, those of Zhang et al. (2018) in typical grassland of Horqin sandy land, China, and those of Wang Q. et al. (2022) in degraded woody plants of a karst area. This finding also indicates that the artificial grass in our study area was better adapted to the karst environment, and thus their LDMC was higher. Furthermore, FRic was negatively correlated with FDis and RaoQ, but FDis was positively correlated with RaoQ in our study. This is inconsistent with the results of Jiang X. et al. (2022) in the Mu Us sandy grassland of China and with those of Wang et al. (2022b) in the Maolan National Karst Forest Nature Reserve, Guizhou, China; however, it is consistent with the results of Petchey and Gaston (2002) on five typical cases of simulated plant functional traits and ecosystem relationships, and with those of Hao et al. (2019) on the functional traits of communities at different stages of succession in the temperate forests of the Changbai Mountains, Northeast China. The reason for this difference may be inconsistencies in species diversity.
The aim of ecological restoration is not only to increase vegetation coverage but also to restore ecosystem quality and function (Zhang M. et al., 2018; Zhang S. et al., 2023). Artificial grassland is an effective way to mitigate the degradation of natural grassland, improve grassland productivity, and ensure ecological security (He et al., 2020; Xu et al., 2020). In this study, DG, LP, and LT were found to have significantly increased aboveground biomass and belowground biomass in the grassland ecosystem compared to NG, with the highest levels being observed in LT. These results are consistent with those of Fox et al. (2020) in mixed seeding grassland in Zurich and those of Li C. et al. (2023) in artificial grassland in Qinghai Lake Basin; this may be because artificial pasture planting has some advantages in increasing forage yield compared to natural grassland, but the advantages of mixed seeding are greater than those of monoculture (Husse et al., 2016). The same trend was also observed in plant, root, and soil total carbon, which indicates that the process of accumulation of soil nutrients was slow during the natural process of recovery of the karst ecosystem, while the mixed-seeding grassland could significantly increase soil organic carbon; the effect of mixed leguminous forage has been found to be especially clear (Bai and Cotrufo, 2022). Furthermore, there is a positive correlation between plant and root carbon and soil carbon, with increases in soil carbon promoting higher plant and root carbon (Su et al., 2019). The results of Peng et al. (2020), in a comparative analysis of alpine meadow and alpine steppe, have also confirmed this viewpoint; this may be due to the fact that the soil environment of artificial grassland tends to be stable, with an increase in surface litter and in the underground root system, which is capable of sustainably recharging litter into the soil, increasing the source of soil organic carbon and promoting organic carbon accumulation (Li X. et al., 2023). Soil water is an important source of plant water and a carrier for nutrient cycling and material transformation (Yang et al., 2016). Soil porosity is an important indicator for characterization of soil aeration and water permeability, and soil with high porosity is more likely to expel water (Zhang et al., 2019). Not only can soil organic carbon enhance the soil’s ability to hold and release fertilizer, but it can also promote the formation of granular structure and improve soil’s water permeability, water-holding capacity, and aeration (Zhang J. et al., 2021). This can explain the findings of this study in which soil field capacity and soil porosity were the highest in LT and the lowest in NG, which is consistent with the results of Yang et al. (2017) study of ecological restoration projects affecting hydrological function in the karst region of southwest China.
Soil plays a crucial role in the formation of grassland ecosystem function and the provision of ecosystem services, as it carries out nutrient cycling (Eekeren et al., 2010; Bell et al., 2012). Relevant studies have shown that restoration of vegetation can effectively improve soil fertility within a relatively short period of time (15 years) (Hu et al., 2020). Soil nitrogen supply plays an important role in determining ecosystem structure and function (Hu P. et al., 2021), and the availability of soil nitrogen is often a key limiting factor for productivity (Wang D. et al., 2020). In this study, DG, LP, and LT significantly increased soil total nitrogen content compared to NG, and this was at its highest in LT. This may be due to the relative stability of the mixed-seeding grassland, which enhances photosynthesis, root activity, and soil microbial activity, and promotes nitrogen accumulation capacity; additionally, the biological nitrogen fixation of legume forage in LT also increased nitrogen content, meaning that the presence of mixed-seeding grassland was more conducive to the accumulation of soil total nitrogen (Nyfeler et al., 2011; Frankow-Lindberg and Dahlin, 2013). This is consistent with results on the planting years of different grasslands obtained in the Sanjiangyuan region of China by Xing et al. (2020), but inconsistent with the results of Hu et al. (2021), who studied the effects of vegetation restoration methods on soil N supply in artificial and natural forests in the karst region of southwest China; this might be related to the different vegetation types studied (Song et al., 2022). Soil phosphorus and potassium are the main nutrients affecting plant growth, and higher levels of phosphorus and potassium imply good soil fertility status and higher system productivity (Cheng et al., 2016; Wang et al., 2019; Zhang Y. et al., 2021). In this study, DG, LP, and LT significantly increased the total phosphorus content compared to NG, and this was at its highest in LT. This may be because the biomass, soil organic carbon, and total nitrogen content were significantly higher in artificial grassland than in natural grassland, providing a favorable soil environment for the accumulation of phosphorus and potassium; because mixed-seeding grassland creates inter-root space for the use of phosphorus and potassium nutrients; and because the advantage of the difference in the utilization of phosphorus and potassium sources contributes to a more pronounced increase in soil phosphorus and potassium content (Wagg et al., 2014; Crème et al., 2016). Although the species diversity in the natural grassland ecosystem was higher than that observed in the single-seeding grassland ecosystem in this study, N is a limiting factor affecting the productivity of natural grassland at present (Song et al., 2022). In contrast, the local dominance of high-productivity species in artificial grassland ecosystems promotes the improvement of overall primary productivity (Duchini et al., 2016), and the ecosystem function of artificial grassland is significantly enhanced under plant and soil interaction. Therefore, the interaction of the above factors resulted in the highest ecosystem productivity, carbon storage, water conservation, and soil fertility conservation occurring in LT, with the lowest occurring in NG.
Although the importance of species diversity and plant functional traits in maintaining ecological functions is still debated, numerous studies have shown that both factors have positive effects on ecosystem function (Cadotte, 2017; Armstrong et al., 2021; Chaves et al., 2021; Gao et al., 2022; Dendoncker et al., 2023; Yan et al., 2023). In this study, CWMLDMC, CWMRDMC, CWMH, the Margalef index, and the Shannon index were positively correlated with ecosystem productivity, carbon storage, water conservation, and soil fertility conservation. This indicates that the functioning of the grassland ecosystem in this region is influenced by both species richness and plant functional traits. CWM can be used to assess the effect of dominant traits on ecosystem function, while the functional trait diversity index can quantify the effect of variation in the trait on ecosystem function (Choudhury et al., 2022). The results of RDA in this study showed that the CWM explained the effects of grassland ecosystem function to a greater extent than the functional trait diversity index and the species diversity index, indicating that the dominant species in the community had a greater influence on ecosystem productivity, carbon storage, water conservation, and soil fertility conservation. This suggests that ecosystem function may depend on the functional traits of the dominant species in the community. Specifically, CWMH, CWMLDMC, and CWMRL were the main factors affecting grassland ecosystem function. This result has also been obtained in studies of degraded grassland ecosystems in the Loess Plateau of China (Jing et al., 2019), Selside meadows in the UK (Sweeney et al., 2020), and subtropical forest ecosystems in the central Himalayas (Sigdel et al., 2022). Therefore, in this study, the mass-ratio hypothesis was found to better explain the response of grassland ecosystem function to plant functional traits under different vegetation restoration models in this region in comparison to the niche differentiation hypothesis.
This research focuses on the desertification control in the karst ecosystem and examines the impact of plant functional traits on the functioning of grassland ecosystems under various vegetation restoration models in an area of karst desertification. In this study, species diversity (assessed via the Margalef index and Shannon index), FDis, and RaoQ were higher in the grassland ecosystem under the natural restoration model than in ecosystems under artificial restoration models, but the differences between the NG and LT were not significant. Furthermore, grassland ecosystem function, including ecosystem productivity, carbon storage, water conservation, and soil fertility conservation, was found to be at its lowest in NG and highest in LT. This is because the CWM explains a larger proportion of the variance in grassland ecosystem function than the functional trait diversity index, and changes in ecosystem function depend largely on the functional traits of the dominant species in the community. Therefore, we conclude that the mass-ratio hypothesis can better explain the response of grassland ecosystem function to plant functional traits under different vegetation restoration models in this region in comparison to the niche differentiation hypothesis. This may be due to the relatively short monitoring period; in order to study changes in the underlying mechanism, we will need to carry out long-term monitoring. In addition, while plant functional traits have a significant impact on grassland ecosystem function in the area of karst desertification, it is important to consider the effects of climate, soil, and anthropogenic interference on ecosystem recovery in future research. This study proved that, among the different vegetation restoration models employed in the area of karst desertification, ecosystem function was the best in the case of mixed-seeding grassland (perennial ryegrass + white clover), which indicates that degraded karst ecosystem function can be restored by the method of mixed-seeding grassland.
The datasets presented in this study can be found in online repositories. The names of the repository/repositories and accession number(s) can be found below: Data available via the figshare https://doi.org/10.6084/m9.figshare.22758158.v1.
SS and KX performed the sampling and contributed to the experimental design, data analysis, and writing of the manuscript. KX provided the study site and funding. KX and YC contributed to the sampling, experimental design, data analysis, and writing of the manuscript. All authors contributed to the article and approved the submitted version.
This research was funded by the Key Science and Technology Program of Guizhou Province: Poverty Alleviation Model and Technology Demonstration for Ecoindustries Derived from Karst Desertification Control (No.5411 2017 QianKehe Pingtai Rencai); the China Overseas Expertise Introduction Program for Discipline Innovation: Overseas Expertise Introduction Center for South China Karst Eco-environment Discipline Innovation (D17016); the Natural Science Research Project of the Education Department of Guizhou Province (Qianjiaohe KY Zi (2022) 157); and the Academic New Seedling Fund Project of Guizhou Normal University (Qianshi Xinmiao B15).
The authors declare that the research was conducted in the absence of any commercial or financial relationships that could be construed as a potential conflict of interest.
All claims expressed in this article are solely those of the authors and do not necessarily represent those of their affiliated organizations, or those of the publisher, the editors and the reviewers. Any product that may be evaluated in this article, or claim that may be made by its manufacturer, is not guaranteed or endorsed by the publisher.
DG, Dactylis glomerata grassland; LP, Lolium perenne grassland; LT, Lolium perenne + Trifolium repens grassland; NG, natural grassland; H, plant height; SLA, specific leaf area; LDMC, leaf dry matter content; RL, root length; RDMC, root dry matter content; CWM, community-weighted mean; CWM.H, community-weighted mean of plant height; CWM.LDMC, community-weighted mean of leaf dry matter content; CWM.SLA, community-weighted mean of specific leaf area; CWM.RL, community-weighted mean of root length; CWM.RDMC, community-weighted mean of root dry matter content; FRic, functional richness index; FDis, functional dispersion index; RaoQ, Rao’s quadratic entropy index; AB, aboveground biomass; BB, belowground biomass; PC, plant C content; RC, root C content; SOC, soil organic carbon; WC, water conservation; SFC, soil field capacity; SP, soil porosity; TN, total nitrogen; TP, total phosphorus; TK, total potassium.
Albert, C. H., Bello, F. D., Boulangeat, I., Pellet, G., Lavorel, S., Thuiller, W. (2012). On the importance of intraspecific variability for the quantification of functional diversity. Oikos 121, 116–126. doi: 10.1111/j.1600-0706.2011.19672.x
Angassa, A. (2014). Effects of grazing intensity and bush encroachment on herbaceous species and rangeland condition in Southern Ethiopia. Land Degrad. Dev. 25, 438–451. doi: 10.1002/ldr.2160
Armstrong, C. G., Miller, J. E. D., McAlvay, A. C., Ritchie, P. M., Lepofsky, D. (2021). Historical indigenous land-use explains plant functional trait diversity. Ecol. Soc. 26, 6. doi: 10.5751/ES-12322-260206
Bai, Y. F., Cotrufo, M. F. (2022). Grassland soil carbon sequestration: Current understanding, challenges, and solutions. Science 377, 603–608. doi: 10.1126/science.abo238
Bell, L. W., Sparling, B., Tenuta, M., Entz, M. H. (2012). Soil profile carbon and nutrient stocks under long-term conventional and organic crop and alfalfa-crop rotations and re-established grassland. Agriculture Ecosyst. Environ. 158, 156–163. doi: 10.1016/j.agee.2012.06.006
Benayas, J. M. R., Newton, A. C., Diaz, A., Bullock, J. M. (2009). Enhancement of biodiversity and ecosystem services by ecological restoration: A meta-analysis. Science 325, 1121–1124. doi: 10.1126/science.1172460
Bessler, H., Oelmann, Y., Roscher, C., Buchmann, N., Scherer-Lorenzen, M., Schulze, E. D., et al. (2012). Nitrogen uptake by grassland communities: contribution of N2 fixation, facilitation, complementarity, and species dominance. Plant Soil 358, 301–322. doi: 10.1007/s11104-012-1181-z
Brown, L. M., Anand, M. P. (2022). Plant functional traits as measures of ecosystem service provision. Ecosphere 13, e3930. doi: 10.1002/ecs2.3930
Cadotte, M. W. (2017). Functional traits explain ecosystem function through opposing mechanisms. Ecol. Lett. 20, 989–996. doi: 10.1111/ele.12796
Canedoli, C., Ficetola, G. F., Corengia, D., Tognini, P., Ferrario, A., Padoa-Schioppa, E. (2021). Integrating landscape ecology and the assessment of ecosystem services in the study of karst areas. Landscape Ecol. 37, 347–365. doi: 10.1007/s10980-021-01351-2
Cardinale, B. J., Duffy, J. E., Gonzalez, A., Hooper, D. U., Perrings, C., Venail, P., et al. (2012). Biodiversity loss and its impact on humanity. Nature 486, 59–67. doi: 10.1038/nature1114
Carmona, C. P., Bello, D. F., Mason, N. W. H., Lepš, J. (2016). Traits without borders: Integrating functional diversity across scales. Trends Ecol. Evol. 31, 382–394. doi: 10.1016/j.tree.2016.02.003
Casanoves, F., Pla, L., Di Rienzo, J. A., Díaz, S. (2011). FDiversity: a software package for the integrated analysis of functional diversity. Methods Ecol. Evol. 2, 233–237. doi: 10.1890/0012-9623-92.2.147
Chaves, J. E., Aranibar, J. N., Gatica, G. (2021). Species and functional plant diversity enhance ecosystem functions in the central Monte desert. J. Vegetation Sci. 32, e12952. doi: 10.1111/jvs.12952
Cheng, Y. T., Li, P., Xu, G. C., Li, Z. B., Cheng, S. D., Gao, H. D. (2016). Spatial distribution of soil total phosphorus in Yingwugou watershed of the Dan River, China. Catena 136, 175–181. doi: 10.1016/j.catena.2015.02.015
Chi, Y. K., Xiong, K. N., Xiao, H., Chen, H., Song, S. Z., Shen, X. Y. (2020). Study on the relationship between disposition models of forest and grass and soil properties in karst rocky desertification areas of southwest China. Fresenius Environ. Bull. 29, 5424–5431.
Chollet, S., Rambal, S., Fayolle, A., Hubert, D., Foulquié, D., Garnier, E. (2014). Combined effects of climate, resource availability, and plant traits on biomass produced in a Mediterranean rangeland. Ecology 95, 737–748. doi: 10.1890/13-0751.1
Choudhury, M. I., Hallin, S., Ecke, F., Hubalek, V., Juhanson, J., Frainer, A., et al. (2022). Disentangling the roles of plant functional diversity and plaint traits in regulating plant nitrogen accumulation and denitrification in freshwaters. Funct. Ecol. 36, 921–932. doi: 10.1111/1365-2435.14001
Cong, W. F., Ruijven, J. V., Mommer, L., Deyn, G. B. D., Berendse, F., Hoffland, E. (2014). Plant species richness promotes soil carbon and nitrogen stocks in grasslands without legumes. J. Ecol. 102, 1163–1170. doi: 10.1111/1365-2745.12280
Conti, G., Díaz, S. (2013). Plant functional diversity and carbon storage - an empirical test in semi-arid forest ecosystems. J. Ecol. 101, 18–28. doi: 10.1111/1365-2745.12012
Crawley, M. J., Harral, J. E. (2001). Scale dependence in plant biodiversity. Science 291, 864–868. doi: 10.3390/f13091505
Crème, A., Rumpel, C., Gastal, F., Gil, M. D. L. L. M., Chabbi, A. (2016). Effects of grasses and a legume grown in monoculture or mixture on soil organic matter and phosphorus forms. Plant Soil 402, 117–128. doi: 10.1007/s11104-015-2740-x
Dendoncker, M., Taugourdeau, S., Messier, C., Vincke, C. (2023). A functional trait-based approach to evaluate the resilience of key ecosystem functions of tropical savannas. Forests 14, 291. doi: 10.3390/f14020291
Diaz, S., Cabido, M. (2001). Vive la différence: plant functional diversity matters to ecosystem processes. Trends Ecol. Evol. 16, 646–655. doi: 10.1016/S0169-5347(01)02283-2
Díaz, S., Lavorel, S., Bello, F. D., Quétier, F., Grigulis, K., Robson, T. M. (2007). Incorporating plant functional diversity effects in ecosystem service assessments. Proc. Natl. Acad. Sci. United States America 104, 20684–20689. doi: 10.1016/j.baae.2010.06.009
Dong, S. K., Tang, L., Zhang, X. F., Liu, S. L., Liu, Q. R., Su, X. K., et al. (2019). Relationship between plant species diversity and functional diversity in alpine grasslands. Acta Ecologica Sin. 37, 1472–1483. doi: 10.5846/stxb201509281981
Duchini, P. G., Guzatti, G. C., Ribeiro-Filho, H. M. N., Sbrissia, A. F. (2016). Intercropping black oat (Avena strigosa) and annual ryegrass (Lolium multiflorum) can increase pasture leaf production compared with their monocultures. Crop Pasture Sci. 67, 574–581. doi: 10.1071/CP15170
Duffy, J. E., Godwin, C. M., Cardinale, B. J. (2017). Biodiversity effects in the wild are common and as strong as key drivers of productivity. Nature 549, 261. doi: 10.1038/nature23886
Eekeren, N. V., Boer, H. D., Hanegraaf, M., Bokhorst, J., Nierop, D., Bloem, J., et al. (2010). Ecosystem services in grassland associated with biotic and abiotic soil parameters. Soil Biol. Biochem. 42, 1491–1504. doi: 10.1016/j.soilbio.2010.05.016
Fan, F., Liang, C. Z., Tang, Y. K., Harker-Schuch, I., Porter, J. R. (2019). Effects and relationships of grazing intensity on multiple ecosystem services in the Inner Mongolian steppe. Sci. Total Environ. 675, 642–650. doi: 10.1016/j.scitotenv.2019.04.279
Faucon, M. P., Houben, D., Reynoird, J. P., Mercadal-Dulaurent, A. M., Armand, R., Hans, L. (2015). Advances and perspectives to improve the phosphorus availability in cropping systems for agroecological phosphorus management. Adv. Agron. 134, 51–79. doi: 10.1016/bs.agron.2015.06.003
Finegan, B., Peña-Claros, M., de Oliveira, A., Ascarrunz, N., Bret-Harte, M. S., Carreño Rocabado, G., et al. (2015). Does functional trait diversity predict above-ground biomass and productivity of tropical forests? Testing three alternative hypotheses. J. Ecol. 103, 191–201. doi: 10.1111/1365-2745.12346
Flynn, D., Mirotchnick, N., Jain, M., Palmer, M., Naeem, S. (2011). Functional and phylogenetic diversity as predictors of biodiversity-ecosystem-function relationships. Ecology 92, 1573–1581. doi: 10.1890/10-1245.1
Fox, A., Suter, M., Widmer, F., Lüscher, A. (2020). Positive legacy effect of previous legume proportion in a ley on the performance of a following crop of Lolium multiflorum. Plant Soil 447, 497–506. doi: 10.1007/s11104-019-04403-4
Frankow-Lindberg, B. E., Dahlin, A. S. (2013). N2 fixation, N transfer,and yield in grassland communities including a deep-rooted legume or non-legume species. Plant Soil 370, 567–581. doi: 10.1007/s11104-013-1650-z
Freitag, M., Kamp, J., Dara, A., Kuemmerle, T., Sidorova, T. V., Stirnemann, I. A., et al. (2021). Post-Soviet shifts in grazing and fire regimes changed the functional plant community composition on the Eurasian steppe. Global Change Biol. 27, 388–401. doi: 10.1111/GCB.15411
Freschet, G. T., Violle, C., Bourget, M. Y., Scherer-Lorenzen, M., Fort, F. (2018). Allocation, morphology, physiology, architecture: the multiple facets of plant above- and below-ground responses to resource stress. New Phytol. 219, 1338–1352. doi: 10.1111/nph.15225
Fry, E. L., Savage, J., Hall, A. L., Oakley, S., Pritchard, W. J., Ostle, N. J., et al. (2018). Soil multifunctionality and drought resistance are determined by plant structural traits in restoring grassland. Ecology 99, 2260–2271. doi: 10.1002/ecy.2437
Gamfeldt, L., Roger, F. (2017). Revisiting the biodiversity-ecosystem multifunctionality relationship. Nat. Ecol. Evol. 1, 168. doi: 10.1038/s41559-017-0168
Gao, D. X., Wang, S., Wei, F. L., Wu, X. T., Zhou, S., Wang, L. X., et al. (2022). The vulnerability of ecosystem structure in the semi-arid area revealed by the functional trait networks. Ecol. Indic. 139, 108894. doi: 10.1016/j.ecolind.2022.108894
Garcia-Cervigon, A. I., García-Lopez, M. A., Piston, N., Pugnaire, F. I., Olano, J. M. (2021). Co-ordination between xylem anatomy, plant architecture and leaf functional traits in response to abiotic and biotic drivers in a nurse cushion plant. Ann. Bot. 127, 919–929. doi: 10.1093/aob/mcab036
Garnier, E., Cortez, J., Billès, G., Navas, M. L., Roumet, C., Debusssche, M., et al. (2004). Plant functional markers capture ecosystem properties during secondary succession. Ecology 85, 2630–2637. doi: 10.1890/03-0799
Garnier, E., Navas, M. L., Karl, G. (2015). Plant functional diversity: organism traits, community structure, and ecosystem properties (Oxford: Oxford University Press). doi: 10.1111/aec.12498
Gonzalez, A., Germain, R. M., Srivastava, D. S., Filotas, E., Dee, L. E., Gravel, D., et al. (2020). Scaling-up biodiversity-ecosystem functioning research. Ecol. Lett. 23, 757–776. doi: 10.1111/ele.13456
Grime, J. P. (1998). Benefits of plant diversity to ecosystems: immediate, filter and founder effects. J. Ecol. 86, 902–910. doi: 10.1046/j.1365-2745.1998.00306.x
Gross, N., Bagousse-Pinguet, L. Y., Liancourt, P., Berdugo, M., Gotelli, N. J., Maestre, F. T. (2017). Functional trait diversity maximizes ecosystem multifunctionality. Nat. Communication Evol. 1, 0132. doi: 10.1016/j.ecolind.2020.106415
Gu, Y. Y., Lin, N. F., Ye, X., Xu, M. J., Qiu, J., Zhang, K., et al. (2022). Assessing the impacts of human disturbance on ecosystem services under multiple scenarios in karst areas of China: Insight from ecological conservation red lines effectiveness. Ecol. Indic. 142, 109202. doi: 10.1016/j.ecolind.2022.109202
Hao, H. J., Guan, X., Cao, M., Li, J. S. (2023). Study on the characteristics of plant communities in different ecological restoration stages of Hulun Buir sandy grassland. J. Environ. Eng. Technol., 13: 1573–1585. doi: 10.1111/1365-2745.13250
Hao, S. J., Li, X. Y., Hou, M. M., Zhao, X. H. (2019). Spatial variations of community functional traits at different successional stages in temperate forests of Changbai Mountains, Northeast China. Chin. J. Plant Ecol. 43, 208–216. doi: 10.17521/cjpe.2018.0295
He, J. S., Liu, Z. P., Yao, T., Zhu, J. X. (2020). Analysis of the main constraints and restoration techniques of degraded grassland on the Tibetan Plateau. Sci. Technol. Rev. 38, 66–80. doi: 10.3981/j.issn.1000-7857.2020.17.007
He, X. J., Wang, L., Ke, B., Yue, Y. M., Wang, K. L., Cao, J. H., et al. (2019). Progress on ecological conservation and restoration for China Karst. Acta Ecologica Sin. 39, 6577–6585. doi: 10.5846/stxb201812292842
Hector, A., Bagchi, R. (2007). Biodiversity and ecosystem multifunctionality. Nature 448, 188–190. doi: 10.1038/s41559-017-0168
Hobbie, S. E. (2015). Plant species effects on nutrient cycling: revisiting litter feedbacks. Trends Ecol. Evol. 30, 357–363. doi: 10.1016/j.tree.2015.03.015
Hou, X. K., Dong, Q. Q., Shi, J. J., Wang, Y. L., Chen, L. L., Song, L. (2015). Study on the community structure characteristics and soil physico-chemical property of Poa Pratensis L.Cv.Qinghai single artificial grasslands in different ages. Chin. J. Grassland 37, 65–69.
Hu, L. Y., Qin, D. Y., Lu, H. Y., Li, W., Shang, K. K., Lin, D. M., et al. (2021). Urban growth drives trait composition of urban spontaneous plant communities in a mountainous city in China. J. Environ. Manage. 293, 112869. doi: 10.1016/J.JENVMAN.2021.112869
Hu, P. L., Xiao, J., Zhang, W., Xiao, L. M., Yang, R., Xiao, D., et al. (2020). Response of soil microbial communities to natural and managed vegetation restoration in a subtropical karst region. Catena 195, 104849. doi: 10.1016/j.catena.2020.104849
Hu, P. L., Zhao, Y., Xiao, D., Xu, Z. Z., Zhang, W., Xiao, J., et al. (2021). Dynamics of soil nitrogen availability following vegetation restoration along a climatic gradient of a subtropical karst region in China. J. Soils Sediments 21, 2167–2178. doi: 10.1007/s11368-021-02915-0
Huang, C., Wei, H., Wu, K. J., He, X. R., Wang, P., Qi, Y. C., et al. (2020). The functional diversity of understory plants during the transformation from Pinus massoniana to Cinnamomum camphora forest. Acta Ecologica Sin. 40, 4573–4584. doi: 10.5846/stxb201911152455
Huang, L. J., Yu, Y. M., An, X. F., Yu, L. L., Xue, Y. G. (2022). Leaf functional traits, species diversity and functional diversity of plant community in Tiankeng forests. Acta Ecologica Sin. 42, 10264–10275. doi: 10.5846/stxb202110273025
Husse, S., Huguenin-Elie, O., Buchmann, N., Lüscher, A. (2016). Larger yields of mixtures than monocultures of cultivated grassland species match with asynchrony in shoot growth among species but not with increased light interception. Field Crops Res. 194, 1–11. doi: 10.1016/j.fcr.2016.04.021
Jäschke, Y., Heberling, G., Wesche, K. (2020). Environmental controls override grazing effects on plant functional traits in Tibetan rangelands. Funct. Ecol. 34, 747–760. doi: 10.1111/1365-2435.13492
Jiang, X. Y., Gao, S. J., Jiang, Y., Tian, B., Jia, X., Cha, T. T. (2022). Species diversity,functional diversity,and phylogenetic diversity in plant communities at different phases of vegetation restoration in the Mu Us sandy grassland. Biodiversity Sci. 30, 15–25. doi: 10.17520/biods.2021387
Jiang, M., He, L. Y., Fan, B. J., Wang, T., Yang, N., Liu, Y. L., et al. (2022). Intraspecific more than interspecific diversity plays an important role on Inner Mongolia grassland ecosystem functions: A microcosm experiment. Sci. Total Environ. 826, 154134. doi: 10.1016/j.scitotenv.2022.154134
Jing, G. H., Cheng, J. M., Su, J. S., Wei, L., Hu, T. M., Li, W. (2019). Community-weighted mean traits play crucial roles in driving ecosystem functioning along long-term grassland restoration gradient on the Loess Plateau of China. J. Arid Environments 165, 97–105. doi: 10.1016/j.jaridenv.2019.01.018
Kazakou, E., Vile, D., Shipley, B., Gallet, C., Garnier, E. (2006). Co-variations in litter decomposition, leaf traits and plant growth in species from a Mediterranean old-field succession. Funct. Ecol. 20, 21–30. doi: 10.1111/j.1365-2435.2006.01080.x
Kearney, S. P., Fonte, S. J., Garcia, E., Siles, P., Chan, K. M. A., Smukler, S. M. (2019). Evaluating ecosystem service trade-offs and synergies from slash-and-mulch agroforestry systems in El Salvador. Ecol. Indic. 105, 264–278. doi: 10.1016/j.ecolind.2017.08.032
Khasanova, A., Lovell, J. T., Bonnette, J., Weng, X. Y., Jenkins, J., Yoshinaga, Y., et al. (2019). The genetic architecture of shoot and root trait divergence between mesic and xeric ecotypes of a perennial grass. Frontiersin Plant Sci. 10. doi: 10.3389/fpls.2019.00366
Kong, X. P., Zhang, M. L., Smet, I. D., Ding, Z. J. (2014). Designer crops: optimal root system architecture for nutrient acquisition. Trends Biotechnol. 32, 597–598. doi: 10.1016/j.tibtech.2014.09.008
Kraft, N. J. B., Godoy, O., Levine, J. M. (2015). Plant functional traits and the multidimensional nature of species coexistence. Proc. Natl. Acad. Sci. United States America 112, 797–802. doi: 10.1073/pnas.1413650112
Lange, M., Eisenhauer, N., Sierra, C. A., Bessler, H., Engels, C., Griffiths, R. I., et al. (2015). Plant diversity increases soil microbial activity and soil carbon storage. Nat. Commun. 6, 6707. doi: 10.1038/ncomms7707
Lei, L. J., Kong, D. L., Li, X. M., Zhou, Z. X., Li, G. Y. (2016). Plant functional traits, functional diversity, and ecosystem functioning: current knowledge and perspectives. Biodiversity Sci. 24, 922–931. doi: 10.17520/biods.2015295
Li, X. Y., Li, Y. H., Qin, W. P., Sun, Y., Ma, Z. W., Liu, X. (2023). Impacts of artificial grasslands on soil organic carbon fractions in a degraded alpine meadow of the Three-River Source Region. Chin. J. Ecol., 1–9.
Li, X. L., Png, G. K., Li, Y. H., Jimoh, S. O., Ding, Y., Li, F., et al. (2021). Leaf plasticity contributes to plant anti-herbivore defenses and indicates selective foraging: Implications for sustainable grazing. Ecol. Indic. 122, 107273. doi: 10.1016/j.ecolind.2020.107273
Li, H. Z., Wang, T. Q., Baoyin, T. G. T., Zhang, T. Y., Yan, W. B., Huang, N., et al. (2022). Effects of plant diversity on root morphology and leaf area index of legume-grass mixture community. Chin. J. Grassland 44, 50–57. doi: 10.16742/j.zgcdxb.20210144
Li, H. N., Zhang, Z. G., Duan, J. T., Li, N., Li, B. X., Song, T. T., et al. (2020). Electrochemical disinfection of secondary effluent from a wastewater treatment plant: Removal efficiency of ARGs and variation of antibiotic resistance in surviving bacteria. Chem. Eng. J. 392, 123674. doi: 10.1016/j.cej.2019.123674
Li, C. D., Zhang, C. P., Yang, Y., Dong, Q. M. (2023). Effects of planting patterns on vegetation and soil characteristics of artificial pasture in the qinghai lake basin. Acta Agrestia Sin. 31, 471–478. doi: 10.11733/j.issn.1007-0435.2023.02.021
Liu, L. B., Hu, J., Chen, X. Y., Xu, X., Yang, Y., Ni, J. (2022). Adaptation strategy of karst forests: Evidence from the community-weighted mean of plant functional traits. Ecol. Evol. 12, e8680. doi: 10.1002/ece3.8680
Liu, C., Li, Y., Yan, P., He, N. (2021). How to improve the predictions of plant functional traits on ecosystem functioning? Front. Plant Sci. 12. doi: 10.3389/fpls.2021.622260
Liu, M. X., Zhang, G. J., Yin, F. L., Wang, S. Y., Li, L. (2022). Relationship between biodiversity and ecosystem multifunctionality along the elevation gradient in alpine meadows on the eastern Qinghai-Tibetan plateau. Ecol. Indic. 141, 109070. doi: 10.1016/j.ecolind.2022.109097
Maire, V., Wright, I. J., Prentice, I. C., Batjes, N. H., Bhaskar, R., Bodegom, P. M. V., et al. (2015). Global effects of soil and climate on leaf photosynthetic traits and rates. Global Ecol. Biogeography 24, 706–717. doi: 10.1111/geb.12296
Mammola, S., Carmona, C. P., Guillerme, T., Cardoso, P. (2021). Concepts and applications in functional diversity. Funct. Ecol. 35, 0269–8463. doi: 10.1111/1365-2435.13882
Mason, N. W. H., Mouillot, D., Lee, W. G., Wilson, J. B. (2005). Functional richness, functional evenness and functional divergence: the primary components of functional diversity. Oikos 111, 112–118. doi: 10.1111/j.0030-1299.2005.13886.x
Mason, N. W. H., Orwin, K., Lambie, S., Woodward, S. L., McCready, T., Mudge, P. (2016). Leaf economics spectrum-productivity relationships in intensively grazed pastures depend on dominant species identity. Ecol. Evol. 6, 3079–3091. doi: 10.1002/ece3.1964
McCormack, M. L., Dickie, I. A., Eissenstat, D. M., Fahey, T. J., Fernandez, C. W., Guo, D., et al. (2015). Redefining fine roots improves understanding of below-ground contributions to terrestrial biosphere processes. New Phytol. 207, 505–518. doi: 10.1111/nph.13363
McCormack, M. L., Guo, D. L., Iversen, C. M., Chen, W. L., Eissenstat, D. M., Fernandez, C. W., et al. (2017). Building a better foundation: improving root-trait measurements to understand and model plant and ecosystem processes. New Phytol. 215, 27–37. doi: 10.1111/nph.14459
Miao, D. N., Peng, X. Y., Teng, Q. M., Sardar, M. F., Zhang, Q. Q., Zhu, X. T., et al. (2023). Different contributions of bacterial and fungal communities to nitrogen mineralization in Moso bamboo-invaded subtropical forests. J. Soils Sediments 23, 1123–1134. doi: 10.1007/s11368-022-03380-z
Mouillot, D., Graham, N. A., Villéger, S., Mason, N. W., Bellwood, D. R. (2013). A functional approach reveals community responses to disturbances. Trends Ecol. Evol. 28, 167–177. doi: 10.1016/j.tree.2012.10.004
Niu, K. C., He, J. S., Lechowicz, M. J. (2016). Grazing-induced shifts in community functional composition and soil nutrient availability in Tibetan alpine meadows. J. Appl. Ecol. 53, 1554–1564. doi: 10.1111/1365-2664.12727
Nyfeler, D., Huguenin-Elie, O., Suter, M., Frossard, E., Lüscher, A. (2011). Grass-legume mixtures can yield more nitrogen than legume pure stands due to mutual stimulation of nitrogen uptake from symbiotic and non-symbiotic sources. Agric. Ecosyst. Environ. 140, 155–163. doi: 10.1016/j.proenv.2015.07.253
Peng, F., Xue, X., Li, C. Y., Lai, C. M., Sun, J., Tsubo, M., et al. (2020). Plant community of alpine steppe shows stronger association with soil properties than alpine meadow alongside degradation. Sci. Total Environ. 733, 139048. doi: 10.1016/j.scitotenv.2020.139048
Perring, M. P., Bernhardt-Romermann, M., Baeten, L., Verheyen, K. (2018). Global environmental change effects on plant community composition trajectories depend upon management legacies. Global Change Biol. 24, 1722–1740. doi: 10.1111/gcb.14030
Petchey, O. L., Gaston, K. J. (2002). Functional diversity (FD), species richness and community composition. Ecol. Lett. 5, 402–411. doi: 10.1046/j.1461-0248.2002.00339.x
Petchey, O. L., Gaston, K. J. (2006). Functional diversity: back to basics and looking forward. Ecol. Lett. 9, 741–758. doi: 10.1111/j.1461-0248.2006.00924.x
Plas, F. V. D. (2019). Biodiversity and ecosystem functioning in naturally assembled communities. Biol. Rev. 94, 1220–1245. doi: 10.1111/brv.12499
Plaza-Bonilla, D., Álvaro-Fuentes, J., Hansen, N. C., Lampurlanés, J., Cantero-Martínez, C. (2014). Winter cereal root growth and aboveground-belowground biomass ratios as affected by site and tillage system in dryland Mediterranean conditions. Plant Soil 374, 925–939. doi: 10.1007/s11104-013-1926-3
Qi, W., Kang, X. M., Knops, J. M. H., Jiang, J. C., Abuman, A., Du, G. Z. (2022). The complex biodiversity-ecosystem function relationships for the Qinghai-Tibetan grassland community. Front. Plant Sci. 12. doi: 10.3389/fpls.2021.772503
Rosenfield, M. F., Müller, S. C. (2020). Plant traits rather than species richness explain ecological processes in subtropical forests. Ecosystems 23, 52–66. doi: 10.1007/s10021-019-00386-6
Roumet, C., Birouste, M., Picon-Cochard, C., Ghestem, M., Osman, N., Vrignon-Brenas, S., et al. (2016). Root structure-function relationships in 74 species: evidence of a root economics spectrum related to carbon economy. New Phytol. 210, 815–826. doi: 10.1111/nph.13828
Sardar, M. F., Younas, F., Farooqi, Z. U. R., Li, Y. L. (2023). Soil nitrogen dynamics in natural forest ecosystem: a review. Front. For. Glob. Change 6. doi: 10.3389/ffgc.2023.1144930
Sasaki, T., Okubo, S., Okayasu, T., Jamsran, U., Ohkuro, T., Takeuchi, K. (2009). Two-phase functional redundancy in plant communities along a grazing gradient in Mongolian rangelands. Ecology 90, 2598–2608. doi: 10.1890/08-1850.1
Schleuter, D., Daufresne, M., Massol, F., Argillier, C. (2010). A user’s guide to functional diversity indices. Ecol. Monogr. 80, 469–484. doi: 10.1890/08-2225.1
Shabaan, M., Asghar, H. N., Zahir, Z. A., Sardar, M. F., Parveen, R., Ali, F. Q. (2022). Halotolerant rhizobacterial consortium confers salt tolerance to maize under naturally salt-affected soil. Soil Sci. Soc Am. J. 86, 1264–1279. doi: 10.1002/saj2.20438
Sharafatmandrad, M., Mashizi, K. A. (2020). Investigating distribution of ecosystem services in rangeland landscapes: an approach based on weighted key functional traits. Ecol. Indic. 111, 105971. doi: 10.1016/j.ecolind.2019.105971
Shen, H., Dong, S. K., Li, S., Xiao, J. N., Han, Y. H., Yang, M. Y., et al. (2019). Grazing enhances plant photosynthetic capacity by altering soil nitrogen in alpine grasslands on the Qinghai-Tibetan plateau. Agriculture Ecosyst. Environ. 280, 161–168. doi: 10.1016/j.agee.2019.04.029
Sigdel, S. R., Liang, E. Y., Rokaya, M. B., Rai, S., Dyola, N., Sun, J., et al. (2022). Functional traits of a plant species fingerprint ecosystem productivity along broad elevational gradients in the Himalayas. Funct. Ecol. 37, 383–394. doi: 10.1111/1365-2435.14226
Siwicka, E., Thrush, S. F., Hewitt, J. E. (2020). Linking changes in species-trait relationships and ecosystem function using a network analysis of traits. Ecol. Appl. 30, e02010. doi: 10.1002/eap.2010
Song, S. Z., Xiong, K. N., Chi, Y. K., He, C., Fang, J. Z., He, S. Y. (2022). Effect of cultivated pastures on soil bacterial communities in the karst rocky desertification area. Front. Microbiol. 13. doi: 10.3389/fmicb.2022.922989
Su, L., Du, H., Zeng, F. P., Peng, W. X., Rizwan, M., Núñez-Delgado, A., et al. (2019). Soil and fine roots ecological stoichiometry in different vegetation restoration stages in a karst area, southwest China. J. Environ. Manage. 252, 109694. doi: 10.1016/j.jenvman.2019.109694
Sweeney, C. J., Vries, F. T. D., Dongen, B. E. V., Bardgett, R. D. (2020). Root traits explain rhizosphere fungal community composition among temperate grassland plant species. New Phytol. 229, 1492–1507. doi: 10.1111/1365-2664.12727
Teng, Q. M., Lu, X. N., Zhang, Q. Q., Cai, L. L., Sardar, M. F., Li, Y. F., et al. (2023). Litterfall quality modulates soil ammonium and nitrate supply through altering microbial function in bamboo encroachment of broadleaf forests. Geoderma 437, 116592. doi: 10.1016/j.geoderma.2023.116592
Tilman, D., Knops, J., Wedin, D., Reich, P., Ritchie, M., Siemann, E. (1997). The influence of functional diversity and composition on ecosystem processes. Science 277, 1300–1302. doi: 10.1126/science.277.5330.1300
Vile, D., Shiple, B., Garnier, E. (2006). Ecosystem productivity can be predicted from potential relative growth rate and species abundance. Ecol. Lett. 9, 1061–1067. doi: 10.1111/j.1461-0248.2006.00958.x
Villéger, S., Mason, N. W. H., Mouillot, D. (2008). New multidimensional functional diversity indices for a multifaceted framework in functional ecology. Ecology 89, 2290–2301. doi: 10.1890/07-1206.1
Violle, C., Navas, M. L., Vile, D., Kazakou, E., Fortunel, C., Hummel, I., et al. (2007). Let the concept of trait be functional! Oikos 116, 882–892. doi: 10.1111/j.0030-1299.2007.15559.x
Wagg, C., Bender, S. F., Widmer, F., Heijden, M. G. A. V. D. (2014). Soil biodiversity and soil community composition determine ecosystem multifunctionality. Proc. Natl. Acad. Sci. United States America 111, 5266–5270. doi: 10.1073/pnas.1320054111
Wang, Y., Chen, J., Zhang, L. M., Feng, L., Yan, L. B., Li, F. B., et al. (2022a). Relationship between diversity and stability of a karst plant community. Ecol. Evol. 12, e9254. doi: 10.1002/ece3.9254
Wang, Q., Rong, L., Wang, M. J., Ye, T. M., Li, T. T., Yang, W. S., et al. (2022). Response of leaf functional traits of woody plants to degraded karst soil in central Guizhou. Earth Environ. 50, 639–647. doi: 10.14050/j.cnki.1672-9250.2022.50.102
Wang, D. J., Xu, Z. H., Blumfield, T. J., Zalucki, J. (2020). The potential of using 15N natural abundance in changing ammonium-N and nitrate-N pools for studying in situ soil N transformations. J. Soils Sediments 20, 1323–1331. doi: 10.1007/s11368-019-02478-1
Wang, K. L., Yue, Y. M., Chen, H. S., Zeng, F. P. (2020). Mechanisms and realization pathways for integration of scientific poverty alleviation and ecosystem services enhancement. Bull. Chin. Acad. Sci. 35, 1264–1272. doi: 10.16418/j.issn.1000-3045.20200729002
Wang, Y., Zhang, L., Chen, J., Feng, L., Li, F., Yu, L. (2022b). Functional diversity of plant communities in relationship to leaf and soil stoichiometry in karst areas of southwest China. Forests 13, 864. doi: 10.3390/f13060864
Wang, Y. Q., Zhang, X. C., Huang, C. Q. (2019). Spatial variability of soil total nitrogen and soil total phosphorus under different land uses in a small watershed on the Loess Plateau, China. Geoderma 150, 141–149. doi: 10.1016/j.geoderma.2009.01.021
Weng, E. S., Farrior, C. E., Dybzinski, R., Pacala, S. W. (2017). Predicting vegetation type through physiological and environmental interactions with leaf traits: Evergreen and deciduous forests in an earth system modeling framework. Global Change Biol. 23, 2482–2498. doi: 10.1111/gcb.13542
Wright, I. J., Reich, P. B., Westoby, M., Villar, R. (2004). The worldwide leaf economics spectrum. Nature 428, 821–827. doi: 10.1038/nature02403
Wu, S. N., Zhang, X., Gao, X. X., Xu, Y. D., Wu, X. H., Shan, X. K., et al. (2019). Succession dynamics of a plant community of degraded alpine meadow during the human-induced restoration process in the Three Rivers Source region. Acta Ecologica Sin. 39, 2444–2453. doi: 10.5846/stxb201804070783
Wu, J. X., Zhao, Y., Yu, C. Q., Luo, L. M., Pan, Y. (2017). Land management influences trade-offs and the total supply of ecosystem services in alpine grassland in Tibet, China. J. Environ. Manage. 193, 70–78. doi: 10.1016/j.jenvman.2017.02.008
Xi, X. Q., Zhao, Y. J., Liu, Y. G., Wang, X., Gao, X. M. (2011). Variation and correlation of plant functional traits in karst area of central Guizhou Province, China. Chin. J. Plant Ecol. 35, 1000–1008. doi: 10.3724/SP.J.1258.2011.01000
Xiao, J., Xiong, K. N. (2022). A review of agroforestry ecosystem services and its enlightenment on the ecosystem improvement of rocky desertification control. Sci. Total Environ. 856, 158538. doi: 10.1016/j.scitotenv.2022.158538
Xing, Y. F., Wang, X. L., Liu, Y. Q., Hua, R., Wang, C., Wu, J. L., et al. (2020). Characteristics of plant community and soil organic carbon and nitrogen in artificial grassland with different establishment years. Acta Agrestia Sin. 28, 521–528. doi: 10.11733/j.issn.1007-0435.2020.02.028
Xiong, K. N., Li, J., Long, M. Z. (2012). Features of soil and water loss and key issues in demonstration areas for combating karst rocky desertification. Acta Geologica Sin. 67, 878–888.
Xiong, K. N., Li, P., Zhou, Z. F., An, Y. L., Lv, T., Lan, A. J. (2002). Remote sensing of karst rocky desertification: a typical GIS study—with a special reference to Guizhou Province (Beijing Geological Press).
Xiong, K. N., Xiao, J., Zhu, D. Y. (2022). Research progress of agroforestry ecosystem services and its implications for industrial revitalization in karst regions. Acta Ecologica Sin. 42, 851–861. doi: 10.5846/stxb202101080090
Xu, T. W., Zhao, X. Q., Zhang, X. L., Xu, S. X. (2020). Sustainable development of ecological grass-based livestock husbandry in Qinghai-Tibet Plateau alpine area: principle, technology and practice. Acta Ecologica Sin. 40, 6324–6337. doi: 10.5846/stxb201912142705
Yan, P., He, N. P., Yu, K. L., Xu, L., Meerbeek, K. V. (2023). Integrating multiple plant functional traits to predict ecosystem productivity. Commun. Biol. 6, 239. doi: 10.1038/s42003-023-04626-3
Yang, S. T., Li, C. J., Lou, H. Z., Wang, P. F., Wu, X. J., Zhang, Y. C., et al. (2021). Role of the countryside landscapes for sustaining biodiversity in karst areas at a semi centennial scale. Ecol. Indic. 123, 107315. doi: 10.1016/j.ecolind.2020.107315
Yang, J., Xu, X., Liu, M., Xu, C., Luo, W., Song, T., et al. (2016). Effects of Napier grass management on soil hydrologic functions in a karst landscape, southwestern China. Soil Tillage Res. 157, 83–92. doi: 10.1016/j.still.2015.11.012
Yang, J., Xu, X., Liu, M., Xu, C., Zhang, Y., Luo, W., et al. (2017). Effects of “Grain for Green” program on soil hydrologic functions in karst landscapes, southwestern China. Agriculture Ecosyst. Environ. 247, 120–129. doi: 10.1016/j.agee.2017.06.025
Yang, C., Zhang, Y. X., Zhang, H., Zheng, C. Y., Zhu, F. (2022). Recent advances in understanding the ecosystem functioning of diverse forage mixtures. Acta Prataculturae Sin. 31, 206–219. doi: 10.11686/cyxb2021341
Zhang, Y. (2011). Physicochemical analysis of soil, water and plant (Beijing, China: Forestry Publishing House).
Zhang, L., Cappelli, S. L., Huang, M. J., Liu, X., Xiao, Y., Cardou, F., et al. (2023). Intraspecific trait variability mediates the effect of nitrogen addition and warming on aboveground productivity. Oikos (2023), 34: e09559. doi: 10.1111/oik.09559
Zhang, J., Chen, H. S., Fu, Z. Y., Wang, K. L. (2021). Effects of vegetation restoration on soil properties along an elevation gradient in the karst region of southwest China. Agriculture Ecosyst. Environ. 320, 107572. doi: 10.1016/j.agee.2021.107572
Zhang, Z. H., Hou, J. H., He, N. P. (2021). Predictability of functional diversity depends on the number of traits. J. Resour. Ecol. 12, 332–345. doi: 10.5814/j.issn.1674-764x.2021.03.003
Zhang, H. M., Li, X. L., Li, L. P., Zhang, J. (2020). Effects of species combination on community diversity and productivity of alpine artificial grassland. Acta Agrestia Sin. 28, 1436–1443. doi: 10.11733/j.issn.1007-0435.2020.05.031
Zhang, M. Y., Wang, K. L., Liu, H. Y., Zhang, C. H., Yue, Y. M., Qi, X. K. (2018). Effect of ecological engineering projects on ecosystem services in a karst region: A case study of northwest Guangxi, China. J. Cleaner Production 183, 831–842. doi: 10.1016/j.jclepro.2018.02.102
Zhang, S. H., Xiong, K. N., Deng, X. H., Kong, L. W., Min, X. Y. (2023). Impact of ecological restoration on ecosystem service trade-offs: Insight from karst desertification control. Land Degradation Dev. 34. doi: 10.1002/ldr.4643
Zhang, Y., Xu, X., Li, Z., Liu, M., Xu, C., Zhang, R., et al. (2019). Effects of vegetation restoration on soil quality in degraded karst landscapes of southwest China. Sci. Total Environ. 650, 2657–2665. doi: 10.1016/j.scitotenv.2018.09.372
Zhang, Y. H., Xu, X. L., Li, Z. W., Xu, C. C., Luo, W. (2021). Improvements in soil quality with vegetation succession in subtropical China karst. Sci. Total Environ. 775, 145876. doi: 10.1016/j.scitotenv.2021.145876
Zhang, S. H., Zhang, Y., Xiong, K. N., Yu, Y. H., Min, X. Y. (2020). Changes of leaf functional traits in karst rocky desertification ecological environment and the driving factors. Global Ecol. Conserv. 24, e01381. doi: 10.1016/j.gecco.2020.e01381
Zhang, J., Zuo, X. A., Lv, P., Yue, X. Y., Zhang, J. (2018). Functional traits and interrelations of dominant plant species on typical grassland in the Horqin Sandy Land, China. Arid Zone Res. 35, 137–143. doi: 10.13866/j.azr.2018.01.17
Zhong, Q. L., Liu, L. B., Xu, X., Yang, Y., Guo, Y. M., Xu, H. Y., et al. (2018). Variations of plant functional traits and adaptive strategy of woody species in a karst forest of central Guizhou Province, southwestern China. Chin. J. Plant Ecol. 42, 562–572. doi: 10.17521/cjpe.2017.0270
Zhou, H., Xu, X. L., Jiang, X., Ding, B., Wu, P., Ding, F. J. (2022). Plant functional trait responses to dolomite and limestone karst forests in southwest China. Forests 13, 2187. doi: 10.3390/f13122187
Zirbel, C. R., Bassett, T., Grman, E., Brudvig, L. A. (2017). Plant functional traits and environmental conditions shape community assembly and ecosystem functioning during restoration. J. Appl. Ecol. 54, 1070–1079. doi: 10.1111/1365-2664.12885
Keywords: plant diversity, functional trait, ecosystem function, ecological restoration, karst desertification
Citation: Song S, Xiong K and Chi Y (2023) Response of grassland ecosystem function to plant functional traits under different vegetation restoration models in areas of karst desertification. Front. Plant Sci. 14:1239190. doi: 10.3389/fpls.2023.1239190
Received: 13 June 2023; Accepted: 20 November 2023;
Published: 12 December 2023.
Edited by:
Manuel Enrique Figueroa, Sevilla University, SpainReviewed by:
Changcheng Liu, North University of China, ChinaCopyright © 2023 Song, Xiong and Chi. This is an open-access article distributed under the terms of the Creative Commons Attribution License (CC BY). The use, distribution or reproduction in other forums is permitted, provided the original author(s) and the copyright owner(s) are credited and that the original publication in this journal is cited, in accordance with accepted academic practice. No use, distribution or reproduction is permitted which does not comply with these terms.
*Correspondence: Kangning Xiong, eGlvbmdrbkBnem51LmVkdS5jbg==
Disclaimer: All claims expressed in this article are solely those of the authors and do not necessarily represent those of their affiliated organizations, or those of the publisher, the editors and the reviewers. Any product that may be evaluated in this article or claim that may be made by its manufacturer is not guaranteed or endorsed by the publisher.
Research integrity at Frontiers
Learn more about the work of our research integrity team to safeguard the quality of each article we publish.